Co-amplification of genes in chromosome 8q24: a robust prognostic marker in hepatocellular carcinoma
Introduction
Hepatocellular carcinoma (HCC) ranks among the most prevalent liver malignancies globally, and its morbidity is rising in most areas of the world (1). HCC has an exceedingly poor prognosis, with only 12% of patients with the disease surviving more than 5 years (2-4). Thus, prognostic models are urgently needed to aid in predicting the outcomes of HCC and to inform individualized management decisions for patients. The majority of studies that have attempted to construct a prognostic model for HCC to date have used clinical characteristics and serum tumor markers (5-7). However, with the rapid development of the genome sequencing technologies, evidence has accumulated showing gene signatures and traditional parameters to have immense promise for prognostic prediction in HCC. Li et al., for instance, constructed a 3-gene prediction signature which proved effective in identifying HCC patients with a high mortality risk (8). Also, Bai et al. identified a 6-microRNA signature as an independent factor to predict HCC recurrence (9). Furthermore, a number of other studies constructed messenger RNA (mRNA) expression signatures including different numbers of genes by adopting similar methods (10-13).
Accumulating research suggests that tumors are caused by multiple gene mutations occurring sequentially in cell lines, at chromosomal and nucleotide levels. Chromosomal DNA amplification is among the molecular mechanisms capable of changing genes. The overexpression of mRNA contributes to the occurrence and progression of a variety of tumors (14,15). Amplification of the chromosome 8q24 is frequently detected in human cancer, and several studies have emphasized the significance of this subchromosomal region in the occurrence and progression of cancers, including prostate (16,17), gastrointestinal (18,19), and breast (20) cancer. For example, Cussenot et al. revealed that 8q24 amplification at the Myc locus correlated with Myc protein expression and was associated with disease progression and biochemical recurrence after radical prostatectomy in prostate cancer (16). Besides, the 8q24 chromosome locus is one of the most common amplified genomic regions in HCC (18,21-23).
Although the functional annotation genes in this region have yet to be fully expounded, several studies have reported the amplification of some genes separately located on the 8q24 chromosome region, such as MYC (24), MAL2 (22), and BOP1 (4), which have been linked to HCC occurrence and progression in recent years. Through informatics analysis of genomic data from 150 HCC tumors, we further confirmed that an increase of 8q24 is the major contributing event in the occurrence and development of HCC (25). Zeng et al. has been genotyped the rs9642880 G >T polymorphism using DNA isolated from blood samples of 271 hepatocellular carcinoma (HCC) patients who received radiotherapy treatment (21). The results showed that patients who carried the GT or TT genotypes had significantly shorter median survival times compared to patients with the GG genotype. Recently Zhang et al. has been reported that Copy number gains and amplification of chromosome 8q24.3 in HCC were determined to be positively correlated with the PRL-3 overexpression, which provided an example of how co-amplified genes work together in HCC (26). In our previous study, we also retrospectively analyzed the genetic testing and clinical follow-up information of 80 patients with HCC. Our results indicated that a gain of 8q24 was significantly associated with poor survival of HCC (P<0.05), with an alteration frequency of 22–30%.
In recent years, the construction of an effective and powerful predictive model which can provide valuable information to inform clinical medical decisions in a convenient way has drawn much attention. In the present study, we focused on copy number variation of genes located on the chromosomal 8q24 region to build a prognostic prediction model for HCC. The mRNA expression data of HCC from The Cancer Genome Atlas (TGCA) were analyzed to identify differentially expressed genes (DEGs). Lasso-Cox regression analysis was performed to build a 7-gene signature, which we validated in an International Cancer Genome Consortium (ICGC) cohort. A nomogram combining the 7-gene prognostic signature and clinical prognostic factors to predict survival was finally constructed. Gene Set Enrichment Analysis (GSEA) was applied for further analysis of the mechanisms potentially underlying the predictive effect of our model. We present the following article in accordance with the REMARK reporting checklist (available at http://dx.doi.org/10.21037/jgo-21-205).
Methods
Data collection
We collected mRNA expression and clinical data from the TCGA-Liver Hepatocellular Carcinoma dataset (TCGA-LIHC, https://portal.gdc.cancer.gov/), cBioportal for Cancer Genomics, and International Cancer Genome Consortium (ICGC, http://cbioportal.org/) (27). The study was conducted in accordance with the Declaration of Helsinki (as revised in 2013). All data were obtained from publicly available, open-access databases; All data can be found here: https://portal.gdc.cancer.gov/repository, https://dcc.icgc.org/projects/LIRI-JP. thus, additional ethical approval was not required.
Identifying and intersecting differentially expressed mRNAs (DEM) in the TCGA-LIHC dataset
The transcripts per million (TPM) method was applied to standardize raw counts of HCC mRNA expression data, which were subjected to log2 conversion. A total of 167 protein-coding genes located in the chromosome 8q24 region were accurately annotated. The R package limma (version 3.36.2) was used to identify the DEMs. The DEMs which had an absolute log2 fold change (FC) >1 and an adjusted P value <0.05 were included in subsequent analyses (https://cancerci.biomedcenral.com/).
Construction of the prognostic gene signature
The prognostic gene signature model was established in a discovery cohort comprising 331 patients from TCGA. For the analysis, we only selected patients with initial treatment whose overall survival (OS) exceeded 1 month. Patients with amplification of ≥2 genes located in the chromosome 8q24 region were assigned to the amplification group (mutation group), while those with amplification of 0–1 gene were included in the non-amplification group (wild-type group). DEGs were identified by univariate Cox regression analysis. Genes for predicting OS of HCC were further screened by Lasso-penalized Cox regression analysis designed using the R package glmnet (28). Finally, a 7-gene signature prognosis model was constructed in the TCGA discovery cohort on the basis of a linear combination of the regression coefficient of the Lasso-Cox regression model coefficients (β) and the mRNA expression level. The prognostic gene signature was presented as risk score = (βmRNA1*expression level of mRNA1) + (βmRNA2*expression level of mRNA2) +…+ (βmRNAn*expression level of mRNAn). For verification of the prediction efficiency of the predictive gene signature, patients form TCGA cohort were randomly separated into training and testing datasets at a ratio of 6:4 using the createDataPartition function in the R package caret. The optimal cutoff values were investigated using the survminer R package. Subsequently, we divided patients into groups with high and low risk based on the correlation of the signature gene expression levels with OS in the training dataset. With the survival R package, we analyzed the survival difference between patients with high and low risk scores by combining Kaplan–Meier (KM) survival curve analysis with the log-rank test. We used time-dependent receiver operating characteristic (ROC) curve analysis and the concordance index (C-index) to assess the accuracy of the prognostic signature in predicting 1-, 3-, and 5-year survival. The prognostic gene signature’s estimated value was further verified in the testing dataset and TCGA discovery cohort. Using the Wilcoxon signed-rank test, we also performed an analysis in the TCGA discovery cohort to compare the mRNA expression levels of the 7 prognosis-related genes included in the signature in the high- and low-risk groups.
External validation of the prognostic gene signature
To validate the prognostic gene signature, we used another cohort comprising 243 patients with HCC from the ICGC. Patients’ risk scores were calculated according to the prognostic gene characteristics. ROC curve and KM curves were drawn to validate the value of the prognostic gene signature for predicting HCC prognosis.
Independence of the prognostic gene signature from other clinical parameters in the TCGA
To determine whether the prediction efficiency of the prognostic model was able to serve independently of other clinical parameters [such as age, sex, alpha-fetoprotein (AFP), body mass index (BMI), tumor grade, vascular tumor invasion (MVI) inflammation, residual tumor, tumor mutation burden (TMB), and tumor stage], we performed univariate and multivariate analyses using a Cox regression model. P value <0.05 indicated statistical significance. We also calculated the hazard ratios (HRs) and 95% confidence intervals (CIs).
Building and validation of the predictive nomogram
Nomograms are commonly applied to predict the prognosis of cancer. All independent prognostic factors were selected for the evaluation of the probability of OS at 1, 3, and 5 years using the multivariable Cox regression method. We validated the nomogram by assessing its discrimination and calibration. To test the nomogram’s predicted probability of OS against the observed rates, we plotted its calibration curve. To examine whether the nomogram was reliable in comparison with tumor stage or risk group, decision curve analysis (DCA) was carried out.
GSEA
To reveal the potential molecular mechanism of the prognostic gene signature, GSEA (v.3.0.) was used to investigate patients in high- and low-risk subgroups in the TCGA discovery cohort.
Pathways related to OS in the 2 risk groups were separately retrieved by searching Molecular Signatures Database v.7.0. We identified gene sets with a P value <0.05 and a false discovery rate (FDR) <25% as having significant enrichment.
Statistical analysis
Data were statistically analyzed with R software v3.6.0 (R Foundation for Statistical Computing, Vienna, Austria). P value <0.05 indicated statistical significance.
Results
Identified DEMs
Figure 1 shows a flow chart of the analytical process. In total, 109 genes were screened out as DEMs between the 8q24 amplification group (n=184) and the non-amplification group (n=109; Table S1).
Construction and validation of the prognostic gene signature
At random, we assigned 184 patients with primary HCC in the TCGA discovery cohort at a ratio of 6:4 to a training dataset (n=111) and a testing dataset (n=73). Table 1 summarizes the baseline characteristics of patients in the 2 datasets. None of the clinical characteristics were significantly different between the testing dataset and training dataset.
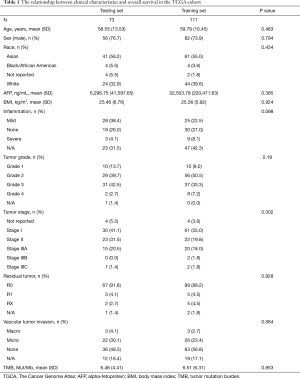
Full table
We further narrowed candidate mRNAs using Lasso-penalized Cox analysis, finally identifying 7 target genes, which were selected to build the prognostic gene signature. These genes included zinc finger protein 7 (ZNF7), trafficking protein particle complex 9 (TRAPPC9), transmembrane protein 65 (TMEM65), POU domain class 5 transcription factor 1B (POU5F1B), DEP domain-containing MTOR interacting protein (DEPTOR), BOP1 ribosomal biogenesis factor (BOP1), and Rho GTPase-activating protein 39 (ARHGAP39), which were found to have a prognostic connection HCC using the regsubsets function of the leaps R package. The risk score= (−1.465*ExprZNF7) + (−1.317*ExprTRAPPC9) + (0.432*ExprTMEM65) + (−0.728*ExprPOU5F1B) + (−0.253*ExprDEPTOR) + (0.357*ExprBOP1) + (0.553*ExprARHGAP39).
The optimal risk-score cutoff value was finally determined to be 2.074. According to this cutoff, patients in the training dataset were dissected into groups with high (n=44) and low (n=169) risk scores. Subsequently, all stratification was based on this optimal cutoff value. Time-dependent ROC and KM survival curves were calculated to evaluate the ability of our 7-gene signature to predict HCC prognosis. The same procedure was employed for both the testing and the entire TCGA datasets. The area under the ROC curve (AUC) values of the signature for OS at 1, 3, and 5 years were: 0.77, 0.741, and 0.784, respectively, in the training dataset; 0.7, 0.636, and 0.633, respectively, in the testing dataset; and 0.739, 0.704, and 0.725, respectively, in the whole TCGA cohort (Figure 2). In all 3 datasets, the OS was significantly poorer in the high-risk group than in the low-risk group (P<0.001, P=0.002, and P<0.001 in the training, testing, and whole TCGA datasets; Figure 3). This result indicated a moderately sufficient specificity of the 7-gene signature for prognostic prediction in HCC. Among the 368 HCC patients in the TCGA cohort, 64 (18%) had at least 1 genetic variation of the 7 selected genes, with amplification being the most common type of genetic variation. In TCGA cohort, the mRNA expression levels of BOP1, TMEM65, and ARHGAP39 were significantly upregulated compared with those in the high-risk group; in contrast, those of TRAPPC9, POU5F1B and DEPTOR were significantly downregulated (Figure 4).
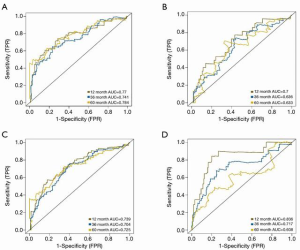
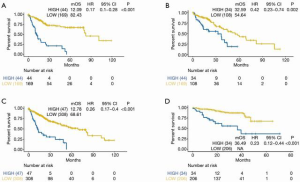
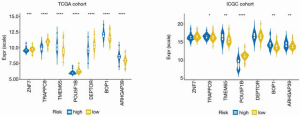
Externally validating the prognostic gene signature in the ICGC cohort
For further assessment of its prediction ability, we tested the 7-gene signature in the ICGC cohort. A total of 240 patients with HCC were classified as high (n=34) or low (n=206) risk using the optimal risk cutoff value described above. In accordance with our findings from the ICGC cohort, patients with a high-risk score had significantly worse OS than patients with a low-risk score (P<0.001, Figure 2D). The AUC for OS at 1, 3, and 5 years was 0.836, 0.717, and 0.608, respectively (Figure 3D).
Independent prognostic role of the gene signature
Next, we conducted Cox univariate and multivariate regression analyses in the TCGA cohort to assess risk group (classified by the 7-gene signature) and clinicopathological variables [including age, sex, AFP, BMI, inflammation, vascular tumor invasion, residual tumor, tumor grade, tumor stage, and tumor mutational burden (TMB)] as independent prognostic predictors. Risk group and tumor stage were independent prognostic factors in the TCGA cohort (risk group, HR: 0.32; 95% CI: 0.2–0.52; P<0.001; tumor stage, HR: 2.13; 95% CI: 1.4–3.19; P<0.001). Details are provided in Table 2.
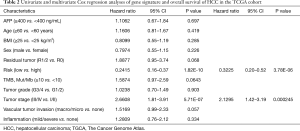
Full table
Building and validating a predictive nomogram
For the purpose of constructing a clinically applicable survival prediction model for patients with HCC, a nomogram was built based on tumor stage and risk score (Figure 5A) (29). Calibration plots showed that the nomogram’s predictive ability was better for short-term (1 and 3 years) than long-term (5 years) survival, which was consistent with the predicted and actual survival rates (Figure 5B). DCA demonstrated that the combined predictive model showed the best net benefit for 1-year and 3 year but not for 5 year as well (Figure 5C).
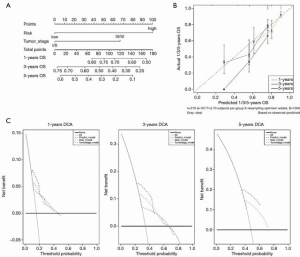
GSEA
With the aim of uncovering the potential molecular-level mechanism of our 7-gene signature, we performed GSEA analysis in the TCGA cohort. As demonstrated in Table 3 and Figure 6, the tumors of patients classified as high risk using the 7-gene signature were significantly enriched in the G2M checkpoint (P<0.001, FDR =0.005), DNA repair (P=0.010, FDR =0.048), MYC targets (P=0.002, FDR =0.006), and mTORC1 signaling (P=0.006, FDR =0.028) pathways or terms. Furthermore, pathways with significant enrichment in patients with low risk were associated with several metabolic processes (including xenobiotic metabolism, bile acid, and fatty acid), adipogenesis, coagulation, and peroxisome function.
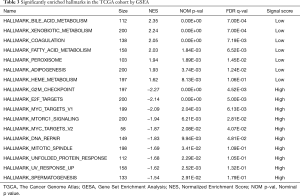
Full table
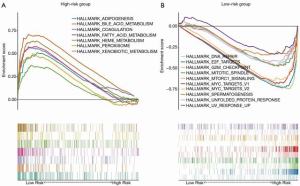
Discussion
Because of the complex molecular mechanisms and cellular heterogeneity of HCC, morbidity and death associated with this disease are continuing to rise in multiple countries (11,25). The discovery of new prognostic biomarkers and the development of prognostic models with higher accuracy are urgently required to improve the clinical dilemma posed by HCC. Compared with a single biomarker, a genetic prognostic prediction model constructed with traditional clinical parameters may offer superior prediction efficiency. In this study, we focused on the amplification of genes located in the 8q24 chromosomal region. Using a TCGA dataset, we successfully established a novel 7-gene signature, which was subjected to validation in ICGC dataset. We found that the risk score based on the signature was an independent factor of HCC prognosis, with patients with a high-risk score having a lower survival rate than patients with a low-risk score. ROC curve analysis and DCA results revealed that the nomogram combining the 7-gene signature with tumor stage showed the best predictive efficacy for short-term survival (1 and 3 years) comparing with the nomograms build with a single prognostic factor, but not for long-term survival (5 years). Altogether, the results demonstrated that our 7-gene signature could be a useful and promising indicator to predict the survival of patients with HCC. Among the 7 genes in our signature, 3 of them have been linked to HCC. Block of proliferation 1 (BOP1) is a WD40 protein, the isolation of which was initially performed through complementary DNA library screening for growth-related sequences in fibroblasts from mouse embryos. BOP1 promotes HCC cell migration and invasion by inducing epithelial–mesenchymal transition (EMT), which is a vital regulatory process, the activation of which occurs during tumor invasion and metastasis (4). BOP1 also stimulates actin stress fiber assembly and RhoA activation, and makes a vital contribution to the enhancement of cell contractility via actin rearrangement and stress fiber formation (30-32). Recently, DEP-domain containing mammalian target of rapamicin (mTOR)-interacting protein (DEPTOR), also known as DEP-domain containing protein 6 (DEPDC6), has emerged as a research hotspot, owing to the discovery that it negatively regulates mTOR. The main components of the protein are mTORC1 and mTORC2 complexes, which inhibit its kinase activity by interacting with mTOR (33,34). The deregulation of mTOR has commonly been linked to tumor pathogenesis. Consistent with inhibitory mTOR activity, the expression of DEPTOR is low in the majority of tumors, although there are some exceptions, including multiple myeloma (MM), thyroid carcinoma, and lung cancer (35,36). DEPTOR promotes EMT and HCC cell metastasis through activation of the TGF-β1-SMAD3/SMAD4-Snail pathway by inhibiting mTOR (37). In embryonic stem cells, OCT4 (also called POU5F1) is an essential participant in the processes of self-renewal, development, and somatic cell reprogramming to pluripotent stem cells. OCT4 has 6 pseudogenes, which are transcribed in many tumors (38,39). Among these OCT4 pseudogenes, POU domain class5 transport factor 1B (POU5F1B) encodes 95% homology to OCT4A. The survival of HCC patients with a high expression POU5F1B is shorter than that of patients with a low expression, with the former group also having a higher level of AKT phosphorylation. POU5F1B was found to promote hepatoma cell proliferation via its activation of AKT; thus, inhibiting AKT in POU5F1B-overexpressing hepatoma cells can in turn inhibit cell proliferation. AKT phosphorylation by TSC2 can also take place at Ser473 sites, which activates Rheb’s stimulation of mTORC1 activity, increasing protein synthesis via phosphorylation of eukaryotic translation initiation factor 4 E (eIF4E) and ribosomal proteins S6 (40).
The roles of Arhgap39, TRAPPC9, ZNF7, and TMEM65 in HCC have not been reported. Rho GTPases are widely known to be participants in cell growth, cell dynamics, apoptosis, and intracellular membrane trafficking. Arhgap39 [also referred to as preoptic regulatory factor-2 (Porf-2) or Vilse] is a multidomain protein containing WW, myosin tail homolog 4 (MyTH4), and RhoGAP domains, which can inactivate Rho GTPases (41). Studies have shown that Arhgap39 regulates Rac/cdc42-dependent cytoskeleton and neurogenesis in hippocampal neurons of Drosophila and mice through WW domains (42). Transport protein particle complex 9 (TRAPPC9), a protein subunit of transport protein particle II (TRAPPII), has been illustrated to play a vital role in the transport of cargo from the endoplasmic reticulum to the Golgi body and endosome-to-Golgi trafficking in yeast cells (43). Besides, studies have indicated that TRAPPC9 mutations are associated with an autosomal recessive disorder characterized by mental retardation, autism in mothers, neonatal microcephaly, hearing loss, and stroke (44,45). A20 (Tumor necrosis factor alpha-induced protein 3, TNFAIP3) is a deubiquitinating enzyme with significant anti-inflammatory functions. Genome-wide studies have illustrated that A20 is a susceptibility gene in a variety of human inflammatory and autoimmune diseases, such as systemic lupus erythematosus, psoriasis, rheumatoid arthritis, and Crohn’s disease (46,47). The binding ability of A20’s ZnF domains 4 and 7 affects its ability to bind to ubiquitin chains. Notably, ZNF7 in A20 is involved in the regulation of the TNF receptor 1 (TNFR1) signaling pathway, and specifically binds to the linear ubiquitin chain (48,49) and inhibits TNFR1-mediated apoptosis and death (50). ZnF7 can inhibit NF-κB (nuclear factor kappa-light-chain-enhancer of activated B cells), the constitutive activation of which is associated with tumorigenesis. Therefore, it can be speculated that the dysregulation of NF-κB activation leads to A20 mutation or deletion, which is the main cause of B-cell lymphoma (51). Transmembrane protein 65 (TMEM65) is an endometrial protein that plays an important role in the function of the mitochondrial respiratory chain (52,53). The biological function of TMEM65 protein has yet to be completely illuminated. One case of a mutation in the TMEM65 gene has been reported in a patient with mitochondrial encephalomyopathy, suggesting that TMEM65 functional changes in mitochondrial function can be caused by TMEM65 gene mutations, resulting in severe cellular and clinical consequences. Besides, the low expression of TMEM65 in skin fibroblasts has been reported to affect the content and respiration rate of mitochondria (54).
In recent years, many studies have constructed effective multi-gene prognostic signature models for HCC. However, a gene signature prognostic model of HCC based on copy number amplification of genes has not been reported previously. Gains of 8q, as the most common changing, have been reported in 27.7% of cancers arising in 27 different tissues and in 31% to 66% of HCCs (23). Amplification of DNA in the 8q24 chromosome region is thought to be associated with progression of multiple cancers including HCC, which indicates that the 8q region harbors 1 or more targeted genes whose amplification promotes their oncogenicity. Our study focused on the type of copy number variant of genes located in the 8q24 chromosome region, and we constructed a predictive signature based on the expression levels of 7 genes. The nomogram combined the 7-gene signature and clinical parameters to determine patient prognosis. The 7 genes may be potential molecular targets to fight HCC and include 4 genes for which a correlation with HCC has not previously been documented, which may be potential research interests or molecular therapeutic targets.
However, some limitations of our study need to be pointed out. Firstly, the mRNA expression data and clinical data were obtained from the TCGA and ICGC databases, in which Europeans and Americans account for the majority of patients. Secondly, our gene signature prognostic model gave a better performance in predicting short-term (1 and 3 years) than long-term (5 years) survival. Thirdly, further investigation and experimentation are needed to explore the underlying functional mechanisms of the 7 predictive signature genes in HCC development and progression.
Conclusions
In summary, our study selected the mRNA expression data of 8q24 genes of HCC patients and focused on the copy number variations of mutation types. A 7-gene signature was successfully constructed to predict the prognosis of HCC. Our prognostic nomogram, which combines the 7-gene signature with clinical factors to predict HCC prognosis, could serve as a reliable tool to identify high-risk HCC patients with poor survival and aid in the development of precise individualized therapy.
Acknowledgments
We thank the patients and investigators who participated in the TCGA, GEO and ICGC for providing data.
Funding: None.
Footnote
Reporting Checklist: The authors have completed the REMARK reporting checklist. Available at http://dx.doi.org/10.21037/jgo-21-205
Conflicts of Interest: All authors have completed the ICMJE uniform disclosure form (available at http://dx.doi.org/10.21037/jgo-21-205). Author Tingting Chen, Wenzhuan Xie, Mengli Huang, and Yuezong Bai were employed by the company 3D Medicines Inc. The other authors have no conflicts of interest to declare.
Ethical Statement: The authors are accountable for all aspects of the work in ensuring that questions related to the accuracy or integrity of any part of the work are appropriately investigated and resolved. The study was conducted in accordance with the Declaration of Helsinki (as revised in 2013). All data were obtained from publicly available, open-access databases; thus, additional ethical approval was not required.
Open Access Statement: This is an Open Access article distributed in accordance with the Creative Commons Attribution-NonCommercial-NoDerivs 4.0 International License (CC BY-NC-ND 4.0), which permits the non-commercial replication and distribution of the article with the strict proviso that no changes or edits are made and the original work is properly cited (including links to both the formal publication through the relevant DOI and the license). See: https://creativecommons.org/licenses/by-nc-nd/4.0/.
References
- Katoh H, Shibata T, Kokubu A, et al. Genetic profile of hepatocellular carcinoma revealed by array-based comparative genomic hybridization: identification of genetic indicators to predict patient outcome. J Hepatol 2005;43:863-74. [Crossref] [PubMed]
- Llovet JM, Montal R, Sia D, et al. Molecular therapies and precision medicine for hepatocellular carcinoma. Nat Rev Clin Oncol 2018;15:599-616. [Crossref] [PubMed]
- Villanueva A. Hepatocellular Carcinoma. N Engl J Med 2019;380:1450-62. [Crossref] [PubMed]
- Chung KY, Cheng IK, Ching AK, et al. Block of proliferation 1 (BOP1) plays an oncogenic role in hepatocellular carcinoma by promoting epithelial-to-mesenchymal transition. Hepatology 2011;54:307-18. [Crossref] [PubMed]
- Budhu A, Forgues M, Ye QH, et al. Prediction of venous metastases, recurrence, and prognosis in hepatocellular carcinoma based on a unique immune response signature of the liver microenvironment. Cancer Cell 2006;10:99-111. [Crossref] [PubMed]
- He YZ, He K, Huang RQ, et al. Preoperative evaluation and prediction of clinical scores for hepatocellular carcinoma microvascular invasion: a single-center retrospective analysis. Ann Hepatol 2020;19:654-61. [Crossref] [PubMed]
- Yoo J, Lee MW, Lee DH, et al. Evaluation of a serum tumour marker-based recurrence prediction model after radiofrequency ablation for hepatocellular carcinoma. Liver Int 2020;40:1189-200. [Crossref] [PubMed]
- Li B, Feng W, Luo O, et al. Development and Validation of a Three-gene Prognostic Signature for Patients with Hepatocellular Carcinoma. Sci Rep 2017;7:5517. [Crossref] [PubMed]
- Bai F, Zhou H, Ma M, et al. A novel RNA sequencing-based miRNA signature predicts with recurrence and outcome of hepatocellular carcinoma. Mol Oncol 2018;12:1125-37. [Crossref] [PubMed]
- Chen PF, Li QH, Zeng LR, et al. A 4-gene prognostic signature predicting survival in hepatocellular carcinoma. J Cell Biochem 2019;120:9117-24. [Crossref] [PubMed]
- Ke K, Chen G, Cai Z, et al. Evaluation and prediction of hepatocellular carcinoma prognosis based on molecular classification. Cancer Manag Res 2018;10:5291-302. [Crossref] [PubMed]
- Qiao GJ, Chen L, Wu JC, et al. Identification of an eight-gene signature for survival prediction for patients with hepatocellular carcinoma based on integrated bioinformatics analysis. PeerJ 2019;7:e6548 [Crossref] [PubMed]
- Wang Z, Teng D, Li Y, et al. A six-gene-based prognostic signature for hepatocellular carcinoma overall survival prediction. Life Sci 2018;203:83-91. [Crossref] [PubMed]
- Brisbin AG, Asmann YW, Song H, et al. Meta-analysis of 8q24 for seven cancers reveals a locus between NOV and ENPP2 associated with cancer development. BMC Med Genet 2011;12:156. [Crossref] [PubMed]
- Wang XC, Wu YP, Ye B, et al. Suppression of anoikis by SKP2 amplification and overexpression promotes metastasis of esophageal squamous cell carcinoma. Mol Cancer Res 2009;7:12-22. [Crossref] [PubMed]
- Fromont G, Godet J, Peyret A, et al. 8q24 amplification is associated with Myc expression and prostate cancer progression and is an independent predictor of recurrence after radical prostatectomy. Hum Pathol 2013;44:1617-23. [Crossref] [PubMed]
- Ciampa J, Yeager M, Amundadottir L, et al. Large-scale exploration of gene-gene interactions in prostate cancer using a multistage genome-wide association study. Cancer Res 2011;71:3287-95. [Crossref] [PubMed]
- White NM, Maher CA. The potential use of lncRNAs found in the 8q24 region as biomarkers for colon cancer. Ann Oncol 2017;28:1688-9. [Crossref] [PubMed]
- Wang X, Liu Y, Shao D, et al. Recurrent amplification of MYC and TNFRSF11B in 8q24 is associated with poor survival in patients with gastric cancer. Gastric Cancer 2016;19:116-27. [Crossref] [PubMed]
- Meng F, Liu B, Xie G, et al. Amplification and overexpression of PSCA at 8q24 in invasive micropapillary carcinoma of breast. Breast Cancer Res Treat 2017;166:383-92. [Crossref] [PubMed]
- Zhao XM, Xiang ZL, Chen YX, et al. A sequence polymorphism on 8q24 is associated with survival in hepatocellular carcinoma patients who received radiation therapy. Sci Rep 2018;8:2264. [Crossref] [PubMed]
- Lopez-Coral A, Del Vecchio GJ, Chahine JJ, et al. MAL2-Induced Actin-Based Protrusion Formation is Anti-Oncogenic in Hepatocellular Carcinoma. Cancers (Basel) 2020;12:422. [Crossref] [PubMed]
- Okamoto H, Yasui K, Zhao C, et al. PTK2 and EIF3S3 genes may be amplification targets at 8q23-q24 and are associated with large hepatocellular carcinomas. Hepatology 2003;38:1242-9. [Crossref] [PubMed]
- Wu M, Zhou Y, Fei C, et al. ID1 overexpression promotes HCC progression by amplifying the AURKA/Myc signaling pathway. Int J Oncol 2020;57:845-57. [Crossref] [PubMed]
- Poon TC, Wong N, Lai PB, et al. A tumor progression model for hepatocellular carcinoma: bioinformatic analysis of genomic data. Gastroenterology 2006;131:1262-70. [Crossref] [PubMed]
- Zhou Q, Zhou Q, Liu Q, et al. PRL-3 facilitates Hepatocellular Carcinoma progression by co-amplifying with and activating FAK. Theranostics 2020;10:10345-59. [Crossref] [PubMed]
- Liu GM, Xie WX, Zhang CY, et al. Identification of a four-gene metabolic signature predicting overall survival for hepatocellular carcinoma. J Cell Physiol 2020;235:1624-36. [Crossref] [PubMed]
- Friedman J, Hastie T, Tibshirani R. Regularization Paths for Generalized Linear Models via Coordinate Descent. J Stat Softw 2010;33:1-22. [Crossref] [PubMed]
- Long J, Zhang L, Wan X, et al. A four-gene-based prognostic model predicts overall survival in patients with hepatocellular carcinoma. J Cell Mol Med 2018;22:5928-5938. [Crossref] [PubMed]
- Narumiya S, Tanji M, Ishizaki T. Rho signaling, ROCK and mDia1, in transformation, metastasis and invasion. Cancer Metastasis Rev 2009;28:65-76. [Crossref] [PubMed]
- Hall A. The cytoskeleton and cancer. Cancer Metastasis Rev 2009;28:5-14. [Crossref] [PubMed]
- Etienne-Manneville S, Hall A. Rho GTPases in cell biology. Nature 2002;420:629-35. [Crossref] [PubMed]
- Peterson TR, Laplante M, Thoreen CC, et al. DEPTOR is an mTOR inhibitor frequently overexpressed in multiple myeloma cells and required for their survival. Cell 2009;137:873-86. [Crossref] [PubMed]
- Caron A, Briscoe DM, Richard D, et al. DEPTOR at the Nexus of Cancer, Metabolism, and Immunity. Physiol Rev 2018;98:1765-803. [Crossref] [PubMed]
- Catena V, Fanciulli M. Deptor: not only a mTOR inhibitor. J Exp Clin Cancer Res 2017;36:12. [Crossref] [PubMed]
- Yao H, Tang H, Zhang Y, et al. DEPTOR inhibits cell proliferation and confers sensitivity to dopamine agonist in pituitary adenoma. Cancer Lett 2019;459:135-44. [Crossref] [PubMed]
- Chen J, Zhu H, Liu Q, et al. DEPTOR induces a partial epithelial-to-mesenchymal transition and metastasis via autocrine TGFbeta1 signaling and is associated with poor prognosis in hepatocellular carcinoma. J Exp Clin Cancer Res 2019;38:273. [Crossref] [PubMed]
- Hayashi H, Arao T, Togashi Y, et al. The OCT4 pseudogene POU5F1B is amplified and promotes an aggressive phenotype in gastric cancer. Oncogene 2015;34:199-208. [Crossref] [PubMed]
- Yi J, Zhou LY, Yi YY, et al. Low Expression of Pseudogene POU5F1B Affects Diagnosis and Prognosis in Acute Myeloid Leukemia (AML). Med Sci Monit 2019;25:4952-9. [Crossref] [PubMed]
- Pan Y, Zhan L, Chen L, et al. POU5F1B promotes hepatocellular carcinoma proliferation by activating AKT. Biomed Pharmacother 2018;100:374-80. [Crossref] [PubMed]
- Nowak FV. Porf-2 = Arhgap39 = Vilse: A Pivotal Role in Neurodevelopment, Learning and Memory. eNeuro 2018;5:ENEURO.0082-18.2018.
- Lee JY, Lee LJ, Fan CC, et al. Important roles of Vilse in dendritic architecture and synaptic plasticity. Sci Rep 2017;7:45646. [Crossref] [PubMed]
- Mbimba T, Hussein NJ, Najeed A, et al. TRAPPC9: Novel insights into its trafficking and signaling pathways in health and disease Int J Mol Med 2018;42:2991-7. (Review). [Crossref] [PubMed]
- Dookeran KA, Zhang W, Stayner L, et al. Associations of two-pore domain potassium channels and triple negative breast cancer subtype in The Cancer Genome Atlas: systematic evaluation of gene expression and methylation. BMC Res Notes 2017;10:475. [Crossref] [PubMed]
- Mohamoud HS, Ahmed S, Jelani M, et al. A missense mutation in TRAPPC6A leads to build-up of the protein, in patients with a neurodevelopmental syndrome and dysmorphic features. Sci Rep 2018;8:2053. [Crossref] [PubMed]
- Skaug B, Chen J, Du F, et al. Direct, noncatalytic mechanism of IKK inhibition by A20. Mol Cell 2011;44:559-71. [Crossref] [PubMed]
- Martens A, Priem D, Hoste E, et al. Two distinct ubiquitin-binding motifs in A20 mediate its anti-inflammatory and cell-protective activities. Nat Immunol 2020;21:381-7. [Crossref] [PubMed]
- Wertz IE, Newton K, Seshasayee D, et al. Phosphorylation and linear ubiquitin direct A20 inhibition of inflammation. Nature 2015;528:370-5. [Crossref] [PubMed]
- Draber P, Kupka S, Reichert M, et al. LUBAC-Recruited CYLD and A20 Regulate Gene Activation and Cell Death by Exerting Opposing Effects on Linear Ubiquitin in Signaling Complexes. Cell Rep 2015;13:2258-72. [Crossref] [PubMed]
- Yamaguchi N, Yamaguchi N. The seventh zinc finger motif of A20 is required for the suppression of TNF-alpha-induced apoptosis. FEBS Lett 2015;589:1369-75. [Crossref] [PubMed]
- Karin M. Nuclear factor-kappaB in cancer development and progression. Nature 2006;441:431-6. [Crossref] [PubMed]
- Nishimura N, Gotoh T, Oike Y, et al. TMEM65 is a mitochondrial inner-membrane protein. PeerJ 2014;2:e349 [Crossref] [PubMed]
- Sharma P, Abbasi C, Lazic S, et al. Evolutionarily conserved intercalated disc protein Tmem65 regulates cardiac conduction and connexin 43 function. Nat Commun 2015;6:8391. [Crossref] [PubMed]
- Nazli A, Safdar A, Saleem A, et al. A mutation in the TMEM65 gene results in mitochondrial myopathy with severe neurological manifestations. Eur J Hum Genet 2017;25:744-51. [Crossref] [PubMed]
(English Language Editor: J. Reynolds)