Development of a dual-energy spectral computed tomography-based nomogram for the preoperative discrimination of histological grade in colorectal adenocarcinoma patients
Introduction
Colorectal adenocarcinoma (CRAC) is the most common histological type of colorectal cancer (CRC) (1,2). Previous studies have shown that the histological differentiation is one of the most significant factors for CRC prognosis (3,4). In clinical practice, the histopathological report of CRC routinely includes the histological grade. CRC tumors are classified as well (G1), moderately (G2), and poorly (G3) differentiated according to the gland formation rate (3). However, well and moderately differentiated tumors exhibit similar biological behaviors, causing inconsistency among observers in differentiating them. Thus, the World Health Organization recommended using a two-tier classification system to replace the traditional one; this new classification defines low-grade CRC as the merge of well and moderately differentiated tumors; and classifies poorly differentiated tumors as high-grade (4). High-grade CRAC has a higher risk of recurrence and poorer prognosis than low-grade CRAC after tumor resection. Preoperative radiotherapy or chemoradiotherapy improves the prognosis and lowers recurrence rate in high-grade CRAC patients (5,6). However, neoadjuvant therapy has also been reported to have serious side effects (7). Therefore, it is essential to correctly identify and treat patients at a high risk of recurrence, while also avoiding unnecessary side effects caused by preoperative overtreatment to low-grade CRAC patients.
A colonoscopic biopsy is a common clinical diagnostic method of CRC that can provide information of histological grade. However, it is an invasive examination that might cause some side effects, such as bleeding and colon perforation (8). Moreover, biopsy has some disadvantages, including poor orientation, intratumoral heterogeneity, and poor sample quality. As a result, inconsistency might occur in the evaluation of histological grade between biopsy and surgical specimens (4). Therefore, a noninvasive, easily repeatable method to identify the histological grade of tumors is highly desirable for more individualized and accurate treatment of CRC patients.
The National Comprehensive Cancer Network guidelines recommend computed tomography (CT) as the clinically preferred imaging examination for CRC (9). However, conventional CT techniques have obvious limitations in evaluating the CRAC histological grades as it cannot be observed by the naked eye. Dual-energy spectral CT (DESCT) provides information on various parameters that could be used as indicators for quantitative analysis (10). Moreover, DESCT has been widely used in clinical practice to identify and malignant lymph nodes, evaluate the response to neoadjuvant treatment, and evaluate microsatellite instability status in patients with CRC (11-14). Previously published studies have also demonstrated the value of energy spectrum CT in other abdominal tumors, including pathological grading of clear cell renal cell carcinoma (15), differential diagnosis of chromophobe renal cell carcinoma and papillary renal cell carcinoma (16), risk classification of gastrointestinal stromal tumor (17), distinguishing between gastric schwannomas and gastrointestinal stromal tumors (18), and identifying liver cancer and hepatic focal nodular hyperplasia (19). Nomograms have been widely used to quantify the risk factors of CRC biological characteristics (20-22). Previous studies (21,23,24) have shown that a combination of clinical factors and CT parameters could effectively improve nomogram performance in tumor diagnosis. However, to date, no study has shown the ability of a nomogram, which combines DESCT parameters and clinical factors, to discriminate between CRAC histological grades. Further, the correlation between such a nomogram-based histological grade prediction and prognosis is unclear. Therefore, this study aimed to develop a nomogram that incorporates both DESCT parameters and clinical factors and can be used for preoperative individualized discrimination of CRAC histological grade. We also aimed to assess the ability of such nomogram to predict the overall survival of CRAC patients.
We present the following article in accordance with the STARD Checklist (available at http://dx.doi.org/10.21037/jgo-20-368).
Methods
The study was conducted in accordance with the Declaration of Helsinki (as revised in 2013). This retrospective study was approved by our institutional review board Lanzhou University Second Hospital Medical Ethics Committee (No. 2020A-189), and the informed consent requirement was waived owing to the retrospective nature of the study.
Patients
We retrospectively evaluated 173 CRAC patients who underwent preoperative abdominal DESCT between April 2016 and August 2019. The inclusion criteria were as follows: (I) pathologically diagnosed CRAC; (II) a definite postoperative tumor histological grade; (III) complete clinical information; and (IV) available overall survival data. The exclusion criteria were as follows: (I) history of any anti-cancer therapy; (II) target lesion invisible on CT images; and (III) poor image quality. Figure 1 shows the patient recruitment flowchart.
Clinical characteristics, outcomes, and follow-up details
Data on the patients’ clinical and pathological characteristics were collected from their medical records. Baseline clinical characteristics included age, sex, and tumor location. Laboratory analysis consisted of carcinoembryonic antigen (CEA; threshold, 5 ng/mL), carbohydrate antigen 125 (CA125; threshold, 35 U/mL), and carbohydrate antigen 199 (CA199; threshold, 37 U/mL).
The prognostic value of the DESCT-based prediction model was evaluated using the overall survival data. Overall survival was defined as the time from CT examination to death or the last follow-up, whichever came first. Patients were monitored every 3–6 months during the first two years after surgery, and then, every 6–12 months over the subsequent 3–5 years. Follow-up was right-censored on October 30, 2019. The patients were followed up for a mean of 21.8 months (range, 1.4–41.6 months).
The histological grade status was evaluated by trained pathologists using the surgical specimens. Two histological grade categories were used: low- and high-grade CRAC. Well or moderately differentiated tumors were considered low-grade CRAC, and poorly differentiated tumors were considered high-grade CRAC.
Dual-energy spectral computed tomography (DESCT) imaging
All patients underwent standard bowel preparation. Contrast-enhanced abdominal dual-energy CT scans were performed on a Discovery CT750 HD system (GE Medical Systems, GE Healthcare, Waukesha, WI, USA), with the patients in a supine position. Patients were intravenously injected with iodixanol (1 mL/kg) using a high-pressure dual-cylinder injector. Arterial (AP) and venous phases (VP) imaging were performed, respectively, 25–30 s and 60–70 s after the contrast agent was administered. Other CT scanning parameters were as follows: fast tube voltage switching, between 70–140 kVp; tube current, 350 mA; pitch, 0.984:1; rotation time, 0.75 s; reconstructed layer thickness, 1.25 mm.
Image post-processing and analysis
Raw CT images were transferred to a GE ADW 4.6 workstation (GE Healthcare, Milwaukee, WI, USA). Gemstone Spectral Imaging (GSI) viewer software in this workstation was used to quantitatively evaluate virtual monochrome images with a default of 70 keV, iodine-based decomposition images and effective atomic number images. Two radiologists with more than 5 years’ of experience in gastrointestinal radiology analyzed the images. Both radiologists were blinded to the patient’s clinicopathological data. The maximum tumor thickness was defined as the maximum diameter perpendicular to the long axis on the cross-sectional image. The tumor gross pattern (TGP) was classified as polypoid or non-polypoid according to the presence of a polypoid appearance. Pericolorectal fat invasion (PFI) was defined as the extension of the primary tumor beyond the muscularis propria and into the pericolorectal fat. Clinical tumor stage (cT stage) and clinical node stage (cN stage) were evaluated following to the 8th edition of the American Joint Committee on Cancer Staging system (25). The regions of interest (ROIs) were uniform circles, with an average area of 97.27 mm2. ROIs were placed over the high-enhancement areas of the tumor. Vessels, calcification, cystic degeneration, and necrosis were carefully avoided. The monochromatic CT values, effective atomic number (Eff-Z), and iodine concentration (IC) in the AP and VP were generated using the GSI viewer software package after loading the AP and VP images to the workstation. All measurements were performed three times to minimize bias, and the average of the three values was taken as the final value. Figure 2 shows a representative DESCT image and the quantitative measurement of the DESCT parameters using the ROI in high- and low-grade CRAC patients.
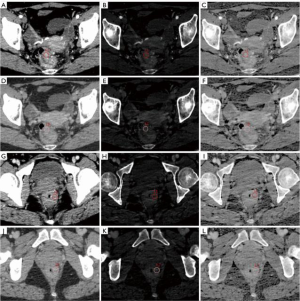
Statistical analysis
All data analyses were performed using an R software package (Version 3.6.3; http://www.Rproject.org). We first built a logistic regression model that could generate a nomogram to discriminate between low- and high-grade CRAC. Second, we also compared the survival between pathological grading, logistic model predicts grading and cox model direct predict low- and high-risk group. The intra-class correlation coefficient (ICC) was used to evaluate interobserver consistency between the radiologists. The Student’s t-test or Mann-Whitney U test and the Chi-square test or Fisher’s exact test were used to compare the DESCT parameters and clinical factors between the high- and low-grade CRAC. Statistically significant features in the univariate analysis were entered into a multivariate logistic regression model to identify the key indicators for histological grade discrimination in CRAC patients.
A backward stepwise selection was applied, in which the stopping rule was based on the likelihood-ratio test with Akaike’s Information Criterion. A quantitative and easy-to-use nomogram, designed to discriminate high-grade CRAC from low-grade CRAC, was then built based on the final regression coefficient. A ROC curve analysis was used to evaluate the nomogram’s diagnostic capabilities. We calculated the area under the curve (AUC) and its 95% CI, sensitivity, and specificity with respect to our reference standard (pathological grading). Calibration curves assessed the goodness fit of the nomogram. To verify the clinical usefulness of the nomogram, we quantified the net benefit at different threshold probabilities in the dataset using decision curve analysis (DCA) curves. Kaplan-Meier (KM) survival analysis and log rank test were performed to evaluate the prognostic value of nomogram. Additionally, we used Cox regression to analyze the correlation between statistically significant predictors and the survival, and assess the discrimination ability between the ground truth of histological grade and the nomogram. The maximally selected rank statistics was used to find cutoff point to distinguish low- and high-groups (26). All statistical tests were two sided, and a P value <0.05 was considered statistically significant.
Results
Interobserver agreement
There was a good consistency between the two radiologists in their evaluation of the five general CT features and six DESCT features evaluated by the two radiologists. The ICC values for the evaluated parameters were as follows: the maximum tumor thickness, 0.884; TGP, 0.838; PFI, 0.922; cT stage, 0.855; cN stage, 0.796; 70 keV monochromatic CT value in the AP (CTAP, 0.904) and VP (CTVP, 0.900); IC values in the AP (ICAP, 0.934) and VP (ICVP, 0.927); Eff-Z in the AP (Eff-ZAP, 0.825) and VP (Eff-ZVP, 0.802).
Clinical and CT imaging characteristics
The average patient age was 57.67±13.43 years, and 95 patients (54.9%) were men. Of the 173 patients, 65 had pathologically confirmed high-grade and 108 had low-grade CRAC. The high-grade CRAC group had significantly higher CA125, CA199, PFI, and cT and cN stages than the low-grade CRAC group (all P<0.05; Table 1). The high-grade CRAC group also had significantly higher values of ICAP, ICVP, Eff-ZAP, and Eff-ZVP, and a significantly lower age (all P<0.05; Table 1). The other clinical and CT imaging characteristics were not significantly different between the two groups (all P>0.05).
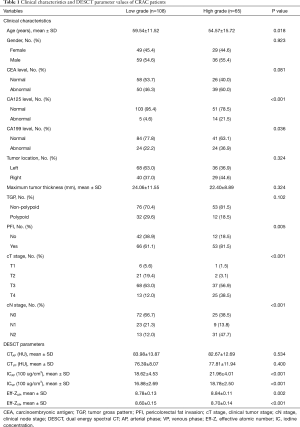
Full table
Development of individualized prediction model for high-grade CRAC
Statistically significant indicators in the univariate analysis were included in the multivariate logistic regression model. Finally, age, cT stage, cN stage, ICAP, and ICVP were identified as independent predictors of high-grade CRAC. A quantitative nomogram was constructed for individualized discrimination between CRAC histological grades based on these parameters (Figure 3). The ROC curves (Figure 4) showed that the nomogram had an excellent discriminating capability, with an AUC of 0.886 (95% CI, 0.834–0.938). The sensitivity and specificity were 0.862 and 0.704, respectively. The calibration curve of the nomogram showed favorable agreement between prediction and observation for in discriminating between the different CRAC histological grades (Figure 5A). The DCA curve of the nomogram also showed that the nomogram added greater benefit than a treat-all or treat-none schemes; this was due to the favorable discrimination between CRAC histological grade of CRAC when the threshold probability of a patient or doctor ranged between 10% and 90% (Figure 5B).
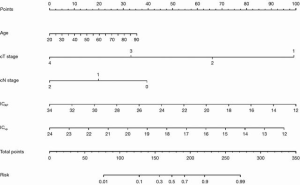
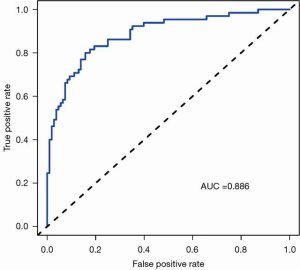
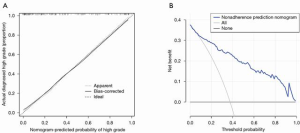
Survival analysis
We stratified the patients into the high- and low-risk groups based on the nomogram score cut-off value. The KM curve for overall survival showed that our nomogram identified a significant difference between the high- and low-risk group (HR, 2.188; 95% CI, 1.072–4.465; log-rank P=0.027; Figure 6A). This difference was similar to that identified by the histological grading as the ground truth (HR, 2.617; 95% CI, 1.407–4.867; log-rank P=0.0016; Figure 6B). Cox multivariate regression analysis showed that histological grade and ICAP were independent predictors for overall survival in CRAC patients. A model that combined the histological grade and ICAP also showed significantly different overall survival between the high- and low-risk groups (HR, 2.617; 95% CI, 1.407–4.867; log-rank P=0.0016; Figure 6C). The log-rank test yielded similar results of the three KM curves indicating that histological grade was the primary prognostic factor.
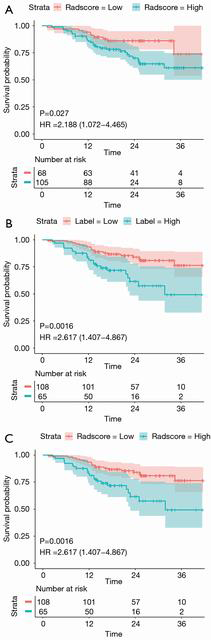
Discussion
No study has previously evaluated the usefulness of a DESCT-based nomogram for discriminating between CRAC histological grades. This study found that incorporating the DESCT features and clinical factors into a nomogram helps to preoperatively discriminate between histological grades in CRAC patients. The nomogram comprised five preoperatively obtainable parameters (age, cT stage, cN stage, ICAP, and ICVP) and showed a high discriminative performance between CRAC histological grades. The calibration and DCA curves also showed excellent model stability and actual benefit. Further, the nomogram was promising in the prediction of overall survival.
High-grade CRAC patients in our study were younger than those in the low-grade group, and age was an independent predictor for CRAC histological grade. Several studies defined young CRC patients as those aged <50 years and found that young CRC patients were more likely to have poorly differentiated tumors (27-29). Moreover, we found that cT and cN stages in high-grade CRAC patients were higher than that in low-grade CRAC patients. This finding is consistent with previous studies that showed an association between high-grade CRAC and more aggressive biological behavior, including an advanced tumor stage (30,31). cT and cN stages were also identified as independent predictors of CRAC histological grade in our study. These are qualitative parameters that can be easily obtained in CT imaging and used as a noninvasive tool for histological grade of CRAC.
Tumor angiogenesis is closely associated with tumor growth, progression, and metastasis (32,33). A high rate of angiogenesis in CRC is correlated with aggressive histopathological features, including a higher tumor histological grade (11,34,35). IC can reflect the degree of tumor vascularization by quantitatively indicating the deposition of iodine in the tissue (36). In the present study, we found significantly higher IC values in the AP and VP of the high-grade CRAC group, suggesting a greater blood supply to these tumors. Our findings are consistent with those reported by Gong et al. (35).
Previous imaging studies have evaluated diverse modalities, including magnetic resonance imaging (MRI), dual-energy spectral CT, and CT-based radiomics, for their ability to differentiate between CRC histological grades. Akashi et al. (37) evaluated the correlation between tumor histopathologic parameters and apparent diffusion coefficient (ADC) values in rectal cancer. They found that tumors with a higher histological grade had lower mean ADC (P<0.05). Other studies that investigated the value of dual-energy spectrum CT for differentiating between high- and low-histological grade CRC tumors also found significantly higher IC values in high-grade tumors (11,35). Huang et al. (4) developed a CT-based radiomics model to discriminate between high- and low-grade CRC. Their model showed excellent discriminatory capability in both the training and validation datasets. In our study, the ROC curve showed that our model had an excellent discriminating performance, with an AUC of 0.886. Our model had a higher AUC than that used by Yang et al. (11), suggesting that a combination of DESCT parameter and clinical factors could improve its histological grade discrimination performance in CRAC. Our study has several limitations. First, there was an inevitable selection bias due to the retrospective study design. This should be addressed by future prospective studies with external validity. Second, the number of patients was limited and, thus, the results should be validated by studies with a larger number of patients. Other commercially available DESCT platforms should also be evaluated. Finally, signet ring cell carcinoma and undifferentiated carcinoma were not included in our study due to the rarity of these tumors. Future studies are expected to use DESCT to differentiate between these rare differentiated tumors and adenocarcinomas.
Conclusions
We developed a nomogram that incorporates both DESCT parameters and clinical factors to discriminate preoperatively between CRAC histological grades. The nomogram could help to develop more reasonable and effective therapeutic strategies for CRAC patients.
Acknowledgments
Funding: This work was supported by the National Natural Science Foundation of China (81772006, 82071872 to JZ); Talent Innovation and Entrepreneurship Project of Lanzhou (2016 RC 58 to JZ); and Open Fund project of Key Laboratory of Medical Imaging of Gansu Province (GSYX202009 to YC).
Footnote
Reporting Checklist: The authors have completed the STARD checklist. Available at: http://dx.doi.org/10.21037/jgo-20-368
Data Sharing Statement: available at: http://dx.doi.org/10.21037/jgo-20-368
Conflicts of Interest: All authors have completed the ICMJE uniform disclosure form (available at: http://dx.doi.org/10.21037/jgo-20-368). The authors have no conflicts of interest to declare.
Ethical Statement: The authors are accountable for all aspects of the work in ensuring that questions related to the accuracy or integrity of any part of the work are appropriately investigated and resolved. The study was conducted in accordance with the Declaration of Helsinki (as revised in 2013). This retrospective study was approved by our institutional review board Lanzhou University Second Hospital Medical Ethics Committee (No. 2020A-189), and the informed consent requirement was waived owing to the retrospective nature of the study.
Open Access Statement: This is an Open Access article distributed in accordance with the Creative Commons Attribution-NonCommercial-NoDerivs 4.0 International License (CC BY-NC-ND 4.0), which permits the non-commercial replication and distribution of the article with the strict proviso that no changes or edits are made and the original work is properly cited (including links to both the formal publication through the relevant DOI and the license). See: https://creativecommons.org/licenses/by-nc-nd/4.0/.
References
- Fleming M, Ravula S, Tatishchev SF, et al. Colorectal carcinoma: Pathologic aspects. J Gastrointest Oncol 2012;3:153-73. [PubMed]
- Barresi V, Reggiani Bonetti L, Ieni A, et al. Prognostic significance of grading based on the counting of poorly differentiated clusters in colorectal mucinous adenocarcinoma. Hum Pathol 2015;46:1722-9. [Crossref] [PubMed]
- Barresi V, Reggiani Bonetti L, Ieni A, et al. Histological grading in colorectal cancer: new insights and perspectives. Histol Histopathol 2015;30:1059-67. [PubMed]
- Huang X, Cheng Z, Huang Y, et al. CT-based Radiomics Signature to Discriminate High-grade From Low-grade Colorectal Adenocarcinoma. Acad Radiol 2018;25:1285-97. [Crossref] [PubMed]
- Sauer R, Becker H, Hohenberger W, et al. Preoperative versus postoperative chemoradiotherapy for rectal cancer. N Engl J Med 2004;351:1731-40. [Crossref] [PubMed]
- Sebag-Montefiore D, Stephens RJ, Steele R, et al. Preoperative radiotherapy versus selective postoperative chemoradiotherapy in patients with rectal cancer (MRC CR07 and NCIC-CTG C016): a multicentre, randomised trial. Lancet 2009;373:811-20. [Crossref] [PubMed]
- Canda AE, Terzi C, Gorken IB, et al. Effects of preoperative chemoradiotherapy on anal sphincter functions and quality of life in rectal cancer patients. Int J Colorectal Dis 2010;25:197-204. [Crossref] [PubMed]
- Senore C, Ederle A, Fantin A, et al. Acceptability and side-effects of colonoscopy and sigmoidoscopy in a screening setting. J Med Screen 2011;18:128-34. [Crossref] [PubMed]
- Yang L, Dong D, Fang M, et al. Can CT-based radiomics signature predict KRAS/NRAS/BRAF mutations in colorectal cancer? Eur Radiol 2018;28:2058-67. [Crossref] [PubMed]
- Li M, Zhang L, Tang W, et al. Identification of epidermal growth factor receptor mutations in pulmonary adenocarcinoma using dual-energy spectral computed tomography. Eur Radiol 2019;29:2989-97. [Crossref] [PubMed]
- Yang CB, He TP, Duan HF, et al. Quantitative assessment of the degree of differentiation in colon cancer with dual-energy spectral CT. Abdom Radiol (NY) 2017;42:2591-6. [Crossref] [PubMed]
- Al-Najami I, Lahaye MJ, Beets-Tan RGH, et al. Dual-energy CT can detect malignant lymph nodes in rectal cancer. Eur J Radiol 2017;90:81-8. [Crossref] [PubMed]
- Al-Najami I, Drue HC, Steele R, et al. Dual energy CT - a possible new method to assess regression of rectal cancers after neoadjuvant treatment. J Surg Oncol 2017;116:984-8. [Crossref] [PubMed]
- Liu H, Yan F, Pan Z, et al. Evaluation of dual energy spectral CT in differentiating metastatic from non-metastatic lymph nodes in rectal cancer: Initial experience. Eur J Radiol 2015;84:228-34. [Crossref] [PubMed]
- Wei J, Zhao J, Zhang X, et al. Analysis of dual energy spectral CT and pathological grading of clear cell renal cell carcinoma (ccRCC). PLoS One 2018;13:e0195699 [Crossref] [PubMed]
- Wang D, Huang X. Differential diagnosis of chromophobe renal cell carcinoma and papillary renal cell carcinoma with dual-energy spectral computed tomography. Acta Radiol 2020;61:1562-9. [Crossref] [PubMed]
- Zhang X, Bai L, Wang D, et al. Gastrointestinal stromal tumor risk classification: spectral CT quantitative parameters. Abdom Radiol (NY) 2019;44:2329-36. [Crossref] [PubMed]
- Liu J, Chai Y, Zhou J, et al. Spectral Computed Tomography Imaging of Gastric Schwannoma and Gastric Stromal Tumor. J Comput Assist Tomogr 2017;41:417-21. [Crossref] [PubMed]
- Xu R, Wang J, Huang X, et al. Clinical value of spectral CT imaging combined with AFP in identifying liver cancer and hepatic focal nodular hyperplasia. J buon 2019;24:1429-34. [PubMed]
- Huang YQ, Liang CH, He L, et al. Development and Validation of a Radiomics Nomogram for Preoperative Prediction of Lymph Node Metastasis in Colorectal Cancer. J Clin Oncol 2016;34:2157-64. [Crossref] [PubMed]
- Huang Y, He L, Dong D, et al. Individualized prediction of perineural invasion in colorectal cancer: development and validation of a radiomics prediction model. Chin J Cancer Res 2018;30:40-50. [Crossref] [PubMed]
- Hu T, Wang S, Huang L, et al. A clinical-radiomics nomogram for the preoperative prediction of lung metastasis in colorectal cancer patients with indeterminate pulmonary nodules. Eur Radiol 2019;29:439-49. [Crossref] [PubMed]
- Golia Pernicka JS, Gagniere J, Chakraborty J, et al. Radiomics-based prediction of microsatellite instability in colorectal cancer at initial computed tomography evaluation. Abdom Radiol (NY) 2019;44:3755-63. [Crossref] [PubMed]
- Zhang G, Zhang J, Cao Y, et al. Nomogram based on preoperative CT imaging predicts the EGFR mutation status in lung adenocarcinoma. Transl Oncol 2021;14:100954 [Crossref] [PubMed]
- Amin MB, Greene FL, Edge SB, et al. The Eighth Edition AJCC Cancer Staging Manual: Continuing to build a bridge from a population-based to a more "personalized" approach to cancer staging. CA Cancer J Clin 2017;67:93-9.
- Wright MN, Dankowski T, Ziegler A. Unbiased split variable selection for random survival forests using maximally selected rank statistics. Stat Med 2017;36:1272-84. [Crossref] [PubMed]
- Khan SA, Morris M, Idrees K, et al. Colorectal cancer in the very young: a comparative study of tumor markers, pathology and survival in early onset and adult onset patients. J Pediatr Surg 2016;51:1812-7. [Crossref] [PubMed]
- Mauri G, Sartore-Bianchi A, Russo AG, et al. Early-onset colorectal cancer in young individuals. Mol Oncol 2019;13:109-31. [Crossref] [PubMed]
- Weinberg BA, Marshall JL, Salem ME. The Growing Challenge of Young Adults With Colorectal Cancer. Oncology (Williston Park) 2017;31:381-9. [PubMed]
- Barresi V, Bonetti LR, Ieni A, et al. Histologic grading based on counting poorly differentiated clusters in preoperative biopsy predicts nodal involvement and pTNM stage in colorectal cancer patients. Hum Pathol 2014;45:268-75. [Crossref] [PubMed]
- Ueno H, Kajiwara Y, Shimazaki H, et al. New criteria for histologic grading of colorectal cancer. Am J Surg Pathol 2012;36:193-201. [Crossref] [PubMed]
- Hu S, Huang W, Chen Y, et al. Spectral CT evaluation of interstitial brachytherapy in pancreatic carcinoma xenografts: preliminary animal experience. Eur Radiol 2014;24:2167-73. [Crossref] [PubMed]
- Thaiss WM, Haberland U, Kaufmann S, et al. Iodine concentration as a perfusion surrogate marker in oncology: Further elucidation of the underlying mechanisms using Volume Perfusion CT with 80 kVp. Eur Radiol 2016;26:2929-36. [Crossref] [PubMed]
- Kim SJ, Lee KK, Kim DG. Tumor size predicts the biological behavior and influence of operative modalities in hepatocellular carcinoma. Hepatogastroenterology 2010;57:121-6. [PubMed]
- Gong HX, Zhang KB, Wu LM, et al. Dual Energy Spectral CT Imaging for Colorectal Cancer Grading: A Preliminary Study. PLoS One 2016;11:e0147756 [Crossref] [PubMed]
- Wu J, Lv Y, Wang N, et al. The value of single-source dual-energy CT imaging for discriminating microsatellite instability from microsatellite stability human colorectal cancer. Eur Radiol 2019;29:3782-90. [Crossref] [PubMed]
- Akashi M, Nakahusa Y, Yakabe T, et al. Assessment of aggressiveness of rectal cancer using 3-T MRI: correlation between the apparent diffusion coefficient as a potential imaging biomarker and histologic prognostic factors. Acta Radiol 2014;55:524-31. [Crossref] [PubMed]