Prognostic value and association of sarcopenic obesity and systemic inflammatory indexes in patients with hepatocellular carcinoma following hepatectomy and the establishment of novel predictive nomograms
Introduction
Hepatocellular carcinoma (HCC) is a common tumor that leads to poor survival outcomes even though the surgical techniques and adjuvant therapy has great advancements. In the era of individualized treatment with precision medicine, how to accurately identify various risk factors in early-stage HCC patients and establish corresponding individualized treatment have become clinical challenges. Recently, both sarcopenia and systemic inflammation have been proven to be closely associated with poor survival outcomes in HCC patients (1). Sarcopenia is a progressive syndrome that is characterized by skeletal muscle dysfunction involving the accelerated loss of muscle mass and function, which is associated with an increased risk of adverse outcomes (2). Sarcopenic obesity (SO) is defined as the coexistence of sarcopenia and obesity (3). Sarcopenia is associated with a poor HCC prognosis (4-14). However, studies on the specific impacts of SO on HCC are scarce, and there are still disagreements about the influence of SO on the survival prognosis in HCC patients (1,15-22). SO is a complex nutritional condition and a new syndrome, and its mechanisms are unknown to the research field of HCC (23). With the decrease in muscle mass and simultaneous accumulation of fat, SO is considered to have more complex mechanisms than sarcopenia alone. A series of evidence has demonstrated that the mechanism of sarcopenia may be related to systemic inflammation, in which resulting in imbalance of protein synthesis and catabolism by activating many of the molecular pathways involved in skeletal muscle loss (24,25). Lin et al. proved an association between the inflammatory index and sarcopenia, and both are considered good prognostic indicators in gastric cancer patients (26). But it is not clear whether SO has similar mechanisms and associations with inflammation in HCC patients. Different inflammatory indexes reflecting systemic inflammation and related inflammatory levels have been reported to play a key role in the development and progression of cancer (27). Different inflammatory indexes based on blood neutrophil, lymphocyte, monocyte, and platelet ratios, such as the neutrophil-lymphocyte ratio (NLR), lymphocyte-monocyte ratio (LMR), and platelet-lymphocyte ratio (PLR), the prognostic nutritional index (PNI) and the systemic immune-inflammation index (SII) were reported to be closely related to the progression and prognosis of HCC (28). What is the intricate relationship among SO, these inflammatory indexes and HCC?
The specific impacts of SO on HCC and the association between SO and systemic inflammation remain unclear, especially in the HCC population with an extremely high infection rate of hepatitis B virus in China. Thus, this study attempted to investigate the relationships among SO, systemic inflammation and HCC. Furthermore, we aimed to evaluate the prognostic abilities of preoperative SO and systemic inflammatory indexes and their combined prognostic ability in predicting outcomes after hepatectomy for HCC to establish a novel prediction system involving nomograms for survival prediction. We present the study in accordance with the REMARK reporting checklist (available at http://dx.doi.org/10.21037/jgo-20-341).
Methods
The study was conducted in accordance with the Declaration of Helsinki (as revised in 2013). The protocol was approved by the institutional review boards of Fujian Provincial Hospital and Fujian Medical University Union Hospital (No. K2019-12-025; 2019KY022), and Informed consent was obtained from all patients before inclusion in the study.
Study population
This was a retrospective study performed in the Fujian Provincial Hospital and Fujian Medical University Union Hospital affiliated with Fujian Medical University from January 2012 to March 2015. We retrospectively reviewed data collected from patients who underwent radical hepatectomy at Fujian Provincial Hospital from January 2012 to March 2015; these patients were considered the training cohort. Patients who underwent radical hepatectomy at Fujian Medical University Union Hospital during the same period were enrolled in the study as the external validation cohort. The inclusion criteria were as follows: (I) patients with histologically confirmed HCC; (II) patients who had not received any other curative treatment before radical hepatectomy (R0); and (III) patients with available preoperative computed tomography (CT) images within 30 days. The exclusion criteria were as follows: (I) other malignant tumors or metastatic disease; (II) unavailable preoperative CT images or incomplete or inaccurate medical records; or (III) death within 30 days after surgery. In total, 452 patients were enrolled in the training cohort, and 275 patients were enrolled in the external validation cohort in this study.
Image analysis and definition of SO
In this study, CT-based measurements were performed with ImageJ (v1.52q, RRID:SCR_003070) by a single trained researcher who was blinded to the outcomes. We measured the cross-sectional skeletal muscle area at the level of the third lumbar vertebra (L3) based on attenuation thresholds of –29 to +150 Hounsfield units (Hu) (19). Muscle area (cm2), which was calculated as the average surface of two consecutive transverse slices, was normalized for the height of the patient (m2) to obtain the L3 skeletal muscle index (SMI; cm2/m2) (29). SMI cutoff values were defined according to the area under the curve (AUC), receiver operating characteristic (ROC) curve and Youden index. SMI cutoff values were 40.86 cm2/m2 for males and 30.71 cm2/m2 for females (Figure 1).
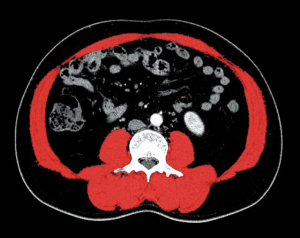
Obesity was defined as a body mass index (BMI) ≥25 kg/m2 (30). Patients were classified into 1 of 2 body composition categories according to the presence of both sarcopenia and obesity: the nonsarcopenic obesity (NSO) group and the SO group.
Markers of systemic inflammation
After a literature review, the following inflammatory indexes calculated from laboratory parameters obtained within 1 week before surgery were included in the analysis: the NLR, PLR, LMR, PNI and SII (31). The optimal cutoff values for the NLR, PLR, LMR, PNI and SII were calculated based on the ROC curves and defined by calculating the Youden index in SPSS. The predictive values of the inflammatory indexes were evaluated by the AUC in SPSS (version 23.0). To avoid collinearity, the index with the highest predictive ability was entered into the multivariate analysis to establish a novel prognostic nomogram.
Follow-up
The patients were followed for 1 month after initial surgical treatment and every 3 months thereafter. The final follow-up evaluation was conducted in March 2020. Follow-up surveillance included routine blood tests, liver function tests, alpha-fetoprotein (AFP) levels, dynamic CT, and magnetic resonance imaging (MRI).
Statistical analysis
Continuous variables were analyzed using the Kruskal-Wallis test or Mann-Whitney U-test, and categorical variables were analyzed using the Pearson chi-square or Fisher’s exact test, as appropriate. Cumulative overall survival (OS) and recurrence-free survival (RFS) rates were calculated using the Kaplan-Meier method, and differences between curves were evaluated using the log-rank test. To investigate the association of prognostic factors with OS and RFS, we used the Cox proportional hazard model for the univariate and multivariate analyses, and hazard ratios (HRs) and 95% confidence intervals (CIs) were calculated. Univariate and multivariate logistical regression analyses were performed to assess the relationship between preoperative SO and systemic inflammation. A nomogram was generated by Rstudio (v1.2.1335, RRID:SCR_000432) and R software (v3.6.3, RRID:SCR_001905) using the “rms, survival, survminer, rmda” packages. The predictive accuracy and discriminative ability of the nomogram were evaluated by the concordance index (C-index) and calibration curves and compared with those of conventional staging systems. P<0.05 was considered significant. All statistical data were generated using SPSS (v23.0, RRID:SCR_002865), R software and Rstudio.
Results
Patient characteristics
Of the 452 patients included in the training cohort, 386 (85.4%) were men and 66 (14.6%) were women. The cohort was divided into two body composition groups: the NSO group, with 370 (81.9%) patients, and the SO group, with 82 (18.1%) patients. The clinical characteristics of each group are shown in Table 1. The median age was 54.00 years. The median SMI was 46.14 (cm2/m2). Men comprised the majority in each group (87.0%, 78.0%). HBsAg-positive patients (n=385) accounted for 85.2% of the training cohort. We found that the SO group had a higher rate of cardiovascular disease than the NSO group (P<0.025). Unlike the NSO group, the SO group had higher rates of multiple tumors, microvascular invasion, moderate differentiation and satellite lesions. The clinical characteristics of the validation cohort are shown in Table 1. These characteristics were not significantly different between the training cohort and validation cohort.
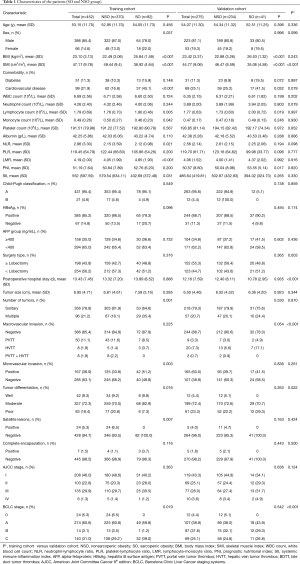
Full table
OS and RFS rates after hepatectomy for HCC
The median survival time was 47.0 months in the training cohort and 43.0 months in the validation cohort. The 1-, 3-, and 5-year OS rates in the entire cohort were 85.4%, 58.5%, and 45.5%, respectively. The 1-, 3-, and 5-year RFS rates in the entire cohort were 59.8%, 41.9%, and 31.8%, respectively. The 1-, 3-, and 5-year OS rates in the training cohort and validation cohort were 80.8%, 56.4%, and 45.3% and 86.2%, 61.8%, and 44.2%, respectively (P=0.713). The 1-, 3-, and 5-year RFS rates in the training cohort and validation cohort were 57.9%, 39.8%, and 29.6% and 62.8%, 45.4%, and 35.5%, respectively (P=0.220).
The 1-, 3-, and 5-year OS rates in the SO group and NSO group in the training cohort were 81.7%, 34.1%, and 17.3% and 84.3%, 61.9%, and 51.5%, respectively. The 1-, 3-, and 5-year RFS rates in the SO group and NSO group in the training cohort were 52.4%, 27.8%, and 12.4% and 60.3%, 43.4%, and 33.5%, respectively. The OS and RFS rates in the SO group were significantly lower than those in the NSO group in the training cohort (P<0.0001 and P=0.00037, respectively) (Figure 2A,B). The 1-, 3-, and 5-year OS rates in the SO group and NSO group in the validation cohort were 78.0%, 48.8%, and 25.9% and 87.6%, 64.1%, and 49.3%, respectively. The 1-, 3-, and 5-year RFS rates in the SO group and NSO group in the validation cohort were 43.9%, 21.3%, and 12.8% and 66.1%, 49.5%, and 39.2%, respectively. The OS and RFS rates in the SO group were significantly lower than those in the NSO group in the validation cohort (P=0.00028 and P<0.0001, respectively) (Figure 2C,D).
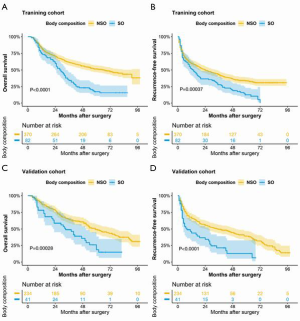
Inflammatory indexes
The following parameters were chosen for analysis: NLR, PLR, LMR, PNI and SII. The definitions and optimal cutoffs identified by calculating the AUCs are shown in Table 2. A higher NLR, higher PLR, higher SII, lower LMR, and lower PNI were correlated with poor OS and RFS (Table 3). The LMR had the highest AUC (0.624) for OS and the highest AUC (0.642) for RFS. The AUC values for the same inflammatory indexes in the validation cohort also followed a similar pattern. Some of the components of each inflammatory index were derived from the same laboratory test values; thus, if all these indexes were entered into the multivariate analysis, the problem of collinearity would be unavoidable. Thus, the LMR was selected for entry into the multivariate analysis to establish a novel prognostic nomogram for OS and RFS to account for collinearity.
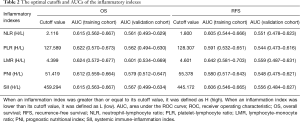
Full table
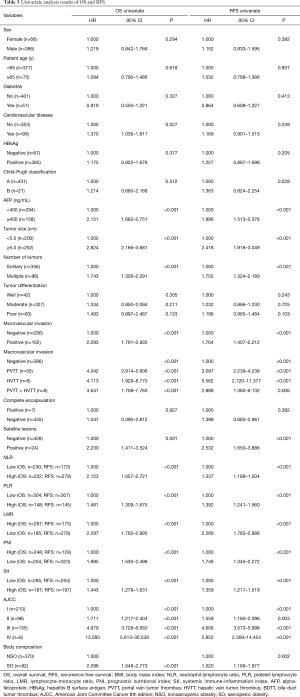
Full table
Prognostic factors for OS and RFS after hepatectomy for HCC
The univariate analysis showed that cardiovascular disease, AFP level, tumor size, number of tumors, microvascular invasion, macrovascular invasion, the presence of satellite lesions, the NLR, the PLR, the LMR (HR, 2.297; 95% CI, 1.762–2.995; P<0.001), the PNI, the SII, body composition (HR, 2.096; 95% CI, 1.548–2.773; P<0.001) and American Joint Committee on Cancer (AJCC) stage (8th edition) were prognostic factors of OS in patients who underwent hepatectomy for HCC (Table 3). The univariate analysis of RFS showed that the AFP level, the tumor size, the number of tumors, microvascular invasion, macrovascular invasion, the presence of satellite lesions, the NLR, the PLR, the LMR (HR, 1.896; 95% CI, 1.513–2.376; P<0.001), the PNI, the SII, body composition (HR, 1.520; 95% CI, 1.169–1.977; P=0.002) and the AJCC stage (8th edition) were prognostic factors of RFS in patients who underwent hepatectomy for HCC (Table 3).
According to the results of the multivariate analysis, SO (HR, 3.024; 95% CI, 2.239–4.084; P<0.001), a low LMR (HR, 2.103; 95% CI, 1.552–2.850; P<0.001), an AFP level ≥400 ng/mL (HR, 1.823; 95% CI, 1.410–2.359; P<0.001) and AJCC stage (8th edition) were independent prognostic factors of OS. Furthermore, SO (HR, 1.923; 95% CI, 1.461–2.530; P<0.001), a low LMR (HR, 2.161; 95% CI, 1.664–2.807; P<0.001), an AFP level ≥400 ng/mL (HR, 1.759; 95% CI, 1.391–2.226; P<0.001) and AJCC stage (8th edition) were also independent prognostic factors of RFS (Figure 3A,B).
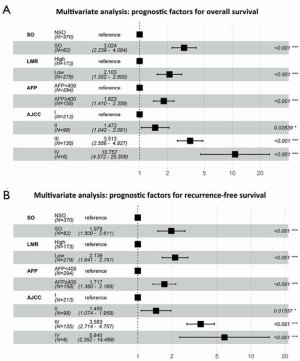
The correlations between SO and inflammatory indexes
Univariate and multivariate logistic regression analyses were performed to investigate whether the inflammatory indexes were associated with SO. The multivariate analysis showed that cardiovascular disease (HR, 3.707; 95% CI, 2.184–6.291; P<0.001), multiple tumors (HR, 2.754; 95% CI, 1.567–4.840; P<0.001), and a low LMR (HR, 2.336; 95% CI, 1.388–3.930; P=0.001) were independent predictors of SO (Table 4). The other four inflammatory indexes—the NLR, PLR, PNI and SII—were not independently associated with an increased risk of SO.
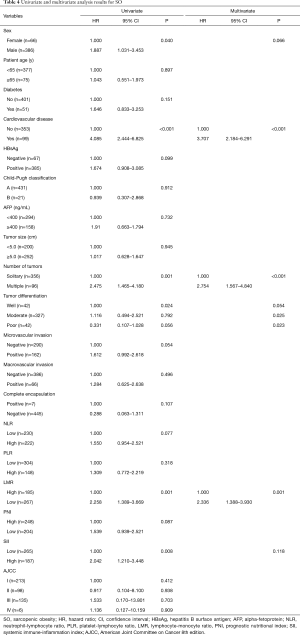
Full table
Subgroup analysis and SOLMR grades
Both SO and a low LMR were associated with poor OS (Figure 4A,B,C,D). To further investigate the relationship between SO and the LMR and their different prognoses, we attempted to combine SO and the LMR to generate four subgroups [SO high (SOH), SO low (SOL), NSO high (NSOH), and NSO low (NSOL)] (Figure 4E,F,G,H). The subgroup of patients with SO and a low LMR had the worst survival rate, and the subgroup of patients with NSO and a high LMR had the longest OS (P<0.0001). The SOH and NSOL subgroups did not have significantly different OS rates (P=0.691). Similar results were observed for RFS (P=0.364) (Figure 4E,F,G,H). Therefore, they were included in the validation cohort. There were no significant differences in prognostic outcomes between the two subgroups; thus, it was not necessary to separate the two subgroups for prognostic evaluation. Consequently, we combined these two subgroups to establish a new classification system called SOLMR grading. Patients with both NSO and a high LMR were classified as grade A. Patients with neither SO nor a low LMR were classified as grade B. Patients with both SO and a low LMR were classified as grade C. We also applied SOLMR grades to RFS (Table 5).
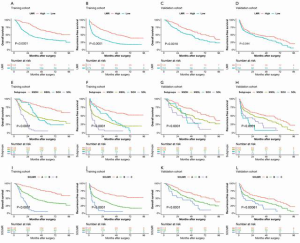
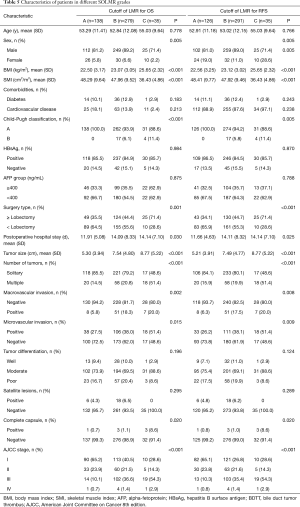
Full table
Kaplan-Meier OS curves and RFS curves were generated for the SOLMR grades. The SOLMR grades showed clearly different prognostic associations with OS and RFS in the training cohort and validation cohort (P<0.0001 and P<0.0001, respectively) (Figure 4I,J,K,L).
To further explore whether the SOLMR grade can replace SO and the LMR as a new independent prognostic factor for OS and RFS, SOLMR grade replaced SO and LMR in the univariate analysis and was found to be a prognostic factor of OS and RFS (P<0.001 and P<0.001, respectively) (Table 6). The multivariate analysis also revealed that the SOLMR grade was an independent prognostic factor for OS (SOLMR grade B: HR, 2.291; P<0.001, SOLMR grade C: HR, 6.535; P<0.001. Figure 5A) and an independent prognostic factor for RFS (SOLMR grade B: HR, 2.357; P<0.001, SOLMR grade C: HR, 3.979; P<0.001) (Figure 5B).
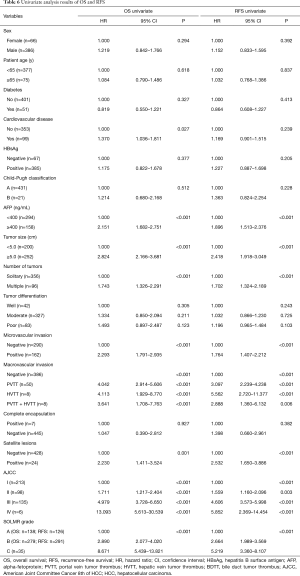
Full table
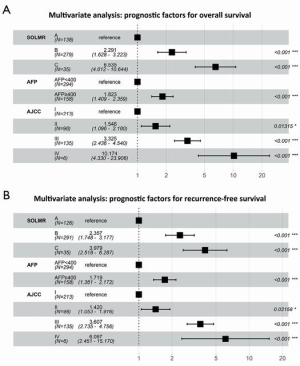
AUCs were evaluated to compare the prognostic accuracy of the SOLMR grade and each of its components—SO and the LMR. The AUCs of the SOLMR grade, SO and the LMR for OS were 0.688, 0.594 and 0.624, respectively (P<0.05). The AUCs of the SOLMR grade, SO and the LMR for OS were 0.687, 0.581 and 0.631, respectively (P<0.05).
Establishment of nomograms based on the SOLMR grade
A nomogram for OS prediction was established based on the identified independent prognostic factors: the SOLMR grade, AFP level, and AJCC stage. A nomogram for RFS prediction was established in the same way (Figure 6, Figure 7). The calibration plots for the probability of OS and RFS at 1, 3, and 5 years showed good correlation between the nomogram prediction and actual observations in the training cohort and validation cohort (Figure 6, Figure 7). Decision curves were plotted to compare the nomograms with other conventional staging systems (Figure 6, Figure 7). The nomograms resulted in the best net benefits. The clinical impact curves clearly revealed the true positive rates of the nomograms (Figure 6, Figure 7). The C-indexes of these two nomograms were 0.761 (95% CI, 0.734–0.788) and 0.736 (95% CI, 0.710–0.762), respectively, which were higher than those of any other system (P<0.0001 for all comparisons with each conventional staging system, Table 6). The same results were observed in the external validation cohort (Table 7).
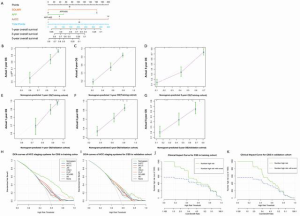
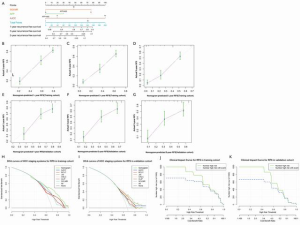
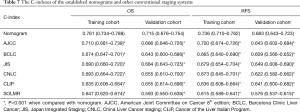
Full table
Discussion
Most European and American studies have found that sarcopenia is an independent risk factor for the prognosis of HCC in patients undergoing surgical resection (32). However, there is a relative lack of research on sarcopenia and SO in Chinese HCC populations. This study is the first to study the relationship between SO and HCC in a Chinese population. Our study identified preoperative SO as an independent risk factor of poor OS and RFS in patients undergoing hepatectomy for HCC. Furthermore, similar to most studies in Europe and the United States, this study found that sarcopenia also had significant effects on the prognosis of HCC in patients undergoing radical surgical resection in the Chinese population (Figures S1,S2; Table S1).
To date, the cutoff value for SO defined by the SMI has not been agreed upon. In this study, a new cutoff value based on the best discriminatory index in the Chinese population was proposed via the ROC curve method; this cutoff value was compared with other SMI cutoff values frequently cited in studies (8,19,29,32-35) (Tables S1,S2; Figures S1,S2). Our SMI cutoff values of 40.86 cm2/m2 for males and 30.71 cm2/m2 for females were very close to those proposed by Kobayashi et al. for the Japanese HCC population (18), which were 40.31 cm2/m2 for males and 30.88 cm2/m2 for females; these similarities may be due to racial similarities or similarity between the Chinese HCC population and the Japanese HCC population. For the specific definition of obesity in SO, some scholars have suggested that the visceral fat area (VFA) measured on CT be used as the new definition of obesity (36,37). The VFA represents only visceral fat; notably, it is a type of obesity distribution but not a comprehensive representation of obesity (38). Diagnosing obesity considering the World Health Organization’s (WHO’s) obesity definition based on BMI is the most recognized and convenient method worldwide. Therefore, this study applied the WHO definition of obesity.
Previous studies have identified an association between systemic inflammation and HCC prognosis (39-41). Whether there is a correlation between SO and systemic inflammation in HCC and its prognostic value remains unclear. In this study, five inflammatory indexes were evaluated, and the LMR was proven to be the best independent predictor of OS and RFS. A low LMR, cardiovascular disease and multiple tumors were closely associated with the occurrence of SO. In the past, many inflammatory indexes, such as the NLR and PLR, have been proposed, but they are rarely applied in clinical practice. This paper further explored their value and their utility when combined with SO, which was calculated by analyzing the clinical data of nonintraperitoneal tissues obtained from imaging examinations, as a new prediction model. Based on the results of our study, we inferred that there is a triangular relationship among SO, a low LMR and a poor HCC prognosis.
Recent studies have shown that skeletal muscle loss with adipose tissue gain leads to increased levels of inflammatory adipokines such as leptin, chemerin, and resistin and cytokines such as tumor necrosis factor (TNF)-α, interleukin (IL)-6, and interferon (INF)-γ as well as decreased concentrations of adiponectin or myokines such as IL-15 (42-44). In addition, liver cirrhosis patients experience reduced insulin inactivation, resulting in a certain amount of insulin resistance. This unfavorable adipokine/cytokine profile further increases insulin resistance, which amplifies inflammation and oxidative stress and contributes to ectopic fat deposition. Wannamethee et al. observed a continuous cycle involving “decline in physical activity-sarcopenia-insulin resistance-inflammation-oxidative stress”, which results in the development of SO (45). All these changes contribute to the progression of HCC.
This study is the first to combine SO and the LMR to obtain a SOLMR grade in HCC research. Based on the above conclusions in this study, we developed a novel classification system called SOLMR grading via subgroup analysis. The multivariate analysis suggested that the SOLMR grade was an independent prognostic factor for patients with HCC. The OS and RFS of patients with SOLMR grade C disease were far worse than those with grades B and A disease. According to the 8th edition AJCC and the Barcelona Clinic Liver Cancer (BCLC) staging systems, we plotted survival curves stratified by different stages (Figure S3). The results suggested that SOLMR grade had better discrimination in each AJCC stage than BCLC stage. Considering that the BCLC staging systems contains performance status (PS) scores, if we use SOLMR grade to further subdivide the general physical condition, it may lead to bias. Therefore, we recommend use the SOLMR grade for stratification on the basis of the AJCC staging systems instead of the BCLC staging systems. Among the clinical characteristics associated with the SOLMR grade, the factors that were considered to be related to poor prognosis, such as age, cardiovascular disease, liver function classification, AFP level, liver resection volume, length of hospital stay, tumor size, number of tumors, occurrence of vascular invasion and tumor stage, were all highest in grade A and lowest in grade C (Table 5). Therefore, the SOLMR grade is quite representative. In clinical practice, the SOLMR grade can be used as a risk stratification tool to supplement the TNM staging system to better stratify patients by risk and to provide an accurate basis for postoperative follow-up and treatment guidance. Close follow-up and aggressive postoperative adjuvant therapies, such as transarterial chemoembolization (TACE), targeted therapy and immunotherapy, are recommended for HCC patients with SOLMR grades B and C.
Although those traditional staging systems provide good guidance for clinical treatment, most of them focus on tumor progression and ignore the general physical condition of the patient, resulting in a biased assessment of the prognosis. In the era of individualized precision medicine, a nomogram was constructed by incorporating both the SOLMR grade and the AJCC stage; this nomogram resulted in better in predictive accuracy and discriminative ability than AJCC staging alone. The nomograms were comprehensive, as they accounted for general body-inflammation status, serological indicators, and tumor stage. Moreover, regarding their convenience in clinical practice, the nomograms utilized three variables that were easy to obtain and required only the basic AJCC stage. The BCLC and China Liver Cancer (CNLC) staging systems also include PS scores to indicate general physical condition. However, PS scores are easily affected by subjective patient factors, and indications for surgery in the BCLC staging seem to be too conservative. With the development of HCC surgery, almost all areas are now accessible, and the relatively conservative indication for surgery in the BCLC system seems to contrast with that development, especially in China where there are many cases of large-volume and advanced-stage HCC. As a new predictive tool in HCC, the application value of our nomograms in China requires long-term evaluation.
There were several limitations in our study. First, as a retrospective, small-sample study, it may have been subject to selection bias, and validation in a larger sample could be more convincing. Second, calculating the SMI requires relatively complex imaging calculations, and a convenient, automated software program needs to be further developed. Finally, the preoperative stress state of the patient or comorbidities may have affected the variation in the LMR. Thus, the optimal time window and physiological status for obtaining blood samples have yet to be determined.
Conclusions
Our study is the first to demonstrate that the preoperative LMR was significantly associated with SO in HCC patients and the first to proposed SOLMR grading in HCC. The novel visualized nomograms developed from the SOLMR grading system combining SO with the inflammatory index provided better prognostic estimates than the traditional staging systems. Thus, more in-depth studies on SO and inflammation and a better understanding of specific molecular mechanisms and pathways may lead to the development of novel therapies to improve HCC outcomes.
Acknowledgments
Funding: None.
Footnote
Reporting Checklist: The authors have completed the REMARK reporting checklist. Available at http://dx.doi.org/10.21037/jgo-20-341
Data Sharing Statement: Available at http://dx.doi.org/10.21037/jgo-20-341
Conflicts of Interest: All authors have completed the ICMJE uniform disclosure form (available at http://dx.doi.org/10.21037/jgo-20-341). The authors have no conflicts of interest to declare.
Ethical Statement: The authors are accountable for all aspects of the work in ensuring that questions related to the accuracy or integrity of any part of the work are appropriately investigated and resolved. The study was conducted in accordance with the Declaration of Helsinki (as revised in 2013). The study was approved by the institutional review boards of Fujian Provincial Hospital and Fujian Medical University Union Hospital (No. K2019-12-025; 2019KY022) and Informed consent was obtained from all patients before inclusion in the study.
Open Access Statement: This is an Open Access article distributed in accordance with the Creative Commons Attribution-NonCommercial-NoDerivs 4.0 International License (CC BY-NC-ND 4.0), which permits the non-commercial replication and distribution of the article with the strict proviso that no changes or edits are made and the original work is properly cited (including links to both the formal publication through the relevant DOI and the license). See: https://creativecommons.org/licenses/by-nc-nd/4.0/.
References
- Fujiwara N, Nakagawa H, Kudo Y, et al. Sarcopenia, intramuscular fat deposition, and visceral adiposity independently predict the outcomes of hepatocellular carcinoma. J Hepatol 2015;63:131-40. [Crossref] [PubMed]
- Cruz-Jentoft AJ, Sayer AA. Sarcopenia. Lancet 2019;393:2636-46. Erratum in: Lancet 2019;393:2590. [Crossref] [PubMed]
- Polyzos SA, Margioris AN. Sarcopenic obesity. Hormones (Athens) 2018;17:321-31. [Crossref] [PubMed]
- Harimoto N, Shirabe K, Yamashita YI, et al. Sarcopenia as a predictor of prognosis in patients following hepatectomy for hepatocellular carcinoma. Br J Surg 2013;100:1523-30. [Crossref] [PubMed]
- Dodson RM, Firoozmand A, Hyder O, et al. Impact of sarcopenia on outcomes following intra-arterial therapy of hepatic malignancies. J Gastrointest Surg 2013;17:2123-32. [Crossref] [PubMed]
- Meza-Junco J, Montano-Loza AJ, Baracos VE, et al. Sarcopenia as a prognostic index of nutritional status in concurrent cirrhosis and hepatocellular carcinoma. J Clin Gastroenterol 2013;47:861-70. [Crossref] [PubMed]
- Kobayashi A, Kaido T, Hamaguchi Y, et al. Impact of postoperative changes in sarcopenic factors on outcomes after hepatectomy for hepatocellular carcinoma. J Hepatobiliary Pancreat Sci 2016;23:57-64. [Crossref] [PubMed]
- Iritani S, Imai K, Takai K, et al. Skeletal muscle depletion is an independent prognostic factor for hepatocellular carcinoma. J Gastroenterol 2015;50:323-32. [Crossref] [PubMed]
- Valero V 3rd, Amini N, Spolverato G, et al. Sarcopenia adversely impacts postoperative complications following resection or transplantation in patients with primary liver tumors. J Gastrointest Surg 2015;19:272-81. [Crossref] [PubMed]
- Voron T, Tselikas L, Pietrasz D, et al. Sarcopenia impacts on short- and long-term results of hepatectomy for hepatocellular carcinoma. Ann Surg 2015;261:1173-83. [Crossref] [PubMed]
- Kamachi S, Mizuta T, Otsuka T, et al. Sarcopenia is a risk factor for the recurrence of hepatocellular carcinoma after curative treatment. Hepatol Res 2016;46:201-8. [Crossref] [PubMed]
- Takagi K, Yagi T, Yoshida R, et al. Sarcopenia and American Society of Anesthesiologists physical status in the assessment of outcomes of hepatocellular carcinoma patients undergoing hepatectomy. Acta Med Okayama 2016;70:363-70. [PubMed]
- Hiraoka A, Hirooka M, Koizumi Y, et al. Muscle volume loss as a prognostic marker in hepatocellular carcinoma patients treated with sorafenib. Hepatol Res 2017;47:558-65. [Crossref] [PubMed]
- Chang KV, Chen JD, Wu WT, et al. Association between loss of skeletal muscle mass and mortality and tumor recurrence in hepatocellular carcinoma: a systematic review and meta-analysis. Liver Cancer 2018;7:90-103. [Crossref] [PubMed]
- Itoh S, Shirabe K, Matsumoto Y, et al. Effect of body composition on outcomes after hepatic resection for hepatocellular carcinoma. Ann Surg Oncol 2014;21:3063-8. [Crossref] [PubMed]
- Levolger S, van Vledder MG, Muslem R, et al. Sarcopenia impairs survival in patients with potentially curable hepatocellular carcinoma. J Surg Oncol 2015;112:208-13. [Crossref] [PubMed]
- Yabusaki N, Fujii T, Yamada S, et al. Adverse impact of low skeletal muscle index on the prognosis of hepatocellular carcinoma after hepatic resection. Int J Surg 2016;30:136-42. [Crossref] [PubMed]
- Kobayashi A, Kaido T, Hamaguchi Y, et al. Impact of sarcopenic obesity on outcomes in patients undergoing hepatectomy for hepatocellular carcinoma. Ann Surg 2019;269:924-31. [Crossref] [PubMed]
- Mei KL, Batsis JA, Mills JB, et al. Sarcopenia and sarcopenic obesity: do they predict inferior oncologic outcomes after gastrointestinal cancer surgery? Perioper Med (Lond) 2016;5:30. [Crossref] [PubMed]
- Kroh A, Uschner D, Lodewick T, et al. Impact of body composition on survival and morbidity after liver resection in hepatocellular carcinoma patients. Hepatobiliary Pancreat Dis Int 2019;18:28-37. [Crossref] [PubMed]
- Hamaguchi Y, Kaido T, Okumura S, et al. Preoperative visceral adiposity and muscularity predict poor outcomes after hepatectomy for hepatocellular carcinoma. Liver Cancer 2019;8:92-109. [Crossref] [PubMed]
- Itoh S, Yoshizumi T, Kimura K, et al. Effect of sarcopenic obesity on outcomes of living-donor liver transplantation for hepatocellular carcinoma. Anticancer Res 2016;36:3029-34. [PubMed]
- Baffy G. Sarcopenic obesity in liver cancer: it is SO complicated. Hepatobiliary Surg Nutr 2019;8:560-2. [Crossref] [PubMed]
- Jo E, Lee SR, Park BS, et al. Potential mechanisms underlying the role of chronic inflammation in age-related muscle wasting. Aging Clin Exp Res 2012;24:412-22. [PubMed]
- Budui SL, Rossi AP, Zamboni M. The pathogenetic bases of sarcopenia. Clin Cases Miner Bone Metab 2015;12:22-6. [PubMed]
- Lin JX, Lin JP, Xie JW, et al. Prognostic value and association of sarcopenia and systemic inflammation for patients with gastric cancer following radical gastrectomy. Oncologist 2019;24:e1091-101. [Crossref] [PubMed]
- Coussens LM, Werb Z. Inflammation and cancer. Nature 2002;420:860-7. [Crossref] [PubMed]
- Bishayee A. The role of inflammation and liver cancer. Adv Exp Med Biol 2014;816:401-35. [Crossref] [PubMed]
- Prado CM, Lieffers JR, McCargar LJ, et al. Prevalence and clinical implications of sarcopenic obesity in patients with solid tumours of the respiratory and gastrointestinal tracts: a population-based study. Lancet Oncol 2008;9:629-35. [Crossref] [PubMed]
- Expert Consultation WHO. Appropriate body-mass index for Asian populations and its implications for policy and intervention strategies. Lancet 2004;363:157-63. Erratum in: Lancet 2004;363:902. [Crossref] [PubMed]
- Chen J, Fang A, Chen M, et al. A novel inflammation-based nomogram system to predict survival of patients with hepatocellular carcinoma. Cancer Med 2018;7:5027-35. [Crossref] [PubMed]
- van Vugt JLA, Alferink LJM, Buettner S, et al. A model including sarcopenia surpasses the MELD score in predicting waiting list mortality in cirrhotic liver transplant candidates: A competing risk analysis in a national cohort. J Hepatol 2018;68:707-14. [Crossref] [PubMed]
- Martin L, Birdsell L, Macdonald N, et al. Cancer cachexia in the age of obesity: skeletal muscle depletion is a powerful prognostic factor, independent of body mass index. J Clin Oncol 2013;31:1539-47. [Crossref] [PubMed]
- Sakurai K, Kubo N, Tamura T, et al. Adverse effects of low preoperative skeletal muscle mass in patients undergoing gastrectomy for gastric cancer. Ann Surg Oncol 2017;24:2712-9. [Crossref] [PubMed]
- Zhuang CL, Huang DD, Pang WY, et al. Sarcopenia is an independent predictor of severe postoperative complications and long-term survival after radical gastrectomy for gastric cancer: analysis from a large-scale cohort. Medicine (Baltimore) 2016;95:e3164 [Crossref] [PubMed]
- Engin A. The definition and prevalence of obesity and metabolic syndrome. Adv Exp Med Biol 2017;960:1-17. [Crossref] [PubMed]
- Choi KM. Sarcopenia and sarcopenic obesity. Korean J Intern Med 2016;31:1054-60. [Crossref] [PubMed]
- Pou KM, Massaro JM, Hoffmann U, et al. Patterns of abdominal fat distribution: the Framingham Heart Study. Diabetes Care 2009;32:481-5. [Crossref] [PubMed]
- Hu B, Yang XR, Xu Y, et al. Systemic immune-inflammation index predicts prognosis of patients after curative resection for hepatocellular carcinoma. Clin Cancer Res 2014;20:6212-22. [Crossref] [PubMed]
- Sanghera C, Teh JJ, Pinato DJ. The systemic inflammatory response as a source of biomarkers and therapeutic targets in hepatocellular carcinoma. Liver Int 2019;39:2008-23. [Crossref] [PubMed]
- Pinato DJ, Stebbing J, Ishizuka M, et al. A novel and validated prognostic index in hepatocellular carcinoma: the inflammation based index (IBI). J Hepatol 2012;57:1013-20. [Crossref] [PubMed]
- Kalinkovich A, Livshits G. Sarcopenic obesity or obese sarcopenia: a cross talk between age-associated adipose tissue and skeletal muscle inflammation as a main mechanism of the pathogenesis. Ageing Res Rev 2017;35:200-21. [Crossref] [PubMed]
- Lutz CT, Quinn LS. Sarcopenia, obesity, and natural killer cell immune senescence in aging: altered cytokine levels as a common mechanism. Aging (Albany NY) 2012;4:535-46. [Crossref] [PubMed]
- Polyzos SA, Kountouras J, Mantzoros CS. Adipose tissue, obesity and non-alcoholic fatty liver disease. Minerva Endocrinol 2017;42:92-108. [PubMed]
- Wannamethee SG, Atkins JL. Muscle loss and obesity: the health implications of sarcopenia and sarcopenic obesity. Proc Nutr Soc 2015;74:405-12. [Crossref] [PubMed]