Construction and validation of a nomogram for predicting disease-free survival after radical resection of rectal cancer using perioperative inflammatory indicators
Highlight box
Key findings
• The nomogram constructed based on tumor-node-metastasis stage III, neural invasion, preoperative neutrophil-to-lymphocyte ratio ≥1.995, postoperative systemic immune-inflammation index ≥451.05, and Δpan-immune-inflammation value ≥144.36 can predict the risk of 3-year disease-free survival (DFS) in patients undergoing curative surgery for rectal cancer.
What is known and what is new?
• Inflammatory indicators serve as prognostic indicators for patients undergoing radical rectal cancer surgery.
• Comprehensively analyzed perioperative inflammatory indicators predicting DFS in patients undergoing radical rectal cancer surgery, and constructed a nomogram.
What is the implication, and what should change now?
• The nomogram can be used to predict the risk of 3-year DFS in patients after radical resection for rectal cancer, providing a more visual assessment of patients’ prognostic risk and thus offering strong support for the development of personalized treatment plans.
Introduction
Background
Rectal cancer is a common malignant tumor worldwide, and radical surgery is the primary treatment for rectal cancer (1). However, the prognosis of patients after surgery varies greatly, with some patients achieving good prognosis and others facing a high risk of metastasis and recurrence (2). Therefore, predicting patient prognosis is of great significance for clinicians to develop postoperative treatment plans and for patients to understand their condition.
Rationale and knowledge gap
Inflammation indicators are biological markers that reflect the immune and inflammatory status of the body. Studies have shown that inflammatory reactions play an important role in tumor occurrence, development, and metastasis, thereby affecting patient prognosis (3,4). Various studies have indicated that high levels of preoperative inflammation indicators suggest an increased risk of postoperative metastasis and recurrence in patients (5,6). In addition to preoperative inflammation indicators, postoperative inflammation indicators and perioperative indicator changes also have prognostic value (7). Because surgical stress can alter the body’s immune and inflammatory systems (8), the resection of the tumor itself can also change the patient’s inflammatory status, resulting in differences in postoperative systemic inflammation between individuals without cancer and those with cancer before surgery (9). Therefore, continuous monitoring of perioperative inflammation indicators is more predictive of postoperative survival risk than detecting inflammation indicators at a single time point during the perioperative period (10). However, comprehensive analysis and comparison of perioperative inflammation indicators regarding their prognostic value in radical surgery for rectal cancer have not been observed in current literature.
Nomograms are statistical prediction models that estimate the prognosis of individual patients by combining important prognostic factors. They have been established for various types of cancer and are superior to the single tumor-node-metastasis (TNM) staging system (11). Therefore, the nomogram has the potential to positively impact clinical practice. It can serve as an important reference for physicians in making clinical decisions: by summing up the scores of each risk factor, calculating the total score, and determining the probability of 3-year disease-free survival (DFS) for the corresponding patients postoperatively. The higher the total score, the greater the likelihood of poor prognosis, necessitating intensified monitoring, timely interventions to reduce recurrence, metastasis, or mortality, and prolonging patient survival. Simultaneously, it can assist healthcare institutions in more effectively allocating resources, prioritizing the management of high-risk patients, and lowering medical costs.
Objective
This study aims to analyze the prognostic value of perioperative inflammatory indicators in patients undergoing radical surgery for rectal cancer, and to assess the prognosis risk of patients more intuitively through the construction of nomogram, thus providing strong support for the formulation of individualized treatment plans. We present this article in accordance with the TRIPOD reporting checklist (available at https://jgo.amegroups.com/article/view/10.21037/jgo-23-977/rc).
Methods
General information
Retrospective analysis of clinical data for 304 patients who underwent laparoscopic radical resection for rectal cancer at the Affiliated Hospital of Xuzhou Medical University from May 1, 2018 to September 30, 2020. The data were divided into a training set (n=213) and a validation set (n=91) with a 7:3 ratio. Inclusion criteria: (I) patients with stage I–III rectal cancer who underwent laparoscopic radical resection; (II) pathologically confirmed rectal adenocarcinoma; and (III) age ≥18 years. Exclusion criteria: (I) currently using immunosuppressants, hormones, or other drugs that affect inflammatory responses; (II) severe infection, inflammation, or other complications; (III) received preoperative adjuvant therapy; (IV) positive surgical margins; and (V) other malignant tumors. The study was conducted in accordance with the Declaration of Helsinki (as revised in 2013). The study was approved by the ethics committee of the Affiliated Hospital of Xuzhou Medical University (No. XYFY2023-KL256-01) and the requirement for individual informed consent was waived for the retrospective analysis.
Study indicators
Study indicators include age, gender, postoperative adjuvant treatment, tumor location, tumor diameter, tumor differentiation degree, vascular invasion, neural invasion, anastomotic leaks, TNM stage, carcinoembryonic antigen (CEA), carbohydrate antigen 19-9 (CA19-9), and perioperative inflammatory indicators. Perioperative inflammatory indicators include 15 inflammation indicators such as preoperative neutrophil-to-lymphocyte ratio (NLR), postoperative NLR, ΔNLR, preoperative platelet-to-lymphocyte ratio (PLR), postoperative PLR, ΔPLR, preoperative lymphocyte-to-monocyte ratio (LMR), postoperative LMR, ΔLMR, preoperative systemic immune-inflammation index (SII), postoperative SII, ΔSII, preoperative pan-immune-inflammation value (PIV), postoperative PIV, and ΔPIV. Detailed information is provided in Table 1. Blood samples for preoperative inflammation indicators are collected within 7 days before surgery; postoperative blood samples are collected 21–56 days after surgery, before starting adjuvant treatment. This is the optimal period for evaluating postoperative inflammation indicators, as trauma and adjuvant chemotherapy after surgery can affect blood cell counts (12).
Table 1
Indicator | Calculation formula |
---|---|
Preoperative NLR | Preoperative neutrophils/lymphocytes |
Postoperative NLR | Postoperative neutrophils/lymphocytes |
ΔNLR | Postoperative NLR minus preoperative NLR |
Preoperative PLR | Preoperative platelets/lymphocytes |
Postoperative PLR | Postoperative platelets/lymphocytes |
ΔPLR | Postoperative PLR minus preoperative PLR |
Preoperative LMR | Preoperative lymphocytes/monocytes |
Postoperative LMR | Postoperative lymphocytes/monocytes |
ΔLMR | Postoperative LMR minus preoperative LMR |
Preoperative SII | Preoperative neutrophils × platelets/lymphocytes |
Postoperative SII | Postoperative neutrophils × platelets/lymphocytes |
ΔSII | Postoperative SII minus preoperative SII |
Preoperative PIV | Preoperative neutrophils × platelets × monocytes/lymphocytes |
Postoperative PIV | Postoperative neutrophils × platelets × monocytes/lymphocytes |
ΔPIV | Postoperative PIV minus preoperative PIV |
NLR, neutrophil-to-lymphocyte ratio; PLR, platelet-to-lymphocyte ratio; LMR, lymphocyte-to-monocyte ratio; SII, systemic immune-inflammation index; PIV, pan-immune-inflammation value.
Follow-up
Patients received follow-up visits every 3 months in the first 2 years after surgery, and then every 6 months for the next 3 to 5 years. Follow-up visits included tumor marker testing, chest computed tomography (CT), abdominal CT, and colonoscopy. The endpoint events were recorded based on these results, defined as postoperative recurrence, metastasis, or death. DFS was defined as the time from surgery to disease recurrence, metastasis, death, or the last follow-up visit. The last follow-up visit was on September 30th, 2023.
Statistical analysis
Application of SPSS 25 and R 4.3.1 analysis. Comparison of count data used Chi-square test. The optimal cutoff values for predicting DFS were determined by the maximum value of the Youden index calculated through the receiver operating characteristic (ROC) curve, and converted to binary variables. The least absolute shrinkage and selection operator (LASSO) regression and ten-fold cross-validation were used to screen all variables, and meaningful variables were used for multivariate Cox regression analysis to determine the independent predictive factors affecting DFS. A nomogram was established to predict the 3-year DFS risk based on the independent predictive factors. ROC curves and calibration curves were drawn to evaluate the discrimination and calibration of the predictive model. The clinical net benefit of the nomogram under different threshold probabilities was evaluated using decision curve analysis (DCA), and the internal validation of the nomogram was performed using the validation set. A P value of less than 0.05 was considered statistically significant.
Results
Best cut-off values for various inflammatory indicators in the training set patients
According to ROC curve analysis, the cut-off values for preoperative NLR, postoperative NLR, ΔNLR, preoperative PLR, postoperative PLR, ΔPLR, preoperative LMR, postoperative LMR, ΔLMR, preoperative SII, postoperative SII, ΔSII, preoperative PIV, postoperative PIV, and ΔPIV are 1.995, 1.81, 0.865, 148.44, 141.05, −2.24, 5.155, 4.76, 0.7, 487.89, 451.05, 68.22, 206.33, 199.23, and 144.36, respectively.
Patient baseline characteristics
A total of 304 patients were included in this study, with 213 patients in the training set and a 3-year DFS rate of 70% (149/213). There were 125 male patients and 88 female patients, with 123 patients aged ≥60 years and 90 patients aged <60 years. The validation set included 91 patients, with a 3-year DFS rate of 69.2% (63/91). There were 55 male patients and 36 female patients, with 62 patients aged ≥60 years and 29 patients aged <60 years. The training set and validation set were balanced and comparable in terms of various variables (Table 2).
Table 2
Variables | Training set (n=213) | Validation set (n=91) | χ2 value | P value |
---|---|---|---|---|
Age (years), n (%) | 2.887 | 0.09 | ||
<60 | 90 (42.3) | 29 (31.9) | ||
≥60 | 123 (57.7) | 62 (68.1) | ||
Gender, n (%) | 0.081 | 0.80 | ||
Female | 88 (41.3) | 36 (39.6) | ||
Male | 125 (58.7) | 55 (60.4) | ||
Postoperative adjuvant therapy, n (%) | 1.048 | 0.36 | ||
No | 78 (36.6) | 39 (42.9) | ||
Yes | 135 (63.4) | 52 (57.1) | ||
Primary tumor site, n (%) | 3.502 | 0.07 | ||
Upper | 83 (39.0) | 46 (50.5) | ||
Middle or low | 130 (61.0) | 45 (49.5) | ||
Tumor size (cm), n (%) | 2.209 | 0.15 | ||
<5 | 151 (70.9) | 72 (79.1) | ||
≥5 | 62 (29.1) | 19 (20.9) | ||
Degree of differentiation, n (%) | 1.071 | 0.32 | ||
Well or moderately | 179 (84.0) | 72 (79.1) | ||
Poorly | 34 (16.0) | 19 (20.9) | ||
Presence of vascular invasion, n (%) | 0.143 | 0.76 | ||
Negative | 168 (78.9) | 70 (76.9) | ||
Positive | 45 (21.1) | 21 (23.1) | ||
Presence of neural invasion, n (%) | 1.224 | 0.28 | ||
Negative | 166 (77.9) | 76 (83.5) | ||
Positive | 47 (22.1) | 15 (16.5) | ||
Anastomotic leaks, n (%) | 0.055 | 0.82 | ||
No | 196 (92.0) | 83 (91.2) | ||
Yes | 17 (8.0) | 8 (8.8) | ||
TNM stage, n (%) | 0.443 | 0.79 | ||
I | 59 (27.7) | 28 (30.8) | ||
II | 68 (31.9) | 26 (28.6) | ||
III | 86 (40.4) | 37 (40.7) | ||
CEA, n (%) | 0.053 | 0.89 | ||
<5 ng/mL | 141 (66.2) | 59 (64.8) | ||
≥5 ng/mL | 72 (33.8) | 32 (35.2) | ||
CA19-9, n (%) | 1.255 | 0.33 | ||
<35 U/mL | 190 (89.2) | 77 (84.6) | ||
≥35 U/mL | 23 (10.8) | 14 (15.4) | ||
Preoperative NLR, n (%) | 0.016 | >0.99 | ||
<1.995 | 106 (49.8) | 46 (50.5) | ||
≥1.995 | 107 (50.2) | 45 (49.5) | ||
Postoperative NLR, n (%) | 0.56 | 0.52 | ||
<1.81 | 87 (40.8) | 33 (36.3) | ||
≥1.81 | 126 (59.2) | 58 (63.7) | ||
ΔNLR, n (%) | 3.29 | 0.08 | ||
<0.865 | 164 (77.0) | 61 (67.0) | ||
≥0.865 | 49 (23.0) | 30 (33.0) | ||
Preoperative PLR, n (%) | 3.075 | 0.09 | ||
<148.44 | 133 (62.4) | 47 (51.6) | ||
≥148.44 | 80 (37.6) | 44 (48.4) | ||
Postoperative PLR, n (%) | 0.361 | 0.61 | ||
<141.05 | 95 (44.6) | 44 (48.4) | ||
≥141.05 | 118 (55.4) | 47 (51.6) | ||
ΔPLR, n (%) | 0.078 | 0.79 | ||
<−2.24 | 76 (35.7) | 34 (37.4) | ||
≥−2.24 | 137 (64.3) | 57 (62.6) | ||
Preoperative LMR, n (%) | 0.108 | 0.80 | ||
<5.155 | 98 (46.0) | 40 (44.0) | ||
≥5.155 | 115 (54.0) | 51 (56.0) | ||
Postoperative LMR, n (%) | 3.443 | 0.07 | ||
<4.76 | 97 (45.5) | 31 (34.1) | ||
≥4.76 | 116 (54.5) | 60 (65.9) | ||
ΔLMR, n (%) | 0.846 | 0.38 | ||
<0.7 | 55 (25.8) | 19 (20.9) | ||
≥0.7 | 158 (74.2) | 72 (79.1) | ||
Preoperative SII, n (%) | 0.504 | 0.52 | ||
<487.89 | 117 (54.9) | 54 (59.3) | ||
≥487.89 | 96 (45.1) | 37 (40.7) | ||
Postoperative SII, n (%) | 0.017 | 0.90 | ||
<451.05 | 93 (43.7) | 39 (42.9) | ||
≥451.05 | 120 (56.3) | 52 (57.1) | ||
ΔSII, n (%) | 1.219 | 0.31 | ||
<68.22 | 120 (56.3) | 45 (49.5) | ||
≥68.22 | 93 (43.7) | 46 (50.5) | ||
Preoperative PIV, n (%) | 0.235 | 0.69 | ||
<206.33 | 139 (65.3) | 62 (68.1) | ||
≥206.33 | 74 (34.7) | 29 (31.9) | ||
Postoperative PIV, n (%) | 0.3 | 0.90 | ||
<199.23 | 117 (54.9) | 49 (53.8) | ||
≥199.23 | 96 (45.1) | 42 (46.2) | ||
ΔPIV, n (%) | 0.37 | 0.55 | ||
<144.36 | 166 (77.9) | 68 (74.7) | ||
≥144.36 | 47 (22.1) | 23 (25.3) |
TNM, tumor-node-metastasis; CEA, carcinoembryonic antigen; CA19-9, carbohydrate antigen 19-9; NLR, neutrophil-to-lymphocyte ratio; PLR, platelet-to-lymphocyte ratio; LMR, lymphocyte-to-monocyte ratio; SII, systemic immune-inflammation index; PIV, pan-immune-inflammation value.
Establishing LASSO-Cox regression model
Using LASSO regression and ten-fold cross-validation to select lambda.1se as the optimal lambda value, ensuring a good fit while incorporating the minimum number of variables, resulting in the most parsimonious predictive model. Finally, six variables were selected, including TNM stage III, neural invasion, preoperative NLR ≥1.995, postoperative SII ≥451.05, postoperative PIV ≥199.23, and ΔPIV ≥144.36 (Figure 1).
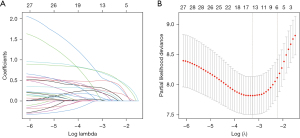
Multifactor Cox regression analysis was performed with independent variables TNM, neural invasion, preoperative NLR, postoperative SII, postoperative PIV, and ΔPIV. The results showed that TNM stage III [hazard ratio (HR) =2.572; 95% confidence interval (CI): 1.529–4.327; P<0.001], neural invasion (HR =2.487; 95% CI: 1.491–4.148; P<0.001), preoperative NLR ≥1.995 (HR =1.845; 95% CI: 1.07–3.183; P=0.02), postoperative SII ≥451.05 (HR =2.687; 95% CI: 1.121–6.438; P=0.02), and ΔPIV ≥144.36 (HR =2.053; 95% CI: 1.139–3.7; P=0.01) were independent predictive factors affecting DFS in patients undergoing rectal cancer radical surgery (Table 3).
Table 3
Variables | HR (95% CI) | P value |
---|---|---|
TNM III | 2.572 (1.529, 4.327) | <0.001 |
Neural invasion | 2.487 (1.491, 4.148) | <0.001 |
Preoperative NLR ≥1.995 | 1.845 (1.07, 3.183) | 0.02 |
Postoperative SII ≥451.05 | 2.687 (1.121, 6.438) | 0.02 |
Postoperative PIV ≥199.23 | 1.531 (0.714, 3.282) | 0.27 |
ΔPIV ≥144.36 | 2.053 (1.139, 3.7) | 0.01 |
DFS, disease-free survival; HR, hazard ratio; CI, confidence interval; TNM, tumor-node-metastasis; NLR, neutrophil-to-lymphocyte ratio; SII, systemic immune-inflammation index; PIV, pan-immune-inflammation value.
Constructing a nomogram
We constructed a nomogram for predicting 3-year DFS following radical resection of rectal cancer using five variables: TNM stage III, neural invasion, preoperative NLR ≥1.995, postoperative SII ≥451.05, and ΔPIV ≥144.36 (Figure 2). By adding the corresponding scores for each variable, the total score corresponds to the probability of 3-year DFS after surgery. A higher total score indicates a lower 3-year DFS rate.
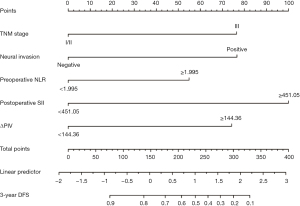
Validation of the nomogram
The internal validation of the nomogram was conducted using the validation set. The results showed that the area under the ROC curve (AUC) of the nomogram was 0.811 (95% CI: 0.778–0.889) for the training set and 0.808 (95% CI: 0.785–0.942) for the validation set, indicating that the nomogram had high discriminatory ability in predicting 3-year DFS after radical resection for rectal cancer (Figure 3). The calibration curve showed that the predicted curve was close to the actual curve, indicating good consistency between the predicted probability by the nomogram and the actual results obtained through pathological biopsy (Figure 4). The DCA results showed that when the threshold probability of the patient’s outcome event was >10%, using the nomogram to predict the 3-year DFS of patients after radical resection for rectal cancer had a significantly higher net benefit than implementing interventions for all patients or no patients (Figure 5), indicating that the model had high clinical practical value.
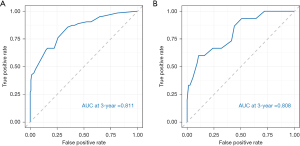
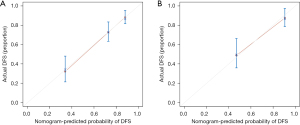
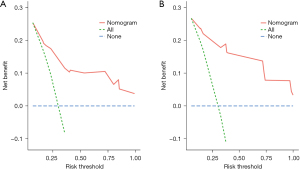
Discussion
Key findings
This study employed LASSO-Cox regression analysis to identify risk factors for DFS after curative resection of rectal cancer. The risk factors identified were TNM stage III, neural invasion, preoperative NLR ≥1.995, postoperative SII ≥451.05, and ΔPIV ≥144.36. Additionally, a prognostic prediction model based on perioperative inflammatory markers was established to predict the 3-year DFS in patients after curative resection of rectal cancer. Furthermore, the ROC curve, calibration curve, and DCA for both the training and validation sets demonstrated excellent predictive performance of the proposed model, indicating its potential application in clinical practice.
Strengths
The prognosis of cancer patients depends not only on factors such as tumor staging and pathological characteristics (13), but also closely relates to their immune status. Inflammation markers can reflect the immune state of the body and are closely associated with the occurrence, development, and prognosis of tumors. Meanwhile, tumors can also induce inflammation, creating a complex and multifaceted relationship (14). Although surgery is the most common and crucial treatment for cancer, it can lead to immune suppression and the release of pro-inflammatory factors, resulting in poor prognosis (15). Therefore, it is of great significance to explore not only preoperative indicators but also postoperative and perioperative indicator changes for predicting the prognosis of rectal cancer. Continuous monitoring of perioperative inflammation markers can better reflect the prognosis of surgical patients.
Previous studies have established predictive models for the prognostic value of preoperative inflammatory indicators on colorectal cancer radical surgery outcomes. Building on this foundation, we innovatively incorporated postoperative inflammatory indicators and perioperative indicator changes, effectively expanding upon existing research. Through the analysis of perioperative inflammatory indicators, we further revealed the role of inflammation in rectal cancer radical surgery outcomes. Moreover, we applied machine learning algorithms to screen variables, with LASSO regression preventing model overfitting and collinearity issues, yielding the most valuable inflammatory indicators (16). As a visualization tool, the nomogram enables clinical physicians to intuitively display predictive model results, providing more accurate decision-making criteria. Notably, our nomogram exhibits superior predictive capabilities with an AUC of 0.811, surpassing that of Memorial Sloan Kettering Cancer Center and AJCC TNM staging (with AUCs of 0.79 and 0.74, respectively) (17).
Explanations of findings and comparison with similar research
TNM staging is a commonly used method for cancer staging, which evaluates the severity and spread of tumors. The higher the stage, the greater the severity of the tumor and the difficulty of treatment. Therefore, TNM staging is applied in many predictive nomograms for colorectal cancer prognosis (18,19). Neural invasion refers to tumor cells invading the nerve sheath or the presence of tumor cells within the nerve sheath, which is associated with tumor invasion, metastasis, cancer-related pain, and poor clinical outcomes. The New England Journal of Medicine has also considered neural invasion as pathological evidence of early metastasis in colorectal cancer, being independently associated with low survival rates (20). This study confirmed that TNM stage III and neural invasion are independent risk factors for DFS in patients after radical rectal cancer surgery, and incorporated them into the predictive model.
Preoperative NLR has been identified as an important biomarker for patients with rectal cancer, and multiple studies have confirmed its close association with poor prognosis. Hamid et al. evaluated 31 studies, including 7,553 patients, and demonstrated that high preoperative NLR is independently associated with inferior DFS in rectal cancer patients undergoing curative surgical treatment, surpassing the predictive value of preoperative PLR (21). Portale et al. analyzed a larger cohort of patients (over 14,000 individuals) and obtained consistent results, showing that higher preoperative NLR is associated with worse DFS in patients undergoing curative surgery for rectal cancer (22). These findings suggest that preoperative NLR can serve as an important prognostic indicator for rectal cancer patients, and patients with elevated preoperative NLR levels may require more aggressive treatment strategies.
Our study also found that postoperative SII is a strong predictor of DFS in patients after radical resection for rectal cancer, which is consistent with the findings of Zhou et al., who discovered that higher postoperative SII in colorectal cancer patients is associated with shorter survival (23). In addition, Qi et al. found that postoperative SII aids in the early prediction of anastomotic leakage after rectal cancer surgery (24), which has a negative impact on local recurrence and survival rates after rectal cancer surgery, and early prediction and timely treatment can improve patient outcomes (25), indicating that postoperative SII has certain predictive value for the prognosis of rectal cancer patients.
PIV is a recently developed inflammation marker based on the data sets of Valentino and TRIBE phase-one trials. Corti et al. found that high baseline PIV and high ΔPIV (PIV after 3/4 weeks of treatment minus baseline PIV) were adverse prognostic factors in patients with metastatic colorectal cancer receiving immunosuppressive therapy, and were independently associated with an increased risk of early disease progression (26). Subsequently, Sato et al. demonstrated that preoperative PIV could also be applied to predict surgical outcomes in I–III stage colorectal cancer patients undergoing surgery (27). This study reveals that high ΔPIV is an independent risk factor for DFS in patients undergoing curative rectal cancer surgery, indicating that it may serve as a reference indicator for monitoring the disease progression in rectal cancer patients.
Implications and actions needed
Our model has been proven to have high value in predicting 3-year DFS after rectal cancer radical resection. This model may assist clinicians in evaluating patients’ prognosis. However, there are certain limitations to this study: (I) it is a single-center, retrospective study, which may lead to statistical bias; our results require confirmation through multi-center, prospective studies; (II) only one-time pre- and post-operative inflammation index data were analyzed, without long-term continuous monitoring of inflammation indices; the value of dynamic changes in inflammation indices on prognosis needs further study; (III) the cut-off values for inflammation indices vary in each study; larger sample and multi-center research is needed to determine the optimal cut-off values for establishing better predictive models; and (IV) neoadjuvant therapy exerts a significant impact on inflammatory markers (28). To mitigate this influence, we opted to exclude patients who underwent neoadjuvant treatment. Naturally, this decision may affect the generalizability of the results. Subsequent studies could delve deeper into the variations of perioperative inflammatory markers under diverse treatment strategies to offer a more comprehensive understanding.
Conclusions
In conclusion, this study constructs an intuitive predictive nomogram for 3-year DFS after radical resection of rectal cancer based on five indicators: TNM stage III, neural invasion, preoperative NLR ≥1.995, postoperative SII ≥451.05, and ΔPIV ≥144.36. The nomogram exhibits high accuracy and clinical practical value, enabling the identification of high-risk patients and aiding clinical physicians in implementing timely and effective interventions.
Acknowledgments
Funding: None.
Footnote
Reporting Checklist: The authors have completed the TRIPOD reporting checklist. Available at https://jgo.amegroups.com/article/view/10.21037/jgo-23-977/rc
Data Sharing Statement: Available at https://jgo.amegroups.com/article/view/10.21037/jgo-23-977/dss
Peer Review File: Available at https://jgo.amegroups.com/article/view/10.21037/jgo-23-977/prf
Conflicts of Interest: All authors have completed the ICMJE uniform disclosure form (available at https://jgo.amegroups.com/article/view/10.21037/jgo-23-977/coif). The authors have no conflicts of interest to declare.
Ethical Statement: The authors are accountable for all aspects of the work in ensuring that questions related to the accuracy or integrity of any part of the work are appropriately investigated and resolved. The study was conducted in accordance with the Declaration of Helsinki (as revised in 2013). The study was approved by the ethics committee of the Affiliated Hospital of Xuzhou Medical University (No. XYFY2023-KL256-01) and the requirement for individual informed consent was waived for the retrospective analysis.
Open Access Statement: This is an Open Access article distributed in accordance with the Creative Commons Attribution-NonCommercial-NoDerivs 4.0 International License (CC BY-NC-ND 4.0), which permits the non-commercial replication and distribution of the article with the strict proviso that no changes or edits are made and the original work is properly cited (including links to both the formal publication through the relevant DOI and the license). See: https://creativecommons.org/licenses/by-nc-nd/4.0/.
References
- Feng L, Xu R, Lin L, et al. Effect of the systemic immune-inflammation index on postoperative complications and the long-term prognosis of patients with colorectal cancer: a retrospective cohort study. J Gastrointest Oncol 2022;13:2333-9. [Crossref] [PubMed]
- Zhou J, Yu W, Xia J, et al. Not all Rectal Cancer Patients Could Benefit From the Surgery on the Primary Site. Cancer Control 2023;30:10732748231180056. [Crossref] [PubMed]
- Sato R, Oikawa M, Kakita T, et al. A decreased preoperative platelet-to-lymphocyte ratio, systemic immune-inflammation index, and pan-immune-inflammation value are associated with the poorer survival of patients with a stent inserted as a bridge to curative surgery for obstructive colorectal cancer. Surg Today 2023;53:409-19. [Crossref] [PubMed]
- Robinson TP, Hamidi T, Counts B, et al. The impact of inflammation and acute phase activation in cancer cachexia. Front Immunol 2023;14:1207746. [Crossref] [PubMed]
- Yang W, Zheng X, Wu M, et al. Development and validation of postoperative and preoperative platelets ratio (PPR) to predict the prognosis of patients undergoing surgery for colorectal cancer: A dual-center retrospective cohort study. Cancer Med 2023;12:111-21. [Crossref] [PubMed]
- Borella F, Bertero L, Valabrega G, et al. Searching for prognostic markers for Stage I epithelial ovarian cancer: A role for systemic inflammatory markers. Int J Gynaecol Obstet 2024;164:262-9. [Crossref] [PubMed]
- Schoenberg MB, Han Y, Li X, et al. Dynamics of Peripheral Blood Immune Cells during the Perioperative Period after Digestive System Resections: A Systematic Analysis of the Literature. J Clin Med 2023;12:718. [Crossref] [PubMed]
- Hosseini SV, Maleknejad A, Salem SA, et al. The pre- and postoperative neutrophil-to-lymphocyte and platelet-to-lymphocyte ratios: The comparison of laparoscopy and laparotomy in colorectal cancer patients. Asian J Endosc Surg 2022;15:44-50. [Crossref] [PubMed]
- Matsui Y, Matsuda A, Maejima A, et al. The clinical significance of perioperative inflammatory index as a prognostic factor for patients with retroperitoneal soft tissue sarcoma. Int J Clin Oncol 2022;27:1093-100. [Crossref] [PubMed]
- Kim JH, Lee JH, Lee HS, et al. Elevated Neutrophil-to-Lymphocyte Ratio in Perioperative Periods is Suggestive of Poor Prognosis in Patients with Colorectal Cancer. J Inflamm Res 2021;14:4457-66. [Crossref] [PubMed]
- Wu J, Lu L, Chen H, et al. Prognostic nomogram to predict the overall survival of patients with early-onset colorectal cancer: a population-based analysis. Int J Colorectal Dis 2021;36:1981-93. [Crossref] [PubMed]
- Chan JCY, Diakos CI, Chan DLH, et al. A Longitudinal Investigation of Inflammatory Markers in Colorectal Cancer Patients Perioperatively Demonstrates Benefit in Serial Remeasurement. Ann Surg 2018;267:1119-25. [Crossref] [PubMed]
- Cohen R, Shi Q, Meyers J, et al. Combining tumor deposits with the number of lymph node metastases to improve the prognostic accuracy in stage III colon cancer: a post hoc analysis of the CALGB/SWOG 80702 phase III study (Alliance) Ann Oncol 2021;32:1267-75. [Crossref] [PubMed]
- Shen LF, Wang QY, Yu Q. The Systemic Immune-Inflammation Index and Albumin as Prognostic Predictors in Laryngeal Carcinoma. Nutr Cancer 2021;73:1916-23. [Crossref] [PubMed]
- Tang G, Zhang L, Huang W, et al. Could Immunonutrition Help in the Fight against COVID-19 in Cancer Patient? Nutr Cancer 2022;74:1203-12. [Crossref] [PubMed]
- Liu Y, Sheng L, Hua H, et al. An Externally Validated Nomogram for Predicting the Overall Survival of Patients With Diffuse Large B-Cell Lymphoma Based on Clinical Characteristics and Systemic Inflammatory Markers. Technol Cancer Res Treat 2023;22:15330338231180785. [Crossref] [PubMed]
- Mahjoub M, Sioson L, Sheen A, et al. Predicting survival in colorectal carcinoma after curative resection: a new prognostic nomogram. Pathology 2022;54:79-86. [Crossref] [PubMed]
- Cai X, Chen F, Liang L, et al. A novel inflammation-related prognostic biomarker for predicting the disease-free survival of patients with colorectal cancer. World J Surg Oncol 2022;20:79. [Crossref] [PubMed]
- Ruan GT, Song MM, Zhang KP, et al. A novel nutrition-related nomogram for the survival prediction of colorectal cancer-results from a multicenter study. Nutr Metab (Lond) 2023;20:2. [Crossref] [PubMed]
- Wang H, Huo R, He K, et al. Perineural invasion in colorectal cancer: mechanisms of action and clinical relevance. Cell Oncol (Dordr) 2024;47:1-17. [Crossref] [PubMed]
- Hamid HKS, Davis GN, Trejo-Avila M, et al. Prognostic and predictive value of neutrophil-to-lymphocyte ratio after curative rectal cancer resection: A systematic review and meta-analysis. Surg Oncol 2021;37:101556. [Crossref] [PubMed]
- Portale G, Bartolotta P, Azzolina D, et al. Prognostic role of platelet-to-lymphocyte ratio, neutrophil-to-lymphocyte, and lymphocyte-to-monocyte ratio in operated rectal cancer patients: systematic review and meta-analysis. Langenbecks Arch Surg 2023;408:85. [Crossref] [PubMed]
- Zhou ZQ, Pang S, Yu XC, et al. Predictive Values of Postoperative and Dynamic Changes of Inflammation Indexes in Survival of Patients with Resected Colorectal Cancer. Curr Med Sci 2018;38:798-808. [Crossref] [PubMed]
- Qi XY, Tan F, Liu MX, et al. Serum and peritoneal biomarkers for the early prediction of symptomatic anastomotic leakage in patients following laparoscopic low anterior resection: A single-center prospective cohort study. Cancer Rep (Hoboken) 2023;6:e1781. [Crossref] [PubMed]
- Huisman DE, Reudink M, van Rooijen SJ, et al. LekCheck: A Prospective Study to Identify Perioperative Modifiable Risk Factors for Anastomotic Leakage in Colorectal Surgery. Ann Surg 2022;275:e189-97. [Crossref] [PubMed]
- Corti F, Lonardi S, Intini R, et al. The Pan-Immune-Inflammation Value in microsatellite instability-high metastatic colorectal cancer patients treated with immune checkpoint inhibitors. Eur J Cancer 2021;150:155-67. [Crossref] [PubMed]
- Sato S, Shimizu T, Ishizuka M, et al. The preoperative pan-immune-inflammation value is a novel prognostic predictor for with stage I-III colorectal cancer patients undergoing surgery. Surg Today 2022;52:1160-9. [Crossref] [PubMed]
- Zhang R, Hu C, Zhang J, et al. Prognostic significance of inflammatory and nutritional markers in perioperative period for patients with advanced gastric cancer. BMC Cancer 2023;23:5. [Crossref] [PubMed]