Identifying prognostic intratumor heterogeneity using pre- and post-radiotherapy 18F-FDG PET images for pancreatic cancer patients
Introduction
Unresectable pancreatic adenocarcinoma (PA) is associated with high mortality (1,2). Surgical resection is the only potentially curative treatment for PA (3,4). The use of chemoradiotherapy (CRT) in the management of borderline resectable (BR) and locally advanced (LA) PA has been historically used as a means to improve local control and down-staging to resectability (5-8). However, accurate evaluation of post-CRT response with standard body CT imaging and RECIST criteria is challenging and often fails to accurately characterize response (9,10). Indeed, identifying those patients at high likelihood for margin negative resection or escalation of radiation dose is essential to maximize the surgical benefit, reduce mortality of surgery and financial burden for those patients who potentially have no benefit from resection.
18F-FDG PET is often used in combination with diagnostic CT or MRI as part of the initial staging workup of pancreatic cancer patients, and has been investigated as a prognostic and predictive tool in patients receiving chemoradiation therapy (11-13). Additionally, pre- and post-treatment PET imaging for assessing response to therapy is an area of active investigation, in particular for adaptive radiotherapy (RT) techniques and development of neoadjuvant clinical protocols (14-16). Currently, the most common metrics used for assessment of PET metabolic response are the maximum and mean standard uptake values (SUV) (17). However, such indices provide only the global metabolic activities of the tumor and fail to accurately characterize locoregional tumor uptake, which critically associates with underlying biophysiologic tumorous transformation, such as perfusion, proliferation and tumor viability. Recent studies suggest that texture feature analysis is a feasible approach to characterize local FDG activity distribution, allowing for the assessment of corresponding biological heterogeneity (18-22).
We hypothesize that changes in treatment-related pancreatic tumor heterogeneity can be characterized by locoregional spatial variation using pre- and post-treatment PET imaging. The goal of this study is to assess the prognostic value of texture variation in predicting therapy response in patients with PA and stratifying the patient risks. A kernel-based approach was used to calculate locoregional texture features, further texture variations were derived by finding the fitting discrepancy of texture maps of pre- and post-treatment images. The prognostic heterogeneity features were selected from a large pool of clinical variables and image textures using lasso/elastic net regression (23-27), and further evaluated using multivariate Cox analysis associated with overall survival (OS). Since both imaging and clinical data can be acquired before surgery, the proposed method could facilitate screening potential patients for surgical resection.
Methods
Patients
With Institutional Review Board approval, 26 PA patients treated with RT from 2011–2013 were included in this retrospective study. All patients underwent 18F FDG-PET/CT scans before and after RT. Patient demographic and clinical outcomes data, including age, tumor stage, serum CA19-9, resectability, and resection status were acquired. Nineteen patients received external-beam RT and concurrent chemotherapy [gemcitabine (79%) or 5-FU (21%)], and seven patients were treated with stereotactic body radiation therapy (SBRT). The majority of patients received standard multi-agent chemotherapy (gemcitabine or 5FU-based) prior to initiating RT. Only patients with at least stable disease noted on post-chemo and pre-RT baseline PET/CT scans received treatment. Therefore, we believe they are an accurate representation of baseline local disease burden. Patients undergoing CRT received a total radiation dose of 54 Gy in 30 fractions and SBRT patients received a total of 30 Gy in 5 fractions. Ten patients underwent surgery after RT in this study.
Pretreatment whole-body 18F-FDG PET/CT was acquired for staging purposes. Approximately 6 weeks (6.3±3.3 weeks) after the completion of RT, patients were scanned with PET/CT to evaluate response to therapy. For PET scans, patients were instructed to fast for a minimum of 6 h before the injection of 18F-FDG. The dose of administered 18F-FDG was 5 MBq/kg, and static emission images were acquired from thigh to head, on average 54 minutes after injection. All PET scans were performed on a whole-body 64-slice PET/CT scanner (Biograph-64 TruePoint PET/CT; Siemens Medical, Erlangen, Germany) in our institution. Routine quality assurance testing is performed monthly on the PET/CT scanner. Acquired images were iteratively reconstructed with CT-based attenuation correction.
PET/CT images and processing
All PET/CT images were registered to the RT planning CT using rigid image registration to preserve target volume rigidity and SUV/image intensity values. The clinical planning target volume (PTV) was used to extract a volume of interest (VOI) for PET images. 18F FDG uptake was measured by the SUV, which represents the radioactivity of the tissue for a given time, mass and initial tracer injection. The maximum uptake value (SUVmax) was used to characterize the tumor metabolic activities for a given VOI. The mean uptake value (SUVmean) was calculated within the metabolic volume, which was defined as the volume with uptake larger than 40% of SUVmax (17,28).
Texture features provide complex measurements of spatial arrangement of voxels in the tumor volumes of interest. In the pre-processing, the PET images were resampled to yield a finite range of gray level (29,30). The images Fpre*Fpost for texture analysis were calculated from the pre-RT PET images Ipre and post-RT image Ipost, respectively
where G=24 and represents the gray scale of binning factor. and
, denote the minimum and maximum uptake values in pre-treatment PET image, whereas
and
were defined for post-RT PET images. Both definitions have the resampling term,
, which resamples voxel values within the VOI to yield a finite range of values allowing texture analysis. To account for the tumor response due to regression or progression, the pre- and post-treatment images are normalized by the normalization term,
, where
is the global maximum uptake value. For the case of tumor regression (
), the post-RT PET is normalized into the gray scale range of the pre-RT PET image with
. Similarly, for the case of tumor progression (
), the pre-RT images are normalized into gray scale range of the post-RT PET image. Without the normalization, the gray level of the post-RT PET will have the same resampled values as the pre-RT PET, and the texture variations between pre-RT and post-RT will be indistinguishable or lead to erratic calculations.
The PET textures were calculated using the gray level co-occurrence matrices (GLCM) (31), which measures the possibilities of different combinations of neighboring pixel values in the VOI. All textures were calculated for 3 dimensions in the VOI. The joint probabilities of occurrence of a pair of gray values were calculated along 13 directions, and three neighbor distances in 1, 2, and 4 voxels. In total, 39 (=3×13) co-occurrence matrices were calculated for a gray scale VOI. Twelve Haralick texture features (18,29,31) were derived from GLCM, including energy, entropy, correlation, contrast, homogeneity, variance, sum mean, inertia, cluster shade, cluster tendency, max probability, and inverse variance. To extract locoregional information, co-occurrence matrices were calculated using the kernel technique with a kernel size of 10×10×10 voxels. Figure 1 illustrates the locoregional textures of pre- and post-RT PET images for one patient.
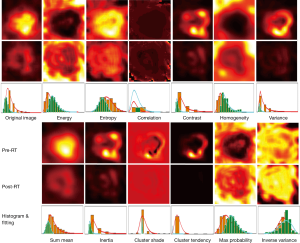
The voxel-wise texture features provide locoregional heterogeneous information based on the gray scale tumor uptakes. To quantify the results for response analysis, each feature was statistically characterized by the histograms. A texture χ was molded by the generalized extreme value (GEV) distribution f (32),
where is the location parameter,
is the scale parameter, and
is the shape parameter. To simplify the analysis, the location parameter, μ, was used to represent the properties of features. In addition, textural difference between pre- and post-RT image was quantified by the difference of the location parameters:
Finally, the global SUVmax and SUVmean obtained from the pre- and post-RT PET images were also included in the analysis. Therefore, 40 image-based features (12 pre-RT, 12 post-RT, 12 variation, and 4 global) were extracted from pre- and post-RT PET images. Together with eight clinical variables, a total of 48 features/variables were included in the study.
Statistics
OS was used as the primary clinical outcome metric for analysis. Statistical analysis was performed using the R software 3.1.1 (The R foundation for statistical computing, Vienna, Austria). Prognostic values for survival outcome were examined along with texture features and clinicopathological factors such as age, tumor size, pathologic tumor stage, lymph node stage, and clinical resectability. The independence of individual clinical and texture variables was examined by correlation. The statistical difference between subgroup patients was evaluated by the Wilcoxon rank-sum test (33).
The survival association of variables was first analyzed using univariate Cox proportional hazard regression analysis, and any variables identified as significant were examined by the Kaplan-Meier curves and log-rank tests. The cutoff values of variables were estimated by the time-dependent survival ROC curves, which were able to identify the threshold value by taking into account the number of months until censoring or recurrence of disease (34,35).
Due to high dimension of input variables, deriving effective survival models directly from the multivariate Cox analysis was difficult (36). In addition, the censored data in survival outcomes also limited the application of general linear model (37). In this work, the prognostic features were selected using the lasso/elastic net regression with Cox hazard model associated with survival outcome (23-27):
where β is the regression coefficient, λ is the regularization parameter, and χ is the input feature set. Output Yij =1 for uncensored data, and Yij =0 for censored data. The coefficient α controls the penalty weights for L1 norm of β of lasso regression, and L2 norm for ridge regression, and α=0.5 was used in this study. The resultant multivariate regression model was validated by 5-fold cross-validated deviance within one standard error of minimum model deviance. Finally, the prognostic values of texture features were further validated using multivariate Cox analysis associated with OS.
Results
The characteristics of the patients are summarized in Table 1. The median age at the time of diagnosis was 69 y (range, 46–86 y), and 61% of patients were male. Twenty-four patients had a T3 or T4 primary tumor, and 8 (31%) had lymph node metastases (Table 1). In this imaging study, no distant metastasis was observed in pre- and post-RT PET scans for all patients.
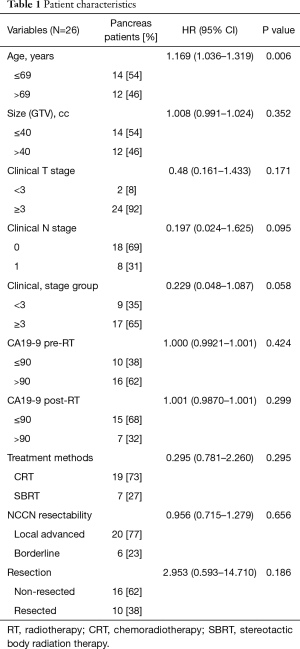
Full table
Results of clinical variable analysis
We first examined the significance of clinicopathological variables using univariate Cox analysis. As shown in Table 1, age was significantly associated with OS (P=0.006), and clinical node stage and overall stage were of borderline significance (P=0.095, and 0.058, respectively). Neither pre- nor post-RT CA19-9 values were significantly associated with OS (P=0.424, and 0.299, respectively, Table 1) when dichotomized at 90. We also evaluated the correlation between CA19-9s and the FDG-uptake values. No significant correlation was observed between CA19-9 and SUVmax (P=0.238 for pre-RT, P=0.684 for post-RT).
Results of global PET parameters
The results of univariate Cox analysis of global PET parameters are shown in Table 2. Three global image parameters, post-RT SUVmax, ∆SUVmax, and ∆SUVmean, were significant with P=0.001, 0.005, and 0.012, respectively.
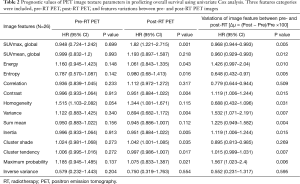
Full table
We evaluated the PET response and its potential impact on clinical outcomes based on the dichotomization of clinical features. The PET response differences based on clinical variable were compared by the Wilcoxon rank-sum tests. To simplify the response evaluation, we used post-RT SUVmax as a metric, since post-RT SUVmax is significantly associated with OS. For a given clinical variable, patients’ post-RT SUVmax was separated into two groups based on the classification of clinical variable. For the age groups (≤69 vs. >69), the PET response is not significantly different with P=0.219. Similarly, the results were P=0.426 for clinical stage group (≤3 vs. >3), and P=0.428 for clinical N stage (=0 vs. >0). In addition, the PET response (SUVmax) difference between borderline (n=20) and unresectable (n=6) was compared, and showed no significant difference between the two groups, P=0.563. Similarly, PET response was compared between SBRT and conventional CRT, and no significant difference was identified (P=0.821). To evaluate temporal effect of response, we divided patients into two groups based on the post-RT time interval (6.3±3.3 weeks) with group 1 (>6.3 week) and group 2 (≤6.3 weeks). The response difference between two groups in terms of post-RT PET SUVmax was evaluated with P=0.162. In summary, no significant PET response difference was observed based on clinical variables.
Results of texture analysis
Texture analysis for pre- and post-RT PET images was performed for all patients. Figure 1 illustrates the results of texture analysis for a 51-year-old patient. As shown in the first column, the VOIs of pre- and post-RT were extracted after rigid image registration, where post-RT image is color-coded in the range of pre-treatment PET image. The intensity image and the corresponding histogram illustrate that pre-RT PET exhibits much higher metabolic activities (SUVmax =10.7) than post-RT PET (SUVmax =4.5). Texture analysis further depicts tumor heterogeneity differences between pre- and post-RT PET data. Compared to the pre-RT image, three features (energy, homogeneity, and max probability) in the post-RT image had positive variation (visually brighter than those of pre-RT). The histograms of post-RT image show right-shifts relative to those of pre-RT. Consequently, the GEV fitting parameter (∆µ) had positive variation with 71.5%, 61.7%, and 51.6% for energy, homogeneity, max probability, respectively. In contrast, other features (entropy, contrast, variance, sum mean, inertia, cluster shade, cluster tendency) of post-RT images were visually darker than those in pre-RT, and had left-shift of histogram, and negative variation in terms of the GEV fitting parameters.
The results of univariate Cox analysis of image features are shown in Table 2. Most of the post-RT features (energy, entropy, contrast, variance, inertia, cluster shade, cluster tendency, and max probability) were significant (P<0.05). Additionally, most of the texture variations ∆µ (energy, entropy, contrast, homogeneity, variance, sum mean, inertia, cluster tendency, max probability) were also significantly associated with OS. The identified prognostic variables were used to stratify patients based on risk, as shown by Kaplan-Meier curves (Figure 2). The post-RT SUVmax stratified patients into two significantly different risk groups based on a cutoff value of SUVmax =4.1 (log-rank P=0.0002). Similarly, additional feature variations between pre- and post-RT also significantly stratified patient risks, as shown in Figure 2B,C,D.
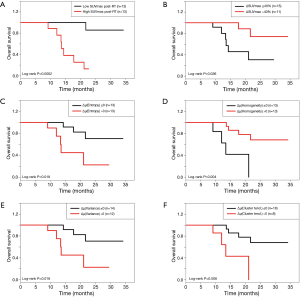
Identification of prognostic variables
The results of univariate analysis indicated that 20 variables/features were potentially significantly associated with survival outcome, which was impractical to derive into a robust multivariate model. To reduce the dimensions of input variable, we used the lasso/elastic net regression with an additive Cox hazard model to identify significant features. With a 5-fold cross-validation, the regression analysis identified seven predictors [age, clinical node stage, post-RT SUVmax, ∆µ(homogeneity), ∆µ(variance), ∆µ(sum mean), and ∆µ(cluster tendency); Table 3]. The coefficients of homogeneity variation have a negative sign compared with the other features, which matched the observed differences between the texture features in Figure 1.
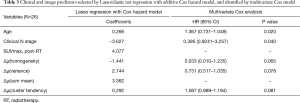
Full table
We performed a multivariate Cox analysis to further evaluate potentially significant prognostic variables for OS. The multivariate analysis was performed in a stepwise fashion using clinical variable and texture features. As a result, the multivariate Cox analysis identified five variables: two clinical variables, age and node stage, were significant with P=0.020, 0.040 respectively, and three texture variables, ∆µ(homogeneity), ∆µ(variance), and ∆µ(cluster tendency), were borderline significant with P=0.065, 0.078, 0.081, respectively.
Patient risk analysis
With the integration of prognostic clinical and imaging variables, the patients were stratified into two groups based on the risk score of multivariate analysis. The risk stratification is illustrated by Kaplan-Meier curves, as shown in Figure 3. The multivariate analysis achieved the significant risk stratification with log-rank P=0.001. Specifically, risk group 1 (n=11) had a longer mean OS of 29.3 months. As a result of favorable clinical and imaging characteristics, risk group 1 also had lower median pre-RT CA19 =1,047 and post-RT SUVmax =3.5, and higher median variation of global SUVmax (∆SUVmax =53.4%) and texture variations [∆µ(homogeneity) =51.9%, ∆µ(variance) =−72.8%, ∆µ(sum mean) =−29.3%, ∆µ(cluster tendency) =−86.7%]. In contrast, risk group 2 had a shorter mean OS (17.7 months), with higher median pre-RT CA 19-9 (=4,692) and post-RT SUVmax (=5.2), less variation of global SUVmax (∆SUVmax =11.2%), and fewer texture variations [∆µ(homogeneity) =2.1%,, ∆µ(variance) =7.7%, ∆µ(sum mean) =3.4%, ∆µ(cluster tendency) =13.4%].
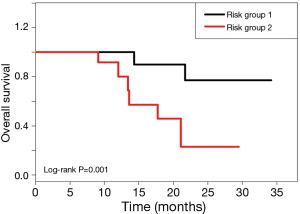
Discussion
Accurate assessment of tumor response to RT is critical in reevaluating the resectability of PA patients. Currently, response is assessed by measuring anatomic tumor characteristics using contrast enhanced CT-based NCCN criteria (2,9). 18F-FDG PET has shown promising results in assessing response to therapy for several cancer sites, including head and neck, esophageal and non-small cell lung cancers. Most of these studies have relied on the evaluation of typical global tumor uptakes values, such as SUVmax and SUVmean (17). However, such parameters fail to accurately characterize locoregional tumor uptake, which critically associate with underlying biophysiological tumor characteristics, such as perfusion, proliferation and tumor viability. With image feature analysis, it may be feasible to integrate tumor heterogeneity into therapy response evaluation, such that the radioresistance regions can be considered as potential targeting regions for improvement of treatment. A few studies (21,38-40) have focused on the link between image feature analysis and tumor biologic parameters. Gillies et al. (38) suggested that imaging can longitudinally characterize spatial variations in the tumor phenotype and its microenvironment so that the system dynamics over time can be quantitatively evaluated. In our study, we utilized pre- and post-RT PET/CT images to stratify patients with the ultimate aim of selecting personalized treatment strategies for patients. This approach relies on the locoregional texture analysis, clinical variables and statistical modeling to characterize the longitudinal variation between pre- and post-treatment PET images.
Texture feature-based analysis of clinical outcomes, also known as radiomics (41-44), is actively being investigated as a prognostic tool in radiation oncology (45,46). However, most approaches have only focused on pre-treatment image analysis as a means of identifying prognostic features (21,38). Our approach focused on the local texture variations in evaluation of therapy response using pre- and post-RT images. Imaging-based therapy response has to consider the spatial-temporal coincidence between pre-treatment and post-treatment data. Since the time span between pre- and post-RT images were generally larger than 2 months, the post-treatment image may have large variation due to changes in tissue anatomy and physiology. In our approach, we provide systematic solutions to several important technical issues, specifically in image alignment, and gray-scale normalization. First, the response evaluation has to distinguish the variations due to mismatching of image alignment or real tumor regression/progression. Rigid registration could lead to large registration errors, however deformable image registration is prone to distort the valuable tumor regression information. Our approach avoids direct voxel based one-to-one comparison which requires sophisticated image registration. Instead, we use statistical parameters of the distribution of texture features, which only require rigid registration. Secondly, the texture analysis strongly relies on the number of gray levels in the calculation of co-occurrence matrices. However, post-RT images generally have much lower metabolic uptakes compared to pre-RT images, and thus direct resampling of post-RT would lead to indistinguishable textures compared to the pre-treatment images. In our approach, all pre-treatment images were resampled in a pre-defined gray level scale and then the resampled post-RT images were further normalized to the maximum uptake of the pre-RT images. In this way, the resultant post-RT texture was normalized to the pre-RT texture, and the corresponding heterogeneity variations represent the differences between pre- and post-RT textures.
The proposed method integrates prognostic clinical and imaging features, and allows risk stratification of PA patients treated with RT. Four main component variables were used: (I) clinical data; (II) pre-treatment PET image with global SUVmax and SUVmean, and texture parameters; (III) post-treatment PET image parameters; (IV) variations between pre- and post-RT PET parameters. Finally, a total of 48 parameters were evaluated. Our multivariate analysis results indicate that both clinical variables and image features are both important prognostic factors for patient risk stratification.
Survival outcome used in this study is censored data, which limits the application of the logistic regression and generalized linear modes (23-26). To address this issue, we used the lasso/elastic net regression for the proportional hazard regression parameter as shown in Eq. [5]. The output response is associated with the time of event, and the OS is used as the end point. The lasso/elastic net regression with the Cox hazard model improves the accuracy of feature selection based on the censored survival information. In addition, the usage of lasso/elastic net method is due to the intrinsic characteristics (sparsity and correlation) of the input variable, which includes clinical variables and image feature sets. For sparse feature selection, lasso regression is preferred over ridge regression since L1 regularization promotes sparsity while L2 regularization does not. However, for high-dimensional data with few samples, the lasso tends to select less optimized variables. It also behaves erratically for highly-correlated data. Therefore, we used the additional L2 terms to stabilize the selection by introduce stronger convexity to the optimization.
A limitation of the present study is that it uses a retrospective analysis of a small patient cohort. Ten patients underwent surgery in this study, however the operation was not significantly associated with OS. Whereas surgical resection is clearly known to be a predictor of improved clinical outcome and more favorable disease biology, we hypothesize lack of clinical significance based on small patient numbers in our study. We recognized the prognostic significance of post-treatment CA 19-9 values, especially in the post-operative setting, yet no such significance was identified within our study perhaps again reflecting the smaller patient numbers. A prospective study involving a larger patient cohort would be needed to assess whether this model accurately predicts prognosis of PA patients.
Our results show that the texture variation of homogeneity, variance, and cluster tendency provided significant prognostic information allowing risk stratification of PA patients. As illustrated in Figure 1, the images of pre- and post-treatment of textures depict the heterogeneity variations due to treatment. The homogeneity of primary tumor was much improved after treatment, and the ring structures in pre-RT variance and cluster tendency were also smeared in the post-RT texture. The results suggest the treatment of the patient largely suppressed metabolic activity in the primary tumor and reduced underlying tissue heterogeneity. An extended application of these results would involve the identification of radioresistant areas of tumor using textural analysis of pre-treatment images. Our results suggest a feasible approach to integrate texture into identification of potential boosting target volume toward reducing the residue disease and adaptive RT.
Conclusions
We developed a novel texture analysis approach for characterizing texture variations between pre- and post-treatment PET images, and further proposed systematic feature selection methods to identify the significant prognostic texture features. Our results suggest that locoregional metabolic texture analysis is a feasible approach for evaluating and predicting response of pancreatic cancer treatment. Texture response can be a significant prognostic factor in predicting the treatment outcome of pancreatic patients. The proposed method can be used to stratify patient risk and aid in the selection of appropriate treatment strategies for individual patients. The results also may be used to predict persistent and local failure of disease, toward implementing response-driven adaptive RT.
Acknowledgements
None.
Footnote
Conflicts of Interest: The authors have no conflicts of interest to declare.
Ethical Statement: The study was approved by institutional ethics board of Cedars-Sinai Medical Center (No. Pro00031452).
References
- Siegel R, Ma J, Zou Z, et al. Cancer statistics, 2014. CA Cancer J Clin 2014;64:9-29. [Crossref] [PubMed]
- Visser BC, Ma Y, Zak Y, et al. Failure to comply with NCCN guidelines for the management of pancreatic cancer compromises outcomes. HPB (Oxford) 2012;14:539-47. [Crossref] [PubMed]
- Sohn TA, Yeo CJ, Cameron JL, et al. Resected adenocarcinoma of the pancreas-616 patients: results, outcomes, and prognostic indicators. J Gastrointest Surg 2000;4:567-79. [Crossref] [PubMed]
- Bockhorn M, Uzunoglu FG, Adham M, et al. Borderline resectable pancreatic cancer: a consensus statement by the International Study Group of Pancreatic Surgery (ISGPS). Surgery 2015;155:977-88. [Crossref] [PubMed]
- Habermehl D, Kessel K, Welzel T, et al. Neoadjuvant chemoradiation with Gemcitabine for locally advanced pancreatic cancer. Radiat Oncol 2012;7:28. [Crossref] [PubMed]
- Loehrer PJ Sr, Feng Y, Cardenes H, et al. Gemcitabine alone versus gemcitabine plus radiotherapy in patients with locally advanced pancreatic cancer: an Eastern Cooperative Oncology Group trial. J Clin Oncol 2011;29:4105-12. [Crossref] [PubMed]
- Bickenbach KA, Gonen M, Tang LH, et al. Downstaging in pancreatic cancer: a matched analysis of patients resected following systemic treatment of initially locally unresectable disease. Ann Surg Oncol 2012;19:1663-9. [Crossref] [PubMed]
- Mian OY, Ram AN, Tuli R, et al. Management options in locally advanced pancreatic cancer. Curr Oncol Rep 2014;16:388. [Crossref] [PubMed]
- Katz MH, Fleming JB, Bhosale P, et al. Response of borderline resectable pancreatic cancer to neoadjuvant therapy is not reflected by radiographic indicators. Cancer 2012;118:5749-56. [Crossref] [PubMed]
- Katz MH, Ahmad S, Nelson H. Borderline resectable pancreatic cancer: pushing the technical limits of surgery. Bull Am Coll Surg 2013;98:61-3. [PubMed]
- Farma JM, Santillan AA, Melis M, et al. PET/CT fusion scan enhances CT staging in patients with pancreatic neoplasms. Ann Surg Oncol 2008;15:2465-71. [Crossref] [PubMed]
- Wang Z, Chen JQ, Liu JL, et al. FDG-PET in diagnosis, staging and prognosis of pancreatic carcinoma: a meta-analysis. World J Gastroenterol 2013;19:4808-17. [Crossref] [PubMed]
- Rijkers AP, Valkema R, Duivenvoorden HJ, et al. Usefulness of F-18-fluorodeoxyglucose positron emission tomography to confirm suspected pancreatic cancer: a meta-analysis. Eur J Surg Oncol 2014;40:794-804. [Crossref] [PubMed]
- Bang S, Chung HW, Park SW, et al. The clinical usefulness of 18-fluorodeoxyglucose positron emission tomography in the differential diagnosis, staging, and response evaluation after concurrent chemoradiotherapy for pancreatic cancer. J Clin Gastroenterol 2006;40:923-9. [Crossref] [PubMed]
- Heinrich S, Schäfer M, Weber A, et al. Neoadjuvant chemotherapy generates a significant tumor response in resectable pancreatic cancer without increasing morbidity: results of a prospective phase II trial. Ann Surg 2008;248:1014-22. [Crossref] [PubMed]
- Kittaka H, Takahashi H, Ohigashi H, et al. Role of (18)F-fluorodeoxyglucose positron emission tomography/computed tomography in predicting the pathologic response to preoperative chemoradiation therapy in patients with resectable T3 pancreatic cancer. World J Surg 2013;37:169-78. [Crossref] [PubMed]
- Zhu A, Lee D, Shim H. Metabolic positron emission tomography imaging in cancer detection and therapy response. Semin Oncol 2011;38:55-69. [Crossref] [PubMed]
- El Naqa I, Grigsby P, Apte A, et al. Exploring feature-based approaches in PET images for predicting cancer treatment outcomes. Pattern Recognit 2009;42:1162-71. [Crossref] [PubMed]
- Xu R, Kido S, Suga K, et al. Texture analysis on (18)F-FDG PET/CT images to differentiate malignant and benign bone and soft-tissue lesions. Ann Nucl Med 2014;28:926-35. [Crossref] [PubMed]
- Tan S, Kligerman S, Chen W, et al. Spatial-temporal [18F]FDG-PET features for predicting pathologic response of esophageal cancer to neoadjuvant chemoradiation therapy. Int J Radiat Oncol Biol Phys 2013;85:1375-82. [Crossref] [PubMed]
- Chicklore S, Goh V, Siddique M, et al. Quantifying tumour heterogeneity in 18F-FDG PET/CT imaging by texture analysis. Eur J Nucl Med Mol Imaging 2013;40:133-40. [Crossref] [PubMed]
- Tuli R, Fraass B, Yang W, et al. Pretreatment 18 F-FDG-PET texture analysis to predict local response of pancreatic cancer to radiotherapy. J Clin Oncol 2014;32:375.
- Tibshirani R. The lasso method for variable selection in the Cox model. Stat Med 1997;16:385-95. [Crossref] [PubMed]
- Zou H, Hastie T. Regularization and variable selection via the elastic net. Journal of the Royal Statistical Society: Series B (Statistical Methodology) 2005;67:301-20. [Crossref]
- Zou H, Hastie T, Tibshirani R. On the “degrees of freedom” of the lasso. The Annals of Statistics 2007;35:2173-92. [Crossref]
- Zhang HH, Lu W. Adaptive Lasso for Cox's proportional hazards model. Biometrika 2007;94:691-703. [Crossref]
- Yue Y, Yang W, Fraass B, et al. Prognostic Modeling of Locally Advanced Pancreatic Cancer Treated With Radiation Therapy Using [18F] FDG-PET Features and CA-19-9. International Journal of Radiation Oncology Biology Physics 2014;90:S361. [Crossref]
- Biehl KJ, Kong FM, Dehdashti F, et al. 18F-FDG PET definition of gross tumor volume for radiotherapy of non-small cell lung cancer: is a single standardized uptake value threshold approach appropriate? J Nucl Med 2006;47:1808-12. [PubMed]
- Haralick RM, Shapiro LG. Computer and Robot Vision, Vol. 1. Boston: Addison-Wesley, 1992:459.
- Tixier F, Le Rest CC, Hatt M, et al. Intratumor heterogeneity characterized by textural features on baseline 18F-FDG PET images predicts response to concomitant radiochemotherapy in esophageal cancer. J Nucl Med 2011;52:369-78. [Crossref] [PubMed]
- Haralick RM, Shanmugam K. Textural features for image classification. IEEE Transactions on Systems, Man, and Cybernetics 1973;3:610-21. [Crossref]
- Beirlant J, Goegebeur Y, Segers J, et al. Statistics of extremes: theory and applications. John Wiley & Sons, 2006.
- Corder GW, Foreman DI. Nonparametric Statistics for Non-Statisticians: A Step-by-Step Approach. Colorado: Wiley, 2009.
- Adams H, Tzankov A, Lugli A, et al. New time-dependent approach to analyse the prognostic significance of immunohistochemical biomarkers in colon cancer and diffuse large B-cell lymphoma. J Clin Pathol 2009;62:986-97. [Crossref] [PubMed]
- Blanche P, Dartigues JF, Jacqmin-Gadda H. Estimating and comparing time-dependent areas under receiver operating characteristic curves for censored event times with competing risks. Stat Med 2013;32:5381-97. [Crossref] [PubMed]
- Fan J, Feng Y, Wu Y. High-dimensional variable selection for Cox’s proportional hazards model. Borrowing Strength: Theory Powering Applications–A Festschrift for Lawrence D. Brown. Institute of Mathematical Statistics 2010:70-86.
- Mardia KV, Kent JT, Bibby JM. Multivariate Analysis. New York: Academic Press, 1979.
- Gillies RJ, Anderson AR, Gatenby RA, et al. The biology underlying molecular imaging in oncology: from genome to anatome and back again. Clin Radiol 2010;65:517-21. [Crossref] [PubMed]
- Morgan B. Opportunities and pitfalls of cancer imaging in clinical trials. Nat Rev Clin Oncol 2011;8:517-27. [Crossref] [PubMed]
- Strauss LG, Pan L, Koczan D, et al. Fusion of positron emission tomography (PET) and gene array data: a new approach for the correlative analysis of molecular biological and clinical data. IEEE Trans Med Imaging 2007;26:804-12. [Crossref] [PubMed]
- Miles KA, Ganeshan B, Griffiths MR, et al. Colorectal cancer: texture analysis of portal phase hepatic CT images as a potential marker of survival. Radiology 2009;250:444-52. [Crossref] [PubMed]
- Goh V, Ganeshan B, Nathan P, et al. Assessment of response to tyrosine kinase inhibitors in metastatic renal cell cancer: CT texture as a predictive biomarker. Radiology 2011;261:165-71. [Crossref] [PubMed]
- Buckler AJ, Bresolin L, Dunnick NR, et al. Quantitative imaging test approval and biomarker qualification: interrelated but distinct activities. Radiology 2011;259:875-84. [Crossref] [PubMed]
- Lambin P, Rios-Velazquez E, Leijenaar R, et al. Radiomics: extracting more information from medical images using advanced feature analysis. Eur J Cancer 2012;48:441-6. [Crossref] [PubMed]
- Cunliffe A, Armato SG 3rd, Castillo R, et al. Lung texture in serial thoracic computed tomography scans: correlation of radiomics-based features with radiation therapy dose and radiation pneumonitis development. Int J Radiat Oncol Biol Phys 2015;91:1048-56. [Crossref] [PubMed]
- Fried DV, Tucker SL, Zhou S, et al. Prognostic value and reproducibility of pretreatment CT texture features in stage III non-small cell lung cancer. Int J Radiat Oncol Biol Phys 2014;90:834-42. [Crossref] [PubMed]