Solute carrier family 16 member 1 as a potential prognostic factor for pancreatic ductal adenocarcinoma and its influence on tumor immunity
Highlight box
Key findings
• Solute carrier family 16 member 1 (SLC16A1) was found to be an independent prognostic factor of pancreatic ductal adenocarcinoma (PDAC) and was closely correlated with tumor immunity.
What is known and what is new?
• Studies have revealed that SLC16A1 is overexpressed in many kinds of cancers and that the upregulation of SLC16A1 is associated with poor prognosis.
• We analyzed the relationship between SLC16A1 and immune infiltration, identified relevant hub genes, and explored the impact of SLC16A1 expression in tumor cells on immune cytotoxicity through in vitro experiments.
What is the implication, and what should change now?
• The study emphasized the critical role of SLC16A1 in the prognosis of tumors and its potential as an immunotherapeutic target. However, the specific mechanism by which SLC16A1 impacts the immune response of pancreatic cancer still requires further clarification.
Introduction
Pancreatic cancer is the seventh leading cause of cancer-related death worldwide, and pancreatic ductal adenocarcinoma (PDAC) is the most common type of pancreatic cancer, contributing to the death of more than 430,000 patients worldwide every year (1,2). It has an extremely poor prognosis, with the lowest reported 5-year overall survival (OS) rate among all malignant tumors from 2010–2016 (3). PDAC is predicted to be the second leading cause of cancer-related death by 2030 (4).
The poor prognosis of PDAC is likely due to the lack of early clinical symptoms, poor sensitivity of PDAC diagnostic tests, and lack of response to standard chemotherapeutic agents (5). Only 10–20% of patients with PDAC qualify for surgical resection due to the presence of distant metastases (6). Therefore, chemotherapy is recommended as first-line treatment to control progression in the majority of PDAC cases (7). For patients with advanced PDAC, multiagent chemotherapy is the standard of care with common regimens including FOLFIRINOX (folinic acid, fluorouracil, irinotecan hydrochloride, and oxaliplatin), gemcitabine/nab-paclitaxel, and nanoliposomal irinotecan/fluorouracil (7-9). Despite aggressive treatment, most patients eventually relapse (5). However, Options for treatment after first-line chemotherapy remain limited (10).
The role of immunotherapy in pancreatic cancer is largely unknown but it serves as an option for patients with poor response to conventional treatment (11-13). The limited benefit of immunotherapy may be related to the immunosuppressive tumor microenvironment (TME) of PDAC (14). As such, novel strategies are needed to improve the immunogenicity of PDAC. One potential target may be solute carrier family 16 member 1 (SLC16A1), which belongs to the monocarboxylate transporter family. The protein encoded by this gene is monocarboxylate transporter-1 (MCT1) which mediates lactate transportation (15,16). Increased SLC16A1 expression contributes to tumor progression (17-20). In recent years, studies have found that SLC16A1 still has implications for tumor immunity through regulating lactic acid metabolism (21,22).
Our study aimed to explore the clinical value and potential mechanism of SLC16A1 in pancreatic cancer immunity. Using the bioinformatics method, we analyzed the transcriptome profiling data of PDAC from The Cancer Genome Atlas (TCGA) and explored the clinical significance of SLC16A1 in PDAC. We divided patients into two groups according to their SLC16A1 expression and compared their immune infiltration status. We then applied ESTIMATE and weighted gene coexpression network analysis (WGCNA) computational methods to screen for immune-related genes from differentially expressed genes (DEGs). We further investigated the underlying mechanism of SLC16A1 by analyzing prognosis-related genes and examined their relationship with immune infiltration to provide new ideas for the clinical treatment of PDAC. We also validated the effect of SLC16A1 on tumor progression and prognosis in patients with clinical samples. Finally, in vitro experiments indicated that the expression of SLC16A1 in tumor cells could have an impact on CD8+ T-cell function. In conclusion, we not only investigated the relationship between SLC16A1 expression level and the prognosis of patients with tumors, but also revealed its impact on the immune microenvironment of tumor patients. We present this article in accordance with the TRIPOD reporting checklist (available at https://jgo.amegroups.com/article/view/10.21037/jgo-24-147/rc).
Methods
Sample information
Data were obtained from TCGA dataset including PDAC samples [TCGA-PAAD (pancreatic adenocarcinoma), T] and normal pancreas samples (N). Transcriptome profiling data, survival data, and clinical information were downloaded from the University of California at Santa Cruz (UCSC) Xena browser (https://xena.ucsc.edu) (23).
Comparison of SLC16A1 expression between PDAC and normal tissues
Gene Expression Profiling Interactive Analysis (GEPIA; http://gepia.cancer-pku.cn/) is a multidimensional cancer genome dataset that integrates big data from TCGA and Genotype-Tissue Expression (GTEx) (24). In our study, differential expression analysis and survival analysis of SLC16A1 were performed.
SLC16A1 expression was compared between PDAC and normal tissues by analyzing immunohistochemical (IHC) images from The Human Protein Atlas (https://www.proteinatlas.org) (25). The time-dependent receiver operating characteristic (ROC) curve (including 1-, 3-, and 5-year survival) was also examined using the R package “timeROC” (The Foundation of Statistical Computing) to reflect the sensitivity and specificity of SLC16A1 (26).
Identification of DEGs
The R package “DESeq2” was used to identify DEGs between high SLC16A1 expression (H-SLC16A1) and low expression (L-SLC16A1) groups according to the threshold of |log2 (fold change)| >1 and a P value <0.05 (27). The R packages “Pheatmap”, “ggpubr”, and “ggthemes” were applied to generate heatmaps and volcano plots for the visualization of DEGs.
Immune infiltration analysis
We quantified stromal and immune scores using the ESTIMATE algorithm and evaluated the TME of each patient with PDAC and determined the stromal score (stromal content), immune score (extent of immune cell infiltration), ESTIMATE score (synthetic mark of stroma and immune), and tumor purity using R (28). Moreover, the “xCell” R package was used to calculate the proportion of immune cells relative to SLC16A1 expression (29). CIBERSORT can accurately estimate the immune composition of tumor tissues and was thus used to analyze the correlation between immune cells and five important genes (30).
WGCNA of key modules according to immunoscore
WGCNA was performed on DEGs using the R package “WGCNA”. Five modules were obtained, and their correlation with cluster, stromal score, immune score, ESTIMATE score, and tumor purity was calculated (31). The results showed that the brown module exhibited the highest correlation with immunoscore.
Enrichment analysis
For the analysis of the potential mechanism of genes in the brown module, Gene Ontology (GO) (32) and Kyoto Encyclopedia of Genes and Genomes (KEGG) analyses (33,34) were applied to understand the functions of genes of the brown module using the R package “clusterProfiler” (35).
Protein-protein interaction (PPI) network
Construction PPI networks were analyzed with the Search Tool for the Retrieval of Interacting Genes/Proteins (STRING) database (https://string-db.org/), and the results were visualized using Cytoscape (v. 3.7.2).
Prognostic modeling
Univariate Cox regression was used to screen the genes in the brown module related to survival, with P<0.01 used as the screening criterion. Subsequently, genes were selected and subjected to least absolute shrinkage and selection operator (LASSO) regression and further screen via Spearman correlation (P<0.05) to determine the most suitable genes (36).
Kaplan-Meier survival plots
The Kaplan-Meier survival plots of five important genes were analyzed via the Kaplan-Meier plotter portal (http://kmplot.com/analysis/) (37).
IHC staining
Fifty paraffin-embedded specimens confirmed to be pancreatic cancer were collected from the Tianjin Medical University Cancer Institute and Hospital. The study was conducted in accordance with the Declaration of Helsinki (as revised in 2013). The study was approved by the Ethics Committee of Tianjin Medical University Cancer Institute and Hospital (No. bc2023074) before the study began. We explained the necessary research procedures to all patients and obtained written informed consent for data and sample use. The tissues were incubated overnight with primary antibodies against SLC16A1 (20139-1-AP; Proteintech, Wuhan, China). After being washed three times with phosphate-buffered saline (PBS) for 15 min, the samples were incubated with secondary antibody at 37 ℃ for 60 min. After another washing, the specimens were stained with DAB and hematoxylin. Finally, the sections were observed under a microscope.
Western blot analysis
The cells were lysed in 10% sodium dodecyl-sulfate (SDS) containing 1% protease inhibitor (BL612A; Biosharp, Hefei, China), and the protein concentration was quantified via Bradford protein assay. A total of 10 µg of protein lysates from each sample was separated in 10% SDS–polyacrylamide gel electrophoresis (PAGE) and then transferred onto polyvinylidene fluoride membranes (IPVH00010; MilliporeSigma, Burling, MA, USA). After blocking was completed with 5% skim milk, the membranes were incubated overnight in a 4 ℃ refrigerator with the primary antibodies against SLC16A1 (20139-1-AP; Proteintech, Wuhan, China) and GAPDH (KM9002; Sungene Biotech, Tianjin, China). After being washed, the membranes were probed with anti-rabbit immunoglobin G (IgG) secondary antibody (ab205718; Abcam, Cambridge, UK) or anti-mouse IgG secondary antibody (ab6728; Abcam).
In vitro cytotoxicity assay for CD8+ T cells
Human pancreatic cancer cell lines (SW1990) were purchased from the American Type Culture Collection (ATCC; Manassas, VA, USA).
Cell transfection was performed using Lipofectamine 2000 (11668030, Invitrogen, Thermo Fisher Scientific, Waltham, MA, USA). To examine T-cell activation, peripheral blood mononuclear cells (PBMCs) and tumor cells were cocultured in RPMI-1640 medium supplemented with 10% fetal bovine serum (FBS; 04-001-1A; Biological Industries, Göttingen, Germany) and 0.01% penicillin/streptomycin. The cells were then incubated with KrO-conjugated anti-CD45 (B36294; Beckman Coulter, Brea, CA, USA), ECD-conjugated anti-CD3 (A07748; Beckman Coulter), A700-conjugated anti-CD8 (737659; Beckman Coulter), APC-conjugated anti-CD69 (A80711; Beckman Coulter), and PE-conjugated anti-programmed cell death protein 1 (PD-1; B30634; Beckman Coulter) at 25 ℃ for 30 min and analyzed via flow cytometry, data analyzed using FlowJo software (BD Biosciences).
After coculture, the CD8+ T cells were isolated from PBMCs by CD8+ T Cell Isolation Kit, human (130-094-156, Miltenyi Biotec, German) and cocultured again with SW1990 cells. The cytotoxicity of CD8+ T cells was assessed using a lactate dehydrogenase (LDH) activity assay kit (BC0685; Solarbio, Beijing, China).
Statistical analysis
Univariate and multivariate Cox regression analyses were used to evaluate the significance of certain factors, including age, gender, tumor size, histological grade stage, lymph node metastasis, TNM stage, and SLC16A1 expression. The χ2 test was used to assess the statistical relationship between SLC16A1 expression and other factors, including age, gender, tumor size, histological grade stage, lymph node metastasis, and TNM stage. All statistical analyses were run in R statistical software (v. 3.6.4) and SPSS statistical software (v. 25.0; IBM Corp., Armonk, NY, USA). P<0.05 indicated statistical significance.
Results
Prognostic value of SLC16A1
In general, the tumor tissues had higher SLC16A1 expression than the normal tissues (Figure 1A). Moreover, IHC images of SLC16A1 antibody showed increased staining levels in PDAC tissues versus normal pancreatic tissues (Figure 1B), indicating the high protein expression in PDAC. Kaplan-Meier survival analysis showed that the H-SLC16A1 group was associated with poorer OS compared with the L-SLC16A1 group (Figure 1C). Univariate and multivariate Cox regression analyses suggested that SLC16A1 may be an independent predictor of OS (Table 1). Reliability was determined via time-dependent ROC curves. The area under the curve (AUC) was 0.56, 0.64, and 0.77 for 1-, 3-, and 5-year survival, respectively, implying the good potential of SLC16A1 in monitoring survival (Figure 1D). In summary, the findings strongly indicated that SLC16A1 could be an independent prognostic factor for PDAC.
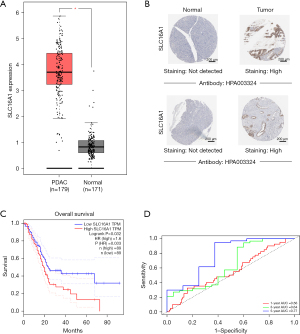
Table 1
Variable | Univariate analysis | Multivariate analysis | |||
---|---|---|---|---|---|
Hazard ratio (95% CI) | P value | Hazard ratio (95% CI) | P value | ||
Age | 1.03 (1.01–1.05) | 0.01* | 1.03 (1.00–1.05) | 0.02* | |
Gender | 1.28 (0.84–1.95) | 0.24 | 1.20 (0.78–1.85) | 0.40 | |
Stage | 1.23 (0.82–1.86) | 0.31 | 0.79 (0.38–1.63) | 0.52 | |
Tumor size | 1.49 (0.92–2.41) | 0.10 | 1.26 (0.66–2.43) | 0.48 | |
LN metastasis | 2.18 (1.28–3.71) | 0.004* | 1.93 (1.11–3.35) | 0.02* | |
Histological grade | 1.34 (1.00–1.80) | 0.05 | 1.16 (0.85–1.59) | 0.34 | |
SLC16A1 | 1.39 (1.06–1.81) | 0.01* | 1.39 (1.03–1.87) | 0.03* |
Data are based on clinical information of patients in TCGA database. *, statistically significant at P<0.05. PDAC, pancreatic ductal adenocarcinoma; LN, lymph node; CI, confidence interval; TCGA, The Cancer Genome Atlas; SLC16A1, solute carrier family 16 member 1.
Identification of immune characteristics and immunity-related genes
To clarify the effect of SLC16A1 on the TME, we examined the infiltration of immune cells in patients. The xCell results showed that SLC16A1 expression was negatively correlated with many immune cells, including CD8+ T cells and CD4+ T cells (Figure 2A), suggesting the active immune response of patients with low SLC16A1 expression. In our search of immune-related genes, we obtained 1,203 DEGs (133 upregulated and 1,070 downregulated) between the two groups (H-SLC16A1 and L-SLC16A1). The results were visualized using a heatmap (Figure 2B) and a volcano plot (Figure 2C). All DEGs were then considered for WGCNA (Figure 2D,2E) and divided into five modules (Figure 2F,2G), to reveal the association network between SLC16A1 and DEGs. The brown module, which contained 140 immunity-related genes (Figure 2H), exhibited the highest correlation with immunoscore (Pearson correlation coefficient =0.86; P<0.0001) (Figure 2I).
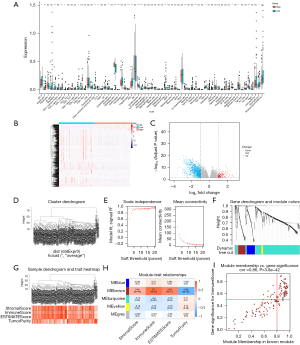
Analysis of the potential mechanism of immunity-related genes
We performed GO (Figure 3A) and KEGG enrichment analyses (Figure 3B) to determine the biochemical functions of the key genes. From the GO terms, we found that these key genes had a close connection to immune processes, such as mononuclear cell differentiation, lymphocyte proliferation, and B-cell activation, which are key functions of immune infiltration. In the KEGG analysis, the pathways related to immunocytes, such as T-cell receptor signaling pathway and type 1 helper (Th1) and Th2 cell differentiation, were found to be significantly enriched.
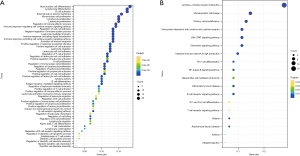
Screening of prognostic genes
We conducted PPI analysis and assessed the correlations across the genes to further reveal the mechanism of SLC16A1 in affecting immune infiltration (Figure 4A). Univariate Cox regression analysis was then performed on the DEGs from the expression matrices of patients to screen for potential prognostic genes. Factors (BCL11A, CARMIL2, SEPT1, LINGO3, SCN4A, AFF3, OCM, and SPINK2) with P<0.01 were sorted out for LASSO Cox regression analysis to identify robust markers (Figure 4B). A prognostic model containing six genes (BCL11A, LINGO3, SCN4A, AFF3, OCM, and SPINK2) was then constructed to evaluate the disease outcome. We next screened parameters with the LASSO Cox regression model according to the coefficients of nine genes (Figure 4C). Cross-validation analysis revealed that the optimal λ was six (Figure 4D). A moderate efficacy was verified with an AUC of 0.69 from the ROC curve (Figure 4E). This prognostic model also showed that patients who died of PDAC had high risk scores (Figure 4F). The distribution and status of OS were then analyzed by ranking the risk scores (Figure 4G-4H). The results showed that the patients with high-risk scores were likely to be deceased. Except for AFF3 (P>0.05) (Figure 5A), all of the genes (BCL11A, LINGO3, SCN4A, OCM, and SPINK2) had a significant correlation with SLC16A1 expression (P<0.05) and were subjected to subsequent analysis (Figure 5B-5F).
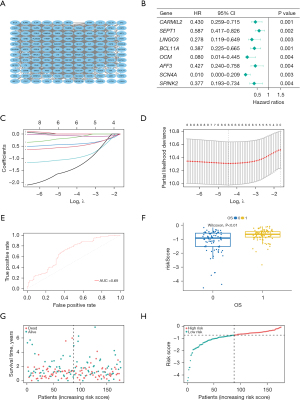
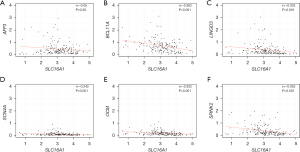
Correlation of the five genes with the proportion of tumor-initiating cells (TICs)
Investigating the types of infiltrating immune cells in patients was necessary to further explore the correlation of the five prognostic-related genes with the immune microenvironment. Hence, CIBERSORT was adopted for the evaluation of the relative proportion of 22 types of immune cells in all PDAC specimens (Figure 6A). The correlation between TICs and the expression of the five genes was confirmed (Figure 6B). The results suggested that the expression of five genes was positively correlated with the infiltration levels of naïve B cells, memory B cells, CD8+ T cells, and activated CD4+ memory T cells. Therefore, these five genes (BCL11A, LINGO3, SCN4A, OCM, and SPINK2) might be responsible for the preservation of the immune-active status in the TME. In addition, Kaplan-Meier survival analysis of OS also indicated that patients with a high expression of these genes had better prognosis (Figure 6C).
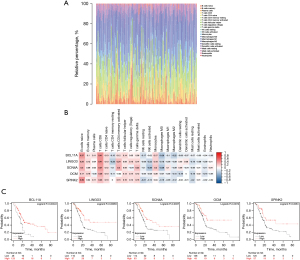
The clinical role of SLC16A1
Based on the bioinformatics method, we confirmed the key role of SLC16A1 in human pancreatic cancer. The overexpression of SLC16A1 was significantly associated with poor prognosis and the immunosuppressive phenotype in the TME. To evaluate the clinical significance of SLC16A1, we performed IHC staining in 50 clinical samples. We found that patients with a high expression of SLC16A1 in primary cancer tissues were associated with poorer OS and recurrence-free survival (RFS) (Figure 7A,7B). Univariate and multivariate Cox regression analyses also suggested that SLC16A1 could be an independent prognostic indicator of OS and recurrence-free survival (Tables 2,3). Furthermore, we analyzed the relationship between SLC16A1 and clinical parameters, including age, gender, tumor size, histological grade stage, lymph node metastasis, and TNM stage. SLC16A1 expression was significantly higher in patients with an advanced histological grade stage, TNM stage, and lymph node metastasis (Table 4).
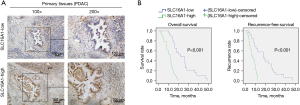
Table 2
Univariate analysis | Overall survival | Recurrence-free survival | |||||
---|---|---|---|---|---|---|---|
HR | 95% CI | P | HR | 95% CI | P | ||
Age | 1.079 | 0.590–1.974 | 0.80 | 0.694 | 0.382–1.261 | 0.23 | |
Gender | 1.002 | 0.552–1.818 | 0.99 | 1.151 | 0.646–2.050 | 0.63 | |
Tumor size | 2.072 | 1.093–3.925 | 0.02* | 1.845 | 1.006–3.382 | 0.04* | |
Histological grade | 1.895 | 1.045–3.436 | 0.03* | 1.890 | 1.057–3.379 | 0.03* | |
LN metastasis | 1.805 | 0.994–3.277 | 0.05 | 1.662 | 0.924–2.989 | 0.09 | |
TNM | 1.912 | 1.01–3.591 | 0.04* | 2.496 | 1.295–4.812 | 0.006* | |
SLC16A1 expression | 6.741 | 3.168–14.347 | <0.001* | 4.877 | 2.319–10.257 | <0.001* |
Data are based on tissue IHC assay. Univariate Cox proportional hazard analysis was used for backward model selection. *, statistically significant at P<0.05. LN, lymph node; HR, hazard ratio; CI, confidence interval; IHC, immunohistochemistry; TNM, postoperatively tumor node metastasis; SLC16A1, solute carrier family 16 member 1.
Table 3
Multivariate analysis | Overall survival | Recurrence-free survival | |||||
---|---|---|---|---|---|---|---|
HR | 95% CI | P | HR | 95% CI | P | ||
Tumor size | 2.119 | 1.073–4.186 | 0.03* | 1.563 | 0.816–2.992 | 0.17 | |
Histological grade | 1.525 | 0.821–2.831 | 0.18 | 1.360 | 0.729–2.539 | 0.33 | |
TNM | 1.180 | 0.536–2.601 | 0.68 | 1.964 | 0.937–4.116 | 0.07 | |
SLC16A1 expression | 5.832 | 2.450–13.883 | <0.001 | 3.229 | 1.418–7.351 | 0.005* |
*, statistically significant at P<0.05. HR, hazard ratio; CI, confidence interval; TNM, postoperatively tumor node metastasis; SLC16A1, solute carrier family 16 member 1.
Table 4
Parameter | SLC16A1 (n) | χ2 | P | r | |
---|---|---|---|---|---|
Low | High | ||||
Age (years) | 0.263 | 0.41 | 0.073 | ||
≤60 | 17 | 14 | |||
>60 | 9 | 10 | |||
Gender | 0.349 | 0.38 | −0.084 | ||
Male | 13 | 14 | |||
Female | 13 | 10 | |||
Tumor size (cm3) | 2.424 | 0.10 | 0.220 | ||
≤3.5 | 12 | 6 | |||
>3.5 | 14 | 18 | |||
Histological grade | 9.641 | 0.002* | 0.439 | ||
G1/G2 | 19 | 7 | |||
G3 | 7 | 17 | |||
LN metastasis | 6.464 | 0.01* | 0.360 | ||
N0 | 20 | 10 | |||
N1 | 6 | 14 | |||
TNM stage | 9.507 | 0.002* | 0.436 | ||
I | 14 | 3 | |||
II | 12 | 21 |
*, statistically significant at P<0.05. SLC16A1, solute carrier family 16 member 1; LN, lymph node; TNM, postoperatively tumor node metastasis.
SLC16A1 suppression of CD8+ T-cell activity
To examine the correlation between the expression of SLC16A1 and tumor immunity, we increased the expression of SLC16A1 in the SW1990 human pancreatic cancer cell line (Figure 8A). Subsequently, we established a coculture model using SW1990 cells and PBMCs to investigate the impact of tumors on immune cells. Through in vitro LDH release assay, we confirmed that the overexpression of SLC16A1 could impair the cytotoxic effect of CD8+ T cells in PBMCs against tumor cells (Figure 8B). Flow cytometry further revealed a reduction in CD69 expression on the surface of CD8+ T cells from PBMCs cocultured with SLC16A1-overexpressed tumor cells (Figure 8C), while the population of exhausted PD-1+ CD8+ T cells remained relatively unchanged (Figure 8D). These findings confirmed that the overexpression of SLC16A1 in PDAC detrimentally affects the in vitro activation and tumor-killing capabilities of CD8+ T cells.
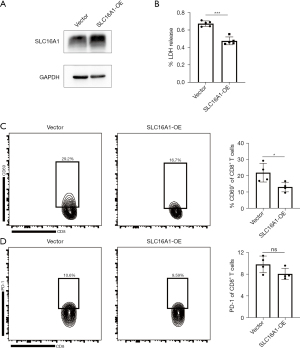
Discussion
Our study suggests that SLC16A1 is expressed at high levels in pancreatic cancer and has a significant negative correlation with the prognosis of PDAC. In addition, we also discussed the effect on TME. SLC16A1 overexpression has been reported in many kinds of cancers, including brain cancer, breast cancer, ovarian cancer, melanoma, and cervical cancer (38-40). It is associated with tumor progression and promotes cancer cell migration and invasion. In cervical carcinoma, induced SLC16A1 and CD147 expression was found to promote cancer cell migration (41). Payen et al. (19) also reported that SLC16A1 independently promotes cancer cell migration and invasion.
Evidence indicates that there is a close correlation between tumor-infiltrating immune cells and cancer progression (42-44). Immunotherapy has been widely recognized as a form of therapy for many cancers, but it is controversial in PDAC (45-47). The limited efficacy of immunotherapy in PDAC may be attributed to its hypoxic and immunosuppressive TME. To improve sensitivity to immunotherapy, novel strategies are required to overcome this immunosuppressive TME. Strategies involving vaccines and stereotactic body radiotherapy have been investigated (48). One potential target is SLC16A1, which when overexpressed suppresses cytotoxic CD8+ T cell activity. In fact, a study has shown that SLC16A1 inhibitors suppress tumor cell proliferation by decreasing lactate transport (49). However, clinical studies are warranted to further evaluate the efficacy of these inhibitors. In exploring the mechanism underlying SLC16A1 impairment of tumor immune infiltration, we detected 1,203 DEGs between the H-SLC16A1 and L-SLC16A1 groups. We then performed WGCNA to identify the DEGs related to immune score and divided them into five modules. We concluded that the brown module exhibited the highest correlation with the immunoscore. According to the GO and KEGG enrichment analyses of the WGCNA-selected module eigengenes, the genes of the brown module were closely related to immune infiltration processes, such as lymphocyte differentiation, lymphocyte proliferation, lymphocyte migration, and Th1 and Th2 cell differentiation.
We then attempted to identify immunoscore-related genes that contribute to survival. We applied Cox regression analysis and the LASSO regression model and identified six prognosis-related genes. By combining these results with the SLC16A1 correlation findings, we ultimately obtained five important genes (BCL11A, LINGO3, SCN4A, OCM, and SPINK2) to further investigating the relationship between SLC16A1 and tumor immunity by identifying potential target genes it might regulate. Correlations tests revealed that the expression of these important genes was negatively correlated with SLC16A1. To explore the effect on immune cell infiltration in tumors, we further adopted CIBERSORT for evaluating the relative proportion of immune cells in each PDAC specimen. As expected, B cells, memory B cells, CD8+ T cells, and activated CD4 memory T cells showed a positive correlation with patient survival (50-52). The potential of SLC16A1 being an independent prognostic factor was verified by clinical samples. Through in vitro study, we found that overexpression of SLC16A1 could weaken CD8+ T-cell activity and impair the killing effect on pancreatic cancer cells. Therefore, SLC16A1 may play an important role in the suppression of tumor immunity and subsequent resistance to immunotherapy. However, some potential limitations of our study still include: first, the size of the patient cohort or the number of samples used for certain analyses may impact the statistical power and generalizability of the results and the study has not analyzed all clinical prognostic factors, such as CA19-9 values. While the study provides insights into the potential prognostic and therapeutic implications of SLC16A1, further validation in independent patient cohorts and functional studies will be necessary to confirm its clinical relevance.
Conclusions
We investigated the role of SLC16A1 in pancreatic cancer through use of bioinformatics, clinical study, and in vitro experiments. SLC16A1 was found to be an independent prognostic factor of PDAC and closely related to tumor immunity. Overall, SLC16A1 has the potential to be a diagnostic and prognostic target for PDAC.
Acknowledgments
We would like to thank all the patients for their important contributions and the administrators of the public databases for providing data.
Funding: This work was supported by
Footnote
Reporting Checklist: The authors have completed the TRIPOD reporting checklist. Available at https://jgo.amegroups.com/article/view/10.21037/jgo-24-147/rc
Data Sharing Statement: Available at https://jgo.amegroups.com/article/view/10.21037/jgo-24-147/dss
Peer Review File: Available at https://jgo.amegroups.com/article/view/10.21037/jgo-24-147/prf
Conflicts of Interest: All authors have completed the ICMJE uniform disclosure form (available at https://jgo.amegroups.com/article/view/10.21037/jgo-24-147/coif). The authors have no conflicts of interest to declare.
Ethical Statement: The authors are accountable for all aspects of the work in ensuring that questions related to the accuracy or integrity of any part of the work are appropriately investigated and resolved. The study was conducted in accordance with the Declaration of Helsinki (as revised in 2013). The study was approved by the Ethics Committee of Tianjin Medical University Cancer Institute and Hospital (No. bc2023074) before the study began. We explained the necessary research procedures to all patients and obtained written informed consent for data and sample use.
Open Access Statement: This is an Open Access article distributed in accordance with the Creative Commons Attribution-NonCommercial-NoDerivs 4.0 International License (CC BY-NC-ND 4.0), which permits the non-commercial replication and distribution of the article with the strict proviso that no changes or edits are made and the original work is properly cited (including links to both the formal publication through the relevant DOI and the license). See: https://creativecommons.org/licenses/by-nc-nd/4.0/.
References
- Rawla P, Sunkara T, Gaduputi V. Epidemiology of Pancreatic Cancer: Global Trends, Etiology and Risk Factors. World J Oncol 2019;10:10-27. [Crossref] [PubMed]
- Halbrook CJ, Lyssiotis CA, Pasca di Magliano M, et al. Pancreatic cancer: Advances and challenges. Cell 2023;186:1729-54. [Crossref] [PubMed]
- Sim W, Lim WM, Hii LW, et al. Targeting pancreatic cancer immune evasion by inhibiting histone deacetylases. World J Gastroenterol 2022;28:1934-45. [Crossref] [PubMed]
- Rahib L, Smith BD, Aizenberg R, et al. Projecting cancer incidence and deaths to 2030: the unexpected burden of thyroid, liver, and pancreas cancers in the United States. Cancer Res 2014;74:2913-21. [Crossref] [PubMed]
- Morrison AH, Byrne KT, Vonderheide RH. Immunotherapy and Prevention of Pancreatic Cancer. Trends Cancer 2018;4:418-28. [Crossref] [PubMed]
- Strobel O, Neoptolemos J, Jäger D, et al. Optimizing the outcomes of pancreatic cancer surgery. Nat Rev Clin Oncol 2019;16:11-26. [Crossref] [PubMed]
- Mizrahi JD, Surana R, Valle JW, et al. Pancreatic cancer. Lancet 2020;395:2008-20. [Crossref] [PubMed]
- Zeng S, Pöttler M, Lan B, et al. Chemoresistance in Pancreatic Cancer. Int J Mol Sci 2019;20:4504. [Crossref] [PubMed]
- Park W, Chawla A, O'Reilly EM. Pancreatic Cancer: A Review. JAMA 2021;326:851-62. [Crossref] [PubMed]
- Fukahori M, Okabe Y, Shimokawa M, et al. Efficacy of second-line chemotherapy after treatment with gemcitabine plus nab-paclitaxel or FOLFIRINOX in patients with metastatic pancreatic cancer. Sci Rep 2023;13:19399. [Crossref] [PubMed]
- Laface C, Memeo R, Maselli FM, et al. Immunotherapy and Pancreatic Cancer: A Lost Challenge? Life (Basel) 2023;13:1482. [Crossref] [PubMed]
- Bear AS, Vonderheide RH, O'Hara MH. Challenges and Opportunities for Pancreatic Cancer Immunotherapy. Cancer Cell 2020;38:788-802. [Crossref] [PubMed]
- Moral JA, Leung J, Rojas LA, et al. ILC2s amplify PD-1 blockade by activating tissue-specific cancer immunity. Nature 2020;579:130-5. [Crossref] [PubMed]
- Erkan M, Hausmann S, Michalski CW, et al. The role of stroma in pancreatic cancer: diagnostic and therapeutic implications. Nat Rev Gastroenterol Hepatol 2012;9:454-67. [Crossref] [PubMed]
- Halestrap AP. The SLC16 gene family - structure, role and regulation in health and disease. Mol Aspects Med 2013;34:337-49. [Crossref] [PubMed]
- Silva A, Cerqueira MC, Rosa B, et al. Prognostic Value of Monocarboxylate Transporter 1 Overexpression in Cancer: A Systematic Review. Int J Mol Sci 2023;24:5141. [Crossref] [PubMed]
- Zhang G, Zhang Y, Dong D, et al. MCT1 regulates aggressive and metabolic phenotypes in bladder cancer. J Cancer 2018;9:2492-501. [Crossref] [PubMed]
- Pinheiro C, Penna V, Morais-Santos F, et al. Characterization of monocarboxylate transporters (MCTs) expression in soft tissue sarcomas: distinct prognostic impact of MCT1 sub-cellular localization. J Transl Med 2014;12:118. [Crossref] [PubMed]
- Payen VL, Hsu MY, Rädecke KS, et al. Monocarboxylate Transporter MCT1 Promotes Tumor Metastasis Independently of Its Activity as a Lactate Transporter. Cancer Res 2017;77:5591-601. [Crossref] [PubMed]
- Zhao Z, Wu MS, Zou C, et al. Downregulation of MCT1 inhibits tumor growth, metastasis and enhances chemotherapeutic efficacy in osteosarcoma through regulation of the NF-κB pathway. Cancer Lett 2014;342:150-8. [Crossref] [PubMed]
- Watson MJ, Vignali PDA, Mullett SJ, et al. Metabolic support of tumour-infiltrating regulatory T cells by lactic acid. Nature 2021;591:645-51. [Crossref] [PubMed]
- Fischer K, Hoffmann P, Voelkl S, et al. Inhibitory effect of tumor cell-derived lactic acid on human T cells. Blood 2007;109:3812-9. [Crossref] [PubMed]
- Haeussler M, Zweig AS, Tyner C, et al. The UCSC Genome Browser database: 2019 update. Nucleic Acids Res 2019;47:D853-8. [Crossref] [PubMed]
- Tang Z, Li C, Kang B, et al. GEPIA: a web server for cancer and normal gene expression profiling and interactive analyses. Nucleic Acids Res 2017;45:W98-102. [Crossref] [PubMed]
- Pontén F, Schwenk JM, Asplund A, et al. The Human Protein Atlas as a proteomic resource for biomarker discovery. J Intern Med 2011;270:428-46. [Crossref] [PubMed]
- Heagerty PJ, Lumley T, Pepe MS. Time-dependent ROC curves for censored survival data and a diagnostic marker. Biometrics 2000;56:337-44. [Crossref] [PubMed]
- Liu S, Wang Z, Zhu R, et al. Three Differential Expression Analysis Methods for RNA Sequencing: limma, EdgeR, DESeq2. J Vis Exp 2021;e62528. [PubMed]
- Yoshihara K, Shahmoradgoli M, Martínez E, et al. Inferring tumour purity and stromal and immune cell admixture from expression data. Nat Commun 2013;4:2612. [Crossref] [PubMed]
- Aran D, Hu Z, Butte AJ. xCell: digitally portraying the tissue cellular heterogeneity landscape. Genome Biol 2017;18:220. [Crossref] [PubMed]
- Chen B, Khodadoust MS, Liu CL, et al. Profiling Tumor Infiltrating Immune Cells with CIBERSORT. Methods Mol Biol 2018;1711:243-59. [Crossref] [PubMed]
- Langfelder P, Horvath S. WGCNA: an R package for weighted correlation network analysis. BMC Bioinformatics 2008;9:559. [Crossref] [PubMed]
- Ashburner M, Ball CA, Blake JA, et al. Gene ontology: tool for the unification of biology. The Gene Ontology Consortium. Nat Genet 2000;25:25-9. [Crossref] [PubMed]
- Kanehisa M, Goto S, Furumichi M, et al. KEGG for representation and analysis of molecular networks involving diseases and drugs. Nucleic Acids Res 2010;38:D355-60. [Crossref] [PubMed]
- Xu Q, Wang W, Hu H, et al. Screening of potential pain genes in pancreatic ductal adenocarcinoma (PDAC) based on bioinformatics methods. J Gastrointest Oncol 2023;14:420-8. [Crossref] [PubMed]
- Yu G, Wang LG, Han Y, et al. clusterProfiler: an R package for comparing biological themes among gene clusters. OMICS 2012;16:284-7. [Crossref] [PubMed]
- Peng Y, Liu C, Li M, et al. Identification of a prognostic and therapeutic immune signature associated with hepatocellular carcinoma. Cancer Cell Int 2021;21:98. [Crossref] [PubMed]
- Nagy Á, Munkácsy G, Győrffy B. Pancancer survival analysis of cancer hallmark genes. Sci Rep 2021;11:6047. [Crossref] [PubMed]
- Payen VL, Mina E, Van Hée VF, et al. Monocarboxylate transporters in cancer. Mol Metab 2020;33:48-66. [Crossref] [PubMed]
- You S, Zhang J, Yu L, et al. Construction of SLC16A1/3 Targeted Gallic Acid-Iron-Embelin Nanoparticles for Regulating Glycolysis and Redox Pathways in Cervical Cancer. Mol Pharm 2023;20:4574-86. [Crossref] [PubMed]
- Chatterjee P, Bhowmik D, Roy SS. A systemic analysis of monocarboxylate transporters in ovarian cancer and possible therapeutic interventions. Channels (Austin) 2023;17:2273008. [Crossref] [PubMed]
- De Saedeleer CJ, Porporato PE, Copetti T, et al. Glucose deprivation increases monocarboxylate transporter 1 (MCT1) expression and MCT1-dependent tumor cell migration. Oncogene 2014;33:4060-8. [Crossref] [PubMed]
- Bremnes RM, Busund LT, Kilvær TL, et al. The Role of Tumor-Infiltrating Lymphocytes in Development, Progression, and Prognosis of Non-Small Cell Lung Cancer. J Thorac Oncol 2016;11:789-800. [Crossref] [PubMed]
- Seminerio I, Kindt N, Descamps G, et al. High infiltration of CD68+ macrophages is associated with poor prognoses of head and neck squamous cell carcinoma patients and is influenced by human papillomavirus. Oncotarget 2018;9:11046-59. [Crossref] [PubMed]
- Zhu YH, Zheng JH, Jia QY, et al. Immunosuppression, immune escape, and immunotherapy in pancreatic cancer: focused on the tumor microenvironment. Cell Oncol (Dordr) 2023;46:17-48. [Crossref] [PubMed]
- Banerjee K, Kumar S, Ross KA, et al. Emerging trends in the immunotherapy of pancreatic cancer. Cancer Lett 2018;417:35-46. [Crossref] [PubMed]
- Henriksen A, Dyhl-Polk A, Chen I, et al. Checkpoint inhibitors in pancreatic cancer. Cancer Treat Rev 2019;78:17-30. [Crossref] [PubMed]
- Sanmamed MF, Chen L. A Paradigm Shift in Cancer Immunotherapy: From Enhancement to Normalization. Cell 2019;176:677. [Crossref] [PubMed]
- Parul A, Hanfei Q, Kabeer M, et al. Overall survival (OS) and pathologic response rate from a phase II clinical trial of neoadjuvant GVAX pancreas vaccine (with cyclophosphamide) in combination with nivolumab and stereotactic body radiation therapy (SBRT) followed by definitive resection for patients with borderline resectable pancreatic adenocarcinoma (BR-PDAC). J Clin Oncol 2023;41:e16309. [Crossref]
- Quanz M, Bender E, Kopitz C, et al. Preclinical Efficacy of the Novel Monocarboxylate Transporter 1 Inhibitor BAY-8002 and Associated Markers of Resistance. Mol Cancer Ther 2018;17:2285-96. [Crossref] [PubMed]
- Speiser DE, Chijioke O, Schaeuble K, et al. CD4(+) T cells in cancer. Nat Cancer 2023;4:317-29. [Crossref] [PubMed]
- Prokhnevska N, Cardenas MA, Valanparambil RM, et al. CD8(+) T cell activation in cancer comprises an initial activation phase in lymph nodes followed by effector differentiation within the tumor. Immunity 2023;56:107-124.e5. [Crossref] [PubMed]
- Matsumoto H, Thike AA, Li H, et al. Increased CD4 and CD8-positive T cell infiltrate signifies good prognosis in a subset of triple-negative breast cancer. Breast Cancer Res Treat 2016;156:237-47. [Crossref] [PubMed]