Correlation analysis of tumor mutation burden of hepatocellular carcinoma based on data mining
Introduction
Hepatocellular carcinoma (HCC) is one of the main malignant digestive tract tumors that is harmful to human health. According to the global report of malignant tumors, the incidence of HCC ranks sixth in the malignant tumor incidence spectrum, and the mortality ranks third in the malignant tumor mortality spectrum. HCC incurs a heavy disease burden and has become a serious global health problem (1,2). Viral hepatitis infection, type 2 diabetes, obesity, and aflatoxin are the major risk factors of HCC (3). Depending on the staging of HCC, hepatectomy is the main treatment for patients with early and resectable liver cancer, while transcatheter arterial chemoembolization, liver transplantation, ablation, and drug therapy are the main treatments for patients with unresectable, and recurrent liver cancer (4,5). The liver has unique immune characteristics, such as high-density immune cell infiltration, strong immunogenicity and a variety of immune microenvironments (6). With the rise of immunotherapy and the development of large-scale clinical trials, a greater evidence base for the immunotherapy of HCC has been constructed (7-9). Tumor mutation burden (TMB) refers to the number of point mutations, insertion deletions, and other gene mutations in the coding region of somatic proteins contained in the average 1 Mb base range of the tumor genome. TMB is an independent predictor of the treatment outcome of a newly established immune checkpoint inhibitor (ICPI), which can predict the sensitivity of tumor tissue to ICPs. It is also one of the biomarkers for predicting the efficacy of programmed cell death protein-1 (PD-1) inhibitors (10,11). The occurrence and development of HCC is a complex formation process, which involves a higher TMB than other solid tumors (12). TMB has been used in the field of non-small cell lung cancer (13); however, at present, the role of TMB and immune infiltration in HCC is not clear.
Immunotherapy involves enhancing the low immune function of the body to ultimately kill tumor cells through a variety of methods (14). ICIs are a kind of immunotherapy developed rapidly in recent years, with PD-1 representing one of the major research hotspots. The results of a clinical trial showed that the overall objective remission rate of anti-PD-1 monoclonal antibody in the treatment of advanced HCC was 20%, and the disease control rate was 64% (15). The results of several clinical trials of different tumor types show that compared with patients with lower TMB, patients with higher TMB have higher remission rate and longer survival period, and TMB is positively correlated with the effect of immunotherapy (11). The tumor microenvironment is mainly composed of extracellular matrix, blood vessels, and other tissues around the tumor, including stromal cells, fibroblasts, and immune cells (16-19). A variety of immune cells are involved in the development of tumors and have a high degree of heterogeneity. Studies by Kim et al. have shown that HCC contains different levels of PD-1 cluster of differentiation 8 (CD8)+ T cells, and that patients with higher levels of PD-1 and high CD8+ T cells are more sensitive to combined therapy with immune checkpoint blockade (20).
The liver itself has a unique immune microenvironment, including a variety of immune cells such as dendritic cells, natural killer cells, and cytotoxic T lymphocytes, in addition to nonimmune cells and cellular products, such as cancer-associated fibroblasts and liver endothelial cells. These cells can regulate the immune response and tolerance of liver tumors, and can affect the development and prognosis of patients (21). The response rate of antitumor-associated antigen CD8+ T cells in patients with early HCC has been found to be higher than that in patients with advanced HCC (22). The results show that the infiltration of macrophages in tumor microenvironment can promote tumor growth, invasion, and metastasis, and that the increase of macrophages is related to the poor prognosis of liver cancer. Targeted macrophages are expected to become part of adjuvant immunotherapy for patients with liver cancer (23-25). At present, however, there are few studies that have examined the relationship between HCC and immune infiltrating cells, or the relationship between recurrence and TMB.
Currently, many HCC prognostic models based on different gene types have been established, but there is no prognostic model based on TMB gene to systematically evaluate the TMB condition of HCC and predict the prognosis of HCC patients. Therefore, the purpose of our study was to explore the correlation between TMB and prognosis of patients with HCC, as well as the differential expression of HCC genes by TMB. At the same time, the correlation and prognostic relationship between infiltrating immune cells and HCC in tumor tissue were investigated.
We present the study in accordance with the REMARK reporting checklist (available at http://dx.doi.org/10.21037/jgo-21-259).
Methods
The study was conducted in accordance with the Declaration of Helsinki (as revised in 2013).
Data set
We downloaded the gene expression microarray containing HCC tissue and normal control tissue or paracancerous normal tissue from The Cancer Genome Atlas (TCGA, https://cancergenome.nih.gov) and the Gene Expression Omnibus (GEO; https://www.ncbi.nlm.nih.gov/geo), and obtained RNA-sequencing (RNA-seq) transcriptome data, tumor mutation simple-nucleotide variant (SNV) data, and related clinical data of primary HCC patients. Using VarScan software, we counted the base mutation number, calculated the mutation number per trillion base of each sample, obtained the resultant mutation load, and further determined the relationship between mutation load and overall survival (OS).
Verification of risk characteristics and prognostic models
The genes obtained by high and low mutation load difference analysis were analyzed again between tumor and normal tissues, and further univariate Cox regression analysis and Lasso regression analysis were performed. After identifying independent immune genes and determining their coefficients, we established a risk score prediction model to divide HCC patients into high-risk and low-risk groups. The receiver operating characteristic (ROC) curve was used to test the prediction efficiency of the survival model, the model was verified by the GEO data set, and the survival analysis was conducted by Kaplan-Meier analysis.
Further verification of model genes
The gene expression microarray data set, GSE14520 was downloaded from GEO (https://www.ncbi.nlm.nih.gov/geo), and included HCC tissue and normal control tissue or normal tissue adjacent to the cancer. The data set contains the clinical data and survival information of 220 normal control cases and 2,422 HCC patients. The model gene was verified by the GSE14520 data set.
Enrichment function analysis
Using the “clusterProfiler”, “richplot”, and “ggplot2” software packages from R (the R Foundation for Statistical Computing), we explored the potential biological function and pathway of immune-related genes in primary liver cancer. With the “clusterProfiler” and “richplot” packages, hypergeometric distributions were used to calculate each item of the P and Q values of GO or KEGG. AP and Q values less than 0.05 were considered to be significantly enriched functions.
Analysis of tumor infiltrating cells
The CIBERSORT deconvolution algorithm was used to calculate the relative abundance of 22 immune cell types in 374 cases of HCC and 50 cases of normal controls.
Survival analysis
Using R language with the “survival” and “survminer” software packages, we conducted univariate Cox regression and Kaplan-Meier analyses to evaluate the prognostic effect of differentially expressed genes. The survival curve was drawn by Kaplan-Meier method with a P value <0.05 being considered as statistically significant.
Analysis of the relationship between clinical features and immune matrix score
The clinical characteristic data of HCC patients were sorted according to TCGA. Only 374 HCC cases had detailed clinical features. The relationship between clinical features and the immune matrix score was analyzed by R, and the significance was determined by Wilcoxon rank-sum or Kruskal-Wallis rank-sum test.
Statistical analysis
The R language package (https://www.r-project.org/) was used for data analysis. The differential genes with a log fold change |logFC| >1 and false discovery rate FDR <0.05 were deemed as significant. Wilcoxon analysis was used to analyze the differences between groups, and spearman method was used to analyze the correlation. P<0.05 was considered to be statistically significant.
Results
Analysis of mutation load, prognosis, and clinical correlation of primary HCC
The SNV data of 50 normal patients, 374 tumors, and tumor mutations were extracted from TCGA database, and the SNV results were analyzed by VarScan software. The results showed that TP53, CTNNB1, TTN, MUC16, and ALB were the coding error genes in the top 5 HCC mutation loads, and the main manifestations were base deletions, mutations, and single-nucleotide polymorphism (SNP) site mutations. Further study on the relationship between mutation load and prognosis showed that mutations with high loads had worse survival (P<0.001) and were correlated with age (P<0.001) and sex (P<0.004; Figure 1).
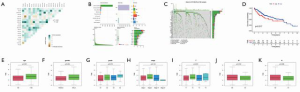
Differential gene analysis of HCC TMB for OS and between tumor and normal tissue
Our prognostic analysis of mutation load showed that only 25% of the cases before mutation load showed a difference in OS. Further analysis of the difference between the first 25% of TMB cases and the last 75% of cases showed that there were 805 gene differences. We then analyzed the difference between tumor and normal tissues by analyzing the difference between high and low mutation load, and obtained 122 differential genes, of which 73 were downregulated and 49 were upregulated (Figure 2).
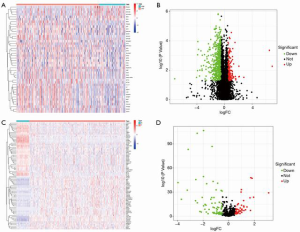
Univariate Cox and Lasso regression analysis of high and low TMB of HCC and establishment of a clinical model
Univariate Cox and Lasso analysis of TMB and differentially expressed genes in high and low TMB tumors showed that SQSTM1, ME1, BAMBI, and PTTG1 were closely related to prognosis. Therefore, we used these 4 genes to construct a nomogram prognostic mode, and predict the prognosis of 1-, 3-, and 5-year OS. Based on the median risk, patients were divided into a high-risk group and a low-risk group. Risk score, survival time, and status of patients showed that patients in the high-risk group had worse OS. Next, we used receiver operating characteristics (ROC) curve analysis to evaluate the prognostic value of the clinical model constructed by these 4 genes. The results showed that the area under the ROC curve (AUC) of 1-, 3-, and 5-year OS was 0.751, 0.643, and 0.628 (Figure 3), respectively.
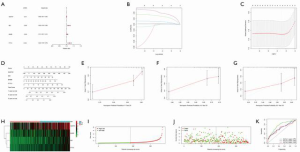
TCGA and GEO database verification
In order to further understand the relationship between the expression of SQSTM1, ME1, BAMBI, and PTTG1 in HCC and normal tissues and prognosis, we first used TCGA database for analysis. Overall, this analysis showed that the high-risk group had worse prognosis (P<0.001). SQSTM1 (0.0002), ME1 (0.0004), BAMBI (P<0.0001), and PTTG1 (P<0.0001) were highly expressed in HCC tissues, and the OS of the high expression group was poor (SQSTM1 P<0.001, ME1 P=0.002, BAMBI P<0.001, PTTG1 P=0.042). Secondly, we further verified the GSE14520 data set in GEO database. Overall analysis showed that high risk was associated with poor OS (P<0.005), SQSTM1 (P<0.0001), ME1 (P<0.0001), BAMBI (P<0.0001), and PTTG1 (P<0.0001) were all highly expressed in HCC tissues, but high expression of BAMBI (P<0.0001) and PTTG1 (P<0.0001) were associated with poor OS; these findings were different from those derived from TCGA database (Figure 4).
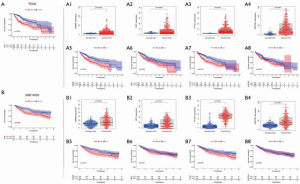
Analysis of enrichment function of the 122 differential genes
In order to further clarify the potential function of the 122 differential genes, we used GO and KEGG pathway functional enrichment analysis. Enrichment function analysis showed that top 5 biological processes included humoral immune response, complement activation, adaptive immune response of immune receptor somatic recombination based on immunoglobulin superfamily domain, lymphocyte-mediated immune response, and circulating immunoglobulin-mediated humoral immune response. The top 5 cellular components included immunoglobulin, collagen-containing extracellular matrix, external side of plasma membrane, blood microparticle, and collagen trimer. The molecular functions were mainly antigen binding and immunoglobulin binding. KEGG pathway analysis showed that these genes were mainly associated with extracellular matrix (ECM)-receptor interaction, transforming growth factor beta (TGF-β) signaling pathway, necrosis factor kappa B (NF-κB) signaling pathway, and leukocyte transendothelial migration (Figure 5). These results show that these differential genes play an important role in the immune system.
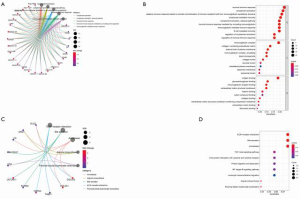
Analysis of immune infiltration in patients with primary liver cancer
The level of immune infiltration and the relationship between different types of immune cells were analyzed using the cohort of primary liver cancer in TCGA. The results showed that NK cell resting, Mast cells resting, T cells CD4 memory resting, B cells memory, Dendritic cells resting and Macrophages Macrophages M1, T cells regulatory (Tregs), T cells follicular helper, T cells CD8 and T cells CD4 memory Activated was positively correlated with the level of infiltration. Mast cells were activated, T cells gamma delta, Neutrophils, Monocytes, Macrophages M2, NK cells were activated, Plasma cells and B cells Naive was inversely correlated with the level of infiltration In order to further understand the difference in the distribution of immune cells between HCC and normal tissues, we used R software for analysis. The results showed B Cells naive, B Cells memory, Plasma cells, T Cells CD4 memory activated, T Cells regulatory (Tregs) and T Cells gamma Delta, NK cell resting, NK cell activated, Monocytes, Macrophages M0, Macrophages M2, Dendritic cells resting, Mast cells RBF, Mast cells activated and Neutrophils have differences in the distribution of HCC and normal tissues, with statistical significance (Figure 6). Further analysis of the relationship between immune cells and the prognosis of HCC patients showed that the increase of M0 macrophages (P<0.001), M2 macrophages (P<0.014), and naïve CD4+ T cells (P<0.017) was associated with poor prognosis, while the decrease of CD8+ T cells (P<0.001) was associated with poor prognosis; meanwhile, the other levels of immune cell infiltration had no significant effect on the prognosis of HCC (Figure 7). Finally, we analyzed the correlation between the risk scores of 22 kinds of immune cells and the model. The results showed that M2 macrophages (P=0.026), resting mast cells (P=0.013), activated NK cells (P=0.012), activated CD4+ memory T cells (P=0.00068), follicular helper T cells (P=0.00011), and Tregs (P=0.0036) were closely related to the model (Figure 8).
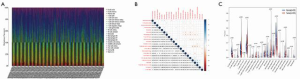
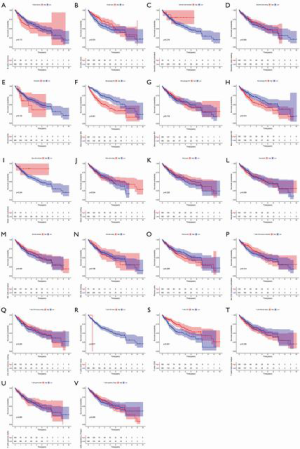
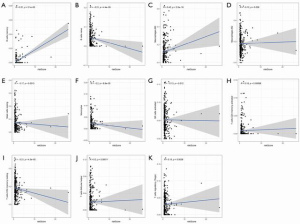
Discussion
At present, there is no clear clinical data or study findings that explain the role of TMB in the formation and development of liver cancer, but its value can be confirmed by other tumors. Snyder et al. confirmed for the first time in 2014 that there is a correlation between the efficacy of TMB and CTLA-4 antibodies in the treatment of malignant melanoma, and that the efficacy of immunosuppressants in patients with high levels of TMB melanoma is better than that of patients with low levels of TMB expression (26). A series of studies in non-small cell lung cancer have found that TMB is related to the therapeutic effect of PD-1 antibody. Patients with high expression of TMB and PD-L1 have longer progression-free survival than patients with high expression of TMB or PD-L1. Furthermore, TMB alone or in combination can predict the efficacy of treatment (27-31). Samstein et al. conducted a large-scale and comprehensive study on the prediction of tumor immunotherapy efficacy with TMB by analyzing the clinical and genomic data of 1,662 patients with advanced cancer treated with ICIs and 5,371 patients who did not undergo ICI therapy. In most cases’ histology, it was observed that there was a correlation between higher TMB and improved survival rate, but there was no unified definition for a threshold that could divide high from low levels of TMB (32). In our study, we found that the expression level of TMB was closely related to age and sex, and the patients with high expression of TMB had a worse prognosis. We speculate that this may be due to two reasons. First, there is heterogeneity among different tumors, and each kind of tumor has a unique mutation spectrum, so the distribution of TMB in different tumor types is also heterogeneous. Second, the liver constitutes a special immune microenvironment and complex immune regulation network. Therefore, it is necessary to analyze the correlation of TMB with HCC using basic research in gene mutations.
Through the analysis of differentially expressed genes in TMB, we found that SQSTM1, ME1, BAMBI, and PTTG1 could be used as independent risk factors for the prognosis of HCC. SQSTM1 protein is a multifunctional ubiquitin-binding folded protein, which plays an important role in autophagy regulation, proteasome pathway, NF-kB, and other signaling pathways. Autophagy inhibition leads to p62 overexpression, and can promote tumor cell proliferation by regulating NF-kB and other signaling pathways, causing reactive oxygens species (ROS) and DNA damage (33). ME1 links glycolysis with the citric acid cycle, which is important for NADPH production, glutamine metabolism, and adipogenesis. Inhibition of ME1 expression can reduce HCC metastasis by inhibiting the epithelial-mesenchymal transformation induced by ROS. ME1 overexpression is associated with poor prognosis in patients with liver cancer (34). BAMBI plays an important role in signal transduction of many developmental and pathological processes, and knockout of BAMBI gene can lead to stronger tumorigenicity of HCC cells (35). PTTG1 is involved in the process of cell differentiation, apoptosis, DNA damage repair, angiogenesis, tumor growth, invasiveness, and invasive behavior, and can promote the transcription of genes directly or indirectly involved in tumorigenesis (36-39). PTTG1 participates in TNF-α-related HCC, through c-myc induction, and its expression level is negatively correlated with the survival rate of HCC patients (40). These studies show that SQSTM1, ME1, BAMBI, and PTTG1 are involved in the emergence and development of HCC, and are closely related to the prognosis of patients with HCC, which is consistent with our findings, but their participation in the specific regulatory process and role of HCC needs further clarification. It has been reported that ECM, not only as the structural support of tumor growth, but also as an important component of tumor microenvironment, participates in the process of occurrence and development of HCC (41). TGF-β contributes to cell growth, differentiation, apoptosis, and cell homeostasis; is involved in ECM regeneration and immunosuppressive messenger RNA (mRNA) transcription; and promotes the development of HCC (42,43). These reports are consistent with the findings of our GO and KEGG studies, but need to be further verified by basic experiments. In our study, a prognostic model based on four TMB-related genes (SQSTM1, ME1, BAMBI, and PTTG1) was developed, and the risk scores generated by this model could be used as independent prognostic markers to distinguish between patients with different survival outcomes. Prognostic models based on TMB related genes can effectively predict overall survival of HCC patients and optimize personalized treatment decisions.
Our study found that there were differences in the expression of many kinds of immune cells between HCC and normal tissues, and that the increase of M0 macrophages, M2 macrophages, and naïve CD4+ T cells was associated with poor prognosis, while the decrease of CD8+ T cells was associated with poor prognosis. Further analysis of the correlation between the immune cells and the model risk score showed that there was a positive or negative correlation between the model risk score and most of the immune cells. Ma et al. have demonstrated that a class of CD8+ T cells that highly express the characteristics of exhaustion-related inhibitory receptors and transcription factors are significantly associated with poor prognosis in patients with HCC (44). Hepatic macrophages are composed of Kupffer cells and monocytes, and the irritation of chronic liver injury can be transformed into activated M1 or M2 macrophages (45). Macrophages form the microenvironment of HCC by regulating immune response and secreting a variety of cytokines, and play an important role in the uncontrollable malignant growth of tumors. Recent studies have shown that macrophages play a vital role in the development and progression of HCC through immunosuppression, enhanced tumor invasion and metastasis, regulation of angiogenesis, maintenance of stem cells, and therapeutic drug resistance (46-50). Our results are consistent with these reports and show that there is a certain correlation between immune cells and the expression level of TMB. The level of TMB in HCC is closely related to the function of ECM, thus enhancing the body’s recognition of tumor cells and expression of tumor suppressor genes through immune cells. Our study has some limitations and lacks experimental verification in vivo and in vitro.
Conclusions
High levels of TMB in patients with HCC are associated with poor survival. TMB can be used as a starting point to further clarify the possible mechanism underlying the emergence, development, and prognosis of HCC. Furthermore, a relationship between immune cells and TMB exists, and may play an important role in HCC. Further study of TMB and immune cells may have considerable value in development of immunotherapy for liver cancer in the future.
Acknowledgments
Funding: None.
Footnote
Reporting Checklist: The authors have completed the REMARK reporting checklist. Available at http://dx.doi.org/10.21037/jgo-21-259
Conflicts of Interest: All authors have completed the ICMJE uniform disclosure form (available at http://dx.doi.org/10.21037/jgo-21-259). The authors have no conflicts of interest to declare.
Ethical Statement: The authors are accountable for all aspects of the work in ensuring that questions related to the accuracy or integrity of any part of the work are appropriately investigated and resolved. The study was conducted in accordance with the Declaration of Helsinki (as revised in 2013).
Open Access Statement: This is an Open Access article distributed in accordance with the Creative Commons Attribution-NonCommercial-NoDerivs 4.0 International License (CC BY-NC-ND 4.0), which permits the non-commercial replication and distribution of the article with the strict proviso that no changes or edits are made and the original work is properly cited (including links to both the formal publication through the relevant DOI and the license). See: https://creativecommons.org/licenses/by-nc-nd/4.0/.
References
- Akinyemiju T, Abera S, Ahmed M, et al. The Burden of Primary Liver Cancer and Underlying Etiologies From 1990 to 2015 at the Global, Regional, and National Level: Results From the Global Burden of Disease Study 2015. JAMA Oncol 2017;3:1683-91. [Crossref] [PubMed]
- Sung H, Ferlay J, Siegel R, et al. Global cancer statistics 2020: GLOBOCAN estimates of incidence and mortality worldwide for 36 cancers in 185 countries. CA Cancer J Clin 2021;71:209-49. [Crossref] [PubMed]
- Fujiwara N, Friedman S, Goossens N, et al. Risk factors and prevention of hepatocellular carcinoma in the era of precision medicine. J Hepatol 2018;68:526-49. [Crossref] [PubMed]
- Forner A, Reig M, Bruix J. Hepatocellular carcinoma. Lancet 2018;391:1301-14. [Crossref] [PubMed]
- Marrero JA, Kulik LM, Sirlin CB, et al. Diagnosis, Staging, and Management of Hepatocellular Carcinoma: 2018 Practice Guidance by the American Association for the Study of Liver Diseases. Hepatology 2018;68:723-50. [Crossref] [PubMed]
- Tagliamonte M, Mauriello A, Cavalluzzo B, et al. Tackling hepatocellular carcinoma with individual or combinatorial immunotherapy approaches. Cancer Lett 2020;473:25-32. [Crossref] [PubMed]
- Prieto J, Melero I, Sangro B. Immunological landscape and immunotherapy of hepatocellular carcinoma. Nat. Rev. Gastroenterol. Hepatol 2015;12:681-700. [Crossref] [PubMed]
- Tai D, Choo S, Chew V. Rationale of Immunotherapy in Hepatocellular Carcinoma and Its Potential Biomarkers. Cancers (Basel) 2019;11:1926. [Crossref] [PubMed]
- Feng Z, Rong P, Wang W. Meta-analysis of the efficacy and safety of PD-1/PD-L1 inhibitors administered alone or in combination with anti-VEGF agents in advanced hepatocellular carcinoma. Gut 2020;69:1904-6. [Crossref] [PubMed]
- Yarchoan M, Hopkins A, Jaffee EM. Tumor Mutational Burden and Response Rate to PD-1 Inhibition. N. Engl. J. Med 2017;377:2500. [Crossref] [PubMed]
- Klempner SJ, Fabrizio D, Bane S, et al. Tumor mutational burden as a predictive biomarker for response to immune checkpoint inhibitors: a review of current evidence. Oncologist 2020;25:e147-e159. [Crossref] [PubMed]
- Alexandrov LB, Nik-Zainal S, Wedge DC, et al. Signatures of mutational processes in human cancer. Nature 2013;500:415-21. Erratum in: Nature 2013;502:258. [Crossref] [PubMed]
- Hellmann MD, Paz-Ares L, Bernabe Caro R, et al. Nivolumab plus ipilimumab in advanced non-small-cell lung cancer. N Engl J Med 2019;381:2020-31. [Crossref] [PubMed]
- Wei SC, Duffy CR, Allison JP. Fundamental Mechanisms of Immune Checkpoint Blockade Therapy. Cancer Discov 2018;8:1069-86. [Crossref] [PubMed]
- El-Khoueiry AB, Sangro B, Yau T, et al. Nivolumab in patients with advanced hepatocellular carcinoma (CheckMate 040): an open-label, non-comparative, phase 1/2 dose escalation and expansion trial. Lancet 2017;389:2492-502. [Crossref] [PubMed]
- Quail DF, Joyce JA. Microenvironmental regulation of tumor progression and metastasis. Nat Med 2013;19:1423-37. [Crossref] [PubMed]
- Wang JP, Tang YY, Fan CM, et al. The role of exosomal non-coding RNAs in cancer metastasis. Oncotarget 2017;9:12487-502. [Crossref] [PubMed]
- Wei F, Tang L, He Y, et al. BPIFB1 (LPLUNC1) inhibits radioresistance in nasopharyngeal carcinoma by inhibiting VTN expression. Cell Death Dis 2018;9:432. [Crossref] [PubMed]
- Wei F, Wu Y, Tang L, et al. BPIFB1 (LPLUNC1) inhibits migration and invasion of nasopharyngeal carcinoma by interacting with VTN and VIM. Br J Cancer 2018;118:233-47. [Crossref] [PubMed]
- Kim HD, Song GW, Park S, et al. Association Between Expression Level of PD1 by Tumor-Infiltrating CD8 T Cells and Features of Hepatocellular Carcinoma. Gastroenterology 2018;155:1936-50.e17. [Crossref] [PubMed]
- Yan L, Xu F, Dai CL. Relationship between epithelial-to-mesenchymal transition and the inflammatory microenvironment of hepatocellular carcinoma. J. Exp. Clin. Cancer Res 2018;37:203. [Crossref] [PubMed]
- Flecken T, Schmidt N, Hild S, et al. Immunodominance and functional alterations of tumor-associated antigen-specific CD8+ T-cell responses in hepatocellular carcinoma. Hepatology 2014;59:1415-26. [Crossref] [PubMed]
- Kim J, Bae JS. Tumor-Associated Macrophages and Neutrophils in Tumor Microenvironment. Mediators Inflamm 2016;2016:6058147 [Crossref] [PubMed]
- Mantovani A, Marchesi F, Malesci A, et al. Tumour-associated macrophages as treatment targets in oncology. Nat Rev Clin Oncol 2017;14:399-416. [Crossref] [PubMed]
- Tian Z, Hou X, Liu W, et al. Macrophages and hepatocellular carcinoma. Cell Biosci 2019;9:79. [Crossref] [PubMed]
- Snyder A, Makarov V, Merghoub T, et al. Genetic basis for clinical response to CTLA-4 blockade in melanoma. N Engl J Med 2014;371:2189-99. [Crossref] [PubMed]
- Rizvi NA, Hellmann M, Snyder A, et al. Cancer immunology. Mutational landscape determines sensitivity to PD-1 blockade in non-small cell lung cancer. Science 2015;348:124-8. [Crossref] [PubMed]
- Antonia SJ, López-Martin JA, Bendell J, et al. Nivolumab alone and nivolumab plus ipilimumab in recurrent small-cell lung cancer (CheckMate 032): a multicentre, open-label, phase 1/2 trial. Lancet Oncol 2016;17:883-95. [Crossref] [PubMed]
- Hellmann MD, Ciuleanu TE, Pluzanski A, et al. Nivolumab plus Ipilimumab in Lung Cancer with a High Tumor Mutational Burden. N Engl J Med 2018;378:2093-104. [Crossref] [PubMed]
- Hellmann MD, Nathanson T, Rizvi H, et al. Genomic features of response to combination immunotherapy in patients with advanced non-small-cell lung cancer. Cancer Cell 2018;33:843-852.e4. [Crossref] [PubMed]
- Ready N, Hellmann M, Awad M, et al. First-Line Nivolumab Plus Ipilimumab in Advanced Non-Small-Cell Lung Cancer (CheckMate 568): Outcomes by Programmed Death Ligand 1 and Tumor Mutational Burden as Biomarkers. J Clin Oncol 2019;37:992-1000. [Crossref] [PubMed]
- Samstein RM, Lee CH, Shoushtari AN, et al. Tumor mutational load predicts survival after immunotherapy across multiple cancer types. Nat Genet 2019;51:202-6. [Crossref] [PubMed]
- Taniguchi K, Yamachika S, He F, et al. p62/SQSTM1-Dr. Jekyll and Mr. Hyde that prevents oxidative stress but promotes liver cancer. FEBS Lett 2016;590:2375-97. [Crossref] [PubMed]
- Wen D, Liu D, Tang J, et al. Malic enzyme 1 induces epithelial-mesenchymal transition and indicates poor prognosis in hepatocellular carcinoma. Tumour Biol 2015;36:6211-21. [Crossref] [PubMed]
- Lee S, Lee M, Zhang J, et al. C-terminal-truncated HBV X promotes hepato-oncogenesis through inhibition of tumor-suppressive β-catenin/BAMBI signaling. Exp Mol Med 2016;48:e275 [Crossref] [PubMed]
- Tong Y, Eigler T. Transcriptional targets for pituitary tumor-transforming gene-1. J Mol Endocrinol 2009;43:179-85. [Crossref] [PubMed]
- Moreno-Mateos MA, Espina ÁG, Torres B, et al. PTTG1/securin modulates microtubule nucleation and cell migration. Mol Biol Cell 2011;22:4302-11. [Crossref] [PubMed]
- Yoon CH, Kim MJ, Lee H, et al. PTTG1 oncogene promotes tumor malignancy via epithelial to mesenchymal transition and expansion of cancer stem cell population. J Biol Chem 2012;287:19516-27. [Crossref] [PubMed]
- Chen R, Duan J, Li L, et al. mTOR promotes pituitary tumor development through activation of PTTG1. Oncogene 2017;36:979-88. [Crossref] [PubMed]
- Lin X, Yang Y, Guo Y, et al. PTTG1 is involved in TNF-α-related hepatocellular carcinoma via the induction of c-myc. Cancer Med 2019;8:5702-15. [Crossref] [PubMed]
- Bonnans C, Chou J, Werb Z. Remodelling the extracellular matrix in development and disease. Nat Rev Mol Cell Biol 2014;15:786-801. [Crossref] [PubMed]
- Neuzillet C, Tijeras-Raballand A, Cohen R, et al. Targeting the TGFβ pathway for cancer therapy. Pharmacol Ther 2015;147:22-31. [Crossref] [PubMed]
- Zhang Q, Hou X, Evans B, et al. LY2157299 Monohydrate, a TGF-βR1 Inhibitor, Suppresses Tumor Growth and Ascites Development in Ovarian Cancer. Cancers (Basel) 2018;10:260. [Crossref]
- Ma J, Zheng B, Goswami S, et al. PD1 CD8 T cells correlate with exhausted signature and poor clinical outcome in hepatocellular carcinoma. J Immunother Cancer 2019;7:331. [Crossref] [PubMed]
- Scott CL, Zheng F, Baetselier PD, et al. Bone marrow-derived monocytes give rise to self-renewing and fully differentiated Kupffer cells. Nat Commun 2016;7:10321. [Crossref] [PubMed]
- Fan QM, Jing YY, Yu GF, et al. Tumor-associated macrophages promote cancer stem cell-like properties via transforming growth factor-beta1-induced epithelial-mesenchymal transition in hepatocellular carcinoma. Cancer Lett 2014;352:160-8. [Crossref] [PubMed]
- Wan S, Zhao E, Kryczek I, et al. Tumor-Associated Macrophages Produce Interleukin 6 and Signal via STAT3 to Promote Expansion of Human Hepatocellular Carcinoma Stem Cells. Gastroenterology 2014;147:1393-404. [Crossref] [PubMed]
- Yao W, Qian B, Li X, et al. A Natural CCR2 Antagonist Relieves Tumor-associated Macrophage-mediated Immunosuppression to Produce a Therapeutic Effect for Liver Cancer. EBioMedicine 2017;22:58-67. [Crossref] [PubMed]
- Yao RR, Li JH, Zhang R, et al. M2-polarized tumor-associated macrophages facilitated migration and epithelial-mesenchymal transition of HCC cells via the TLR4/STAT3 signaling pathway. World J Surg Oncol 2018;16:9. [Crossref] [PubMed]
- Zhang YL, Li Q, Yang XM, et al. SPON2 Promotes M1-like Macrophage Recruitment and Inhibits Hepatocellular Carcinoma Metastasis by Distinct Integrin-Rho GTPase-Hippo Pathways. Cancer Res 2018;78:2305-17. [Crossref] [PubMed]
(English Language Editor: J. Gray)