Identification of gene biomarkers and immune cell infiltration characteristics in rectal cancer
Introduction
Colorectal cancer (CRC) is one of the highest incidence cancers in the world, and it ranks second in the United States for cancer-related mortality (1). CRC can be divided into 2 major categories: rectal cancer and colon cancer. In patients with CRC, the rectum is the more frequently affected site compared to colon. In China, the incidence rate is significantly higher in males than females. Common early detection methods for CRC involve colonoscopic, fecal, and blood sample examinations. According to the tumor size, location, and degree, as well as genetic changes and the patient’s health status, CRC treatment can include surgery, chemotherapy, radiotherapy, molecular-targeted therapy, and immunotherapy (2-5).
It is important to note that rectal cancer and colon cancer are not identical, and appropriate treatment based on their own characteristics is of great importance. Rectal cancers account for about 30% of CRCs and the incidence rate increased more significantly compared to colon cancer (6). Early diagnosis or screening may reduce the morbidity of rectal cancer effectively. Currently, the standard treatment plan for locally advanced rectal cancer is neoadjuvant chemoradiotherapy (nCRT) followed by the total mesorectal excision, but there are a lack of specific biomarkers to monitor treatment effect (7,8). Thus, sensitive and reliable biomarkers are necessary to improve the early diagnosis and treatment of the disease.
Biomarkers based on tissue or blood samples may have a certain sensitivity and specificity for early prediction of disease occurrence or treatment response. To date, methods for the early diagnosis and treatment of CRC have been widely researched. For rectal cancer, the biomarkers that have been found, including gene expression profiles, DNA mutation and methylation, single-nucleotide polymorphism, circulating cell-free nucleic acids, microRNAs, long non-coding RNAs, antigens, enzymes, amino acids, lipids, circulating tumor cells (CTCs), tumor immune cell infiltration, and immune inflammatory cytokines (9). However, these biomarkers have not been fully applied in the clinic setting. A possible reason for this is the limitation of biomarker research. Firstly, the expression of a single biomarker can vary greatly depending on race, region, or study cohort (especially sample size), so a single biomarker does not have universal applicability. Secondly, the human body is a life system with multiple synergistic biological functions, and a single biomarker cannot fully reflect the state of the patient’s disease or body; therefore, the integration of different biomarkers of the same category or even different biomarker categories is needed. An increasing bank of studies shows that integrated biomarkers are more significant compared with a single biomarker for the diagnosis and treatment of diseases.
Abnormal gene expression may play a key mechanistic role in cancer initiation. The occurrence of many types of cancers, results from the imbalance of expression of multiple genes. Therefore, variance in gene expression can provide clues about cancer occurrence during the early stage. The screening of differentially expressed genes (DEGs) between cancer patients and healthy individuals can be an effective way to discover early biomarkers. Besides gene regulation, the tumor microenvironment (TME) also performs a vital role in the initiation and progress of cancer. Immune cells are important constituents of the TME. It has been recognized that the immune system plays an irreplaceable role in cancer. Genomic changes lead to the production of tumor antigens, which are recognized by the immune system as non self sources. In this case, cellular immune response is triggered. The immune system plays a role in immune surveillance through the way that immune cells infiltrated into the tumor microenvironment and regulated tumor progression. As one of the characteristics of cancer, immune cell infiltration is a key factor affecting the outcome of cancer immunotherapy. Immunotherapy can also affect the infiltration level of immune cells in tumor tissues. Previous studies have shown that the number of tumor infiltrating lymphocytes (TILs) is closely associated with the prognosis of CRC, for instance, a high density of CD8+ T lymphocytes is related to a better prognosis (10). The cytotoxic lymphocyte infiltration and its related immunogenomic pathways may be effective targets for CRC (11).
Considering the limitations of a single biomarker and the importance of tumor microenvironment, we propose gene biomarkers combined with tumor immune cell infiltration maybe a more effective indicator of disease progression. The reliability of biomarkers can be improved by clinical research based on different countries, multiple centers, and a large sample size. Therefore, in this investigation, rectal cancer microarray datasets with a sample size of more than 30 in the Gene Expression Omnibus (GEO) database were integrated using the robust rank aggregation (RRA) method to obtain the DEGs between normal and tumor tissue samples. The functions of robust DEGs were subsequently annotated through enrichment analyses of Gene Ontology (GO) and Kyoto Encyclopedia of Genes and Genomes (KEGG) pathway (12,13). Then, the protein-protein interaction (PPI) network of the DEGs was set up to analyze the hub genes, and the survival analysis was also carried out to screen the prognostic genes based on a rectal adenocarcinoma (READ) dataset in The Cancer Genome Atlas (TCGA) database. Furthermore, the CIBERSORT algorithm was adopted to investigate the immune cell infiltration state of rectal cancer tissue samples in comparison with normal ones, in order to find immunology characteristics of rectal cancer. We aim to integrate genetic and immunological features as precise biomarkers for rectal cancer.
We present the following article in accordance with the REMARK and MDAR reporting checklist (available at https://dx.doi.org/10.21037/jgo-21-255).
Methods
The study was conducted in accordance with the Declaration of Helsinki (as revised in 2013). Since this was a retrospective database study based on a public database, approval from the ethics committee was not required.
Data collection and processing
The platform annotation document and matrix files of microarray datasets of rectal cancer were selected and downloaded from the GEO database (https://www.ncbi.nlm.nih.gov/geo/) according to the following inclusion criteria: (I) species were limited to Homo sapiens; (II) the minimum sample size was 30; (III) the experimental type of expression profiling was array; and (IV) both normal tissue samples and tumor tissue samples were available. Only 2 datasets GSE87211 and GSE90627 were included. There were 160 normal and 203 rectal cancer tissue samples in the GSE87211dataset, and 96 normal and 32 rectal cancer tissue samples in the GSE90627 dataset. The platforms were GPL13497 and GPL17077, respectively. The DEGs between the normal and rectal cancer tissue samples in each dataset were identified with the limma package in R4.0.2 (R Foundation for Statistical Computing, Vienna, Austria). The cut-off criteria were: |log2 fold change (FC)| >1 with P value <0.05. Additionally, the transcriptome profiles and corresponding clinical information of patients with READ were downloaded from the TCGA database (https://portal.gdc.cancer.gov/) for a survival analysis, which involved 2 normal and 84 READ tissue samples.
Robust DEG identification
The RRA method was used to integrate the 2 microarray datasets, and the robust DEGs were analyzed with R package Robust Rank Aggreg. Robust DEGs with |log2FC| >1 and P value <0.05 were considered to be Significant.
GO and KEGG enrichment analyses
GO enrichment analyses including molecular function (MF), biological process (BP), cellular component (CC), and KEGG enrichment analyses were further performed, and the results were visualized with R packages including “digest”, “GOplot”, “clusterprofiler”, “org.Hs.eg.db”, “enrichplot”, and “ggplot2”. P value <0.05 was deemed to have statistical significance.
Hub gene identification in the DEG network
The robust DEGs were put into the STRING database to obtain the PPI network with the confidence score set at 0.4. The results were visualized with the Cytoscape v3.6.0 software, in which the MCODE plugin was applied for identification of the significant modules in the PPI network. In line with previous research (14), the cytoHubba plugin in Cytoscape was used to perform an integrated analysis of multiple algorithms for topological parameters including Maximal Clique Centrality (MCC), Density of Maximum Neighborhood Component (DMNC), Maximum Neighborhood Component (MNC), Degree, Edge Percolated Component (EPC), BottleNeck, EcCentricity, Closeness, Radiality, and Betweenness. Hub genes involved in the regulation of rectal cancer were screened based on the scores of above 10 topological parameters.
Biological value of hub genes
Expression distribution, correlation, and receiver operating characteristic (ROC) curve analyses of the hub genes were performed with R package. The clinical information and corresponding RNA-seq-Fragments Per Kilobase of Exon Per Million Fragments Mapped (FPKM) data of patients with READ in the TCGA were extracted for a survival analysis of the screened hub genes. The survival and survminer packages of R language were used for the analysis, with the level of statistical significance set at P<0.05.
Immune infiltration analysis
The expression matrix of 22 types of immune cells was obtained using the CIBERSORT algorithm. The relative abundance of 22 types of immune cells was extracted for each sample, and the DEGs between normal and rectal cancer tissue samples were filtered by P value <0.05 using R packages “ggpubr” and “cluster”. Then, the differences between normal and rectal cancer tissue samples were uncovered by performing principal component analysis (PCA). The correlation between each other of infiltrated immune cells was analyzed using R package “corrplot”.
Association of prognostic hub gene expression with immune cell infiltration
To explore the relationships between prognostic hub genes and infiltrated immune cells, correlation scatter plots were drawn using R packages “ggplot2”, “ggpubr” and “ggpmisc”. The evaluation criteria were as follows: a correlation coefficient | R | between 0.8–1.0 stood for a very strong correlation; 0.6–0.8 stood for a strong correlation; 0.4–0.6 stood for a moderate correlation; 0.2–0.4 stood for a weak correlation; and 0.0–0.2 stood for a very weak or no correlation.
Statistical analysis
The statistical analyses were performed using R 4.0.2 (https://www.r-project.org/) and ActivePerl (https://www.activestate.com/). Plots were drawn with the R package software. Correlation analyses were also performed using the R package software. All the statistical tests were 2-sided, and P<0.05 was considered statistically significant.
Results
Clinical characteristics
Among the cases in the GSE87211 dataset, there were 248 (68.32%) males and 115 (31.68%) females, with ages ranging from 35.7 to 81.5 years old (62.90±9.69 years old); for 2 cases, the sex and age were not recorded. Among the GSE90277 dataset cases, there were 76 (59.375%) males and 52 (40.625%) females, whose ages ranged from 27 to 79 years old (58.56±12.53 years old). Among the cases from the TCGA database, there were 47 (56.63%) males and 36 (43.37%) females included, and the age range was from 33 to 90 years old (64.18±11.62 years old); for 1 case, the sex and age were not recorded. The cases with incomplete information were excluded.
Screening of DEGs in each dataset
After an integrated analysis of the GSE87211 and GSE90277 microarray data using R language, 256 normal tissue samples and 235 rectal cancer tissue samples were included in this study. After filtering according to the cut-off criteria of |log2 FC| >1 and P value <0.05, 2,897 DEGs were identified in the GSE87211 dataset, including 1,398 upregulated and 1,499 downregulated genes, while there were 2,803 DEGs in GSE90277 dataset, including 1,404 upregulated and 1,399 downregulated genes. The distribution of statistical parameters of different genes in the 2 datasets is shown in separate volcano plots (Figure 1A,B).
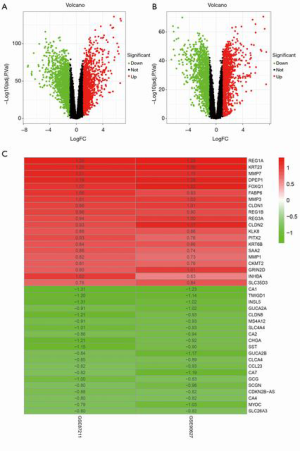
Identification of robust DEGs
The RRA method can be applied for effective integration of different datasets and can minimize bias and errors among them. Through this approach, as screened using the same cut-off criteria as those for DEGs, 137 robust DEGs were distinguished, including 49 upregulated and 88 downregulated genes. The specific information of all robust DEGs is listed in Table S1, and among them the top 20 upregulated and downregulated ones are visualized in the heatmap (Figure 1C). A consistent change trend of the same genes in different datasets can be observed.
Functional analysis of robust DEGs
The functions of the screened robust DEGs were further explored through GO enrichment and KEGG pathway enrichment analyses. The GO enrichment results revealed that the robust DEGs were mainly enriched in MFs of receptor ligand activity, signaling receptor activator activity and carbonate dehydratase activity; the most significant BPs were bicarbonate transport, chloride transport and antimicrobial humoral response; the most significant CCs included the apical part of cell, apical plasma membrane and zymogen granule (Figure 2A, Table S2). The KEGG pathway enrichment analysis indicated that the top 5 pathways through which the robust DEGs regulated the rectal cancer were: nitrogen metabolism, proximal tubule bicarbonate reclamation, cytokine-cytokine receptor interaction, pyruvate metabolism, and pancreatic secretion (Figure 2B, Table S3).
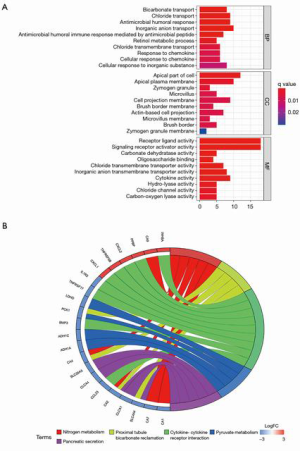
Construction of the PPI Network
The PPI interaction network of the robust DEGs was constructed using the STRING database and visualized with Cytoscape 3.6.0. There were 86 nodes and 156 edges, with 34 upregulated and 52 downregulated genes, after the removal of the disconnected nodes (Figure 3A). The whole PPI network was modularized into 5 key parts using the MCODE plugin; the scores of modules 1, 2, 3, 4 and 5 were 7.429, 6, 4, 3.333 and 3, respectively. According to the enrichment analyses of GO and KEGG (Tables S2,S3), module 1 was mainly related to the pancreatic secretion pathway (Figure 3B); module 2 was mainly related to the cytokine-cytokine receptor interaction pathway (Figure 3C); module 3 was mainly related to the BPs of antimicrobial humoral response and humoral immune response and the MFs of peptidoglycan binding and oligosaccharide binding (Figure 3D); module 4 was mainly related to the BP of the collagen catabolic process and the MFs of metallopeptidase activity and serine-type endopeptidase activity (Figure 3E); and module 5 was related to the BP of calcium-independent cell-cell adhesion via plasma membrane cell-adhesion molecules (Figure 3F).
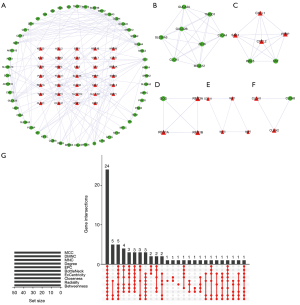
Hub gene identification
To comprehensively analyze the hub genes in the PPI network, the scores of 10 topological parameters were integrated using the Cytoscape 3.6.0 cytoHubba plugin. The top 50 genes according to each algorithm were ranked, and the intersection of the ranked genes of 10 algorithms were taken and exhibited as UpSet diagram in order to obtain the hub genes. Finally, 24 common genes were identified as hub genes (Figure 3G). The detailed information of the 24 hub genes is given in Table 1.
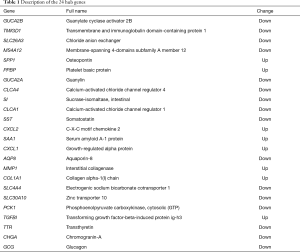
Full table
Diagnostic and prognostic value of hub genes
The expression distribution of the 24 hub genes in normal and rectal cancer tissue samples is illustrated as a boxplot (Figure 4A). Significant differences in these genes existed between the 2 tissue groups. GO terms for the BPs of the 24 hub genes were concentrated in the migration and chemotaxis of leukocytes and neutrophils, while the main rectal cancer-related KEGG pathways were the interleukin (IL)-17 signaling pathway and proximal tubule bicarbonate reclamation (Figure 4B,C, Tables S4,S5). The other functions of the hub genes were similar to those of the robust DEGs.
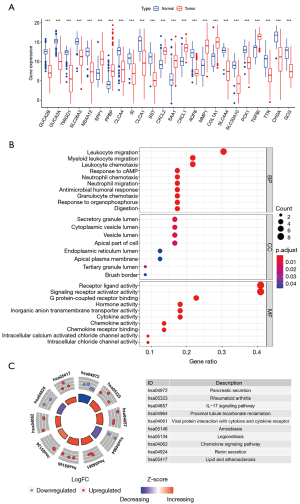
Correlation analysis revealed that all the hub genes were related to each other (Figure 5A). ROC curve analysis by R package indicated that all 24 hub genes had a high diagnostic value for identifying rectal cancer: GUCA2B (full name of genes were listed in Table 1) area under the curve (AUC) =0.970, GUCA2A AUC =0.965, TMIGD1 AUC =0.966, SLC26A3 AUC =0.945, MS4A12 AUC =0.938, SPP1 AUC =0.910, PPBP AUC =0.911, CLCA4 AUC =0.919, SI AUC =0.855, CLCA1 AUC =0.860, SST AUC =0.955, CXCL2 AUC =0.936, SAA1 AUC =0.928, CXCL1 AUC =0.948, AQP8 AUC =0.954, MMP1 AUC =0.927, COL1A1 AUC =0.909, SLC4A4 AUC =0.975, SLC30A10 AUC =0.946, PCK1 AUC =0.927, TGFBI AUC =0.955, TTR AUC =0.880, CHGA AUC =0.941, and GCG AUC =0.947 (Figure 5B).
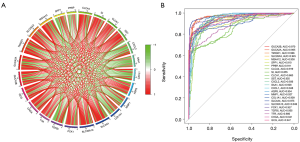
The prognostic value of hub genes was verified using the TCGA database. READ tissue samples in the TCGA dataset were divided into groups with high and low expression of hub genes according to the best-separation cutoff value, Kaplan-Meier (K-M) survival curves, and the correlation between hub genes and overall survival (OS) were analyzed using R package. The hub DEGs SAA1 (P=0.036), SPP1 (P=0.001), SI (P=0.02), CLCA1 (P=0.039), AQP8 (P=0.018), COLIA1 (P=0.025), MMP1 (P=0.016), and TGFBI (P=0.01) were found to be significantly associated with OS in the READ population. However, only the correlations of SAA1, SPP1, SI, and CLCA1with prognosis were consistent with the clinical significance of gene overexpression (Figure 6); the correlations between AQP8, COLIA1, MMP1, and TGFBI and prognosis were not in accordance with the clinical significance of gene overexpression (Figure S1). The diagnostic and prognostic value of CLCA1 in colon cancer and rectal cancer has been reported previously.
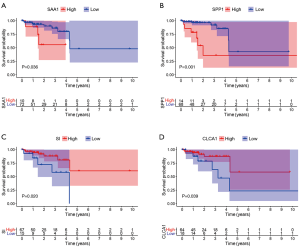
Analysis of immune cell infiltration
The 22 types of infiltrated immune cells in normal tissues and rectal cancer tissues were analyzed using the CIBERSORT algorithm. The distribution of the 22 types of immune cells in each sample showed there to be immunological differences between normal and rectal cancer tissue samples (Figure 7A). The abundance of immune cells in each sample is illustrated as a heatmap in Figure 7B, and the violin plot in Figure 7C visualizes the differences in each type of immune cell between the 2 groups. Compared with normal tissue samples, rectal cancer tissue samples displayed significantly increased abundance of T cells CD4 naive, T cells CD4 memory activated, natural killer (NK) cells resting, monocytes, macrophages M0, dendritic cells activated, mast cells activated, and neutrophils. Immune cells with significantly reduced abundance levels included B cells memory, plasma cells, T cells CD8, T cells CD4 memory resting, T cells follicular helper, macrophages M2, and mast cells resting. Immune cells such as B cells naive, T cells regulatory (Tregs), T cells gamma delta, NK cells activated, macrophages M1, dendritic cells resting, and eosinophils showed no difference between the 2 groups. The 15 differentially expressed immune cells may be closely related to rectal cancer occurrence and development. The PCA results of immune cell infiltration implied that there were differences between the normal and rectal cancer tissue groups, although in some individual samples, no difference was found between the 2 groups (Figure 7D).
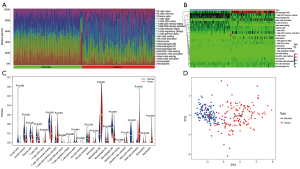
Correlation analysis showed that 12 of the 15 infiltrated immune cells were related to each other, with the exception of T cells follicular helper, NK cells resting, and dendritic cells activated (Figure 8A). The sensitivity and specificity of these 15 differential immune cells for the diagnosis of rectal cancer were further verified by ROC curve analysis (Figure 8B). The results showed that except for T cells follicular helper (AUC =0.422), NK cells resting (AUC =0.551), and dendritic cells activated (AUC =0.621), the other 12 types of infiltrated immune cells were all sensitive for the identification of rectal cancer, with AUCs >0.65. Therefore, the characteristics of immune cell infiltration maybe an effective indicator of rectal cancer.
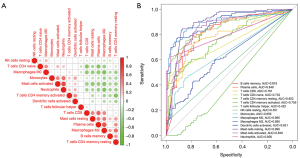
Relationship between prognostic genes and immune cell infiltration
The relationship between prognostic genes and immune infiltration in rectal cancer was further analyzed. According to relationship evaluation criteria, SAA1 exhibited a strong correlation with macrophages M0, mast cells resting, and macrophages M2; a moderate correlation with T cells CD4 memory activated, plasma cells, T cells CD4 memory resting, neutrophils, mast cells activated, T cells CD4 naive, and T cells CD8; and a weak correlation with B cells memory and monocytes. SPP1 exhibited a strong correlation with macrophages M0 and M2, mast cells resting, and mast cells activated; a moderate correlation with T cells CD4 memory resting, neutrophils, plasma cells, T cells CD4 naive, T cells CD8, and B cells memory; and a weak correlation with T cells CD4 memory activated and monocytes. SI exhibited a strong correlation with macrophages M2, neutrophils, and plasma cells; a moderate correlation with mast cells resting, T cells CD4 memory resting, B cells memory, macrophages M0, T cells CD4 naive, mast cells activated, and T cells CD8; and a weak correlation with T cells CD4 memory activated and monocytes (Figure 9). CLCA1 also exhibited correlations with the 12 types of infiltrated immune cells (Figure S2).
Discussion
Due to the complexity of pathogenesis and heterogeneity of cancer, the use of a single biomarker is not reliable or suitable for diagnosing or prognosticating the disease. It is necessary to integrate multiple biomarkers or assessment indices to obtain a more accurate indicator of cancer. Open public data platforms such as GEO and TCGA provide an opportunity for the discovery and validation of tumor biomarkers. For instance, a study in China showed that tissue expression of myc proto-oncogene protein (MYC), proliferating cell nuclear antigen (PCNA), and Metalloproteinase inhibitor 1 (TIMP1) protein combining with MRI-detected extramural vascular invasion could provide additional prognostic details for the preoperative treatment of rectal cancer (7) . Another study, also from China, integrated 3 GEO microarray datasets to analyze gene biomarkers of CRC. In the present work, the included 2 microarray datasets of rectal cancer from different countries, each of which had a minimum sample size of 32, which may be helpful in reducing the deviation caused by racial, regional, and individual differences in biomarker research. The same upward or downward trend for each gene in the 2 datasets suggested the reliability of the results.
The 137 robust DEGs including 49 upregulated genes and 88 downregulated genes were identified using a standard and robust RRA method, and 24 hub genes were further obtained. Analyses of GO enrichment and KEGG pathway indicated that the DEGs were mainly involved in bicarbonate transport and material metabolism-related BPs, MFs and signaling pathways. Besides, hub genes mainly took part in proximal tubule bicarbonate reclamation and the IL-17 signaling pathway. Previous studies have shown that the transport of bicarbonate plays an important role in the diagnosis and treatment of multiple cancers, and the expression levels of bicarbonate transporter in patients with colon cancer have changed widely compared with that in healthy bodies. The results of this work confirm that bicarbonate transport also plays an important role in rectal cancer.
In the PPI network, 24 hub genes were discovered through the integration of 10 algorithms to improve the accuracy. GUCA2B binding with GUCA2A can trigger the activation of a transmembrane receptor expressed on intestinal epithelial cells, guanylyl cyclase C (GUCY2C), which participates in regulatory mechanism of intestinal homeostasis, and its deletion may be related to the occurrence of CRC (15,16). Downregulation of GUCA2B and GUCA2A confirmed their important roles in rectal cancer development. In renal cancer, TMIGD1 is a tumor suppressor via regulation of p21Cip1/p27Kip1, but its relationship with other cancers is unclear (17). Attention has increasingly been paid to the role of plasma membrane transporters in cancer; for instance, the representative SLC26A3 is a tumor suppressor for colon cancer. As a Cl−/HCO3− exchanger, SLC26A3 can promote the outflow of HCO3− and possibly play an anti-tumor effect through the regulation of intracellular pH (18). As a member of the membrane-spanning 4-domains subfamily, MS4A12 is specifically expressed in colon epithelium, which can affect the colon cancer cell proliferation and cell cycle, and is a potential target for colon cancer immunotherapy (19). The calcium-activated chloride channel protein CLCA4 and the cyclic tetradeca peptide hormone SST can inhibit the proliferation and invasion of CRCs (20,21). SI deficiency can lead to dyspepsia, but its correlation with CRC is unclear (22). The calcium-activated chloride channel protein CLCA1 has been proposed as a diagnostic and prognostic biomarker for both colon and rectal cancer (23). Over expression of AQP8, a member of aquaporins family, can inhibit the proliferation and invasion of colon cancer cells (24). The decreased expression of CHGA in the early stage of colon cancer may be a novel biomarker for colon cancer diagnosis (25). Downregulated SLC4A4 can suppress progression of CRC (26). The relationship between TTR, GCG, and CRC has not yet been illuminated. A single-chip-based study identified PPBP as the core gene of rectal cancer (27). Chemokine (C-X-C motif) ligands including CXCL1 and CXCL2 are the only diagnostic and prognostic biomarkers for colon cancer (28). SPP1 is related to a poor prognosis of colon cancer, and MMP1 is related to a poor prognosis of CRC (20,29). SAA1 can promote breast cancer metastasis via immune cell infiltration, and it is highly expressed in colon cancer as well as TGFBI (30-32). The effects of downregulation or upregulation of the above genes demonstrate that they also play a major role in the development of rectal cancer. Additionally, COL1A1, SLC30A10, and PCK1 can promote CRC metastasis via regulation of the WNT/PCP pathway, the miR-21c/APC axis, and nucleotide synthesis (33-35). Furthermore, the expression levels of cancer promoting SLC30A10 and PCK1 were decreased in rectal cancer samples based on the 2 GEO datasets, which needs to be further verified. In brief, GUCA2B, GUCA2A, TMIGD1, SLC26A3, MS4A12, CLCA4, SST, SI, CLCA1, AQP8, SLC4A4, GCG, CXCL1, and SLC30A10 which have been reported to be diagnostic biomarkers in CRC by other microarray dataset studies, PPBP and CXCL2 which were found to be biomarkers of rectal cancer in a single microarray dataset study, and CHGA, TTR, SAA1, SPP1, MMP1, TGFBI, COL1A1, and PCK1, may constitute a gene biomarker group for the identification of rectal cancer.
The correlation analysis between these gene markers and survival time based on the TCGA database showed that 8 genes were significantly correlated with survival time (P<0.05). Among them, high expression levels of SAA1 and SPP1 are associated with a poor prognosis, while high expression levels of SI and CLCA1 are associated with an improved prognosis of rectal cancer, and the prognostic significance of CLCA1 has also been evidenced by research of other datasets, thus confirming the reliability of the study (23). For AQP8, MMP1, COL1A1, and TGFBI, the relationship between genes and prognosis is not consistent with the clinical significance of gene overexpression, which needs to be further verified using a larger sample. Thus, SAA1, SPP1, and SI could be new prognostic biomarkers of rectal cancer.
A variety of treatment methods based on immune classification exhibit certain advantages against cancer. Tumor immune infiltrating can directly or indirectly interfere with the progress of tumor through the release of cytokines or cytokine receptors and interaction with other components in the TME (36). The prognostic value of different immune cells depends on the type of cancer, and the type and abundance of infiltrated immune cells are important factors for tumor immune infiltration affecting the clinical prognosis. An understanding of the characteristics of immune cells and the body’s immune state is helpful for evaluating the pathological status or therapeutic effects. Analysis of the distribution of the 22 types of infiltrated immune cells using the CIBERSORT algorithm showed that 15 types of immune cells differed significantly between normal and rectal cancer tissues, including 1 type of B lymphocyte, 1 type of plasma cell, 5 types of T lymphocytes, 1 type of NK cell, 1 type of monocyte, 2 types of macrophages, 1 type of dendritic cell, 2 types of mast cells, and neutrophils. Previous studies have shown that Tregs have both positive and negative effects in CRC, which may depend on the location of invasion and the definition of “Tregs” used (37). However, there was no difference of Tregs in this study, which may be related to the above factors, or the region, race and number of samples. The increased abundance of T cells CD4 naive, T cells CD4 memory activated, NK cells resting, monocytes, macrophages M0, dendritic cells activated, mast cells activated, and neutrophils implies that these cells may be positively correlated with rectal cancer, whereas the decreased abundance of B cells memory, plasma cells, T cells CD8, T cells CD4 memory resting, T cells follicular helper, macrophages M2, and mast cells resting implies that these cells may be negatively associated with rectal cancer. Among the 12 sensitive immune cells, the prognostic genes SAA1, SPP1, and SI all have the closest correlation with macrophages M2. As a reported diagnostic and prognostic signature of colon cancer and rectal cancer, CLCA1 is also closely correlated with the 12 immune cells. Other studies have shown that in CRC, infiltrated T helper 1 cells, T cells follicular helper, macrophages M1, dendritic cells, and NK cells are associated with a good prognosis, while macrophages M2, myeloid-derived suppressor cells, T helper 17 cells, and B cells are associated with a poor prognosis (38). Because features of rectal cancer may be not entirely identical to those of colon cancer, the correlation between these infiltrated immune cells and the prognosis of rectal cancer needs to be further studied.
In conclusion, a biomarker group of 24 differential genes integrating with immune cell infiltration characteristics was found to be conducive to obtaining a comprehensive reflection of the body state and effectively indicating rectal cancer. Functional enrichment analysis indicated that the hub genes were closely associated with the process of rectal cancer. Among them, SAA1, SPP1, and SI may be novel prognostic biomarkers of rectal cancer. Of course, due to the limitation resulting from sample sizes in the GEO and TCGA databases, validation of our results in clinical cohort studies based on multiple centers and a large sample size is needed in the future. This study may provide reference for early diagnosis and treatment monitoring of rectal cancer at molecular and immune levels.
Acknowledgments
Funding: This work was supported by National Natural Science Foundation of China (No. 81803773), Incubating Program of Beijing Municipal Administration of Hospitals (PX2020078), and Open Project of Beijing Key Laboratory of Urine Cell Molecular Diagnosis (No. 2020-KF18).
Footnote
Reporting Checklist: The authors have completed the REMARK and MDAR reporting checklist. Available at https://dx.doi.org/10.21037/jgo-21-255
Conflicts of Interest: Both authors have completed the ICMJE uniform disclosure form (available at https://dx.doi.org/10.21037/jgo-21-255). The authors have no conflicts of interest to declare.
Ethical Statement: The authors are responsible for all aspects of the work to ensure that issues relating to the accuracy or completeness of any part of the work are properly investigated and resolved. Since this was a retrospective database study based on a public database, approval from the ethics committee was not required. The study was conducted in accordance with the Declaration of Helsinki (as revised in 2013).
Open Access Statement: This is an Open Access article distributed in accordance with the Creative Commons Attribution-NonCommercial-NoDerivs 4.0 International License (CC BY-NC-ND 4.0), which permits the non-commercial replication and distribution of the article with the strict proviso that no changes or edits are made and the original work is properly cited (including links to both the formal publication through the relevant DOI and the license). See: https://creativecommons.org/licenses/by-nc-nd/4.0/.
References
- Siegel RL, Miller KD, Goding Sauer A, et al. Colorectal cancer statistics, 2020. CA Cancer J Clin 2020;70:145-64. [Crossref] [PubMed]
- Modest DP, Pant S, Sartore-Bianchi A. Treatment sequencing in metastatic colorectal cancer. Eur J Cancer 2019;109:70-83. [Crossref] [PubMed]
- Piawah S, Venook AP. Targeted therapy for colorectal cancer metastases: A review of current methods of molecularly targeted therapy and the use of tumor biomarkers in the treatment of metastatic colorectal cancer. Cancer 2019;125:4139-47. [Crossref] [PubMed]
- Vassos N, Piso P. Metastatic Colorectal Cancer to the Peritoneum: Current Treatment Options. Curr Treat Options Oncol 2018;19:49. [Crossref] [PubMed]
- Jiao Q, Ren Y, Ariston Gabrie AN, et al. Advances of immune checkpoints in colorectal cancer treatment. Biomed Pharmacother 2020;123:109745 [Crossref] [PubMed]
- Bailey CE, Hu CY, You YN, et al. Increasing disparities in the age-related incidences of colon and rectal cancers in the United States, 1975-2010. JAMA Surg 2015;150:17-22. [Crossref] [PubMed]
- Dayde D, Tanaka I, Jain R, et al. Predictive and Prognostic Molecular Biomarkers for Response to Neoadjuvant Chemoradiation in Rectal Cancer. Int J Mol Sci 2017;18:573. [Crossref] [PubMed]
- De Felice F, Crocetti D, Maiuri V, et al. Locally Advanced Rectal Cancer: Treatment Approach in Elderly Patients. Curr Treat Options Oncol 2020;21:1. [Crossref] [PubMed]
- Oh HH, Joo YE. Novel biomarkers for the diagnosis and prognosis of colorectal cancer. Intest Res 2020;18:168-83. [Crossref] [PubMed]
- Galon J, Angell HK, Bedognetti D, et al. The continuum of cancer immunosurveillance: prognostic, predictive, and mechanistic signatures. Immunity 2013;39:11-26. [Crossref] [PubMed]
- Shen Y, Guan Y, Hummel JJ, et al. Immunogenomic pathways associated with cytotoxic lymphocyte infiltration and survival in colorectal cancer. BMC Cancer 2020;20:124. [Crossref] [PubMed]
- Kolde R, Laur S, Adler P, et al. Robust rank aggregation for gene list integration and meta-analysis. Bioinformatics 2012;28:573-80. [Crossref] [PubMed]
- Niu J, Yan T, Guo W, et al. Identification of Potential Therapeutic Targets and Immune Cell Infiltration Characteristics in Osteosarcoma Using Bioinformatics Strategy. Front Oncol 2020;10:1628. [Crossref] [PubMed]
- Chin CH, Chen SH, Wu HH, et al. cytoHubba: identifying hub objects and sub-networks from complex interactome. BMC Syst Biol 2014;8:S11. [Crossref] [PubMed]
- Xu H, Ma Y, Zhang J, et al. Identification and Verification of Core Genes in Colorectal Cancer. Biomed Res Int 2020;2020:8082697 [Crossref] [PubMed]
- Pattison AM, Merlino DJ, Blomain ES, et al. Guanylyl cyclase C signaling axis and colon cancer prevention. World J Gastroenterol 2016;22:8070-7. [Crossref] [PubMed]
- Meyer RD, Zou X, Ali M, et al. TMIGD1 acts as a tumor suppressor through regulation of p21Cip1/p27Kip1 in renal cancer. Oncotarget 2018;9:9672-84. [Crossref] [PubMed]
- Bhutia YD, Babu E, Ramachandran S, et al. SLC transporters as a novel class of tumour suppressors: identity, function and molecular mechanisms. Biochem J 2016;473:1113-24. [Crossref] [PubMed]
- Koslowski M, Sahin U, Dhaene K, et al. MS4A12 is a colon-selective store-operated calcium channel promoting malignant cell processes. Cancer Res 2008;68:3458-66. [Crossref] [PubMed]
- Chen L, Lu D, Sun K, et al. Identification of biomarkers associated with diagnosis and prognosis of colorectal cancer patients based on integrated bioinformatics analysis. Gene 2019;692:119-25. [Crossref] [PubMed]
- Chen H, Liu Y, Jiang CJ, et al. Calcium-Activated Chloride Channel A4 (CLCA4) Plays Inhibitory Roles in Invasion and Migration Through Suppressing Epithelial-Mesenchymal Transition via PI3K/AKT Signaling in Colorectal Cancer. Med Sci Monit 2019;25:4176-85. [Crossref] [PubMed]
- Kim SB, Calmet FH, Garrido J, et al. Sucrase-Isomaltase Deficiency as a Potential Masquerader in Irritable Bowel Syndrome. Dig Dis Sci 2020;65:534-40. [Crossref] [PubMed]
- Wei FZ, Mei SW, Wang ZJ, et al. Differential Expression Analysis Revealing CLCA1 to Be a Prognostic and Diagnostic Biomarker for Colorectal Cancer. Front Oncol 2020;10:573295 [Crossref] [PubMed]
- Chow PH, Bowen J, Yool AJ. Combined Systematic Review and Transcriptomic Analyses of Mammalian Aquaporin Classes 1 to 10 as Biomarkers and Prognostic Indicators in Diverse Cancers. Cancers (Basel) 2020;12:1911. [Crossref] [PubMed]
- Zhang X, Zhang H, Shen B, et al. Chromogranin-A Expression as a Novel Biomarker for Early Diagnosis of Colon Cancer Patients. Int J Mol Sci 2019;20:2919. [Crossref] [PubMed]
- Yang H, Lu Y, Lan W, et al. Down-regulated Solute Carrier Family 4 Member 4 Predicts Poor Progression in Colorectal Cancer. J Cancer 2020;11:3675-84. [Crossref] [PubMed]
- Liu BX, Huang GJ, Cheng HB. Comprehensive Analysis of Core Genes and Potential Mechanisms in Rectal Cancer. J Comput Biol 2019;26:1262-77. [Crossref] [PubMed]
- Zhuo C, Wu X, Li J, et al. Chemokine (C-X-C motif) ligand 1 is associated with tumor progression and poor prognosis in patients with colorectal cancer. Biosci Rep 2018;38:BSR20180580 [Crossref] [PubMed]
- Choe EK, Yi JW, Chai YJ, et al. Upregulation of the adipokine genes ADIPOR1 and SPP1 is related to poor survival outcomes in colorectal cancer. J Surg Oncol 2018;117:1833-40. [Crossref] [PubMed]
- Gutfeld O, Prus D, Ackerman Z, et al. Expression of serum amyloid A, in normal, dysplastic, and neoplastic human colonic mucosa: implication for a role in colonic tumorigenesis. J Histochem Cytochem 2006;54:63-73. [Crossref] [PubMed]
- Hansen MT, Forst B, Cremers N, et al. A link between inflammation and metastasis: serum amyloid A1 and A3 induce metastasis, and are targets of metastasis-inducing S100A4. Oncogene 2015;34:424-35. [Crossref] [PubMed]
- Zhu J, Chen X, Liao Z, et al. TGFBI protein high expression predicts poor prognosis in colorectal cancer patients. Int J Clin Exp Pathol 2015;8:702-10. [PubMed]
- Zhang Z, Wang Y, Zhang J, et al. COL1A1 promotes metastasis in colorectal cancer by regulating the WNT/PCP pathway. Mol Med Rep 2018;17:5037-42. [Crossref] [PubMed]
- Hou L, Liu P, Zhu T. Long noncoding RNA SLC30A10 promotes colorectal tumor proliferation and migration via miR-21c/APC axis. Eur Rev Med Pharmacol Sci 2020;24:6682-91. [PubMed]
- Yamaguchi N, Weinberg EM, Nguyen A, et al. PCK1 and DHODH drive colorectal cancer liver metastatic colonization and hypoxic growth by promoting nucleotide synthesis. Elife 2019;8:52135. [Crossref] [PubMed]
- Li W, Jin X, Guo S, et al. Comprehensive analysis of prognostic immune-related genes in the tumor microenvironment of colorectal cancer. Aging (Albany NY) 2021;13:5506-24. [Crossref] [PubMed]
- Jochems C, Schlom J. Tumor-infiltrating immune cells and prognosis: the potential link between conventional cancer therapy and immunity. Exp Biol Med (Maywood) 2011;236:567-79. [Crossref] [PubMed]
- Roelands J, Kuppen PJK, Vermeulen L, et al. Immunogenomic Classification of Colorectal Cancer and Therapeutic Implications. Int J Mol Sci 2017;18:2229. [Crossref] [PubMed]
(English Language Editor: J. Reynolds)