Identification of hub genes and functional modules in colon adenocarcinoma based on public databases by bioinformatics analysis
Introduction
Colorectal cancer (CRC) is one of the most common gastrointestinal malignancies. According to the Global Cancer Incidence, Mortality and Prevalence (GLOBOCAN 2020) database released by the International Agency for Research on Cancer (IARC), in 2020 there was an estimated 1,931,600 new cases of CRC and 935,200 related deaths worldwide. This makes CRC the third most prevalent type of cancer and the second most deadly among all malignant tumors (1). The distinction between the colon and the rectum is largely anatomical, but they have same implications for surgical and radiotherapeutic management that can impact prognosis (2). Rectal cancer is common in China, while colon cancer is common in the USA (3). Statistical data from the American Cancer Society (ACS) shows that of the 147,950 patients diagnosed with CRC in 2020, 70% had colon cancer (104,610 patients), while only 30% had rectal cancer (43,340 patients) (4). More than half of all these cases and related deaths were attributed to modifiable risk factors, such as smoking, an unhealthy diet, excessive alcohol consumption, physical inactivity, and obesity, meaning some CRC cases are potentially preventable (5). Consequently, the morbidity and mortality of CRC could be better mitigated by more frequent screening and monitoring (6).
The reason for CRC’s high mortality rate is that its early clinical symptoms are not distinct, resulting in it often being detected only in the middle or late stages. It has been noted that the 5-year survival rate of CRC patients diagnosed in the early stage of the disease is around 90%, while that of patients diagnosed in the late stage is as low as 5–10% (7). Due to the application of efficient screening methods, both the incidence and mortality of CRC in the USA have been decreasing, while the 5-year survival rate has increased significantly (8). Colonoscopy and abdominal imaging examination are costly, invasive or radiative, and frequent examination does increase the physical discomfort and economic burden of patients. The effectiveness, associated costs, and safety improve the patient acceptance of detecting tumor markers, and hence it could be used for CRC screening (9). Tumor markers in cells, tissues, body fluids, and excreta can be detected quantitatively or qualitatively. These markers have application value in assessing prognosis, selecting treatment options, predicting efficacy, and monitoring recurrence (10). Presently, some of the most commonly used tumor markers associated with diagnosing CRC include carcinoembryonic antigen (CEA), carbohydrate antigen 19-9 (CA19-9), carbohydrate antigen 242 (CA242), carbohydrate antigen 72-4 (CA72-4), and carbohydrate antigen 50 (CA50) (11). According to the US National Comprehensive Cancer Network (NCCN) guidelines, CEA is perhaps the most important tumor marker for CRC and is the only tumor marker recommended for routine screening of CRC (12). However, the sensitivity and specificity of tumor markers are not sufficiently high, and thus their application is limited (13). Therefore, it is necessary to identify and explore novel tumor markers to facilitate early diagnosis and prognosis monitoring of CRC. The aim of this study was thus to search for novel tumor markers for the treatment of colon adenocarcinoma (COAD). To this end, COAD RNA sequencing (RNA-seq) data were downloaded from the Cancer RNA-Seq Nexus (CRN) database, and those hub genes that figured prominently in the occurrence and prognosis of COAD were identified via bioinformatics as potential biomarkers. We present the following article in accordance with the MDAR reporting checklist (available at https://dx.doi.org/10.21037/jgo-21-415).
Methods
Data sources
The RNA-seq data of 439 COAD and 40 normal tissue samples were downloaded from the CRN database (http://syslab5.nchu.edu.tw/CRN/). This public database provides protein-coding transcript/long non-coding RNA (lncRNA) expression profiles and messenger RNA (mRNA)-lncRNA coexpression networks in cancer cells (14). In this study, the CRC RNA-seq dataset consisted of 10 subsets and 479 samples (Table 1).
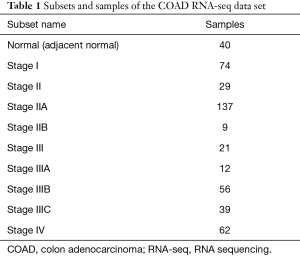
Full table
Identifying differentially expressed protein-coding genes
This study aimed to screen differentially expressed COAD protein-coding genes, which were obtained by comparing protein-coding genes in cancerous tissues at various stages to those in adjacent normal tissues. A Venn diagram was plotted using OmicShare tools, a free online platform for data analysis (http://www.omicshare.com/tools). As the CRN database has annotated, normalized and analyzed COAD RNA-seq data, we were also able to use this data directly for our research, with P values <0.01 being considered significant. The study was conducted in accordance with the Declaration of Helsinki (as revised in 2013).
Functional enrichment of differentially expressed protein-coding genes
The differentially expressed protein-coding genes were subjected to functional enrichment analysis in the Database for Annotation, Visualization and Integrated Discovery (DAVID), which provides a comprehensive set of functional annotation tools to understand the biological meaning behind large lists of genes (15). The results were plotted as bubble diagrams using the ggplot2 package in R. A P value <0.05 was set as the threshold for significance.
Identifying hub genes and functional modules among differentially expressed protein-coding genes
The Search Tool for the Retrieval of Interacting Genes (STRING) database was used to predict and visualize the protein-protein interaction (PPI) networks of the differentially expressed protein-coding genes we obtained (16). Experimentally validated interactions with a combined score of >0.4 were selected to construct the PPI networks through Cytoscape 3.8.2, which is an open-source software platform for integrating biomolecular interaction networks with high-throughput expression data and other molecular states into a unified conceptual framework (17). The Cytoscape plugin cytoHubba was used for screening hub genes according to node score rank (18), while the plugin ClueGO was used to decipher the functional ontology and pathway annotation networks of hub genes (19). The screening criteria were Gene Ontology-biological process (GO-BP) and pathways with P<0.05. Minimal Common Oncology Data Elements (MCODE) was used to detect densely connected modules in large PPI networks that may represent molecular complexes (20). The criteria were as follows: MCODE scores >3 and number of nodes >4. Kyoto Encyclopedia of Genes and Genomes (KEGG) pathway enrichment analysis was also used to analyze genes in the modules.
Verifying the expression of COAD hub genes
The expression of COAD hub genes was validated in the gene expression profiling interactive analysis (GEPIA) database according to the instructions on the platform. GEPIA is a newly developed interactive web server that uses a standard processing pipeline to analyze the RNA-seq expression data of 9,736 tumors and 8,587 normal samples from The Cancer Genome Atlas (TCGA) and genotype-tissue expression (GTEx) projects (21).
Analysis of the surviving hub genes
The survival data of the hub genes were downloaded from the OncoLnc database, a tool for interactively exploring survival correlations and for downloading clinical data coupled with the expression data of mRNAs, microRNAs (miRNAs), or lncRNAs (22). Based on the median values of the mRNA expression of hub genes, patients with COAD were divided into high and low expression groups. The log-rank (Mantel-Cox) test was used to analyze and draw survival curves.
Statistical analysis
GraphPad Prism 7 software (GraphPad Inc., San Diego, CA, USA) was used for statistical analysis. The Kaplan-Meier method was used to evaluate the survival of patients, and the log-rank (Mantel-Cox) test was used to assess the significance. An unpaired t-test and Mann-Whitney U test were used to analyze the expression of hub genes in the clinical stage of COAD. A P value <0.05 indicated a statistically significant difference.
Results
Identifying differentially expressed protein-coding transcripts
We retrieved and compared the differentially expressed protein-coding transcripts of COAD tissues at various stages to those of adjacent normal tissues. To ensure the reliability of the study, we selected and compared the overlapping differentially expressed protein-coding transcripts between each subgroup for our subsequent analysis. Following this, 2,460 protein-coding transcripts were identified (Figure 1).
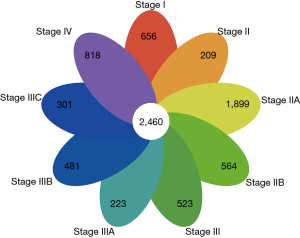
Functional enrichment of differentially expressed protein-coding genes
The 2,460 differentially expressed protein-coding transcripts were first identified and converted into official genes using the DAVID database. After conversion, 1,807 genes with official gene symbols were left. These 1,807 protein-coding genes were then subjected to functional enrichment analysis to determine their biological process (BP), cellular components (CC), and molecular function (MF) for GO and KEGG pathway. The top 10 hits of each item are presented as bubble plots (Figure 2). The results revealed that the functional differentially expressed protein-coding genes were associated with nervous system development, plasma membrane, adenylate cyclase (ADCY) binding, and calcium signaling pathway.

Identifying hub genes and functional modules among differentially expressed protein-coding genes
Based on the qualified network data provided by the STRING database, we used Cytoscape to reconstruct the networks. The plugin cytoHubba was used to calculate a node score by using the degree method, and the following top 10 genes were considered to be hub genes (Figure 3): MYC, SNAP25, ACTB, ADCY5, PTPRC, PIK3R1, ADCY9, GNG2, ADCY2, and GNG13. Among these genes, MYC had the highest node score of 126. Compared normal tissues, MYC was upregulated and the other hub genes were downregulated in COAD. Furthermore, we clarified all hub gene’s GO-BP using the ClueGO plugin. The results showed that the main BPs were response to platelet aggregation inhibitor, cellular response to alcohol, and cAMP biosynthetic process (Figure 4). The MCODE app was also used for screening functional modules in PPI networks. Based on the above screening criteria, 39 functional modules were identified. The top 3 modules were subjected to KEGG pathway functional enrichment analysis in the DAVID database (Figure 5).
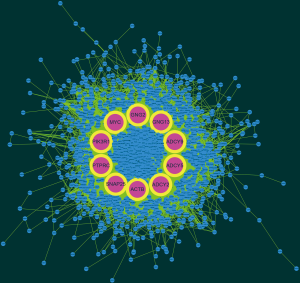
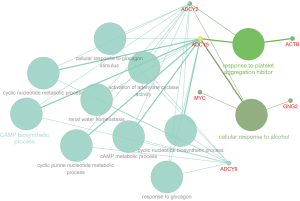
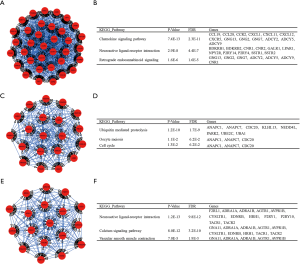
Verifying the expression of COAD hub genes
Based on the parameters, |log2FC| cutoff =1, P value cutoff =0.01, log scale “yes”, and jitter size =0.4, the expression of MYC in COAD tissues were found to be higher than that in normal tissues, while the expression of ADCY5, ADCY9, PTPRC, GNG2, GNG13, and SNAP25 was lower in COAD than in normal tissues (Figure 6, with P<0.05 indicating statistical significance).
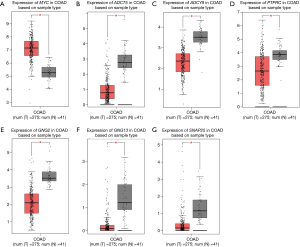
Survival analysis of the hub genes
The results of our hub gene survival analysis indicated that the low expressions of ADCY5 (P=0.0362), GNG2 (P=0.0065), and PTPRC (P=0.0023) were significantly associated with an improved COAD prognosis (Figure 7A-7C). Furthermore, we analyzed the expression levels of ADCY5, GNG2, and PTPRC in different clinical stages of COAD. The results showed that the expression level of ADCY5 in stages I/II was lower than that in stages III/IV, which could be attributed to the improved prognosis in stage I and II patients (P=0.01, Figure 7D-7F).
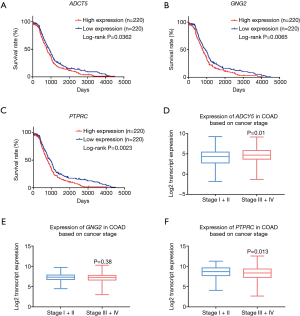
Discussion
Several freely available public databases, such as TCGA, gene expression omnibus (GEO), cBio Cancer Genomics Portal (cBioPortal), and catalogue of somatic mutations in cancer (COSMIC) hold massive amounts of RNA-seq data, methylation data, and copy number variation data (23-26). This data can be downloaded to conduct the secondary analysis of genes and publish further gene-related research. In this study, protein-coding transcript data and survival data were obtained from the CRN database and OncoLnc databases, respectively. The raw data from TCGA database was standardized, annotated, and analyzed in the CRN and OncoLnc databases. After preliminary processing of the original data, statistical analysis was performed in the CRN database to identify significant protein-coding transcript data (P<0.01). Subsequently, the data were classified and summarized according to clinical stages and made available for download (14). Therefore, the data information from the CRN database can be used directly. To ensure the reliability of the results, we selected the protein-coding transcripts common to all clinical stages for subsequent analyses.
In total, 2,460 protein-coding transcripts were identified, of which 1,807 protein-coding genes with official gene symbols were obtained after conversion. Cytoscape was then used to screen 10 hub genes: MYC, SNAP25, ACTB, ADCY5, PTPRC, PIK3R1, ADCY9, GNG2, ADCY2, and GNG13. Among these, MYC was upregulated in COAD, while the other hub genes were downregulated. Moreover, based on the expression data in the GEPIA database, we also confirmed that MYC, SNAP25, ADCY5, PTPRC, ADCY9, GNG2, and GNG13 were differentially expressed in COAD tissues versus normal tissues. The survival analysis showed that the low expression of ADCY5, PTPRC, and GNG2 had a significant association with the prognosis of COAD. In addition, COAD prognosis was significantly related to a patient’s clinical stage, with a later stage being associated with a worse prognosis (27). For the majority of early stage (stages I/II) CRC patients, partial or total colectomy alone is considered to be the primary treatment option, while late-stage (stages III/IV) CRC cases are often treated with neoadjuvant chemotherapy plus radiation and chemotherapy (28). Thus, by analyzing the expression level of ADCY5, GNG2, and PTPRC in different clinical stages of COAD, we found that the expression level of ADCY5 in stages I/II was lower than that in stages III/IV, which was consistent with the results of the survival analysis. This explains why patients with a low expression of ADCY5 are mainly in stages I/II and have a good prognosis. These findings suggest that ADCY5 is a prognostic risk marker of COAD.
ADCY5 is a member of the membrane-bound ADCY family, which converts adenosine triphosphate (ATP) into second messenger cyclic adenosine monophosphate (cAMP) and pyrophosphate (29). It may be the most abundant member of the ADCY family in human islets (30). Current studies have mainly focused on movement disorders related to ADCY5 mutation (31,32), but other research has also reported that the genetic variation of ADCY5 affects glucose metabolism, leading to the occurrence of type 2 diabetes (33). Although no previous studies have reported a correlation between ADCY5 and CRC, several bioinformatic analyses suggest that ADCY5 is a hub gene of CRC and have designated it as a potential biomarker of CRC (34). These findings are consistent with the results of this study.
In addition to the hub genes, some functional modules were screened using the Cytoscape software in this study. The top 3 modules were identified and underwent functional enrichment analysis using the KEGG pathway. The primary pathways were the chemokine signaling pathway, ubiquitin mediated proteolysis, and neuroactive ligand-receptor interaction. Chemokines are small molecule-secreting proteins and are produced by tumor and stromal cells. Chemokine receptors are also expressed on the surface of tumor and stromal cells and both can directly and indirectly control tumor growth. This can be seen, for example, in the way the receptors directly activate the signaling pathways that regulate the proliferation and metastasis of tumor cells while also indirectly effecting vascular endothelial cells and immune response after migration and organization of immune cells (35). Zhuo et al. have reported that chemokine (C-X-C motif) ligand 1 (CXCL1) is associated with tumor progression and poor prognosis in CRC patients (36). The ubiquitin mediated proteolysis is an ATP-dependent protein degradation pathway in the cytoplasm and nucleus. It maintains homeostasis by regulating the proteins it degrades through the ubiquitination-proteasome-deubiquitination mechanism. The destabilization of these regulated proteins causes cancer and other diseases (37). Fujita et al. also found that the overexpression of UBCH10, one of the ubiquitin-conjugating members, altered the cell cycle profile and accelerated the tumor proliferation in colon cancer (38). The neuroactive ligand-receptor interaction signaling pathway is a collection of all receptors and ligands on the plasma membrane associated with intracellular and extracellular signaling pathways. The critical role of neuroactive ligand-receptor interaction signaling pathways has been reported in Parkinson’s disease and psychiatric disorders (39,40), but not in cancer. Recently, it has been identified as a major molecular pathway of the Wnt10B gene, which is known to effect the prognosis of CRC (41).
Conclusions
This study has provided a comprehensive bioinformatic analysis of protein-coding genes which might be involved in COAD pathogenesis. Our findings suggest that ADCY5 could be a prognostic risk indicator or therapeutic target in the treatment of COAD.
Acknowledgments
We acknowledge CRN, GEPIA, and OncoLnc databases for providing their platforms and the contributors for uploading the data sets.
Funding: None.
Footnote
Reporting Checklist: The authors have completed the MDAR reporting checklist. Available at https://dx.doi.org/10.21037/jgo-21-415
Conflicts of Interest: All authors have completed the ICMJE uniform disclosure form (available at https://dx.doi.org/10.21037/jgo-21-415). The authors have no conflicts of interest to declare.
Ethical Statement: The authors are accountable for all aspects of the work in ensuring that questions related to the accuracy or integrity of any part of the work are appropriately investigated and resolved. CRN, GEPIA, and OncoLnc are public databases. The patients involved in the database have given their ethical approval. Users can download relevant data for research and the publishing relevant articles. The current study is based on open-source data, and hence there are no ethical issues or other conflicts of interest. The study was conducted in accordance with the Declaration of Helsinki (as revised in 2013).
Open Access Statement: This is an Open Access article distributed in accordance with the Creative Commons Attribution-NonCommercial-NoDerivs 4.0 International License (CC BY-NC-ND 4.0), which permits the non-commercial replication and distribution of the article with the strict proviso that no changes or edits are made and the original work is properly cited (including links to both the formal publication through the relevant DOI and the license). See: https://creativecommons.org/licenses/by-nc-nd/4.0/.
References
- Ferlay J, Ervik M, Lam F, et al. Global cancer observatory: cancer today. Lyon: International Agency for Research on Cancer, 2021.
- Cancer Genome Atlas Network. Comprehensive molecular characterization of human colon and rectal cancer. Nature 2012;487:330-7. [Crossref] [PubMed]
- Wang X. Discussion of the importance of early diagnosis and treatment of colorectal cancer from the epidemiological characteristics of colorectal cancer in China and United States of America. Chinese Journal of Colorectal Diseases 2021;10:26-33. (Electronic Edition).
- Siegel RL, Miller KD, Goding Sauer A, et al. Colorectal cancer statistics, 2020. CA Cancer J Clin 2020;70:145-64. [Crossref] [PubMed]
- Islami F, Goding Sauer A, Miller KD, et al. Proportion and number of cancer cases and deaths attributable to potentially modifiable risk factors in the United States. CA Cancer J Clin 2018;68:31-54. [Crossref] [PubMed]
- Winawer SJ, Zauber AG. The advanced adenoma as the primary target of screening. Gastrointest Endosc Clin N Am 2002;12:1-9. v. [Crossref] [PubMed]
- Lee PY, Chin SF, Low TY, et al. Probing the colorectal cancer proteome for biomarkers: Current status and perspectives. J Proteomics 2018;187:93-105. [Crossref] [PubMed]
- Henley SJ, Ward EM, Scott S, et al. Annual report to the nation on the status of cancer, part I: national cancer statistics. Cancer 2020;126:2225-49. [Crossref] [PubMed]
- Bresalier RS, Grady WM, Markowitz SD, et al. Biomarkers for early detection of colorectal cancer: the early detection research network, a framework for clinical translation. Cancer Epidemiol Biomarkers Prev 2020;29:2431-40. [Crossref] [PubMed]
- Duffy MJ, Lamerz R, Haglund C, et al. Tumor markers in colorectal cancer, gastric cancer and gastrointestinal stromal cancers: European group on tumor markers 2014 guidelines update. Int J Cancer 2014;134:2513-22. [Crossref] [PubMed]
- Jiang J. Clinical application of tumor markers in colorectal cancer. Chinese Journal of Difficult and Complicated Cases 2020;19:860-4.
- Konishi T, Shimada Y, Hsu M, et al. Association of preoperative and postoperative serum carcinoembryonic antigen and colon cancer outcome. JAMA Oncol 2018;4:309-15. [Crossref] [PubMed]
- Yang W, Luo Y, Hu S, et al. Value of combined detection of serum carcino-embryonic antigen, carbohydrate antigen 19-9 and cyclooxygenase-2 in the diagnosis of colorectal cancer. Oncol Lett 2018;16:1551-6. [Crossref] [PubMed]
- Li JR, Sun CH, Li W, et al. Cancer RNA-Seq Nexus: a database of phenotype-specific transcriptome profiling in cancer cells. Nucleic Acids Res 2016;44:D944-51. [Crossref] [PubMed]
- Huang da W. Sherman BT, Lempicki RA. Systematic and integrative analysis of large gene lists using DAVID bioinformatics resources. Nat Protoc 2009;4:44-57. [Crossref] [PubMed]
- Szklarczyk D, Gable AL, Lyon D, et al. STRING v11: protein-protein association networks with increased coverage, supporting functional discovery in genome-wide experimental datasets. Nucleic Acids Res 2019;47:D607-13. [Crossref] [PubMed]
- Shannon P, Markiel A, Ozier O, et al. Cytoscape: a software environment for integrated models of biomolecular interaction networks. Genome Res 2003;13:2498-504. [Crossref] [PubMed]
- Chin CH, Chen SH, Wu HH, et al. cytoHubba: identifying hub objects and sub-networks from complex interactome. BMC Syst Biol 2014;8:S11. [Crossref] [PubMed]
- Bindea G, Mlecnik B, Hackl H, et al. ClueGO: a Cytoscape plug-in to decipher functionally grouped gene ontology and pathway annotation networks. Bioinformatics 2009;25:1091-3. [Crossref] [PubMed]
- Bader GD, Hogue CW. An automated method for finding molecular complexes in large protein interaction networks. BMC Bioinformatics 2003;4:2. [Crossref] [PubMed]
- Tang Z, Li C, Kang B, et al. GEPIA: a web server for cancer and normal gene expression profiling and interactive analyses. Nucleic Acids Res 2017;45:W98-W102. [Crossref] [PubMed]
- Anaya J. OncoLnc: linking TCGA survival data to mRNAs, miRNAs, and lncRNAs. PeerJ Comput Sci 2016;2:e67 [Crossref]
- Barrett T, Wilhite SE, Ledoux P, et al. NCBI GEO: archive for functional genomics data sets--update. Nucleic Acids Res 2013;41:D991-5. [Crossref] [PubMed]
- Wang Z, Jensen MA, Zenklusen JC. A practical guide to The Cancer Genome Atlas (TCGA). Methods Mol Biol 2016;1418:111-41. [Crossref] [PubMed]
- Gao J, Aksoy BA, Dogrusoz U, et al. Integrative analysis of complex cancer genomics and clinical profiles using the cBioPortal. Sci Signal 2013;6:pl1. [Crossref] [PubMed]
- Forbes SA, Beare D, Gunasekaran P, et al. COSMIC: exploring the world's knowledge of somatic mutations in human cancer. Nucleic Acids Res 2015;43:D805-11. [Crossref] [PubMed]
- Miller KD, Nogueira L, Mariotto AB, et al. Cancer treatment and survivorship statistics, 2019. CA Cancer J Clin 2019;69:363-85. [Crossref] [PubMed]
- Luo R, Song J, Zhang W, et al. Identification of MFI2-AS1, a novel pivotal lncRNA for prognosis of stage III/IV colorectal cancer. Dig Dis Sci 2020;65:3538-50. [Crossref] [PubMed]
- Defer N, Best-Belpomme M, Hanoune J. Tissue specificity and physiological relevance of various isoforms of adenylyl cyclase. Am J Physiol Renal Physiol 2000;279:F400-16. [Crossref] [PubMed]
- Eizirik DL, Sammeth M, Bouckenooghe T, et al. The human pancreatic islet transcriptome: expression of candidate genes for type 1 diabetes and the impact of pro-inflammatory cytokines. PLoS Genet 2012;8:e1002552 [Crossref] [PubMed]
- Vijiaratnam N, Bhatia KP, Lang AE, et al. ADCY5-related dyskinesia: improving clinical detection of an evolving disorder. Mov Disord Clin Pract 2019;6:512-20. [Crossref] [PubMed]
- Miyamoto R, Kawarai T, Takeuchi T, et al. Efficacy of istradefylline for the treatment of ADCY5-related disease. Mov Disord Clin Pract 2020;7:852-3. [Crossref] [PubMed]
- Lin R, Yuan Z, Zhang C, et al. Common genetic variants in ADCY5 and gestational glycemic traits. PLoS One 2020;15:e0230032 [Crossref] [PubMed]
- Miao Y, Li Q, Wang J, et al. Prognostic implications of metabolism-associated gene signatures in colorectal cancer. PeerJ 2020;8:e9847 [Crossref] [PubMed]
- Nagarsheth N, Wicha MS, Zou W. Chemokines in the cancer microenvironment and their relevance in cancer immunotherapy. Nat Rev Immunol 2017;17:559-72. [Crossref] [PubMed]
- Zhuo C, Wu X, Li J, et al. Chemokine (C-X-C motif) ligand 1 is associated with tumor progression and poor prognosis in patients with colorectal cancer. Biosci Rep 2018;38:BSR20180580 [Crossref] [PubMed]
- Hanahan D, Weinberg RA. Hallmarks of cancer: the next generation. Cell 2011;144:646-74. [Crossref] [PubMed]
- Fujita T, Ikeda H, Taira N, et al. Overexpression of UbcH10 alternates the cell cycle profile and accelerate the tumor proliferation in colon cancer. BMC Cancer 2009;9:87. [Crossref] [PubMed]
- Adkins DE, Khachane AN, McClay JL, et al. SNP-based analysis of neuroactive ligand-receptor interaction pathways implicates PGE2 as a novel mediator of antipsychotic treatment response: data from the CATIE study. Schizophr Res 2012;135:200-1. [Crossref] [PubMed]
- Kong Y, Liang X, Liu L, et al. High throughput sequencing identifies microRNAs mediating α-synuclein toxicity by targeting neuroactive-ligand receptor interaction pathway in early stage of drosophila Parkinson's disease model. PLoS One 2015;10:e0137432 [Crossref] [PubMed]
- Chen G, Ding H, Diao T, et al. WNT10B as a new prognostic molecular marker for colorectal cancer and the effect of Wnt family on the progression of colorectal cancer. Chinese Journal of Current Advances in General Surgery 2018;21:543-8.
(English Language Editors: J. Jake and J. Gray)