Validation of multiple deep learning models for colorectal tumor differentiation with endoscopic ultrasound images: a dual-center study
Highlight box
Key findings
• Endoscopic ultrasound with artificial intelligence (EUS-AI) demonstrated superior diagnostic performance for colorectal cancer (CRC) and adenoma compared to experienced endoscopists. Residual Network 50 is a promising tool for improving diagnostic accuracy in clinical practice.
What is known and what is new?
• Previous studies have shown the potential of AI in medical imaging, but most were limited by small sample sizes or single-center data.
• Our study is the first to validate multiple EUS-AI models using a large, dual-center dataset, demonstrating superior performance over experienced endoscopists. We introduced a three-category classification (CRC, adenoma, and controls), providing a more comprehensive diagnostic approach compared to previous binary classifications.
What is the implication, and what should change now?
• The findings suggest that EUS-AI models, particularly ResNet-50, could significantly enhance the accuracy of colorectal tumor diagnosis in clinical settings. However, its clinical value over white-light endoscopy/narrow-band imaging remains uncertain. Prospective studies are needed to determine whether EUS-AI influences clinical management. Furthermore, future work should focus on expanding datasets and exploring advanced model architectures to further improve performance and generalizability. Additionally, multicenter validation studies are needed to confirm the robustness of these models across diverse patient populations.
Introduction
Colorectal cancer (CRC) ranks third in terms of incidence and is the second leading cause of cancer-related mortality worldwide (1). Approximately 20% of patients newly diagnosed with CRC are diagnosed with metastasis (2). Patients diagnosed with metastatic colorectal cancer (mCRC) often face poor prognosis and a low quality of life. It is crucial to identify and classify colorectal tumors early, prior to the progression to mCRC. Most CRCs evolve from adenomas, which often serve as clinically significant precursors to CRC (3,4). The adenoma detection rate (ADR) is inversely associated with the risks of interval CRC, advanced-stage interval cancer, and fatal interval cancer (5). Early detection and removal of colorectal adenoma are thus essential for reducing CRC morbidity and mortality (6-8). Current diagnostic methods for colorectal lesions include endoscopy, computed tomography (CT) colonography, laboratory tests, and histopathological examination (9). Endoscopy is recommended for the detection of CRC and adenoma. However, endoscopy is limited, as it can only detect mucosal surface abnormalities, lacking the capability to evaluate oncologic activity (10).
Endoscopic ultrasound (EUS), an invasive screening technique, offers distinct advantages for the diagnosis of gastrointestinal lesions, enabling the differentiation of benign and malignant colorectal tumors based on the location and detailed morphology of the lesions (11-13). However, the diagnostic accuracy of EUS is highly dependent on the experience of the endoscopist, leading to variability in clinical practice (14). Deep learning (DL) models and convolutional neural networks (CNNs) are among the most successful algorithms used in the medicine field in recent years (15). Combining artificial intelligence (AI) with ultrasound or endoscopy has led to considerable advances in the diagnosis of liver tumors (16), esophageal cancer (17), and gastric cancer (18). Using DL models to assist clinicians on diagnosing CRC via EUS images can lighten workload and increase accuracy.
Carter et al. (19) demonstrated the feasibility of using DL to detect rectal cancer in endorectal ultrasonography (ERUS) images. While EUS is traditionally used for rectal cancer staging and subepithelial lesion evaluation, emerging evidence supports its application in proximal colorectal lesions. Song et al. (13) proposed a computer-aided diagnosis (CAD) system for distinguishing benign and early malignant tumors using a deep neural network (DNN) model in proximal colorectal locations. Their system incorporated EUS image analysis and demonstrated effectiveness in their dataset. Both studies highlighted the potential of AI in improving CRC diagnosis compared to conventional EUS interpretation but require further validation in clinical practice. However, these and other studies on this subject have the following limitations. First, the bulk of this research has been limited by an insufficient number of images. Second, the applicability of EUS-AI studies conducted in a single-center studies is limited. Third, EUS-AI has only been applied for binary classification (cancer vs. non-cancer) and may not adequately reflect practical clinical applications. AI-based diagnosis of colorectal tumors on EUS images based on larger, multicenter data may have greater generalizability and practical applicability in the differential diagnosis.
To further evaluate the feasibility of EUS-AI for colorectal lesions differentiation and broaden its clinical applicability, we conducted this study to validated the efficacy of EUS-AI systems in diagnosing CRC and adenoma via EUS images based on two large centered datasets. We present this article in accordance with the TRIPOD reporting checklist (available at https://jgo.amegroups.com/article/view/10.21037/jgo-2024-1024/rc).
Methods
Patients
In our study, we aimed to maximize the number of available images to enhance the robustness and generalizability of our AI models. We collected a large dataset of EUS images from Zhujiang Hospital of Southern Medical University and Nanfang Hospital of Southern Medical University, Guangzhou, Guangdong, China, between January 2020 and December 2023. This retrospective study included colorectal tumor specimens from surgical or endoscopic resection that were confirmed via pathology. To validate the efficacy of the EUS-AI models for diagnosing CRC and adenoma, the EUS images were classified into three groups (CRC, adenoma, and controls). The inclusion criteria were as follows: (I) EUS performed for colorectal lesions by EUS expert between January 2020 and December 2023; and (II) available data on histopathological diagnoses. The exclusion criteria were (I) images of non-diseased areas; (II) a history of inflammatory bowel disease, colon or rectal resection, and chemotherapy or radiotherapy to the abdomen; (III) poor-quality EUS images caused by air in the colorectum or strong artifacts. Patients or images that did not meet the inclusion criteria or that met any of the exclusion criteria were excluded and not included in this study.
The study was conducted in accordance with the Declaration of Helsinki and its subsequent amendments. The study was approved by Institutional Ethics Committees of Zhujiang Hospital (No. 2024-KY-181-01) and Nanfang Hospital of Southern Medical University (No. NFEC-2022-170). Informed consent was taken from all individual participants.
EUS images
All EUS images were acquired by EUS experts with at least 3 years of experience in EUS procedures using a conventional echoendoscope (GF-UE260-AL5 and GF-UCT260, Olympus Corporation, Tokyo, Japan; EG-530UR2 and EG-580U, Fujifilm Corporation, Tokyo, Japan) at 5–20 MHz, mini-probes (Fujifilm Corporation: UM-2R, frequency 12 MHz; UM-3R, frequency 20 MHz; P-2726-12, frequency 12 MHz; P-2726-20, frequency 20 MHz) and ultrasound systems (EU-ME1 or EU-ME2, Olympus Corporation; Fujifilm 7000, Fujifilm Corporation; ARIETTA 850, Prosound F75, or Prosound SSDα-10, Hitachi Aloka Medical, Tokyo, Japan). For proximal colorectal lesions, imaging was performed using a combination of long-length echoendoscopes (Olympus GF-UCT260) and through-the-scope mini-probes. Mini-probes were advanced through the accessory channel of a colonoscope (Olympus CF-HQ190) under fluoroscopic guidance (Siemens Artis Q system; Siemens Healthineers AG, Erlangen, Germany) to ensure precise positioning. Lesions in the transverse or descending colon were imaged with patients in modified lateral decubitus positions to optimize probe contact. The frequency was set high (12 or 20 MHz) for the observation of the originating layer of the lesion and was changed to a lower frequency (5–7.5 MHz) when the entire image could not be obtained. To ensure the quality of the EUS images, the following points were considered: (I) filling with sufficient water to completely submerge the lesion; (II) the use of sufficient water to remove residue and mucus from the intestinal canal before EUS images were acquired; and (III) slight lifting of the probe from the lesion to avoid deformation of the lesion.
Predictors and outcome
In our study, the predictors used for model training and testing were the visual features extracted from EUS images, specifically the regions of interest (ROIs) manually marked by experienced endoscopists and the corresponding labels based on histopathological confirmation. No additional clinical or pathological variables (such as lesion size, location, and patient demographics) were included as predictors. To ensure the robustness and consistency of the input data, all EUS images were preprocessed as follows: ultrasound images were converted to JPEG format using the NEXUS viewing software (Fujifilm Medical, Tokyo, Japan). The outlines of the abnormal tumors were manually labeled by two endoscopists with at least 3 years of experience in EUS procedures, and the tumor portion was labeled with an external frame.
In this study, we classified other benign tumors (e.g., lipomas, fibromas, and polyps) into the control category. These benign tumors are typically slow-growing, well-defined, and nonmetastatic, and they differ significantly from CRC and adenomas in their biological characteristics and clinical presentation. This classification approach allowed us to focus on distinguishing between malignant and precancerous lesions while minimizing the impact of benign tumor diversity on model performance. The outcome was the classification of tumor types into three categories: cancer, adenoma, and controls (other noncancerous or nonadenomatous tumors).
In the training phase, models were trained on individual EUS images to learn visual features associated with each category. During inference, multiple images from each patient were predicted, and a majority voting strategy was used to determine the patient-level classification. Specifically, the category with the most votes among a patient’s images was assigned as the patient’s final classification. For example, if three out of five images for a patient were predicted to be cancer, the patient was classified in the cancer category. The final classification accuracy was calculated based on these patient-level majority voting results, rendering the model’s performance evaluation more clinically relevant.
Building training and test datasets
All pathologically confirmed colorectal lesions were categorized into three groups: CRC, adenoma, and control. In this study, we divided the entire dataset into a training dataset and a test dataset, which were mutually exclusive at a ratio of 2:1, using random sampling based on cases rather than images. All EUS images were trimmed to the same size of 224×224 square pixels.
Development of the AI system
We developed a system for classifying EUS images, with the aim of automating the diagnostic process. Our model architecture follows the “feature extractor + multilayer perceptron (MLP) classifier” framework. We incorporated three CNN-based feature extractors, namely ResNet50, EfficientNet-B0, and Visual Geometry Group 11_BN (VGG_11_BN) and included Vision Transformer (ViT)-based model as a feature extractor. The detailed illustration of the model architectures for the four different feature extractors used are shown in Figure 1. For the MLP classifier, we used a single linear layer with three output channels to match our three categories. All models were implemented using PyTorch, a compact yet highly effective model for our specific applications (https://github.com/lukemelas/EfficientNet-PyTorch).
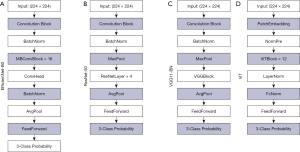
During the training phase, we used pretrained weights from the ImageNet dataset to initialize the feature extractors and fine-tuned all layers for optimal convergence. Our experiment involved categorizing images from our dataset into three distinct classes: CRC, adenoma, and other lesions. To enhance our model’s precision, we carefully labeled the images, marking the lesion areas with bounding boxes. We then expanded these bounding boxes by 1.5 times and cropped the corresponding regions for both training and testing.
All images were then resized to uniform squares (224×224 pixels) to fit the input size for the original deep learning algorithm. During training, the algorithm’s parameters were gradually adjusted to reduce the discrepancy between the actual and predicted values. We used cross-entropy loss to optimize our models.
Our strategy focuses on patient-based classification, ensuring strong generalizability. We used the Adam optimizer with a learning rate of 1e−6 and a maximum of 200 epochs for all models. All experiments were conducted using a single Nvidia RTX A6000 GPU (Nvidia, Santa Clara, CA, USA).
Figure 2 outlines the complete structure of our AI system. The process begins with obtaining original images of various sizes from hospital databases. These images are then manually marked with bounding boxes in a step we call “Cut”, to highlight the ROIs. This involves cropping the image to match the exact dimensions of the bounding box. To ensure the model’s robustness and retain important background context, the ROIs are enlarged to 1.5 times their original size before being fed into the AI model, a step we refer to as “Broaden”. The AI model follows a “feature extractor + MLP classifier” framework. The four unique feature extractors included in the AI system are, depicted in Figure 1, and more detailed visuals of these feature extractors can be found in Figure 2. The MLP classifier consists of a single linear layer with three output channels, matching our three categories. The classifier produces a probability distribution across these three categories, with the total probabilities adding up to 1. The category with the highest probability is chosen as the predicted category. For models that use the ViT architecture, the image is split into patches to meet the Transformer architecture’s requirements, a process we call “Patch”.

Blinding in predictor assessment
To ensure unbiased evaluation, we implemented specific blinding procedures in our study. (I) Images from the same patient were exclusively allocated to either the training or testing sets to prevent data leakage. (II) Histopathological diagnoses, serving as the gold standard, were accessed only by two endoscopists who together performed ROIs annotation and classification during model training. (III) For model validation, all test images were assessed independently by expert and non-expert endoscopists who were completely naive to the whole dataset and pathology.
Outcome measures
The preliminary outcome measure was the diagnostic performances of the four EUS-AI models for diagnosing CRC and adenoma among the three-category classifications (CRC, adenoma, and control), and the primary outcome measure was the difference in performance between the most accurate EUS-AI model and the four endoscopists in diagnosing CRC and adenoma. Among the four endoscopists, two experts were member of Endoscopic Ultrasonography Group of Guangdong Anti-Cancer Association. Both experts had more than 5 years of experience in evaluating colorectal lesions and had conducted more than 1,500 EUS examinations of colorectal lesions. Two nonexperts had 3–5 years of experience and had conducted fewer than 500 EUS examinations. Four EUS-AI models were trained by the same training dataset and tested by the same testing dataset. Four endoscopists were requested to make a diagnosis of “CRC”, “adenoma” or “controls” for each patient in the identical testing dataset with that of the EUS-AI models. During the statistical analysis, the four endoscopists were divided into two groups, experts and nonexperts. The best-performing model was selected through a comparison of the F1 scores among the different EUS-AI models. The final diagnosis was evaluated by comparing the diagnostic results of the selected EUS-AI model and the two endoscopists groups (experts and nonexperts), using metrics including accuracy, sensitivity, specificity, positive predictive value (PPV), and negative predictive value (NPV).
Statistical analysis
A two-sided McNemar test with a significance level of 0.05 was used to compare differences in accuracy, sensitivity, and specificity. Continuous variables are expressed as medians and ranges. Categorical variables are expressed as percentages. The Kruskal-Wallis test was used for continuous variables, and the Fisher exact test was used for categorical variables. F1 scores were used to evaluate and compare the performance of different EUS-AI models. The accuracy of the three-category classification performance was compared among the four EUS-AI models and endoscopists. The accuracy, sensitivity, specificity, PPV and NPV of the selected EUS-AI models and four endoscopists for diagnosing CRC and adenoma were calculated separately. Statistical significance was set at P<0.05, and all tests were two-sided. Interobserver agreement among the EUS-AI models, experts, and nonexperts was assessed using the kappa statistic. SPSS version 26.0 (IBM Corp., Armonk, NY, USA) was used for all the statistical analyses.
Results
Characteristics of the AI models
The progression of DL techniques, specifically CNNs and Transformer models, has led to substantial improvements in the classification of medical images, enhancing diagnostic capabilities. CNN and ViT are two representative DL models that are widely used in computer vision and image classification. EfficientNet-B0, Residual Network (ResNet), and Visual Geometry Group (VGG) are all CNN models. To obtain better performance, EfficientNet-B0 scales all the different dimensions uniformly using a simple but effective composite coefficient, ResNet introduces residual learning to overcome the degradation problem of deeper networks, and VGG combines numerous 3×3 convolutions followed by 2×2 max pooling results in a highly efficient and effective DL architecture. The ViT model, which is based on the Transformer architecture and embeds patches through self-attention mechanisms within the Transformer to learn global features from the image data effectively, has demonstrated exceptional performance on large-scale datasets (20).
Characteristics of the patients
A flow chart of the patient inclusion process is shown in Figure 3.
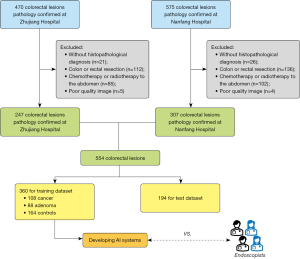
A total of 1,045 patients with suspected colorectal tumors were identified; among them, 47 patients with no histopathological confirmation (violating inclusion criterion II), 248 with postoperative review (meeting exclusion criterion II), and 187 treated with chemotherapy (meeting exclusion criterion III), and 9 with poor-quality EUS images (meeting exclusion criterion V) were excluded, leaving 554 patients for inclusion in the analysis of this study. A total of 8,738 images were collected from the 554 pathologically confirmed colorectal tumors, and there were 167 CRC cases, 136 colorectal adenoma cases, and 251 controls. After random sampling, 5,810 images from 360 patients (1,453 images from 88 colorectal adenomas, 2,629 images from 108 CRCs, and 1,728 images from 164 controls) were used as the training dataset, and 2,928 images from 194 patients (858 images from 48 colorectal adenomas, 1,250 images from CRCs, and 820 images from 87 controls) were used as the test dataset.
Table 1 provides the detailed clinical characteristics of the patients in the training and test sets. The differences were not significant for age, sex, lesion location, lesion size on EUS images, CRC tumor stage, or adenoma category between the training and test datasets.
Table 1
Characteristics | Training (n=360) | Test (n=194) | H/χ2 | P value |
---|---|---|---|---|
Age (years), median (range) | 55 (10–85) | 56 (13–90) | 0.474 | 0.49 |
Sex, n (%) | <0.001 | 0.98 | ||
Male | 200 (55.6) | 108 (55.7) | ||
Female | 160 (44.4) | 86 (44.3) | ||
Tumor size (mm), median (range) | 13.8 (1.3–144) | 14.7 (2.0–66.5) | 0.912 | 0.34 |
Lesion location, n (%) | 0.927 | 0.92 | ||
Colon | 175 (48.6) | 90 (46.4) | ||
Ascending | 71 (19.7) | 33 (17.0) | ||
Transverse | 27 (7.5) | 17 (8.8) | ||
Descending | 22 (6.1) | 12 (6.2) | ||
Sigmoid | 55 (15.3) | 28 (14.4) | ||
Rectum | 185 (51.4) | 104 (53.6) | ||
Pathological type, n (%) | 0.026 | 0.99 | ||
Cancer | 108 (30.0) | 59 (30.4) | ||
Adenoma | 88 (24.4) | 48 (24.8) | ||
Controls | 164 (45.6) | 87 (44.8) | ||
GIST | 26 (15.9) | 12 (13.8) | ||
Leiomyoma | 4 (2.4) | 8 (9.2) | ||
Schwannoma | 4 (2.4) | 3 (3.4) | ||
NET | 69 (42.1) | 38 (43.7) | ||
Lipoma | 61 (37.2) | 26 (29.9) | ||
Cancer (T staging), n (%) | 6.779 | 0.15 | ||
Tis | 32 (29.6) | 21 (35.6) | ||
T1 | 18 (16.7) | 7 (11.9) | ||
T2 | 13 (12.0) | 3 (5.1) | ||
T3 | 21 (19.4) | 7 (11.9) | ||
T4 | 24 (22.2) | 21 (35.6) | ||
Adenoma type, n (%) | 2.866 | 0.60 | ||
Tubular | 36 (40.9) | 26 (54.2) | ||
Tubular-villous | 32 (36.4) | 12 (25.0) | ||
Villous | 3 (3.4) | 2 (4.1) | ||
Serrated | 2 (2.3) | 1 (2.1) | ||
Adenomatous polyps | 15 (17.0) | 7 (14.6) |
Controls are neither cancer nor adenoma. GIST, gastrointestinal stromal tumor; NET, neuroendocrine tumor.
Predictions of the EUS-AI models and endoscopists
Figure 4 displays three representative test set images (CRC, adenoma, and control), while Table 2 presents the predicted probabilities for the CRC image from four EUS-AI models. In the test set, there were three predictions results for the EUS-AI: “0”, “1”, and “2”, representing the probability of “CRC”, “adenoma”, and “controls”, respectively.

Table 2
Model | Output results |
---|---|
VGG_11_BN | Predict:0, score: [0.9958875775337219, 0.004112320486456156, 7.552134206889605e−08] |
ResNet50 | Predict:0, score: [0.9999969005584717, 2.9347788768063765e−86, 1.443480073248793e−07] |
ViT | Predict:0, score: [0.5161225199699402, 0.4329724609851837, 0.0509050190448761] |
EfficientNet-B0 | Predict:0, score: [0.9916547536849976, 0.00809609703719616, 0.00024913367815315723] |
AI, artificial intelligence; CRC, colorectal cancer; EUS, endoscopic ultrasonography; ResNet50, Residual Network 50; VGG_11_BN, Visual Geometry Group 11_BN; ViT, Vision Transformer.
Performance assessment of DL models
In the context of differentiating precancerous and malignant tumors in CRC, we focused on two key metrics, accuracy and recall, indicating the model’s accuracy and its capacity to minimize misclassifying errors. A model achieved a high recall rate in identifying cancer and adenoma from a large dataset, indicating its strong ability to capture characteristics of cancer and adenoma. High recall is crucial for detecting the majority of cancerous and adenomatous lesions. Concurrently, higher accuracy reduces the chance of the model incorrectly classifying controls (non-tumorigenic or non-precancerous lesions) as CRC or precancer, resulting in fewer false positives. Given these considerations, the F1 scores, serving as the harmonic mean of precision and recall, offers a comprehensive assessment of the model’s overall performance. Moreover, macro-F1 is more suitable for imbalanced datasets with large variations in class numbers, as it provides a better reflection of the classification performance on minority classes.
During our training experiment, we implemented an early stopping strategy during our training experiment. Model training would be terminated upon observation of no substantial improvement in loss and accuracy over a predefined number of epochs, indicating saturation in model performance. Initially, the data were divided into training, validation, and test sets. However, the training loss curve indicated overfitting, and model performance was suboptimal. To address this, we increased the sample size and ultimately retained only training and test sets. Although overfitting remained to some extent, the model demonstrated improved performance. The omission of a separate validation set or cross-validation was due to the requirement for a larger sample size in model development. We considered that the currently collected samples were insufficient to be further divided for validation or cross-validation. Figure 5 shows the final results generated after continuous adjustment of the models to reach optimal performance. We observed that while the loss curve continued to decrease, the loss on the test dataset stilled to overfitting. Further validation of the models on the test set was conducted.

Three‑category classification performance
The confusion matrix for the per-category diagnostic performance of the four EUS-AI models and endoscopists is presented in Table 3. The accuracies of the four EUS-AI models (VGG_11_BN, ViT, EfficientNet-B0 and ResNet-50) for the three-category classification (cancer, adenoma, and controls) were 70.10%, 63.40%, 68.56% and 70.62%, respectively, among which ResNet-50 had the highest accuracy of 70.62%. The accuracy ranged from 45.88% to 60.31% for all the endoscopists, with the accuracy of experts and nonexperts being 57.22–60.31% and 45.88–46.39%. The accuracies of the experts were greater than those of the nonexperts (P<0.01). Compared to that of all the endoscopists, the accuracy of ResNet-50 (P=0.007), VGG_11_BN (P=0.001), and EfficientNet-B0 (P=0.02) were significantly greater. As shown in Table 4, the interobserver agreement among the AI systems (Fleiss’ κ =0.674) and the experts (Fleiss’ κ =0.557) for the three-category classification was fair, and that among the nonexperts was slight (Fleiss’ κ =0.284).
Table 3
Pathological type | EUS diagnosis | Total | Accuracy (%) | ||
---|---|---|---|---|---|
Cancer | Adenoma | Controls | |||
VGG_11_BN | 70.10 | ||||
Cancer | 42 | 14 | 3 | 59 | |
Adenoma | 16 | 22 | 10 | 48 | |
Controls | 4 | 11 | 72 | 87 | |
ViT | 63.40 | ||||
Cancer | 43 | 13 | 3 | 59 | |
Adenoma | 21 | 15 | 12 | 48 | |
Controls | 16 | 6 | 65 | 87 | |
EfficientNet-B0 | 68.56 | ||||
Cancer | 45 | 11 | 3 | 59 | |
Adenoma | 15 | 21 | 12 | 48 | |
Controls | 9 | 11 | 67 | 87 | |
ResNet-50 | 70.62 | ||||
Cancer | 43 | 11 | 5 | 59 | |
Adenoma | 14 | 23 | 11 | 48 | |
Controls | 7 | 9 | 71 | 87 | |
Expert1 | 57.22 | ||||
Cancer | 37 | 12 | 10 | 59 | |
Adenoma | 25 | 16 | 7 | 48 | |
Controls | 22 | 7 | 58 | 87 | |
Expert2 | 60.31 | ||||
Cancer | 39 | 10 | 10 | 59 | |
Adenoma | 24 | 17 | 7 | 48 | |
Controls | 20 | 6 | 61 | 87 | |
Nonexpert1 | 46.39 | ||||
Cancer | 30 | 18 | 11 | 59 | |
Adenoma | 32 | 11 | 5 | 48 | |
Controls | 31 | 7 | 49 | 87 | |
Nonexpert2 | 45.88 | ||||
Cancer | 31 | 18 | 10 | 59 | |
Adenoma | 29 | 12 | 7 | 48 | |
Controls | 32 | 9 | 46 | 87 |
Controls are neither cancer nor adenoma. AI, artificial intelligence; EUS, endoscopic ultrasonography; ResNet50, Residual Network 50; VGG_11_BN, Visual Geometry Group 11_BN; ViT, Vision Transformer.
Table 4
Group | 95% CI | Fleiss kappa | P value |
---|---|---|---|
AI models | 0.632, 0.715 | 0.674 | <0.001 |
Experts | 0.453, 0.661 | 0.557 | <0.001 |
Non-experts | 0.181, 0.387 | 0.284 | <0.001 |
AI, artificial intelligence; CI, confidence interval.
Comparisons of model performance are presented in Table 5. Given that ResNet-50 achieved the highest F1 scores for the three-category classification task, it was selected as the superior model that would be compared with the four endoscopists in diagnosing CRC and adenoma.
Table 5
Models | Recall (%) | Precision (%) | F1 (%) |
---|---|---|---|
VGG_11_BN | 70.12* | ||
Cancer | 71.19 | 67.74 | 69.42 |
Adenoma | 45.83 | 46.81 | 46.32 |
Control | 82.76 | 84.71 | 83.72 |
ViT | 62.78* | ||
Cancer | 72.88 | 53.75 | 61.87 |
Adenoma | 31.25 | 44.12 | 36.59 |
Control | 76.71 | 81.25 | 77.84 |
EfficientNet-B0 | 68.36* | ||
Cancer | 76.27 | 65.22 | 70.31 |
Adenoma | 43.75 | 48.84 | 46.15 |
Control | 77.01 | 81.71 | 79.29 |
ResNet-50 | 70.37* | ||
Cancer | 72.88 | 67.19 | 69.92 |
Adenoma | 47.92 | 53.49 | 50.55 |
Control | 81.61 | 81.61 | 81.61 |
Controls: non-cancerous or non-precancerous lesions. *, F1-weighted: the weighted average F1 score across all classes, where the weights are the number of true instances for each class. ResNet50, Residual Network 50; VGG_11_BN, Visual Geometry Group 11_BN; ViT, Vision Transformer.
The diagnostic yields of ResNet-50 and endoscopists for CRC and adenoma are summarized in Table 6 (principal analysis). The per-category sensitivity of ResNet-50 and endoscopists were the highest for CRC (72.88% and 62.71–66.10%, respectively) and lowest for adenoma (47.92% and 33.33–35.42%, respectively).
Table 6
Pathological type | Diagnostic performance (%) | ||||
---|---|---|---|---|---|
Accuracy | Sensitivity | Specificity | PPV | NPV | |
Cancer† | |||||
ResNet-50 | 80.93 | 72.88 | 84.44 | 67.19 | 87.69 |
All endoscopists | 59.54 | 58.08 | 60.01 | 39.25 | 76.46 |
All experts | 65.72 | 64.41 | 66.30 | 45.52 | 81.00 |
Expert1 | 64.43 | 62.71 | 65.19 | 44.05 | 80.00 |
Expert2 | 67.01 | 66.10 | 67.41 | 46.99 | 81.99 |
All nonexperts | 53.35 | 51.70 | 53.73 | 32.98 | 71.92 |
Nonexpert1 | 52.58 | 50.85 | 53.33 | 32.26 | 71.29 |
Nonexpert2 | 54.12 | 52.54 | 54.12 | 33.70 | 72.55 |
Adenoma‡ | |||||
ResNet-50 | 76.80 | 47.92 | 86.30 | 53.49 | 83.44 |
All endoscopists | 71.27 | 29.17 | 85.11 | 39.64 | 78.50 |
All experts | 74.75 | 34.38 | 88.02 | 48.61 | 80.32 |
Expert1 | 73.71 | 33.33 | 86.99 | 45.71 | 79.88 |
Expert2 | 75.78 | 35.42 | 89.04 | 51.51 | 80.75 |
All nonexperts | 67.79 | 23.96 | 82.20 | 30.67 | 76.68 |
Nonexpert1 | 68.04 | 22.92 | 82.88 | 30.56 | 76.58 |
Nonexpert2 | 67.53 | 25.00 | 81.51 | 30.77 | 76.77 |
†, the performance in differentiating colorectal cancer from non-malignant diseases; ‡, the performance in differentiating adenomas from non-adenoma diseases. AI, artificial intelligence; EUS, endoscopic ultrasonography; NPV, negative predictive value; PPV, positive predictive value; ResNet50, Residual Network 50.
For the diagnosis of cancer (Table 6), the accuracy, sensitivity, specificity, and PPV and NPV of the ResNet-50 were 80.93%, 72.88%, 84.44%, 67.19% and 87.69%, respectively, while for the experts, these values were 74.75% (range, 73.71–75.78%), 64.41% (range, 62.71–66.10%), 66.30% (range, 65.19–67.41%), 45.52% (range, 44.05–46.99%) and 81.00% (range, 80.00–81.99%), respectively. The accuracy of ResNet-50 was greater than that of all endoscopists (P<0.05), its specificity was greater than that of all the experts (P<0.001), and its sensitivity was greater than that of all the nonexperts (P<0.05). The interobserver agreement among the experts (Fleiss’ κ=0.590) for differentiating cancers from noncancers was fair, and that among the nonexperts was slight (Fleiss’ κ=0.184).
For the diagnosis of adenoma (Table 6), the accuracy, sensitivity, specificity, and PPV and NPV of the ResNet-50 were 76.80%, 47.92%, 86.30%, 53.49%, and 83.44%, respectively, while for the EUS experts, these values were 74.75% (range, 73.7–75.78%), 34.38% (range, 33.33–35.42%), 88.02% (range, 86.99–89.04%), 48.61% (range, 45.71–51.51%) and 80.32% (range, 79.88–80.75%), respectively. There was no significant difference between the sensitivity of ResNet-50 and those of the experts, while the sensitivity of ResNet50 was greater than those of the nonexperts (47.92% vs. 22.92–25.00%, P<0.05). There was no significant difference between the specificity of ResNet-50 and that of any of the endoscopists. The interobserver agreement among the experts (Fleiss’ κ=0.216) and the nonexperts (Fleiss’ κ=0.223) was slight.
Discussion
In this study, we included histopathologically confirmed CRCs, colorectal adenomas, and other colorectal tumors from two centers in China. To our knowledge, this is the first study to evaluate the ability of multiple EUS-AI models to diagnose CRC and adenomas and to compare their performance with that of endoscopists. Importantly, we found that the EUS-AI model demonstrated a considerable had great potential capacity to promote improve the diagnosis performance of CRC and adenoma. A key strength of the study is its multi-center design, utilizing EUS images from two clinical centers. However, limitations such as sample size and study design may affect the generalizability of these findings. The performance of the AI models is also heavily reliant on the quality of the training data, which could limit their applicability if the data are not representative of all patient populations. Additionally, while this study highlights emerging evidence for EUS in assessing colorectal lesions, it does not directly compare EUS-AI with established techniques like white-light endoscopy (WLE) or narrow-band imaging (NBI), which already provide reliable mucosal assessments. The incremental diagnostic value of EUS-AI in routine practice remains unquantified. However, the success of this study demonstrates that EUS can provide valuable depth information for colorectal tumors that are difficult to differentiate as benign or malignant, offering a complementary perspective to conventional optical techniques like WLE/NBI. Given its unique strengths, integrating EUS with these established modalities may further enhance diagnostic precision and clinical decision-making. Future study should focus on not only compare EUS-AI with WLE/NBI but also investigate the potential benefits of integrating these modalities to optimize clinical outcomes. Moreover, although our results demonstrate improved diagnostic accuracy of EUS-AI for colorectal lesions, the clinical impact of applying EUS beyond the rectum remains uncertain. It is unclear whether EUS findings would influence clinical management, such as reducing unnecessary biopsies or guiding therapeutic decisions, compared to standard modalities. Given the technical complexity and invasiveness of EUS, its routine adoption for differentiating adenomas or early carcinoma requires further justification through prospective studies linking EUS-AI findings to actionable clinical outcomes, including resection strategies, recurrence rates, and survival benefits. Furthermore, this study did not utilize advanced endoscopic imaging techniques such as NBI, i-Scan, or chromoendoscopy during EUS evaluation. While these modalities are widely adopted to improve the detection and differentiation of colorectal lesions through enhanced mucosal visualization. Their absence in our protocol may lower the accuracy of EUS-AI models. Future research should explore the potential synergy between EUS-AI and advanced endoscopic imaging to optimize lesion classification and reduce reliance on invasive diagnostics.
AI-based image classification systems have been proven to be able to assist humans in lesion diagnosis (21,22). The integration of AI has made significant strides in the management of clinical diseases. Wagner et al. (23) introduced a novel Transformer-based pipeline that enhances the prediction of microsatellite instability (MSI) biomarkers from pathology slides in CRC. Wesp et al. (24) applied DL models using CT colonography images to classify polyps as premalignant or benign, yielding an area under the receiver operating characteristic curve of 0.83. Moreover, Dembrower et al. (25) demonstrated that AI could outperform traditional radiologist double reading, achieving a 4% higher non-inferior cancer detection rate when used for independent interpretation of screening mammograms.
In recent years, CAD systems have also been widely applied in the diagnosis of colorectal tumors using colonoscopy images. Chen et al. (26) used an artificial neural network (ANN) algorithm and shear wave elastography (SWE)-assisted EUSto predict tumor deposition (TD) in rectal cancer. Song et al. (13) combined several DNN models with deep multiview fusion (horizontal and longitudinal) in the diagnosis of colorectal tumors. Xu et al. (27), Wallace et al. (28) and Shaukat et al. (29) conducted AI-assisted colonoscopy in the differentiation of CRC and adenomas.
However, previous studies in this field have been limited by the following issues: a limited number of cases, tumors originating from a single rectum, or the application of AI for binary classification. Therefore, their results should be carefully considered, and their utility in real clinical practice scrutinized. Compared with conventional EUS, SWE is technically more difficult and more demanding for physicians (30). EUS combines ultrasound with endoscopic visualization, allowing for high-resolution and real-time visualization of the digestive tract lumen as compared with colonoscopy (31,32); however, EUS with multiview fusion increases the workload of endoscopists. Thus, on the basis of improved accuracy, applying EUS-AI models to three-category classification can not only reveal more types of colorectal tumors but also accelerate the diagnostic process of EUS, which is more in line with practical clinical applications, providing greater feasibility and clinical value. In contrast to previous studies that have focused on colorectal adenomas or polyps (5,33), our work employed AI to categorize the developmental stages of tumors. Notably, no other study has used three-category classification to assess EUS-AI models and endoscopists, so the diagnostic performance of the AI system in this study was compared to the diagnostic performance of the four endoscopists (two experts and two non-experts).
The diagnosis of EUS is subjective, and the accuracy is closely related to the endoscopist’s endoscopist’s knowledge, experience, and skill level. AI has the ability to process large amounts of data with high precision (34). When AI is used in conjunction with EUS, it provides objective, simple, and fast examination (12). Based on this, we combined several recently developed mainstream DNN structures, ResNet-50, EfficientNet-B0, VGG_11_BN, and Transformer-based ViT with EUS. EUS images of colorectal tumors were classified into three categories: CRC, adenoma, and controls (other colorectal tumors). The test results of four EUS-AI models were compared one another, and the one with the highest accuracy was further compared to that of four endoscopists to determine whether EUS-AI models provide adjunctive value in diagnosing CRC and adenoma.
As it is essential to fully test an AI system on a multicenter test cohort, this study included EUS images obtained from two clinical centers. In the three-category classification, the accuracies of ResNet-50, VGG_11_BN and EfficientNet-B0 were significantly higher than those of all the other endoscopists (70.62% vs. 70.10% vs. 68.56% vs. 46.39–60.31%, respectively), with the accuracy of ResNet-50 being higher than that of the best-performing expert by 10.31% (70.62% vs. 60.31%). ResNet-50 was selected to compete with endoscopists because it had the highest F1 score among the four EUS-AI models. In the diagnosis of CRC, the accuracy and specificity of ResNet-50 were significantly greater than those of all endoscopists, whose accuracy and specificity were 13.92% and 17.03%, respectively, and higher than those of the expert with the best performance (accuracy: 80.93% vs. 67.01%; specificity: 84.44% vs. 67.41%). The sensitivity of ResNet50 was significantly greater than that of the nonexperts. In diagnosing adenoma, the sensitivity of ResNet50 was significantly greater than that of the best performing nonexpert by 22.92% (47.92% vs. 25.00%).
Our findings are comparable to those reported in other studies involving the AI-assisted diagnosis of colorectal tumors. For instance, Carter et al. (19) reported an accuracy of 78% for CRC diagnosis using a similar EUS-AI model, while Song et al. (13) achieved an accuracy of 85% in a single-center study. We achieved an accuracy of 80.93% for binary classification (cancer and noncancer) in the test dataset, demonstrating the robustness of the ResNet-50 model. Despite the overfitting issue, the ResNet-50 model still demonstrated robust diagnostic accuracy in the validation dataset, with an accuracy of 80.93% and a high specificity of 84.44%. Even with a few limitations, the EUS-AI model could still be a valuable tool for assisting clinicians in diagnosing CRC and adenomas using EUS images. A noteworthy issue is that in the diagnosis of endoscopists, samples that are difficult to assess are more often regarded as malignant. This approach is used to avoid missing malignant samples as much as possible during diagnosis to avoid delays in patient treatment.
This study is unique in that the collected EUS images were divided into three categories, and the performance of four EUS-AI models was compared to that of endoscopists. CRC and its principal precursor—adenoma, which is strongly associated with poor patient prognosis—were listed separately, and the third group included other colorectal tumors.
Our study involved several limitations that should be acknowledged. First, the model exhibited overfitting, likely due to an insufficient sample size. Second, measures to reduce overfitting led to a significant decrease in model performance. Third, the majority voting strategy used during inference output discrete category labels rather than continuous probability values, preventing the calculation of the receiver operating characteristic and area under the curve. Future work should focus on expanding the dataset, exploring regularization techniques, and modifying the voting strategy to address these issues. The results of this article will be the cornerstone of our subsequent study. Our findings suggest that the EUS-AI models demonstrated considerable potential for diagnosing CRC and adenoma. However, the current study was not specifically designed to evaluate the AI system’s ability to distinguish T0 (non-invasive) from T1 (invasive) lesions. Future work is suggested to address this limitation by focusing on epithelial tumors and the diagnosis of adenomas with carcinoma in situ or those beyond T1 (indicating tumors that have infiltrated beyond the mucosa or submucosa).
Conclusions
The EUS-AI had promising application value in future medical practice. ResNet-50 can be used to assist endoscopists and anorectologists as a tool for diagnosing CRC and colorectal adenoma.
Acknowledgments
This work was supported by the Department of Gastroenterology at Zhujiang Hospital and Nanfang Hospital of Southern Medical University, as well as the computer team at Sun Yat-sen University.
Footnote
Reporting Checklist: The authors have completed the TRIPOD reporting checklist. Available at https://jgo.amegroups.com/article/view/10.21037/jgo-2024-1024/rc
Data Sharing Statement: Available at https://jgo.amegroups.com/article/view/10.21037/jgo-2024-1024/dss
Peer Review File: Available at https://jgo.amegroups.com/article/view/10.21037/jgo-2024-1024/prf
Funding: This research was supported by
Conflicts of Interest: All authors have completed the ICMJE uniform disclosure form (available at https://jgo.amegroups.com/article/view/10.21037/jgo-2024-1024/coif). H.M., X.P., H.L. and Z.L. report funding support from the Guangdong Provincial Science and Technology Innovation Project (grant No. pdjh2024b093). S.Q.J. and S.H. report funding support from the Southern Medical University Program (grant No. 202412121303) and the Guangdong Provincial Science and Technology Innovation Project (grant No. pdjh2024b093). T.O.Y. reports funding support from the Guangdong Provincial Natural Science Foundation (grant No. 2023A1515010729). Z.X. reports funding support from the Southern Medical University Program (grant No. 202412121303). F.X. reports funding support from the National Natural Science Foundation of China (grant No. 82470539), and Guangdong Provincial Natural Science Foundation (grant No. 2023A1515010729). Z.L. and S.H. reports funding support from the Guangdong Provincial Science and Technology Innovation Strategy Special Funds (grant No. 2023A0505010038). The other authors have no conflicts of interest to declare.
Ethical Statement: The authors are accountable for all aspects of the work in ensuring that questions related to the accuracy or integrity of any part of the work are appropriately investigated and resolved. The study was conducted in accordance with the Declaration of Helsinki and its subsequent amendments. The study was approved by Institutional Ethics Committees of Zhujiang Hospital (No. 2024-KY-181-01) and Nanfang Hospital of Southern Medical University (No. NFEC-2022-170). Informed consent was taken from all individual participants.
Open Access Statement: This is an Open Access article distributed in accordance with the Creative Commons Attribution-NonCommercial-NoDerivs 4.0 International License (CC BY-NC-ND 4.0), which permits the non-commercial replication and distribution of the article with the strict proviso that no changes or edits are made and the original work is properly cited (including links to both the formal publication through the relevant DOI and the license). See: https://creativecommons.org/licenses/by-nc-nd/4.0/.
References
- Sung H, Ferlay J, Siegel RL, et al. Global Cancer Statistics 2020: GLOBOCAN Estimates of Incidence and Mortality Worldwide for 36 Cancers in 185 Countries. CA Cancer J Clin 2021;71:209-49. [Crossref] [PubMed]
- Biller LH, Schrag D. Diagnosis and Treatment of Metastatic Colorectal Cancer: A Review. JAMA 2021;325:669-85. [Crossref] [PubMed]
- Chang WY, Chiu HM. Can image-enhanced endoscopy improve adenoma detection rate? Dig Endosc 2022;34:284-96. [Crossref] [PubMed]
- Gharib E, Robichaud GA. From Crypts to Cancer: A Holistic Perspective on Colorectal Carcinogenesis and Therapeutic Strategies. Int J Mol Sci 2024;25:9463. [Crossref] [PubMed]
- Lui TK, Lam CP, To EW, et al. Endocuff With or Without Artificial Intelligence-Assisted Colonoscopy in Detection of Colorectal Adenoma: A Randomized Colonoscopy Trial. Am J Gastroenterol 2024;119:1318-25. [Crossref] [PubMed]
- Wieszczy P, Kaminski MF, Franczyk R, et al. Colorectal Cancer Incidence and Mortality After Removal of Adenomas During Screening Colonoscopies. Gastroenterology 2020;158:875-883.e5. [Crossref] [PubMed]
- Duvvuri A, Chandrasekar VT, Srinivasan S, et al. Risk of Colorectal Cancer and Cancer Related Mortality After Detection of Low-risk or High-risk Adenomas, Compared With No Adenoma, at Index Colonoscopy: A Systematic Review and Meta-analysis. Gastroenterology 2021;160:1986-1996.e3. [Crossref] [PubMed]
- Chen B, Scurrah CR, McKinley ET, et al. Differential pre-malignant programs and microenvironment chart distinct paths to malignancy in human colorectal polyps. Cell 2021;184:6262-6280.e26. [Crossref] [PubMed]
- Gupta S. Screening for Colorectal Cancer. Hematol Oncol Clin North Am 2022;36:393-414. [Crossref] [PubMed]
- Kou S, Thakur S, Eltahir A, et al. A portable photoacoustic microscopy and ultrasound system for rectal cancer imaging. Photoacoustics 2024;39:100640. [Crossref] [PubMed]
- Zhu C, Hua Y, Zhang M, et al. A Multimodal Multipath Artificial Intelligence System for Diagnosing Gastric Protruded Lesions on Endoscopy and Endoscopic Ultrasonography Images. Clin Transl Gastroenterol 2023;14:e00551. [Crossref] [PubMed]
- Yang X, Wang H, Dong Q, et al. An artificial intelligence system for distinguishing between gastrointestinal stromal tumors and leiomyomas using endoscopic ultrasonography. Endoscopy 2022;54:251-61. [Crossref] [PubMed]
- Song D, Zhang Z, Li W, et al. Judgment of benign and early malignant colorectal tumors from ultrasound images with deep multi-View fusion. Comput Methods Programs Biomed 2022;215:106634. [Crossref] [PubMed]
- Kleemann T, Freund R, Braden B, et al. An international survey on the geographical differences in practice patterns and training of endoscopic ultrasound. J Transl Int Med 2025;13:48-64. [Crossref] [PubMed]
- Yin Z, Yao C, Zhang L, et al. Application of artificial intelligence in diagnosis and treatment of colorectal cancer: A novel Prospect. Front Med (Lausanne) 2023;10:1128084. [Crossref] [PubMed]
- Nishida N, Yamakawa M, Shiina T, et al. Artificial intelligence (AI) models for the ultrasonographic diagnosis of liver tumors and comparison of diagnostic accuracies between AI and human experts. J Gastroenterol 2022;57:309-21. [Crossref] [PubMed]
- Shimamoto Y, Ishihara R, Kato Y, et al. Real-time assessment of video images for esophageal squamous cell carcinoma invasion depth using artificial intelligence. J Gastroenterol 2020;55:1037-45. [Crossref] [PubMed]
- Uema R, Hayashi Y, Kizu T, et al. A novel artificial intelligence-based endoscopic ultrasonography diagnostic system for diagnosing the invasion depth of early gastric cancer. J Gastroenterol 2024;59:543-55. [Crossref] [PubMed]
- Carter D, Bykhovsky D, Hasky A, et al. Convolutional neural network deep learning model accurately detects rectal cancer in endoanal ultrasounds. Tech Coloproctol 2024;28:44. [Crossref] [PubMed]
- Dosovitskiy A, Beyer L, Kolesnikov A, et al. An Image is Worth 16x16 Words: Transformers for Image Recognition at Scale. 2021. Available online: https://iclr.cc/virtual/2021/oral/3458
- Maron RC, Haggenmüller S, von Kalle C, et al. Robustness of convolutional neural networks in recognition of pigmented skin lesions. Eur J Cancer 2021;145:81-91. [Crossref] [PubMed]
- Sharma P, Hassan C. Artificial Intelligence and Deep Learning for Upper Gastrointestinal Neoplasia. Gastroenterology 2022;162:1056-66. [Crossref] [PubMed]
- Wagner SJ, Reisenbüchler D, West NP, et al. Transformer-based biomarker prediction from colorectal cancer histology: A large-scale multicentric study. Cancer Cell 2023;41:1650-1661.e4. [Crossref] [PubMed]
- Wesp P, Grosu S, Graser A, et al. Deep learning in CT colonography: differentiating premalignant from benign colorectal polyps. Eur Radiol 2022;32:4749-59. [Crossref] [PubMed]
- Dembrower K, Crippa A, Colón E, et al. Artificial intelligence for breast cancer detection in screening mammography in Sweden: a prospective, population-based, paired-reader, non-inferiority study. Lancet Digit Health 2023;5:e703-11. [Crossref] [PubMed]
- Chen LD, Li W, Xian MF, et al. Preoperative prediction of tumour deposits in rectal cancer by an artificial neural network-based US radiomics model. Eur Radiol 2020;30:1969-79. [Crossref] [PubMed]
- Xu H, Tang RSY, Lam TYT, et al. Artificial Intelligence-Assisted Colonoscopy for Colorectal Cancer Screening: A Multicenter Randomized Controlled Trial. Clin Gastroenterol Hepatol 2023;21:337-346.e3. [Crossref] [PubMed]
- Wallace MB, Sharma P, Bhandari P, et al. Impact of Artificial Intelligence on Miss Rate of Colorectal Neoplasia. Gastroenterology 2022;163:295-304.e5. [Crossref] [PubMed]
- Shaukat A, Lichtenstein DR, Somers SC, et al. Computer-Aided Detection Improves Adenomas per Colonoscopy for Screening and Surveillance Colonoscopy: A Randomized Trial. Gastroenterology 2022;163:732-41. [Crossref] [PubMed]
- Filipov T, Teutsch B, Szabó A, et al. Investigating the role of ultrasound-based shear wave elastography in kidney transplanted patients: correlation between non-invasive fibrosis detection, kidney dysfunction and biopsy results-a systematic review and meta-analysis. J Nephrol 2024;37:1509-22. [Crossref] [PubMed]
- Sooklal S, Chahal P. Endoscopic Ultrasound. Surg Clin North Am 2020;100:1133-50. [Crossref] [PubMed]
- Huang J, Fan X, Liu W. Applications and Prospects of Artificial Intelligence-Assisted Endoscopic Ultrasound in Digestive System Diseases. Diagnostics (Basel) 2023;13:2815. [Crossref] [PubMed]
- Wang J, Li Y, Chen B, et al. A real-time deep learning-based system for colorectal polyp size estimation by white-light endoscopy: development and multicenter prospective validation. Endoscopy 2024;56:260-70. [Crossref] [PubMed]
- Wu X, Li W, Tu H. Big data and artificial intelligence in cancer research. Trends Cancer 2024;10:147-60. [Crossref] [PubMed]
(English Language Editor: J. Gray)