Prediction of colorectal cancer risk among adults in a lower middle-income country
Introduction
Globally, colorectal cancer (CRC) is an important public health problem (1). In 2015, it was the fourth ranking cancer worldwide, the second most common cancer among men and the third among women in the South-East Asia region (2). Cancer incidence data of Sri Lanka in 2010 ranked CRC as the fourth common cancer among men and sixth common among women (3).
Reduction of morbidity and mortality associated with early detection of CRC (4) is the basis for adoption of a population level programme to screen all adults in developed countries (5). Tests available to screen for CRC vary from a simple test such as faecal occult blood test to more technical and invasive methods such as flexible sigmoidoscopy and colonoscopy. Of the methods, faecal occult blood test, is not very useful due to its low sensitivities and specificities. The only fecal occult blood testing known to have higher sensitivity and specificity, based on using immunochemical methods, its high cost has precluded it being used as the test in screening programme at population level (4). Flexible sigmoidoscopy and colonoscopy are the other screening methods which allow direct observation of the large bowel and need to be performed by a skilled person in an endoscopy unit. In developed countries such as Germany and United States of America, adults above 50 years of age are offered colonoscopy or flexible sigmoidoscopy as a screening test for CRC followed by repeated screening in every ten years (6).
Unavailability of skilled health personnel, high cost and other logistic issues have prevented developing countries from considering colonoscopy or flexible sigmoidoscopy at population level screening programmes for CRC. However, the importance of the diseases and existing evidence indicate that developing countries would benefit by offering colonoscopy or flexible sigmoidoscopy screening at least to the groups at high risk for CRC (7). One mechanism of identifying high-risk population groups to undergo CRC screening is by risk stratification, using risk prediction models. This is a low-cost and less invasive method (8). Though many risk prediction models for CRC are being used in some parts of the world, the fact that risk factors included in prediction models and their strengths are specific for the setting in which they were developed limit their common use in other countries.
Sri Lanka, like many other countries in South East Asian Region (SEAR), does not offer population-based screening programmes for CRC. In 2010, Sri Lanka reported a total of 1,083 (ASR—5.6/100,000) CRC cases (3), while there were 120,225 (ASR—7.5/100,000) cases identified in SEAR in 2012 (2). This high burden of CRC in the region as well as in the country indicates the need of adopting a screening programme at least for high-risk groups.
None of the available validated risk models to predict CRC were originated from the SEAR or any developing country (9). There have not been any previous attempts to develop and validate a country specific risk prediction models to identify high-risk population for CRC in Sri Lanka. Furthermore, the risk predictors in the model produce the platform for primary prevention where this model can be utilized as a counselling tool in managing high risk individuals. In this background, the present study aimed at developing and validating a risk prediction model to identify the high-risk groups for CRC.
Methods
Development and validation of the risk prediction model was performed in a step-wise manner as shown in Figure 1.
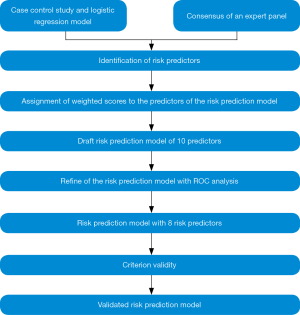
Identification of the risk factors for CRCs
We identified the risk factors to be included in the CRC risk prediction model by two steps as described below.
Step 1: case control study
As the first step, an unmatched case control study was conducted in two districts that report highest incidence of CRC in Sri Lanka. This study included 65 new clinically confirmed CRC cases and 130 colonoscopy negative controls from major five tertiary care hospitals, recruited using consecutive sampling method. Information on lifestyle related, environmental, socio-demographic, genetic and co-morbid risk factors for CRC were obtained using an interviewer-administered questionnaire with verification through medical records when appropriate (10). Logistic regression analyses by backward likelihood ratio method were performed to develop a model to predict the risk of an adult developing CRC. The six risk factors (age more than 50 years, frequent consumption of red meat and deep-fried food, history of CRC and other cancers as uterine, ovary and breast among first degree relatives and presence of hypertension for more than 10 years) that were found to be significant in the multivariate analyses were included in the prediction model.
Step 2: consensus of an expert panel
Secondly, a few additional risk factors consistently identified in the literature for CRC were included in the risk prediction model with consensus of a panel of experts. This panel of experts involved 11 experts in the fields of community medicine (n=5), general surgery (n=2), oncology (n=2) and statistics (n=2). They were invited for a discussion meeting to share their expertise in assessing the need to include additional predictors to the model to estimate the risk of a Sri Lankan adult developing CRC. To facilitate this assessment, the experts were provided with the information on the factors and the coefficients used in the prediction models to estimate CRC developed by other researchers in other countries (9) together with the factors that were found to be significant in the bivariate analyses of the selected risk prediction model. The risk factors that were agreed upon by 75% of the panel were included as additional predictors to the risk model being developed (frequent consumption of processed meat for the period of last 20 years and beyond, history of histologically confirmed inflammatory bowel disease diagnosed before 10 years or more, presence of medically confirmed diabetes for more than 10 years and history of histologically confirmed intestinal polyps diagnosed before 10 years).
Assignment of ‘weighted scores’ to the predictors of the risk prediction model
The risk prediction model being developed was designed as a formula where each of the factors included would be assigned a value which would depict its relative contribution to predict CRC when applied to individuals. For the six predictors selected into the model based on the logistic regression model, the adjusted odds ratios (OR), rounded up to have no decimals were considered as these values. The values assigned for the four predictors selected other than from the logistic regression model were based on the unadjusted OR from the bivariate analysis in the present study or pooled ORs from meta-analysis from other published literature in the absence of the significant results due to small sample of the positives. These values were considered as ‘weighted scores’ in the risk prediction model and the weighted scores were to be summated into a single summary score to predict the overall risk of an individual to develop CRC.
Table 1 shows the draft risk prediction model including the six predictors identified from logistic regression and the four predictors identified from the expert opinion with their assigned weighted scores.
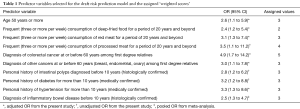
Full table
Refining of the risk prediction model
Receiver operator characteristic (ROC) curve analysis and calculating the area under the curve (AUC) was used to assess the discriminative performance of the model using the validation sample described below. According to the score for each risk predictor in the risk prediction model, a summary risk score was derived for each case and control of the validation sample, by adding all the individual weighted scores of the selected 10 predictors. The predictors were incorporated to the model one by one. ROC curve analysis and AUC analyses were performed for each of the models that were generated. Predictor combination containing the highest AUC was selected as the final model to be used. The final model contained only eight of the ten predictors.
Criterion validity
The criterion validity of the refined risk prediction model was assessed in a validation study using a separate case-control design from November 2014 to November 2015. Histological confirmation of the lesion via colonoscopy and biopsy was used as the criterion (11). The study population comprised of CRC cases, defined as histologically confirmed incidence cases (diagnosed within six months prior to the study), aged 30 years and above who had no previous diagnosis of any other form of cancer and were resident in the two districts where the first case-control study was conducted. A control was defined as a person with a negative colonoscopy report excluding CRC, aged 30 years and above who had no previous diagnosis of any other form of cancer and was resident in the same districts. The cases were recruited from the National Cancer Institute, Maharagama, a specialized unit for cancer care, while the controls were selected from those who underwent colonoscopy at four major tertiary care institutions. At all hospitals, study unit were recruited using a consecutive sampling method.
Estimation of the size of the sample required was based on the expected sensitivity or the specificity of the risk prediction model. The expected minimum sensitivity was 90% and minimum specificity was 95%, with a precision of 15%, at a confidence interval of 95%. The required minimum sample size was 65 per group, allowing for the non-response of 5% (12).
Summary score with the selected eight variables were calculated for cases and controls for the assessment of criterion validity. Optimal cut off value to categorize each participant into ‘at risk’ of developing CRC or ‘not at risk’ of developing CRC was obtained by the maximum length from AUC to the diagonal line. Based on the cut off value, the indicators of the validity of the risk prediction model, sensitivity, specificity, positive predictive value (PPV), negative predictive value (NPV) and likelihood ratios were estimated.
Information on the predictors was obtained using a pre-tested structured questionnaire administered by four trained medical officers. When assessing the genetic factors, the details were verified by inquiring another question related to the referral to an oncologist or to any one of the cancer treatment centers in any part of the country while the comorbid factors were verified with medical records. The age at diagnosis was also inquired followed by verification with medical records. Informed and written consent was obtained from all study participants included in the study and the study was approved by the Ethics Review Committee of Faculty of Medicine, University of Colombo, Sri Lanka prior to the commencement of the study.
Provision to indicate the scores assigned to each predictor and provision to indicate the total score were provided in the instrument itself to facilitate estimation of the total score at the end of administering the tool. When assigning the scores, the risk level of each predictor was assigned the agreed upon value and the reference level was assigned a score of zero. Reliability was assessed by re-administrating the risk prediction model, employing test-re-test method among cases and the controls.
Results
In the criterion validity study, all selected cases and controls participated giving a response rate of 100.0%. A majority of the cases (n=57, 87.7%) and controls (n=35, 53.8%) were aged 50 years or more while most of the cases (n=36, 55.4%) were males with most of the controls (n=33, 50.8%) being females.
ROC curve analyses performed by incorporating each predictor one at a time to the model showed that the best model which had the highest AUC of 0.849 (95% CI: 0.8–0.9) consisted of eight predictors. Table 2 shows the best performing risk prediction model with the eight predictors and their scores while the ROC analysis is demonstrated in Figure 2. The AUC was statistically significant (P<0.001) and demonstrated good performance where 84.9% of the variability of the CRC is explained by the summary risk score.
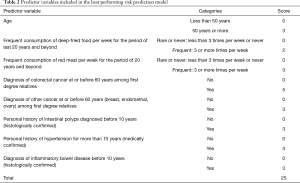
Full table
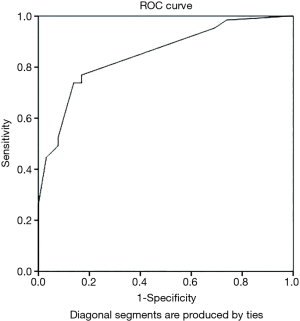
Cut off values of summary risk score ranged from −1 to 20. The shortest distance (d2) in the ROC curve was 0.082. It corresponded with the summary risk score of 5.5 indicating that 5.5 to be the optimal cut off value to categorize each participant into ‘at risk’ of developing CRC or ‘not at risk’ of developing CRC. Having a sensitivity of 76.9% (95% CI: 66.7–87.1%) a specificity of 83.1% (95% CI: 74.0–92.2%), a PPV of 82.0% (95% CI: 72.3–91.6%) and a NPV of 79.3% (95% CI: 68.5–88.0%) were indicative of good prediction ability of the model. Furthermore, the likelihood ratios (LR) were also calculated (LR+ =4.6 and LR− =0.3). The results of the test re-test reliability showed good test re-test reliability with the correlation coefficient of 0.88, at 0.05 significance level.
Discussion
Our approach of the present study was to build a simple and user-friendly risk prediction model for CRC among adults, that can be applied in a community setting or at a clinic setting by a trained person who may not be health staff. Many measures were taken to ensure that the developed risk prediction model was user friendly and easy to be used in the community settings as a screening tool to identify high risk individuals. The tool to collect information on predictors was designed as a simple eight question interviewer administered tool with data to be obtained from the history. Thus, the tool fulfilled an important feature of being suitable to be administered by a trained data collector in a community setting.
Although several other risk prediction models exist for CRC, these available models are either applicable to specialized populations (13-15), predictions are based purely on literature and expert opinion (16) or are designed to predict different outcomes such as having CRC or an advanced polyp (8). The prediction model developed by the current study was mostly based on adjusted risk factors that were found to be associated with developing CRC derived from a case control study among adults over 30 years in the local setting. Thus, the model was more valid for the local setting but can be used only for adults over 30 years of age. Similar to the processes followed by many other researchers in developing risk prediction models (17,18), the present study also incorporated the predictors of clinically important risk factors by obtaining expert opinion in addition to the risk factors identified through statistical methods. In addition, incorporation of additional predictors was performed through an objective process. Most of the risk predictors included in the prediction model such as older age, consumption of red meat, family history of CRC, inflammatory bowel disease, history of polyps, are mostly common with the risk predictors incorporated in other models in other settings (8,9), which is the result of incorporating predictors via two steps to develop a comprehensive model. Inclusion of predictors such as presence of long-term hypertension is unique to the current model, in comparison of other models though this was highlighted as a risk factor in many epidemiological studies (19,20). All the predictors can be verified from the history following a short interview with the participant, which is an advantage in the utilization of the model in the field setting as well as in busy institutional clinics at the local setting.
In addition to the model being easy to administer, imposing minimal discomfort on the participants, it must also be valid and reliable to be applied to predict development of CRC among the members in the community. Validation studies to evaluate criterion validity are advocated to assess the psychometric properties as well as the discriminatory power of the risk prediction models (21). An advantage in assessing criterion validity was the ability to determine the best cut off for the summary risk score to accurately distinguish adults at risk from adults not at risk for CRC, against a gold standard. The gold standard used in this study was colonoscopy examination which has a sensitivity of 95% in detecting CRC (4). Verification bias did not occur in the present study because all study subjects underwent the same gold standard which is the colonoscopy examination. Since colonoscopy is not routinely available as a screening programme, due to its invasiveness of the procedure and financial cost, the individuals with gastro-intestinal symptoms undergoing colonoscopy and individuals undergoing colonoscopy for insurance purposes were selected as the control population. This can be further justified as the present study avoided the ethically inappropriate conduction of colonoscopy among healthy individuals. One can argue that the study could have used volunteers with financial cost recovery. However, this would have introduced volunteer bias of relatively healthier subjects volunteering to recruit in the study than the others, making the controls not representative of the source population.
The validity indicators of the present model indicate that the model was with high specificity at the expense of sensitivity. It is well accepted that specificity of a model should be increased in the expense of sensitivity when the costs or the risks associated with further diagnostic procedures is an important criterion to consider (22). When applied to the present study, screening to detect ‘at risk’ for developing CRC among adults will be required to be followed up with an expensive and somewhat invasive colonoscopy for definitive diagnosis. Thus, a model with high specificity and low sensitivity is justifiable. An AUC of 0.849 indicated that the model has good discriminative power to differentiate between at risk individual from not at-risk of developing CRC. Among other risk prediction models developed for CRC, none of the researches have assessed the criterion validity with respect to the accuracy of the models. The present risk prediction model has an improved discriminative power (0.849) than the other models validated (8,14,23). The present risk prediction model also has a high positive predictive value owing to its high specificity even though the preclinical disease of CRC in the population is not common.
The reliability of the risk prediction model was high (Kappa coefficient 0.88), indicating its ability to produce consistent results with repeated use. The calibration power was assessed by other models by calculating the agreements between the observed and predicted risks of developing CRC which were also found to indicate good agreement (13,14,16). In the absence of population attributable risks and age specific CRC hazard rates at national level, the developed risk prediction model does not allow estimation of the absolute risk of developing CRC in a defined period which can be considered as a limitation. In addition, the validation study was conducted including a hospital control group who has undergone colonoscopy examination, which is a limitation of the study.
Conclusions
The risk prediction model developed to predict the risk of CRC consisting eight predictors was proven to be valid and reliable and is recommended to be used to detect those ‘at risk’ of CRC, among adults over 30 years of age in Sri Lanka. It possessed many features of a model that can be easily administered in a community or a clinical setting by a trained person. The scoring system to identify those ‘at risk’ also is indicated in the tool for easy use.
Acknowledgements
Authors wish to thank the health administrators of the districts where the study was conducted, clinicians of the institutions and the study participants for their support and participation.
Funding: This study received a grant of LKR 500,000.00 from the Medical Research Institute of Sri Lanka, which is a governmental organization, under the grant number 2015/025.
Footnote
Conflicts of Interest: The authors have no conflicts of interest to declare.
Ethical Statement: Informed and written consent was obtained from all study participants included in the study and the study was approved by the Ethics Review Committee of Faculty of Medicine, University of Colombo, Sri Lanka prior to the commencement of the study.
References
- Boyle P, Langman JS. ABC of colorectal cancer: epidemiology. BMJ 2000;321:805-8. [Crossref] [PubMed]
- World Health Organization. GLOBOCAN 2012, Colorectal Cancer: Estimated Incidence, Mortality and Prevalence Worldwide in 2012. Available online: http://globocan.iarc.fr/Pages/fact_sheets_cancer.aspx#, accessed 19 January 2016.
- National Cancer Control program SL (2010). PDF_PUBLICATIONS/Cancer_Incidence_Data_2010.pdf. Available online: http://www.nccp.health.gov.lk/images/, accessed 29 April 2017.
- Bretthauer M. Colorectal cancer screening. J Intern Med 2011;270:87-98. [Crossref] [PubMed]
- Alteri R, Brooks D, Gansler T, et al. Colorectal Cancer Facts and Figures 2014-2016. Atlanta, Georgia: American Cancer Society, 2014:1-30.
- Levin B, Lieberman DA, McFarland B, et al. Screening and surveillance for the early detection of colorectal cancer and adenomatous polyps: a joint guideline from the American Cancer Society, the US Multi‐Society Task Force on Colorectal Cancer, and the American College of Radiology. CA Cancer J Clin 2008;58:130-60. [Crossref] [PubMed]
- Boyle P, Leon ME. Epidemiology of colorectal cancer. British Medical Bulletin 2002;64:1-25. [Crossref] [PubMed]
- Win AK, Macinnis RJ, Hopper JL, et al. Risk Prediction Models for Colorectal Cancer: A Review. Cancer Epidemiol. Biomarkers Prev 2012;21:398-410. [Crossref] [PubMed]
- Usher-Smith JA, Walter FM, Emery JD, et al. Risk Prediction Models for Colorectal Cancer: A Systematic Review. Cancer Prev Res (Phila) 2016;9:13-26. [Crossref] [PubMed]
- Samarakoon YM. Risk factors and risk prediction of colorectal cancer among adults in the districts of Colombo and Gampaha [MD thesis]. Colombo: University of Colombo, 2016:240.
- Rockey DC, Paulson E, Niedzwiecki D, et al. Analysis of air contrast barium enema, computed tomographic colonography, and colonoscopy: prospective comparison. Lancet 2005;365:305-11. [Crossref] [PubMed]
- Hulley SB, Cummungs SR, Browner WS, et al. Designing Clinical Research, 3rd Edition. Lippincott Williams & Wilkins, Philadelphia, PA, 2007.
- Freedman AN, Slattery ML, Ballard-Barbash R, et al. Colorectal cancer risk prediction tool for white men and women without known susceptibility. J Clin Oncol 2009;27:686-93. [Crossref] [PubMed]
- Driver JA, Gaziano JM, Gelber RP, et al. Development of a Risk Score for Colorectal Cancer in Men. Am J Med 2007;120:257-63. [Crossref] [PubMed]
- Selvachandran SN, Hodder RJ, Ballal MS, et al. Prediction of colorectal cancer by a patient consultation questionnaire and scoring system: a prospective study. Lancet 2002;360:278-83. [Crossref] [PubMed]
- Colditz GA, Atwood KA, Emmons K, et al. Harvard report on cancer prevention volume 4: Harvard Cancer Risk Index. Risk Index Working Group, Harvard Center for Cancer Prevention. Cancer Causes Control 2000;11:477-88. [Crossref] [PubMed]
- Kumari PBVR. Risk factors and risk assessment of Breast Cancer among women in the district of Colombo [MD thesis]. Colombo: University of Colombo, 2013:286.
- Tammemagi CM, Pinsky PF, Caporaso NE, et al. Lung Cancer Risk Prediction: Prostate, Lung, Colorectal and Ovarian Cancer Screening Trial Models and Validation. J Natl Cancer Inst 2011;103:1058-68. [Crossref] [PubMed]
- Stürmer T, Buring JE, Lee I, et al. Metabolic Abnormalities and Risk for Colorectal Cancer in the Physicians’ Health Study. Cancer Epidemiol Biomarkers Prev 2006;15:2391-7. [Crossref] [PubMed]
- Ahmed RL, Schmitz KH, Kristin E, et al. The Metabolic Syndrome and Risk of Incident Colorectal Cancer. Cancer 2006;107:28-36. [Crossref] [PubMed]
- Freedman AN, Seminara D, Gail MH, et al. Cancer risk prediction models: a workshop on development, evaluation and application. J Natl Cancer Inst 2005;97:715-23. [Crossref] [PubMed]
- Hennekens CH, Buring JE. Epidemiology in Medicine. Boston, Unites States of America, 1987.
- Park Y, Freedman AN, Gail MH, et al. Validation of a colorectal cancer risk prediction model among white patients age 50 years and older. J Clin Oncol 2009;27:694-8. [Crossref] [PubMed]