Comprehensive genomic profiling of small bowel adenocarcinoma with liver metastasis
Highlight box
Key findings
• In this study, we investigated the genomic landscape of small bowel adenocarcinoma (SBA) liver metastasis (LM) and identified molecular characteristics associated with liver metastases. We analyzed the mutations from 97 patients with SBA including 48 patients without metastasis, 29 with LM, and 20 with extrahepatic metastases. We examined the differential mutation rates between different groups, identified genes potentially associated with metastasis, and developed a reliable model to identify patients with LM.
What is known and what is new?
• The liver is the most common site of metastasis in patients with SBA, and LM is a significant factor in poor prognosis. Early identification of patients at high risk of LM and timely intervention can potentially improve survival rates.
• Our study examined the differential mutation rates between SBA patients with and without metastasis, identified genes potentially associated with metastasis, and developed a reliable model to identify patients with LM.
What is the implication, and what should change now?
• This study is the first to report the molecular characteristics of metastatic SBA and the correlation between TP53 mutations and liver metastases.
• It can deepen our understanding of the potential mechanisms of LM in SBA and may provide new insights into future drug development and SBA treatment.
Introduction
Small bowel adenocarcinoma (SBA) is a malignant tumor that develops in the digestive tract (1). The small bowel begins at the pyloroduodenal junction and ends at the ileocecal junction, comprising the duodenum, jejunum, and ileum (2). The primary histological types of SBA include adenocarcinoma, neuroendocrine tumors, lymphoma, sarcoma, and gastrointestinal stromal tumors (3). The prevalence of SBA is relatively low, accounting for approximately 3% to 5% of gastrointestinal malignancies; however, there has been a significant upward trend in its occurrence (4,5). The estimated number of newly diagnosed cases of SBA in the year 2020 was approximately 64,477 (5).
Malignant small-bowel tumors are frequently diagnosed at an advanced stage due to the absence of specific symptoms (6). One study on SBA revealed that approximately 10% of individuals were diagnosed with stage I disease, 30% with stage II, 25% with stage III, and 35% of patients with stage IV (7). Additionally, the overall 5-year survival rate for SBA is approximately 30%, whereas metastatic disease exhibits rates ranging from 3.0% to 5.0% (2,8-12). The presence of distant metastases is a significant prognostic indicator for poor outcomes in patients with SBA. Similar to colorectal cancer (CRC), SBA has the potential to metastasize to the liver, lung, and peritoneum; however, liver metastasis (LM) remains the most common site for disease progression (13). Several studies have reported that LM is an adverse factor affecting survival (14-16). Therefore, the early detection of metastasis in patients and the timely implementation of intervention measures are crucial to ensuring a good outcome. It is necessary to research the LM-related factors in SBA in order to improve patient survival rates. However, due to the rarity of SBA, there is a lack of studies that have evaluated the mechanism of metastasis in this particular malignancy, and no predictive model for determining the risk of LM in patients with SBA has been developed.
In this study, we retrospectively analyzed the genomic characteristics of 97 patients with SBA and metastasis. By comparing the two groups of patients with and without LM, we characterized the molecular characteristics of LM in SBA. We present this article in accordance with the TRIPOD reporting checklist available at https://jgo.amegroups.com/article/view/10.21037/jgo-2025-131/rc).
Methods
Patients and tissue samples
A total of 132 patients with SBA who underwent target capture next-generation sequencing (NGS) of 1,021 cancer genes were enrolled in this study. Among them, 35 patients (26.5%) were excluded due to tissue unavailability. These patients received treatment at Zhejiang Hospital and Affiliated Hangzhou First People’s Hospital between July 2013 and July 2022. Paraffin-embedded primary lesion tissue samples were collected from all patients for testing. The clinicopathologic data regarding gender, age, metastasis, location, and stage at diagnosis (Table 1) were collected from the medical records of each patient. The study was conducted in accordance with the Declaration of Helsinki (as revised in 2013). The study was approved by the Ethics Committee of Zhejiang Hospital (No. 2024073K). Affiliated Hangzhou First People’s Hospital was informed and agreed with this study. Since this study only involves human biological samples and has a relatively large time span, and the patients had already signed the relevant informed consent forms when the samples were collected, the informed consent for this study is waived.
Table 1
Characteristics | Value (n=97) |
---|---|
Age (years), median [range] | 59 [30–82] |
Sex, n (%) | |
Male | 60 (61.9) |
Female | 37 (38.1) |
Treated, n (%) | |
No | 68 (70.1) |
Yes | 29 (29.9) |
Stage, n (%) | |
I–III | 48 (49.5) |
IV | 49 (50.5) |
Location, n (%) | |
Duodenum | 75 (77.3) |
Jejunum | 7 (7.2) |
Ileum | 5 (5.2) |
Unknown | 10 (10.3) |
Metastasis, n (%) | |
No | 48 (49.5) |
Liver | 29 (29.9) |
Lung | 6 (6.2) |
Peritoneum | 10 (10.3) |
Other | 4 (4.1) |
MSI status, n (%) | |
MSI-H | 2 (2.1) |
MSS | 72 (74.2) |
Unknown | 23 (23.7) |
MSI, microsatellite instability; MSI-H, microsatellite instability-high; MSS, microsatellite stability.
NGS
Formalin-fixed, paraffin-embedded (FFPE) specimens were collected for sequencing. The genomic DNA from FFPE specimens was extracted using the QIAamp DNA FFPE Tissue kit (Qiagen GmbH, Hilden, Germany). Peripheral blood leukocyte (PBL) DNA was extracted for germline mutation filtration using the QIAamp DNA Mini Blood kit (Qiagen GmbH), the quantification and quality control of which were evaluated with a Nanodrop 2000 spectrophotometer, a Qubit 2.0 fluorometer, and the Qubit dsDNA HS Assay Kit (Thermo Fisher Scientific, Waltham, MA, USA). DNA libraries were prepared using the KAPA DNA Library Preparation Kit (Kapa Biosystems, Wilmington, MA, USA). Capture experiments were conducted as described previously (17-19). The libraries of genomic DNA were captured with a 1,021 cancer-related gene panel and sequenced using the Gene+Seq-2000 (GenePlus-Suzhou, Suzhou, China). Matched PBLs were sequenced as a control for the filtering of germline variations.
Sequencing data analysis
FASTP (20) was used to remove reads with low-quality and terminal adaptor sequences. The remaining reads were aligned to the reference human genome (hg19) using the Burrows-Wheeler Aligner (version 0.7.12-r1039). Duplicate reads were removed using GATK (version 3.4–46-gbc02625). To detect single-nucleotide variants (SNVs) and insertions or deletions (indels), MuTect (version 1.1.4) was employed. CONTRA (v2.0.8) was then applied to detect somatic copy number alterations. The microsatellite instability (MSI) status was analyzed and reported simultaneously (21). All sequencing data for the concerned variants were verified using Integrative Genomics Viewer browser.
Prediction model development and validation
Untreated patients were assigned to the construction set, while treated patients were assigned to the validation set. Genomic factors were selected for least absolute shrinkage and selection operator (LASSO) regression. LASSO regression is a variable screening method. It imposes penalties on the coefficients of all variables, causing the coefficients of relatively unimportant independent variables to become zero and thus be excluded from the model. Therefore, LASSO regression can effectively address the problem of multicollinearity and has significant advantages in the screening of high-dimensional variables. The marker genes selected by LASSO were analyzed via multiple logistic regression, and an LM prediction model was established.
Statistical analysis
Cox regression analysis was used to assess the genes associated with LM. The graphs were generated using GraphPad Prism (version 8.0.2; GraphPad Software, San Diego, CA, USA) and R software (version 4.1.1; The R Foundation for Statistical Computing, Vienna, Austria). The “timeROC” package in R was employed to generate receiver operating characteristic (ROC) curves in order to evaluate the accuracy of the risk model. Differences between subgroups were analyzed using Fisher exact tests, and P values less than 0.05 were considered statistically significant.
Results
Patient characteristics
We retrospectively collected the information about of genetic tests conducted at Zhejiang Hospital and Affiliated Hangzhou First People’s Hospital from July 2013 to July 2022. A total of 132 patients underwent genetic testing, and 35 were excluded due to tissue unavailability, with ultimately 97 patients (60 males and 37 females) with a median age of 59 years (range, 30–82 years) being included. The duodenum was the most extensively affected segment, accounting for 77.3% of cases, while the jejunum and ileum were involved in 7.2% and 5.2% of cases respectively. There were 48 patients without metastasis and 49 patients with metastasis. The liver was the most common metastatic site (29 patients, 29.9%), followed by peritoneal metastases (8 patients, 8.2%).
Genetic landscape of SBA
The mutation landscape of the patients with SBA is presented in Figure 1. All 97 patients with SBA were found to have somatic variants. The five genes with the highest mutation frequency in the overall samples were the TP53 (62%), KRAS (45%), APC (19%), CDKN2A (18%), and SMAD4 (18%) genes. MYC copy number variation was the most common (14%) (Figure 1A). The clinically actionable mutations were further assessed. Overall, 176 actionable mutations that had potential impact on therapy or prognosis were detected in 77 (79%) cases. The most frequently observed actionable alterations were in KRAS (57%), CDKN2A (20%), and PIK3CA (10%) (Figure 1B). In addition, six patients were found to have BRAF mutations; however, none of them had the BRAF V600E mutation. The identified BRAF mutations included N581I, G469R, N581S, C532Y, and G466V. Among these mutations, N581S was found in two patients.
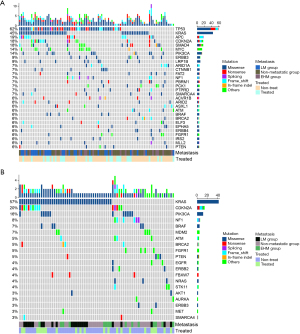
Comparisons of the mutation rates between the metastatic group and the non-metastatic group
We retrospectively collected data from 97 patients diagnosed with SBA and classified them into metastatic and non-metastatic groups. The frequencies of gene mutations and actionable genes mutations were compared between the metastatic group and the non-metastatic group (Figure 2A,2B). Figure 2C,2D shows the genomic characteristics of LM group and non-LM group (NLM group). TP53 was significantly more frequently mutated in the LM group than in the NLM group (79% vs. 54%; P=0.041; Figure 2C). We further divided the metastatic group into an LM group and extrahepatic metastasis group (EHM group). The distribution of affected organs in the EHM group was as follows: 5 in the lung, 8 in the peritoneum, and 7 in “other sites” (lymph nodes, colon, pancreas, and ovaries). Next, we explored the differences between the LM group, EHM group, and non-metastatic group. Similarly, the frequency of TP53 mutations was found to be significantly higher in the LM group compared to the non-metastatic group (Figure 2E). There was no significant difference between different groups in actionable genetic mutations (Figure 2D,2F).
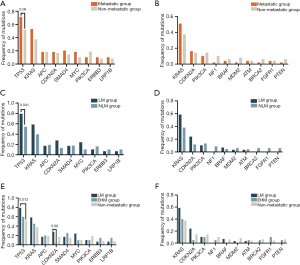
Identification of LM-related genes in patients with SBA for model inclusion
To evaluate the candidate genes associated with LM in patients with SBA, we analyzed the differential mutation rates between the LM group and non-metastatic group, the LM group and NLM group, and between the LM group and EHM group (Figure 3). Through differential gene screening, we identified 24 genes potentially associated with metastasis (P<0.20) (Table S1). However, certain genes had lower mutation rates (<3) and were subsequently excluded from model construction. Consequently, we proceeded with further analysis using the remaining 19 genes.
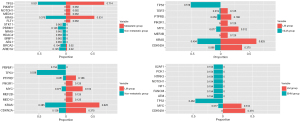
Construction of the LM prediction model and internal validation
To identify the gene signatures that could predict LM, treatment-naive patients were assigned to the construction set while treated patients were allocated to the validation set. We selected treatment-naive patients and divided them into different groups: an LM group, NLM group, non-metastatic group, and EHM group. We then compared the mutation frequency across these different groups (Figure S1). LASSO logistic regression was used on the mutation status of the 19 mutated genes in the construction set to identify significant predictive features associated with the risk of LM (Figure S2). Finally, 9 mutated genes (FLT1, TP53, KIT, PIK3R1, KRAS, NF1, PTPRD, MYC, and CDKN2A) were identified to construct the LM prediction model.
The ROC curve was generated in the construction cohort, revealing an area under the curve (AUC) of 0.867, and the cut-off value was 0.351 (sensitivity 75%; specificity 90.4%) (Figure 4A). In the internal validation with 100-sample bootstrapping, the model yielded an AUC of 0.802 and a Brier score of 0.134 in the construction set. The ROC for the validation cohort was 0.724 (Figure 4B). There was no significant difference between the two models (P=0.21) (Figure S3).
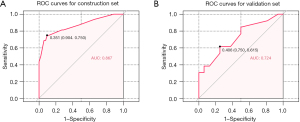
Multivariate Cox regression analyses of the predictive ability of the model
Subsequently, we performed multivariate analyses to assess the potential of our model to be an independent predictor of LM. The clinicopathological characteristics (age, sex, and treatment status) and the LM-related gene signature were included as covariates. Our findings demonstrated that the LM score was the only significant predictor of LM, with a hazard ratio (HR) of 0.043 [95% confidence interval (CI): 0.09–0.210; P<0.001] among all patients (Table 2).
Table 2
Variables | HR | 95% CI | P value |
---|---|---|---|
Age (>60 years) | 0.026 | 0.0001–1.235 | 0.87 |
Sex (male) | 0.97 | 0.056–3.214 | 0.33 |
Treated (yes) | 2.03 | 0.678–3.891 | 0.15 |
FLT1 (mut) | 0.933 | 0.766–1.287 | 0.33 |
TP53 (mut) | 2.37 | 0.863–3.223 | 0.12 |
PBRM1 (mut) | 1.969 | 0.753–2.372 | 0.16 |
KIT (mut) | 0.636 | 0.325–2.069 | 0.43 |
MYC (mut) | 0.128 | 0.047–1.035 | 0.72 |
CDKN2A (mut) | 0.764 | 0.486–1.896 | 0.38 |
LM score (high) | 0.043 | 0.09–0.210 | <0.001 |
SBC, small-bowel cancer; HR, hazard ratio; CI, confidence interval; mut, mutant; LM, liver metastasis.
Discussion
SBA is a rare malignant tumor with a poor prognosis. The main factor that affects the prognosis of patients with SBA is the clinical stage, especially stage IV disease (distant metastasis), which occurs in 35% to 40% of diagnosed patients (22,23), posing a considerable threat to public health. In this study, we conducted a comprehensive analysis of the genetic characteristics and clinically actionable genetic variations from 97 patients with SBA. The liver is the most common metastasis site of SBA (24). Therefore, we analyzed the genetic factors implicated in LM and established a prognostic model related to LM of SBA.
We found that the most commonly altered genes in SBA were TP53, KRAS, APC, CDKN2A, SMAD4, and PIK3CA. The mutation rate of APC in patients with SBA was relatively lower than that of CRC, and the converse was true for CDKN2A, which is in line with previous reports (25,26). The median tumor mutation burden of the 81 samples was 5 muts/Mb (0.96–294 muts/Mb). This suggest that SBA may exhibit distinct genomic characteristics and tumorigenic mechanisms in comparison to CRC. We further analyzed the actionable genes that inform treatment selection. The most frequently observed actionable alterations were in KRAS (57%), CDKN2A (20%), and PIK3CA (10%). BRAF mutations were detected in 6.2% (6/97) of patients with SBA, whereas no BRAF V600E mutations were found. Compared to other types of cancer, SBA has limited research and few relevant clinical guidelines. These actionable genes can be considered for future clinical trials.
Approximately one-third of patients diagnosed with SBA present with stage IV disease, and a high proportion of those with resected stage I–III disease ultimately experience relapse, with the liver being the most common site of metastasis (27). However, only a few studies have reported on LM in small-bowel cancer due to the limitation of a small sample size. Ye et al. reported that histological type, tumor size, N stage, and primary tumor location were associated with LM in SBA (14). However, the genomic characteristics associated with LM have not been reported. We categorized patients with SBA into an LM group and an NLM group. The frequency of TP53 mutations was significantly higher in the LM group compared to the NLM group. Similar results have been reported for CRC (28,29). Alvi et al. found that patients with TP53 mutations exhibited poorer survival compared to other patients (30). However, a study has reported no significant association between TP53 mutations and prognosis (31). Not only the findings in this study, but also TP53, as a commonly mutated gene in the distant metastasis of various cancers (such as oral squamous cell carcinoma, lung cancer, colorectal cancer, head and neck squamous cell carcinoma, liver cancer, ovarian cancer, etc.), significantly increases the risk of distant metastasis of cancers through multiple mechanisms. These mechanisms include promoting genomic instability, inhibiting the cGAS/STING-mediated innate immune response, activating the Golgi secretion pathway (such as the PAQR11-ARF1 axis), and enhancing the clonal advantage of tumor cells in a chronic inflammatory environment (32-34). Especially in colorectal cancer, it has been confirmed that TP53 mutations can promote the occurrence, tumor progression, and distant metastasis of colorectal cancer (35).
The LASSO model identified nine genes (FLT1, TP53, KIT, PIK3R1, KRAS, NF1, PTPRD, MYC, and CDKN2A) to construct a predictive model for predicting LM in patients with SBA. FLT1, a tyrosine kinase receptor, promotes tumor growth and metastasis (36). TP53, KIT, and KRAS are common driver mutations in gastrointestinal cancers (28,37). Patients with SBA with KRAS mutation have a shorter overall survival (38). PIK3R1 mutations commonly activate the carcinogenic PI3K pathway and have been linked to various types of cancer (39). Inactivating mutations in NF1 can lead to the prolonged activation of the RAS/RAF/MAPK signaling pathway, ultimately resulting in increased cellular proliferation (40).
However, the clinical significance of NF1 mutations remains to be elucidated. Studies have shown that PTPRD is associated with invasion and progression in colorectal and gastric cancer (41-43). Meanwhile, MYC promotes tumor invasion and migration by recruiting stromal cells and tumor-infiltrating cells (44). Shi et al. demonstrated that CDKN2A induces epithelial-mesenchymal transition and promotes the metastasis of CRC, for which it may be an independent prognostic factor (45). These genetic mutations have not been extensively examined within the context of SBA, and thus further research is needed. Our results demonstrate that the LM score has value as a predictor for LM in patients with SBA.
There are several limitations to this study that should be acknowledged. The retrospective design of restricted our ability to collect a more comprehensive array of clinical data, thereby limiting the scope for more extensive analyses. Additionally, although we developed and validated our predictive model using separate training and validation sets, the absence of external data for validation might have introduced internal bias. Therefore, it is imperative to conduct further prospective studies involving multicenter cohorts in order to mitigate potential biases.
Conclusions
We analyzed the molecular characteristics of metastatic SBA and identified genes associated with LM in SBA patients. The results of this study may further our understanding of the biological mechanisms underlying LM in metastatic SBA and may contribute to individualized treatment strategies, early intervention, and the delay of cancer progression in clinical practice.
Acknowledgments
None.
Footnote
Reporting Checklist: The authors have completed the TRIPOD reporting checklist. Available at https://jgo.amegroups.com/article/view/10.21037/jgo-2025-131/rc
Data Sharing Statement: Available at https://jgo.amegroups.com/article/view/10.21037/jgo-2025-131/dss
Peer Review File: Available at https://jgo.amegroups.com/article/view/10.21037/jgo-2025-131/prf
Funding: This study was supported by grants from
Conflicts of Interest: All authors have completed the ICMJE uniform disclosure form (available at https://jgo.amegroups.com/article/view/10.21037/jgo-2025-131/coif). The authors have no conflicts of interest to declare.
Ethical Statement: The authors are accountable for all aspects of the work in ensuring that questions related to the accuracy or integrity of any part of the work are appropriately investigated and resolved. The study was conducted in accordance with the Declaration of Helsinki (as revised in 2013). The study was approved by the Ethics Committee of Zhejiang Hospital (No. 2024073K). Affiliated Hangzhou First People’s Hospital was informed and agreed with this study. Since this study only involves human biological samples and has a relatively large time span, and the patients had already signed the relevant informed consent forms when the samples were collected, the informed consent for this study is waived.
Open Access Statement: This is an Open Access article distributed in accordance with the Creative Commons Attribution-NonCommercial-NoDerivs 4.0 International License (CC BY-NC-ND 4.0), which permits the non-commercial replication and distribution of the article with the strict proviso that no changes or edits are made and the original work is properly cited (including links to both the formal publication through the relevant DOI and the license). See: https://creativecommons.org/licenses/by-nc-nd/4.0/.
References
- Symons R, Daly D, Gandy R, et al. Progress in the Treatment of Small Intestine Cancer. Curr Treat Options Oncol 2023;24:241-61. [Crossref] [PubMed]
- Colina A, Hwang H, Wang H, et al. Natural history and prognostic factors for localised small bowel adenocarcinoma. ESMO Open 2020;5:e000960. [Crossref] [PubMed]
- Vashistha N, Singhal D. Current Oncologic Standards for Surgery of Small Bowel Cancers. J Gastrointest Cancer 2022;53:434-8. [Crossref] [PubMed]
- Sakae H, Kanzaki H, Nasu J, et al. The characteristics and outcomes of small bowel adenocarcinoma: a multicentre retrospective observational study. Br J Cancer 2017;117:1607-13. [Crossref] [PubMed]
- de Back TR, Linssen JDG, van Erning FN, et al. Incidence, clinical management and prognosis of patients with small intestinal adenocarcinomas from 1999 through 2019: A nationwide Dutch cohort study. Eur J Cancer 2024;199:113529. [Crossref] [PubMed]
- Wilson JM, Melvin DB, Gray GF, et al. Primary malignancies of the small bowel: a report of 96 cases and review of the literature. Ann Surg 1974;180:175-9. [Crossref] [PubMed]
- Overman MJ, Hu CY, Kopetz S, et al. A population-based comparison of adenocarcinoma of the large and small intestine: insights into a rare disease. Ann Surg Oncol 2012;19:1439-45. [Crossref] [PubMed]
- Teufel A, Meindl-Beinker NM, Hösel P, et al. Characteristics and outcome of patients with small bowel adenocarcinoma (SBA). J Cancer Res Clin Oncol 2023;149:4579-90. [Crossref] [PubMed]
- Giuliano K, Nagarajan N, Canner J, et al. Gastric and small intestine gastrointestinal stromal tumors: Do outcomes differ? J Surg Oncol 2017;115:351-7. [Crossref] [PubMed]
- de Back T, Nijskens I, Schafrat P, et al. Evaluation of Systemic Treatments of Small Intestinal Adenocarcinomas: A Systematic Review and Meta-analysis. JAMA Netw Open 2023;6:e230631. [Crossref] [PubMed]
- Di Nardo P, Garattini SK, Torrisi E, et al. Systemic Treatments for Advanced Small Bowel Adenocarcinoma: A Systematic Review. Cancers (Basel) 2022;14:1502. [Crossref] [PubMed]
- Yamashita K, Oka S, Yamada T, et al. Clinicopathological features and prognosis of primary small bowel adenocarcinoma: a large multicenter analysis of the JSCCR database in Japan. J Gastroenterol 2024;59:376-88. [Crossref] [PubMed]
- Ye X, Wang L, Xing Y, et al. Frequency, prognosis and treatment modalities of newly diagnosed small bowel cancer with liver metastases. BMC Gastroenterol 2020;20:342. [Crossref] [PubMed]
- Gu Y, Deng H, Wang D, et al. Metastasis Pattern and Survival Analysis in Primary Small Bowel Adenocarcinoma: A SEER-Based Study. Front Surg 2021;8:759162. [Crossref] [PubMed]
- Rompteaux P, Gagnière J, Gornet JM, et al. Resection of small bowel adenocarcinoma metastases: Results of the ARCAD-NADEGE cohort study. Eur J Surg Oncol 2019;45:331-5. [Crossref] [PubMed]
- Manguso N, Nissen N, Hendifar A, et al. Prognostic factors influencing survival in small bowel neuroendocrine tumor with liver metastases. J Surg Oncol 2019;120:926-31. [Crossref] [PubMed]
- Xu J, Wang R, Wang T, et al. Targeted DNA profiling and the prevalence of NTRK aberrations in Chinese patients with head and neck cancer. Oral Oncol 2021;119:105369. [Crossref] [PubMed]
- Ai X, Cui J, Zhang J, et al. Clonal Architecture of EGFR Mutation Predicts the Efficacy of EGFR-Tyrosine Kinase Inhibitors in Advanced NSCLC: A Prospective Multicenter Study (NCT03059641). Clin Cancer Res 2021;27:704-12. [Crossref] [PubMed]
- Wang T, Cao J, Song Q, et al. Structure-based classification of EGFR mutations in operable pre-invasive and invasive non-small cell lung cancer: a cross-sectional study. J Thorac Dis 2022;14:3508-16. [Crossref] [PubMed]
- Chen S, Zhou Y, Chen Y, et al. fastp: an ultra-fast all-in-one FASTQ preprocessor. Bioinformatics 2018;34:i884-90. [Crossref] [PubMed]
- Liu Y, Chen Y, Wu H, et al. scMSI: Accurately inferring the sub-clonal Micro-Satellite status by an integrated deconvolution model on length spectrum. PLoS Comput Biol 2024;20:e1012608. [Crossref] [PubMed]
- Axelrad JE, Olén O, Sachs MC, et al. Inflammatory bowel disease and risk of small bowel cancer: a binational population-based cohort study from Denmark and Sweden. Gut 2021;70:297-308. [PubMed]
- Legué LM, Simkens GA, Creemers GM, et al. Synchronous peritoneal metastases of small bowel adenocarcinoma: Insights into an underexposed clinical phenomenon. Eur J Cancer 2017;87:84-91. [Crossref] [PubMed]
- Dabaja BS, Suki D, Pro B, et al. Adenocarcinoma of the small bowel: presentation, prognostic factors, and outcome of 217 patients. Cancer 2004;101:518-26. [Crossref] [PubMed]
- Pan H, Cheng H, Wang H, et al. Molecular profiling and identification of prognostic factors in Chinese patients with small bowel adenocarcinoma. Cancer Sci 2021;112:4758-71. [Crossref] [PubMed]
- Yuan W, Zhang Z, Dai B, et al. Whole-exome sequencing of duodenal adenocarcinoma identifies recurrent Wnt/β-catenin signaling pathway mutations. Cancer 2016;122:1689-96. [Crossref] [PubMed]
- Gelsomino F, Balsano R, De Lorenzo S, et al. Small Bowel Adenocarcinoma: From Molecular Insights to Clinical Management. Curr Oncol 2022;29:1223-36. [Crossref] [PubMed]
- Pandey R, Johnson N, Cooke L, et al. TP53 Mutations as a Driver of Metastasis Signaling in Advanced Cancer Patients. Cancers (Basel) 2021;13:597. [Crossref] [PubMed]
- Kondo I, Iida S, Takagi Y, et al. MDM2 mRNA expression in the p53 pathway may predict the potential of invasion and liver metastasis in colorectal cancer. Dis Colon Rectum 2008;51:1395-402. [Crossref] [PubMed]
- Alvi MA, McArt DG, Kelly P, et al. Comprehensive molecular pathology analysis of small bowel adenocarcinoma reveals novel targets with potential for clinical utility. Oncotarget 2015;6:20863-74. [Crossref] [PubMed]
- Tatsuguchi A, Yamada T, Ueda K, et al. Genetic analysis of Japanese patients with small bowel adenocarcinoma using next-generation sequencing. BMC Cancer 2022;22:723. [Crossref] [PubMed]
- Li L, Li M, Wang X. Cancer type-dependent correlations between TP53 mutations and antitumor immunity. DNA Repair (Amst) 2020;88:102785. [Crossref] [PubMed]
- Rodriguez-Meira A, Norfo R, Wen S, et al. Single-cell multi-omics identifies chronic inflammation as a driver of TP53-mutant leukemic evolution. Nat Genet 2023;55:1531-41. [Crossref] [PubMed]
- Parikh K, Dimou A, Leventakos K, et al. Impact of EML4-ALK Variants and Co-Occurring TP53 Mutations on Duration of First-Line ALK Tyrosine Kinase Inhibitor Treatment and Overall Survival in ALK Fusion-Positive NSCLC: Real-World Outcomes From the GuardantINFORM database. J Thorac Oncol 2024;19:1539-49. [Crossref] [PubMed]
- Öner MG, Rokavec M, Kaller M, et al. Combined Inactivation of TP53 and MIR34A Promotes Colorectal Cancer Development and Progression in Mice Via Increasing Levels of IL6R and PAI1. Gastroenterology 2018;155:1868-82. [Crossref] [PubMed]
- Zhang SD, McCrudden CM, Kwok HF. Prognostic significance of combining VEGFA, FLT1 and KDR mRNA expression in lung cancer. Oncol Lett 2015;10:1893-901. [Crossref] [PubMed]
- Cho S, Kitadai Y, Yoshida S, et al. Deletion of the KIT gene is associated with liver metastasis and poor prognosis in patients with gastrointestinal stromal tumor in the stomach. Int J Oncol 2006;28:1361-7. [Crossref] [PubMed]
- Jun SY, Kim M, Jin Gu M, et al. Clinicopathologic and prognostic associations of KRAS and BRAF mutations in small intestinal adenocarcinoma. Mod Pathol 2016;29:402-15. [Crossref] [PubMed]
- Tharin Z, Richard C, Derangère V, et al. PIK3CA and PIK3R1 tumor mutational landscape in a pan-cancer patient cohort and its association with pathway activation and treatment efficacy. Sci Rep 2023;13:4467. [Crossref] [PubMed]
- Philpott C, Tovell H, Frayling IM, et al. The NF1 somatic mutational landscape in sporadic human cancers. Hum Genomics 2017;11:13. [Crossref] [PubMed]
- Funato K, Yamazumi Y, Oda T, et al. Tyrosine phosphatase PTPRD suppresses colon cancer cell migration in coordination with CD44. Exp Ther Med 2011;2:457-63. [Crossref] [PubMed]
- Bae WJ, Ahn JM, Byeon HE, et al. PTPRD-inactivation-induced CXCL8 promotes angiogenesis and metastasis in gastric cancer and is inhibited by metformin. J Exp Clin Cancer Res 2019;38:484. [Crossref] [PubMed]
- Ou C, Peng Q, Zeng C. An integrative prognostic and immune analysis of PTPRD in cancer. Math Biosci Eng 2022;19:5361-79. [Crossref] [PubMed]
- Meškytė EM, Keskas S, Ciribilli Y. MYC as a Multifaceted Regulator of Tumor Microenvironment Leading to Metastasis. Int J Mol Sci 2020;21:7710. [Crossref] [PubMed]
- Shi WK, Li YH, Bai XS, et al. The Cell Cycle-Associated Protein CDKN2A May Promotes Colorectal Cancer Cell Metastasis by Inducing Epithelial-Mesenchymal Transition. Front Oncol 2022;12:834235. [Crossref] [PubMed]
(English Language Editor: J. Gray)