Differential analysis of RNA methylation regulators in gastric cancer based on TCGA data set and construction of a prognostic model
Introduction
In recent years, many studies have shown that RNA methylation is closely related to the occurrence and development of tumors. The modification level of transcription methylation is dynamically regulated by methyltransferases (encoders), binding proteins (readers), and demethylases (decoders). Abnormal regulation of these three molecules can lead to the generation and progression of tumors. The m1A, m5C, and m6A are three common methods of RNA methylation. The m6A is the most common type of modification of mRNA. It has been widely studied in glioblastoma, colorectal cancer, pancreatic cancer, hepatocellular carcinoma, acute lymphoblastic leukemia, and other malignant tumors, and was verified to play an important role in regulating the pluripotency of tumor stem cells, tumor proliferation, tumor metastasis, and tumor-related immunity (1). In contrast, m5C and m1A have been rarely studied, but have been identified in rRNAs, tRNAs, and most recently mRNAs, especially m5C enrichment near untranslated and argonaute binding regions (2). In circulating tumor cells of lung cancer patients, RNA m5C levels were elevated compared to whole blood cells. In addition, it has been reported that decreased expression of m1A methylated binding protein TRMT61B was observed in ER negative breast cancer (3).
Therefore, in this study, we used bioinformatics to classify these three common RNA methylation modification methods, aiming to analyze the correlation between the expression of RNA methylation regulators and the clinical characteristics of gastric cancer. Furthermore, key molecules were selected to construct several reliable prognostic risk models for gastric cancer patients. This study provides a new approach for the establishment of a prognostic risk model of gastric cancer patients and lays the foundation for mechanistic explorations of RNA methylation diversification modification of gastric cancer development and progression. We present the following article in accordance with the TRIPOD reporting checklist (available at https://dx.doi.org/10.21037/jgo-21-325).
Methods
Acquisition of data
RNA sequencing (RNA-seq) transcriptome data of gastric cancer samples and normal control samples, as well as corresponding clinical information data were downloaded from The Cancer Genome Atlas (TCGA) (n=407) of the National Cancer Institute Genome Data Commons (NCI-GDC) (https://gdc.cancer.gov). A total of 32 precancerous tissues and 375 cancerous tissues were included for subsequent analysis. Clinical information included age, sex, grade, stage, overall survival (OS) time, and survival status.
Data acquisition and differential analysis of RNA methylation regulators
A total of 30 known m1A, m5C, and m6A RNA methylated modulators were selected through a review of the latest literature. There were 6 m1A methylated internodes, including 3 encoders (TRMT61A, TRMT61B, and TRMT10C), 1 code reader (YTHDF3), and 2 eliminate decoders (ALKBH3 and ALKBH1). A total of 8 m5C methylated modulators were obtained, including 5 encoders (NSUN6, NSUN2, DNMT2, DNMT3B, and NOP2), 1 code reader (YBX1), and 2 eliminate decoders (TET3 and TET1). Furthermore, 18 m6A methylated modulators were selected, including 7 encoders (METTL16, METTL5, METTL3, RBM15B, RBM15, ZCCHC4, and KIAA1429), 8 code readers (YTHDF3, HNRNPG, HNRNPA2B1, IGF2BP3, IGF2BP2, IGF2BP1, PRRC2A, and EIF3A), and 3 eliminate decoders (FTO, ALKBH5, and ALKBH3). According to the data of gastric cancer tissues and paracancerous tissues obtained by TCGA, the differences were analyzed by the R package, and violin diagrams and heat maps were generated to preliminarily analyze the role in gastric cancer progression.
Construction and evaluation of the least absolute shrinkage and selection operator (LASSO) Cox regression risk proportional model
To determine the prognostic value of these RNA methylation regulators in gastric cancer, we extracted the transcriptional data of 30 methylation regulator RNA sequences. After integrating this data with the clinical data obtained from TCGA, we established the LASSO Cox regression model for m1a, m5C, m6A, and the total 30 genes. All factors related to RNA methylation were included in the LASSO Cox regression model. The risk prediction model was established by using the LASSO algorithm, and based on the results, patients were classified into a high-risk group or low-risk group. The risk score was calculated by the following formula: , where Coefi is the coefficient, and xi is the expression value for each selected molecule. Using survival data, gastric cancer patients were classified into a high-risk group or low-risk group according to the median risk score, which was then was used to draw the survival curve. The differences in OS between the high-risk group and the low-risk group were calculated by the Kaplan-Meier method and the two-way log-rank test. To evaluate the predictive efficiency of the model, we constructed the receiver operating characteristic (ROC) curve, and cluster heat maps were used to assess the correlations between differential genes and the distribution of clinical data in cancer and adjacent tissues. Univariate and multivariate Cox regression were used to analyze the independent prognostic effects of risk scores.
Gene set enrichment analysis (GSEA) based on TCGA differential genes
In order to clarify the functional influence of differentially expressed genes, gene function analysis was carried out for differentially obtained genes from TCGA database through the R package, and multiple GSEAs were performed.
Construction and analysis of a prognostic model for the risk of RNA methylation in Asians
The samples from the TCGA database mainly come from whites and African Americans, and there are few studies on other races. Therefore, we conducted a separate analysis on the data of Asians to clarify the predictive value of the methylation prognostic model for Asians. Download the expression data and clinical information of Asian gastric cancer and adjacent tissues in the TCGA database. Also based on the above 30 RNA methylation genes, using the same method described above, carry out the difference analysis and the construction and evaluation of the four risk prediction models of m1A, m5C, m6A and the total.
Statistical analysis
The Wilcoxon rank sum test was used to compare the differences in gene expression between normal tissues and tumor tissues. The LASSO Cox regression algorithm was used to establish the risk prognosis model. The OS rates of the high-risk group and low-risk group were determined by the Kaplan-Meier method. An ROC curve was used to test the accuracy of risk score prediction. Univariate and multivariate Cox regression analyses were used to evaluate the risk of value at risk (VAR) and clinicopathological features in the prognosis of gastric cancer. The Chi square test was used to compare the differences in risk gene expression between the different risk groups and clinical stages. All statistical analyses were performed by SPSS 19.0 and GraphPad Prism 7.0 software. P<0.05 was statistically significant.
The study was conducted in accordance with the Declaration of Helsinki (as revised in 2013).
Results
Differential analysis of the expression of RNA methylated modulators
Based on the data of gastric cancer and paracancerous tissues extracted from TCGA, the expression differences of 3 methylation-related genes (m1A, m5C, and m6A) were analyzed by the R package. The results revealed significant differences in the expression levels of most genes between cancer tissues and adjacent tissues in each group (Figure 1A-1F). At the same time, significant differences were also observed in the expression of 30 methylation-related genes between cancer tissues and adjacent tissues (Figure 1G,1H).
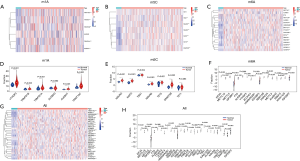
Construction of the prognostic risk model
We used the LASSO algorithm to screen 3 methylation regulators (FTO, ALKBH5, and RBM15) from the above genes, and established the risk prediction model of m6A (Figure 2A-2C). Five methylation regulators (FTO, ALKBH5, TRMT61B, RBM15, and YXB1) were screened from the total 30 genes to establish a methylation risk prediction model (Figure 2D-2F). According to the median risk value, gastric cancer patients were divided into a high-risk group and low-risk group. However, the key gene set of m1A and m5C methylation regulators could not be obtained by LASSO Cox regression analysis, so it was impossible to construct further prognostic risk models for m1A and m5C molecules.
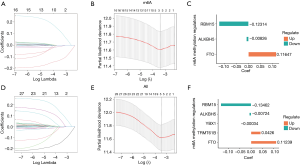
Prognostic survival analysis and ROC curves of gastric cancer patients in the high- and low-risk groups
We calculated the OS difference between the high-risk group and low-risk group by the Kaplan-Meier method and performed the two-way log-rank test, then generated the survival curves. The survival rate of gastric cancer patients in the low-risk group of the two models was significantly higher than that in the high-risk group (P<0.05). The ROC curve was constructed and indicated that the predictive efficiency of the model was good [area under the ROC curve (AUC) >0.6] (Figure 3A-3D).
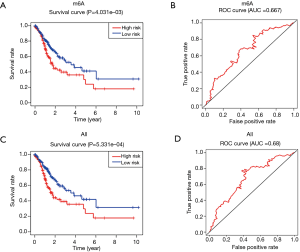
The differences in clinicopathological features and the prognosis of gastric cancer patients in different risk groups
Cluster analysis was used to analyze the differences in clinicopathological characteristics, survival prognosis, and the expression of regulatory factors in different risk groups. The results showed that FTO was highly expressed in the high-risk group, while ALKBH5 and RBM15 were lowly expressed in the high-risk group. In the overall prognosis model, FTO was highly expressed in the high-risk group, while TRMT61B, YBX1, ALKBH5, and RBM15 were lowly expressed in the high-risk group. Further analysis showed that there were significant differences in T stage (P<0.01) and grade (P<0.05) between different risk groups of the two models (Figure 4A,4B).
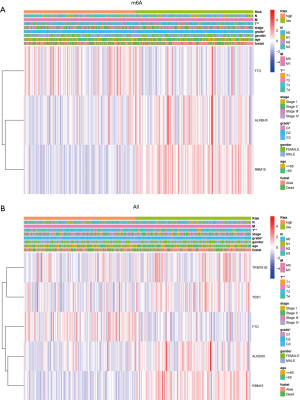
Univariate and multivariate Cox regression analyses
Univariate and multivariate Cox regression analyses were used to analyze the clinicopathological characteristics and risk values of gastric cancer patients in TCGA database. Based on the two methylation risk prognosis models of m6A and the whole population, univariate analysis showed that age (P<0.05), stage (P<0.001), T stage (P<0.05), N stage (P<0.001), and risk value (P<0.001) were significantly correlated with the OS rate (Figure 5A,5B). Multivariate Cox regression analysis showed that age (P<0.001) and risk value (P<0.001) were significantly correlated with the OS rate (P<0.001) (Figure 5C,5D). Univariate and multivariate Cox regression analysis showed that the risk score had an independent prognostic effect (P<0.001) (Figure 5C,5D). The above results suggested that risk value could be used as an independent prognostic factor for gastric cancer.
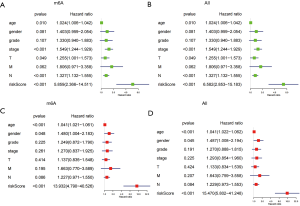
GSEA
GSEA was performed based on m6A and the three RNA methylation-related genes. Overall, gene sets related to the cell cycle, homologous recombination, RNA degradation, and P53 signaling pathways were significantly enriched in the low-risk group. Calcium signaling pathways, neuroactive ligand-receptor interactions, ECM receiver interactions, and vascular smooth muscle contractile protein kinase active were significantly enriched in the high-risk group (Figure 6A,6B).
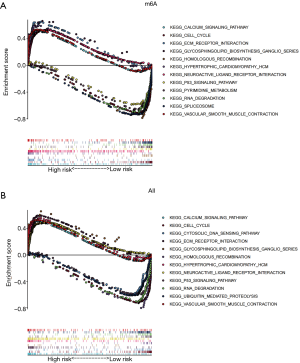
Construction and analysis of a prognostic model for the risk of RNA methylation in Asians
The transcriptome data of Asian gastric cancer tissues and adjacent tissues downloaded from the TCGA database were used to analyze differences and construct prognostic models. The results suggest that the m1A RNA methylation model cannot effectively assess the prognosis of patients, while the survival analysis and multivariate regression analysis of the m5C (DNMT3B, YBX1 and NOP2), m6A (IGF2BP3, ZCCHC4, METTL5, RBM15B, PRRC2A, RBM15, KIAA1429, FTO, IGF2BP1, METTL3, ALKBH5, IGF2BP2 and EIF3A) and overall (FTO, HNRNPA2B1, METTL3 and NOP2) three RNA methylation-related risk models have confirmed that their risk values can be used as predictors of the survival of patients with gastric cancer.(Figure 7A-7C).
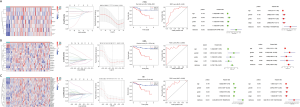
Discussion
Epigenetics regulates the expression of eukaryotic genes and is closely related to major human diseases, such as tumors, neurodegenerative diseases, and autoimmune diseases. It has also been considered as one of the most concerned topics in biological research (4). Methylation is a very important way of epigenetic modification, which can occur at any stage of gene expression, not only at the gene and protein level, but RNA can also undergo methylation modification (5,6). At present, its research technology is relatively mature, and a lot of data related to methylation has been unearthed (7,8). In recent years, with the continuous deepening of DNA methylation and histone methylation, m6A has not only opened a new era of post-transcriptional gene regulation in eukaryotes, but has quickly become a research hotspot in the field of RNA methylation modification (9). Immediately, m6A methylation modification, m5C methylation modification, and m1A methylation modification have been proved to play an important role in the regulation of a series of malignant biological behaviors such as tumor cell proliferation, invasion, and metastasis. Epigenetic silencing is almost a universal feature of human malignant tumors, and its impact involves all key signaling pathways from tumor initiation to development (10). Therefore, targeted epigenetic changes have great potential. A variety of targeted epigenetic drugs have begun clinical trials, especially DNA methyltransferase inhibitors that have achieved outstanding efficacy in some blood-borne tumors and solid tumors. For example, Decitabine is a natural 2'-deoxycytidine adenosine analogue that inhibits DNA methyltransferase and reduces DNA methylation, thereby inhibiting tumor cell proliferation and preventing the occurrence of drug resistance. It is suitable for the treatment of myelodysplastic syndrome (11). EZH2 inhibitors and DOT1L inhibitors and other histone methylation inhibitors have been approved or are undergoing clinical trials (12). Nowadays, scientific research results and transformation cases in this field are pouring out, and it can be foreseen that the application of epigenetic laws in clinical diagnosis and treatment will be more extensive in the future. In this study, we combined gastric cancer transcriptome data with clinical data, and 3 m6A methylation regulators (FTO, ALKBH5, and RBM15) and global methylation regulators (RBM15, ALKBH5, YXB1, TRMT61B, and FTO) related to prognosis were screened by LASSO Cox regression analysis. A prognostic risk model was constructed which showed a good correlation of clinical characteristics with gastric cancer.
RNA m1A methylation is a recently discovered mRNA modification of N1-methyladenosine that affects the localization, stability, translation, and splicing of mRNAs (13), and m1A methylation proceeds depending on the TRMT6/TRMT61A methyltransferase complex (14). TRMT61A has the catalytic activity of tRNA adenine-N1-methyltransferase, and TRMT6 plays an important role in tRNA binding (15). In addition, TRMT10C and TRMT61B were reported to catalyze m1A at positions 9 and 58 of mitochondrial tRNAs (16,17). Studies have shown that consumption of TRM6/61 reduces glioma cell proliferation and increases death. In addition, compared to grade II/III glioblastoma, TRM6/TRM61 mRNA expression was significantly up-regulated in highly aggressive glioblastoma (18). TRMT10C is another important encoder of the m1A methylation modification process. Its recessive mutation will reduce the stability of the MRPP1 protein and promote defects in RNA processing, leading to the occurrence of mitochondrial diseases (19). However, there are fewer studies related to RNA m1A methylation of gastric cancer. The current study found that, compared to normal tissue, TRMT61A, TRMT61B, and TRMT10C were significantly overexpressed in gastric cancer. In the overall methylation prognostic model based on TRMT61B, the survival rate of the low-risk group was significantly higher than that of the high-risk group, and the risk value could be used as an independent risk factor for the prognosis of gastric cancer. These results indicate that TRMT61B gene expression plays a role in the development of gastric cancer, and may indicate a poor clinical outcome in patients with gastric cancer. GSEA results showed that gene sets related to the cell cycle, RNA degradation, the cytosolic DNA-sensing pathway, and P53 signaling pathways were significantly enriched in the low-risk group, suggesting their potential role in tumorigenesis.
RNA m5C methylation means that the fifth position C of the RNA cytosine is modified by methylation. The m5C methylation was first discovered in rRNA and then in mRNA. In eukaryotes, C5 methylation of the RNA cytosine is catalyzed by the NOL1/NOP2/SUN domain (NSUN) family of enzymes and the DNA methyltransferase homolog DNMT2 (20-22). Research by Okamoto et al. (23) showed that the protein expression of NSUN2 is elevated in a variety of cancers, such as esophageal, gastric, pancreatic, and breast cancer. The m5C methyltransferase NOP2/NSUN2 in gallbladder cancer promotes tumor cell proliferation and tumorigenesis in vitro and in vivo through close cooperation with RPL6 (24). LncRNA-hPVT1 promotes cell proliferation, the cell cycle, and stem-like properties of HCC cells by stabilizing the NOP2 protein (25). In addition, the loss of NSUN2 methylation modification function can also lead to neurodevelopmental disorders (26). Methyltransferase DNMT3B has been confirmed to be overexpressed in colorectal, prostate, and breast cancer, and is significantly associated with poor prognosis (27). DNMT3B -579G>T polymorphism is significantly related to the risk of gastric cancer in the Chinese population, but is not related to the risk of lung cancer. The DNMT3B polymorphism may predict the long-term survival of gastric cancer. However, further research is needed to reveal the potential biological role of the DNMT3B polymorphism (28). In addition, YBX1 is a newly discovered m5C code reader, which has been proven to regulate the stability of intracellular mRNA (29). In bladder cancer, YBX1 is recognized by an indole ring of W65 in its cold shock domain (CDS) (23) and binds to m5C-modified mRNA (30). Our study found that NOP2, YBX1, NSUN2, and DNMT3B were significantly overexpressed in gastric cancer compared with normal tissues. In the overall methylation risk model including YBX1, the survival rate of gastric cancer patients in the low-risk group was significantly higher than that in the high-risk group. This result is consistent with the conclusions of the above research results. Evidently, YBX1 is indeed involved in the occurrence of gastric cancer, and its high expression may indicate a poor clinical outcome of gastric cancer patients.
RNA m6A methylation is the most common post-transcriptional modification of RNA and plays a key role in cancer pathogenesis. A study by Strick et al. (31) showed that decreased mRNA levels of ALKBH5 and fat mass and obesity-related protein (FTO) are related to shortened OS and cancer-specific survival after nephrectomy, and m6A methylated silencers ALKBH5 and FTO are clear renal cancer prognostic biomarkers. Zhang et al. (32) suggested that ALKBH5 promotes gastric cancer invasion and metastasis by demethylating lncRNA NEAT1, which may be a potential therapeutic target for gastric cancer. Yang et al. (33) revealed that m6A mRNA demethylation through FTO increased melanoma growth and reduced the response to anti-PD-1 immunotherapy. Knockout of FTO can increase m6A methylation in endogenous genes of important tumorigenic melanoma cells including PD-1 (PDCD1), CXCR4, and SOX10, resulting in increased RNA attenuation caused by the m6A reader YTHDF2, and increased sensitization of melanoma to anti-PD-1 treatment in mice. Our study found that compared with normal tissues, FTO, ALKBH5, and RBM15 had higher expression in gastric cancer tissues. In the model constructed based on the 3 genes, the survival rate of gastric cancer patients in the low-risk group was significantly higher than that in the high-risk group. FTO, ALKBH5, and RBM15 m6A RNA methylation-modified genes play important roles in the development of gastric cancer. GSEA results showed that gene sets related to pathways such as the cell cycle, RNA degradation, and the P53 signaling pathway were significantly enriched in the low-risk group, suggesting their possible role in tumorigenesis.
In this study, using bioinformatics, two prognostic risk prediction models based on m6A methylation regulators and m6A, m5C, and m1A methylation regulators were established. And we initially conducted a separate analysis for a small amount of Asian methylation-related data in the data. Gastric cancer patients can be effectively divided into high-risk groups and low-risk groups based on methylation-related genes, but there are still many shortcomings. Firstly, this research is based on database mining, lacking our own verification data and prospective experimental research, and the results of the analysis have not yet been reported in gastric cancer, requiring further exploration. Secondly, all the data in this study comes from the TCGA database. The samples included in this database are mainly from whites and African Americans. There are few studies on other races. It is not clear whether our current prognostic model is applicable to other races. Therefore, in further research, we will focus on the Asian population and collect more multicenter gastric cancer cases to improve the accuracy of the prediction model. Moreover, there is currently no unified prognostic model used in clinical practice, and the clinical application of the risk assessment model established in this study needs to be further explored. However, the risk assessment model still has a certain value for cancer patients. The model can intuitively distinguish between high- and low-risk patients, thereby providing individualized treatment plans.
Conclusions
The abnormal expression of m1A, m5C, and m6A RNA methylation regulators in gastric cancer and adjacent tissue samples may play an important role in the development of gastric cancer. The tumor model we constructed can effectively predict the prognosis of patients with gastric cancer. This study is expected to stratify the prognosis of gastric cancer, and provide a reference for the verification of related prognostic molecules and the therapeutic targets of gastric cancer. However, our research results need to be fully verified and explored in further clinical studies.
Acknowledgments
Funding: This work was supported financially by the open fund from the Key Laboratory of Ministry of Education for TCM Viscera-State Theory and Applications, Liaoning University of Traditional Chinese Medicine (zyzx1710) and Science and Technology Plan Project of Shenyang City (19-112-4-079).
Footnote
Reporting Checklist: The authors have completed the TRIPOD reporting checklist. Available at https://dx.doi.org/10.21037/jgo-21-325
Conflicts of Interest: All authors have completed the ICMJE uniform disclosure form (available at https://dx.doi.org/10.21037/jgo-21-325). The authors have no conflicts of interest to declare.
Ethical Statement: The authors are accountable for all aspects of the work in ensuring that questions related to the accuracy or integrity of any part of the work are appropriately investigated and resolved. The study was conducted in accordance with the Declaration of Helsinki (as revised in 2013). Institutional ethical approval and informed consent were waived.
Open Access Statement: This is an Open Access article distributed in accordance with the Creative Commons Attribution-NonCommercial-NoDerivs 4.0 International License (CC BY-NC-ND 4.0), which permits the non-commercial replication and distribution of the article with the strict proviso that no changes or edits are made and the original work is properly cited (including links to both the formal publication through the relevant DOI and the license). See: https://creativecommons.org/licenses/by-nc-nd/4.0/.
References
- Pan Y, Ma P, Liu Y, et al. Multiple functions of m6A RNA methylation in cancer. J Hematol Oncol 2018;11:48. [Crossref] [PubMed]
- Chen X, Sun YZ, Liu H, et al. RNA methylation and diseases: experimental results, databases, Web servers and computational models. Brief Bioinform 2019;20:896-917. [Crossref] [PubMed]
- Couch FJ, Kuchenbaecker KB, Michailidou K, et al. Identification of four novel susceptibility loci for oestrogen receptor negative breast cancer. Nat Commun 2016;7:11375. [Crossref] [PubMed]
- Sharma S, Kelly TK, Jones PA. Epigenetics in cancer. Carcinogenesis 2010;31:27-36. [Crossref] [PubMed]
- Li X, Yang X, Fan Y, et al. A ten-gene methylation signature as a novel biomarker for improving prediction of prognosis and indicating gene targets in endometrial cancer. Genomics 2021;113:2032-44. [Crossref] [PubMed]
- Hashimoto H, Vertino PM, Cheng X. Molecular coupling of DNA methylation and histone methylation. Epigenomics 2010;2:657-69. [Crossref] [PubMed]
- Ovcharenko A, Rentmeister A. Emerging approaches for detection of methylation sites in RNA. Open Biol 2018;8:180121 [Crossref] [PubMed]
- Bibikova M, Fan JB. Genome-wide DNA methylation profiling. Wiley Interdiscip Rev Syst Biol Med 2010;2:210-23. [Crossref] [PubMed]
- Liu ZX, Li LM, Sun HL, et al. Link Between m6A Modification and Cancers. Front Bioeng Biotechnol 2018;6:89. [Crossref] [PubMed]
- Miranda Furtado CL, Dos Santos Luciano MC, Silva Santos RD, et al. Epidrugs: targeting epigenetic marks in cancer treatment. Epigenetics 2019;14:1164-76. [Crossref] [PubMed]
- Gnyszka A, Jastrzebski Z, Flis S. DNA methyltransferase inhibitors and their emerging role in epigenetic therapy of cancer. Anticancer Res 2013;33:2989-96. [PubMed]
- Morera L, Lübbert M, Jung M. Targeting histone methyltransferases and demethylases in clinical trials for cancer therapy. Clin Epigenetics 2016;8:57. [Crossref] [PubMed]
- Dominissini D, Nachtergaele S, Moshitch-Moshkovitz S, et al. The dynamic N(1)-methyladenosine methylome in eukaryotic messenger RNA. Nature 2016;530:441-6. [Crossref] [PubMed]
- Li X, Xiong X, Zhang M, et al. Base-Resolution Mapping Reveals Distinct m1A Methylome in Nuclear- and Mitochondrial-Encoded Transcripts. Mol Cell 2017;68:993-1005.e9. [Crossref] [PubMed]
- Anderson J, Phan L, Hinnebusch AG. The Gcd10p/Gcd14p complex is the essential two-subunit tRNA(1-methyladenosine) methyltransferase of Saccharomyces cerevisiae. Proc Natl Acad Sci U S A 2000;97:5173-8. [Crossref] [PubMed]
- Vilardo E, Nachbagauer C, Buzet A, et al. A subcomplex of human mitochondrial RNase P is a bifunctional methyltransferase--extensive moonlighting in mitochondrial tRNA biogenesis. Nucleic Acids Res 2012;40:11583-93. [Crossref] [PubMed]
- Chujo T, Suzuki T. Trmt61B is a methyltransferase responsible for 1-methyladenosine at position 58 of human mitochondrial tRNAs. RNA 2012;18:2269-76. [Crossref] [PubMed]
- Macari F, El-Houfi Y, Boldina G, et al. TRM6/61 connects PKCα with translational control through tRNAi(Met) stabilization: impact on tumorigenesis. Oncogene 2016;35:1785-96. [Crossref] [PubMed]
- Metodiev MD, Thompson K, Alston CL, et al. Recessive Mutations in TRMT10C Cause Defects in Mitochondrial RNA Processing and Multiple Respiratory Chain Deficiencies. Am J Hum Genet 2016;98:993-1000. [Crossref] [PubMed]
- Khoddami V, Cairns BR. Identification of direct targets and modified bases of RNA cytosine methyltransferases. Nat Biotechnol 2013;31:458-64. [Crossref] [PubMed]
- Yang X, Yang Y, Sun BF, et al. 5-methylcytosine promotes mRNA export - NSUN2 as the methyltransferase and ALYREF as an m5C reader. Cell Res 2017;27:606-25. [Crossref] [PubMed]
- Frye M, Watt FM. The RNA methyltransferase Misu (NSun2) mediates Myc-induced proliferation and is upregulated in tumors. Curr Biol 2006;16:971-81. [Crossref] [PubMed]
- Okamoto M, Hirata S, Sato S, et al. Frequent increased gene copy number and high protein expression of tRNA (cytosine-5-)-methyltransferase (NSUN2) in human cancers. DNA Cell Biol 2012;31:660-71. [Crossref] [PubMed]
- Gao Y, Wang Z, Zhu Y, et al. NOP2/Sun RNA methyltransferase 2 promotes tumor progression via its interacting partner RPL6 in gallbladder carcinoma. Cancer Sci 2019;110:3510-9. [Crossref] [PubMed]
- Wang F, Yuan JH, Wang SB, et al. Oncofetal long noncoding RNA PVT1 promotes proliferation and stem cell-like property of hepatocellular carcinoma cells by stabilizing NOP2. Hepatology 2014;60:1278-90. [Crossref] [PubMed]
- Flores JV, Cordero-Espinoza L, Oeztuerk-Winder F, et al. Cytosine-5 RNA Methylation Regulates Neural Stem Cell Differentiation and Motility. Stem Cell Reports 2017;8:112-24. [Crossref] [PubMed]
- Wang SL, Huang Y, Su R, et al. Silencing long non-coding RNA HOTAIR exerts anti-oncogenic effect on human acute myeloid leukemia via demethylation of HOXA5 by inhibiting Dnmt3b. Cancer Cell Int 2019;19:114. [Crossref] [PubMed]
- Chen B, Wang J, Gu X, et al. The DNMT3B -579G>T Polymorphism Is Significantly Associated With the Risk of Gastric Cancer but not Lung Cancer in Chinese Population. Technol Cancer Res Treat 2017;16:1259-65. [Crossref] [PubMed]
- Yang Y, Wang L, Han X, et al. RNA 5-Methylcytosine Facilitates the Maternal-to-Zygotic Transition by Preventing Maternal mRNA Decay. Mol Cell 2019;75:1188-1202.e11. [Crossref] [PubMed]
- Chen X, Li A, Sun BF, et al. 5-methylcytosine promotes pathogenesis of bladder cancer through stabilizing mRNAs. Nat Cell Biol 2019;21:978-90. [Crossref] [PubMed]
- Strick A, von Hagen F, Gundert L, et al. The N6 -methyladenosine (m6 A) erasers alkylation repair homologue 5 (ALKBH5) and fat mass and obesity-associated protein (FTO) are prognostic biomarkers in patients with clear cell renal carcinoma. BJU Int 2020;125:617-24. [Crossref] [PubMed]
- Zhang J, Guo S, Piao HY, et al. ALKBH5 promotes invasion and metastasis of gastric cancer by decreasing methylation of the lncRNA NEAT1. J Physiol Biochem 2019;75:379-89. [Crossref] [PubMed]
- Yang S, Wei J, Cui YH, et al. m6A mRNA demethylase FTO regulates melanoma tumorigenicity and response to anti-PD-1 blockade. Nat Commun 2019;10:2782. [Crossref] [PubMed]