Underlying mechanism of sorafenib resistance in hepatocellular carcinoma: a bioinformatics study based on validated resistance-related genes
Introduction
The multi-targeted tyrosine kinase inhibitors (TKIs) including sorafenib, lenvatinib and donafenib showed important roles in the first-line treatment of patients with unresectable hepatocellular carcinoma (HCC). In 2007, sorafenib became the first small-molecule TKI approved for first-line treatment of advanced hepatocellular carcinoma (HCC) (1). Sorafenib is a multi-kinase inhibitor with anti-angiogenic, pro-apoptotic, and anti-proliferative effects that targets vascular endothelial growth factor receptor (VEGFR)-1, VEGFR-2, VEGFR-3, platelet-derived growth factor receptor (PDGFR)-b, c-KIT, FMS-like tyrosine kinase 3 (FLT-3), and RET (1). However, the effects of sorafenib can be compromised (1-4); it has been shown to significantly extend the median survival time of treated patients, but only by 3–5 months. This has largely been attributed to the development of resistance within 6 months (3-6). Combination of sorafenib and cytotoxic chemotherapeutic agents, or molecular targeted agents, or immunotherapeutic drugs were studied to overcome sorafenib resistance in HCC (7).
Resistance to sorafenib is related to multiple cellular phenomena, including hypoxic microenvironment, epithelial-mesenchymal transition (EMT), apoptosis resistance and dysregulation of cell-cycle control, cancer stem cells (CSCs), autophagy, the PI3K/Akt and JAK-STAT pathways, endothelial growth factor receptor (EGFR) activation, c-Jun activation, and AKT activation (8-10). Therefore, it is difficult to comprehensively explain sorafenib resistance in HCC in terms of a single molecule or pathway.
Most recently, bioinformatics analysis has been viewed as a crucial tool in the study of complex mechanisms (11). It has also been used to sketch the landscape of the molecular mechanisms involved in sorafenib resistance (12,13). Several functions and pathways, such as negative regulation of endopeptidase activity, cholesterol homeostasis, DNA replication and repair, coagulation cascades, insulin resistance, RNA transport, cell cycle, adhesion, coagulation, vasculature development, steroid metabolic process, and lipid homeostasis have been found to relate to resistance by bioinformatics analyses based on differentially expressed genes (DEGs) identified using microarrays. In addition, hub genes, including kininogen 1 (KNG1), vascular cell adhesion molecule 1 (VCAM-1), apolipoprotein C3 (APOC3), alpha 2-HS glycoprotein (AHSG), erb-b2 receptor tyrosine kinase 2 (CD340), secreted protein acidic and cysteine rich (SPARC), vitronectin, and vimentin were also identified (12,13).
Bioinformatics analyses have generally been conducted based on hundreds and thousands of DEGs identified from microarrays or RNA sequencing (12). The power of the bioinformatics analysis was determined by the quality and quantity of DEGs involved in the analysis. However, the expression levels of the identified DEGs generally need to be validated by quantitative real-time polymerase chain reaction (qPCR) (14,15), and their role in promoting or inhibiting resistance must be investigated using in vitro or in vivo studies. Due to the limitations of practical capacity, it is difficult to validate the massive number of DEGs identified. Therefore, bioinformatics analysis based on validated resistance driver genes should be conducted. A bioinformatic analysis-based validation of DEGs has not yet been reported. Thus, in the present study, we collected experimentally determined sorafenib-related resistance genes (SRRGs) from publications and performed a SRRG-based bioinformatics analysis to investigate their participation in sorafenib resistance in HCC. We present the following article in accordance with the STREGA reporting checklist (available at https://dx.doi.org/10.21037/jgo-21-377).
Methods
Search strategy, study selection, and SRRGs collection
Data from Medline and Embase were retrieved using an advanced strategy combining keywords of hepatocellular (hepatic, hepatocellular, or liver), sorafenib, and resistance (resistance or resistant). The search was conducted on 19 November 2018. Studies that focused on resistance-related molecules, both in vivo and in vitro, were eligible. All publications were independently reviewed and evaluated by 2 investigators (LW Zhang and L Fang) according to the abstracts and full texts, if necessary. Genes were investigated with respect to their ability to promote or inhibit the behavior of resistance to sorafenib, and were identified as SRRGs upon validation by genetic or pharmacologic interventions to alter expression levels of genes or proteins in cell lines or animal models of HCC. Disagreements between the investigators were resolved by consensus.
Gene ontology and pathway enrichment analysis of SRRGs
To annotate the SRRGs at the functional level, Gene Ontology (GO) and Kyoto Encyclopedia of Genes and Genomes (KEGG) pathway (http://www.genome.jp/) enrichment analysis was performed using the Database for Annotation, Visualization and Integrated Discovery (DAVID) online tool (https://david.ncifcrf.gov/). Statistical significance was set at P<0.05.
Protein-protein interaction (PPI) network and hub genes
Interactive relationships among SRRGs were evaluated by PPI analysis. The SRRGs were mapped to the Search Tool for the Retrieval of Interacting Genes (STRING) database to construct interactions among genes, and only experimentally validated interactions with a combined score >0.4 were deemed significant. Then, PPI networks were built using the Cytoscape software package (version 3.6.1, National Institute of General Medical Sciences, Bethesda, MD, USA) according to the Molecular Complex Detection (MCODE) (16) and in terms of the function and localization similarity of proteins within predicted complexes with the 2 of degree cutoff, 2 of K-core, 0.2 of node score cutoff, and 100 of maximum depth from seed.
Statistical analysis
A P value less than 0.05 is statistically significant.
Results
Identification of SRRGs
The search strategy described above generated a list of 833 publications (Figure 1). Of these, 688 studies were excluded based on the following criteria: non-basic research (n=55), other drugs (n=138), non-hepatocellular cancer (n=61), duplicate studies (n=52), irrelevant to resistance (n=237), inaccessible information (n=18), no validated relation to the promotion or inhibition of resistance (n=116), and focused on long non-coding (lnc)RNAs or micro (mi)RNAs (n=11). A total of 145 articles were found to be relevant. Only 11 and 1 of the papers reported 2 and 3 molecules, respectively, while the other papers focused on a single gene. A total of 145 genes, with 117 resistance-promoting genes and 28 resistance-inhibiting genes, were included. A total of 23 (15.86%), 2 (1.38%), 2 (1.38%), 1 (0.69%), and 1 (0.69%) genes were reported in 2, 3, 4, 6, and 14 publications, respectively. Of these, AKT1, TGFB1, EGFR, MAPK14, ERK, and SCD1 were the most frequently reported genes in 14, 6, 4, 4, 3, and 3 studies, respectively.
GO of SRRGs
A total of 1,721, 1,474, and 202 GO terms were enriched based on total SRRGs, resistance-promoting genes, and resistance-inhibiting genes, respectively. The positive regulation of cell migration (P=3.63E-19), regulation of epithelial cell proliferation (P=9.28E-19), response to oxygen levels (P=3.31E-18), positive regulation of epithelial cell proliferation (P=1.08E-17), epithelial cell proliferation (P=1.89E-17), response to hypoxia (P=1.23E-16), response to decreased oxygen levels (P=1.95E-16), regulation of DNA binding transcription factor activity (P=3.78E-16), gland development (P=7.55E-16), and positive regulation of neurogenesis (P=7.55E-16), were significantly enriched.
Pathway enrichment analysis of SRRGs
There was a total of 113 pathways identified as being enriched using the KEGG database. The top 10 pathways were: prostate cancer (P=6.16E-16), proteoglycans in cancer (P=2.37E-15), PI3K-Akt signaling pathway (P=1.45E-14), melanoma (P=1.23E-13), EGFR tyrosine kinase inhibitor resistance (P=4.33E-13), breast cancer (P=5.22E-13), MAPK signaling pathway (P=3.58E-12), gastric cancer (P=9.56E-12), HIF-1 signaling pathway (P=9.93E-12), and AGE-RAGE signaling pathway in diabetic complications (P=9.93E-12) (Figure 2). The pathway of cancer was the pathway involving the highest number of genes (Figure 3).
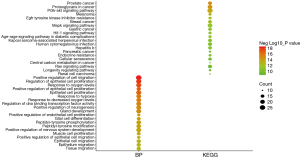
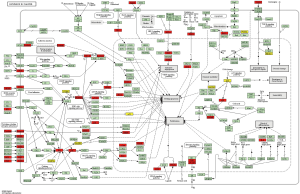
Moreover, pathways related to cell proliferation or apoptosis (Ras, Rap1, JAK-STAT, AMPK, VEGF, ErbB, mTOR, FoxO, apoptosis), immune function (Th17 cell differentiation, Th1 and Th2 cell differentiation, interleukin (IL)-17, T cell receptor, nuclear factor (NF)-kappa B, Toll-like receptor, B cell receptor, tumor necrosis factor (TNF), c-type lectin receptor, Fc epsilon RI, RIG-I-like receptor, NOD-like receptor), endocrine metabolism (prolactin, thyroid hormone, adipocytokine, GnRH, apelin, insulin), and stem cell and differentiation (regulating pluripotency of stem cells, focal adhesion, adherens junction, regulation of actin cytoskeleton) were significantly related to upregulated genes. Central carbon metabolism in cancer, transforming growth factor (TGF)-beta signaling pathway, miRNAs in cancer, cellular senescence, human T-cell leukemia virus 1 infection, p53, and adherens junction proteins were significantly related to downregulated genes.
PPI network and hub genes
When the cutoff value of degrees was set to 10, a total of 81 genes were identified as hub genes. The top 25 hub nodes [value of degree], included: TP53 [87], AKT1 [82], EGFR [69], STAT3 [67], VEGFA [63], JUN [62], MAPK1 [59], IL6 [59], PTEN [58], CTNNB1 [53], KRAS [52], FGF2 [50], MTOR [46], CD44 [43], HGF [42], HIF1A [39], CXCR4 [38], HDAC1 [38], PTGS2 [36], RELA [35], MAPK14 [35], PIK3CA [35], IGF1R [34], CDKN1A [34], and MET [33], Table 1. Among these genes, 44 were involved in 3 clusters (Figure 4). The 3 clusters included 32, 3, and 9 genes with HIF1A, CCNE1, and CD24 as seed nodes, respectively.
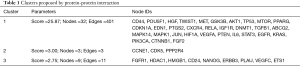
Full table
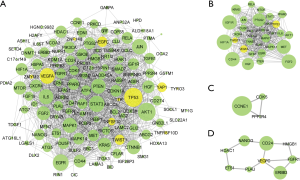
Discussion
The underlying mechanism of sorafenib resistance has been proposed using bioinformatics analysis based on hundreds, or even thousands of DEGs, from high-throughput methods, such as microarray and RNA sequencing (12,13). However, most of the DEGs were not validated in terms of expression levels or their actual role in resistance. Therefore, in the present study, a comprehensive bioinformatics analysis was conducted based on a collection of experimentally validated resistance-related genes in HCC.
A total of 145 SRRGs were collected from 145 studies and used for bioinformatics analysis. More than 4/5 of the genes were upregulated in the resistance-related samples, and promoted resistance. Approximately 80% and 16% of the included genes were reported in only 1 or 2 publications, respectively, despite 6 genes being reported by 14 (AKT1), 6 (TGFB1), 4 (EGFR and MAPK14), and 3 (ERK and SCD1) studies. Although the size of the gene collection seems moderate, in comparison with previously published bioinformatics analysis of HCC, which included 256 (17), 541 (13), 602 (18), 1,167 (19), 1,230 (20), and 1,319 (12) DEGs, the statistical power was significant and included 1,721 related functions, 113 pathways, 81 hub genes, and 3 clusters.
Biological functions including cell proliferation, cell migration, development, response to oxygen levels, EMT, cell skeleton, protein function, and autophagy were proposed as crucial functions related to resistance in this study. This is consistent with the findings of previous studies. Sorafenib acts as an anti-angiogenic agent by reducing microvessel density, promoting intra-tumoral hypoxia, and upregulating hypoxia-inducible factors (HIFs). A hypoxic microenvironment favors resistant cells (8). In addition to anti-angiogenesis, anti-clonogenesis and cytotoxic effects are crucial in the antitumor effects elicited by sorafenib (21). Autophagy has been reported as a controversial role of sorafenib in HCC treatment, where it has been shown to improve the power of sorafenib against HCC cells, or conversely, serve as a protective factor against HCC cells from sorafenib (22). The EMT caused drug resistance across various solid tumors, and was also found to be the main source of sorafenib resistance by reprogramming cancer cells and generating CSCs after sorafenib treatment (23,24).
Pathways related to cell proliferation, apoptosis, immune function, endocrine metabolism, stem cell function, and differentiation were enriched, based on SRRGs. Most recently, many studies have focused on the role of immunosuppression in sorafenib resistance. Immunosuppression-related resistance to sorafenib has been attributed to hypoxia, demonstrated by the upregulated expression of immune checkpoint inhibitor programmed death ligand-1 (PD-L1) (25) and increased infiltration of Gr1+ myeloid-derived suppressive cells, regulatory T cells, M2 macrophages (26), and tumor-associated neutrophils (27), which may be associated with increased SDF1α, CXCR4, CCL2/CCR2, and CCL17 expression levels in tumors (25-29). Modulation of immune responses by combining a CCR2 antagonist with a CXCR4 inhibitor and an anti-PD-1 antibody synergized sorafenib effects through elevated intratumoral infiltration of CD8+ T cells, and increased expression levels of IL-2, TNF-α, and interferon gamma (IFN-γ) (25,26,28,30).
A total of 81 hub genes were identified, among which 2, 12, and 25 were involved in more than 80, 50, and 30 degrees, respectively. The top 3 hub genes, TP53, AKT1, and EGFR, contributed 87, 82, and 69 edges, respectively. Although TP53, a coded tumor-suppressor protein p53, was validated as an SRRG in only 1 study, the sensitivity of sorafenib can be abrogated by p53 knockdown in HepG2 cells and recovered by p53 overexpression in Hep3 B cells (31), and it was identified as the top hub gene. As a transcription factor, p53 is a master regulator of cell cycle arrest, DNA repair, apoptosis, senescence, angiogenesis, autophagy, metabolism, development, stem cell function, and chemoresistance in HCC (32-34). The other 2 top hub genes, EGFR and AKT, have frequently been reported as key molecules causing sorafenib resistance (10). The PI3K/AKT signaling pathway (35,36) and HGF/c-Met/AKT signaling (37,38) are 2 well-known tumor survival mechanisms that play crucial roles in the development and progression of HCC by overcoming sorafenib-induced cell apoptosis and autophagic death (37,38). Blocking AKT increases the sensitivity of HCC cells to sorafenib.
The findings described herein are consistent with previously published reviews (8-10,21-23,39,40), but inconsistent with the bioinformatics analysis based on DEGs conducted by Huang et al. (12). In a previous study, the function of DNA damage and repair, the extracellular matrix, and others (negative regulation of endopeptidase activity, cholesterol homeostasis, fibrinolysis, and platelet degranulation) were addressed (12). The pathways related to complement, lysosome, insulin resistance, metabolic pathways, fat digestion and absorption, ribosome biogenesis in eukaryotes, RNA transport, fanconi anemia pathway, taste transduction, and cell cycle were proposed to be crucial in sorafenib resistance (12). In addition, 8 hub genes (KNG1, VCAM1, APOC3, AHSG, ERBB2, SPARC, VTN, and VIM) were selected in Huang et al.’s study using the same cutoff criterion (>10) (12), and no genes were shared between the two collections of hub genes identified. Compared with our study, a narrow mechanism contributed to sorafenib resistance in Huang’s paper was reported. The clear reasons contributing to this inconsistency are not clear, and may be due in part to individual characteristics of HuH-7 cell.
A comprehensive bioinformatics analysis, based on validated genes, has not yet been published. It was interesting to test a validated molecule-based analysis. As a preliminary result, it seems to have higher statistical power in comparison to an analysis based on DEGs. Some limitations of this study should be addressed. First, the genes were collected from individual studies, which had been validated by different methods, such as genetic or pharmaceutical intervention, based on various cell lines or animal models. The heterogeneity of each study was considered. Moreover, the reported genes tended to focus on ‘popular’ molecules, which had been frequently investigated and reported. Although a comprehensive analysis of the mechanism was provided, this approach does not provide an efficient method to explore novel mechanisms of resistance, as it only includes experimentally validated SRRGs.
In conclusion, the present study identified experimentally validated SRRGs from publications and illustrated the underlying mechanism of sorafenib resistance using bioinformatics analysis based on the SRRGs. Our findings further revealed the mechanisms of sorafenib resistance in HCC and provided potential biomarkers for outcome prediction and novel treatment targets.
Acknowledgments
Funding: The work was supported by the National Natural Science Foundation of China (81773819, 81973396, 82003851), Natural Science Foundation of Zhejiang Province (Y16H160129, Q17H300007, LY19H160006), Science and Technology in Zhejiang Province Chinese Medicine Program (2015ZA036, LSY19H300001), the Medical Science and Technology Program of Zhejiang province (2013KYA027) and Zhejiang Provincial Program for 151 Talents (LF).
Footnote
Reporting Checklist: The authors have completed the STREGA reporting checklist. Available at https://dx.doi.org/10.21037/jgo-21-377
Conflicts of Interest: All authors have completed the ICMJE uniform disclosure form (available at https://dx.doi.org/10.21037/jgo-21-377). The authors have no conflicts of interest to declare.
Ethical Statement: The authors are accountable for all aspects of the work in ensuring that questions related to the accuracy or integrity of any part of the work are appropriately investigated and resolved.
Open Access Statement: This is an Open Access article distributed in accordance with the Creative Commons Attribution-NonCommercial-NoDerivs 4.0 International License (CC BY-NC-ND 4.0), which permits the non-commercial replication and distribution of the article with the strict proviso that no changes or edits are made and the original work is properly cited (including links to both the formal publication through the relevant DOI and the license). See: https://creativecommons.org/licenses/by-nc-nd/4.0/.
References
- Keating GM. Sorafenib: A Review in Hepatocellular Carcinoma. Target Oncol 2017;12:243-53. [Crossref] [PubMed]
- Brower V. Sorafenib plus cisplatin for hepatocellular carcinoma. Lancet Oncol 2016;17:e424 [Crossref] [PubMed]
- Llovet JM, Ricci S, Mazzaferro V, et al. Sorafenib in advanced hepatocellular carcinoma. N Engl J Med 2008;359:378-90. [Crossref] [PubMed]
- Cheng AL, Kang YK, Chen Z, et al. Efficacy and safety of sorafenib in patients in the Asia-Pacific region with advanced hepatocellular carcinoma: a phase III randomised, double-blind, placebo-controlled trial. Lancet Oncol 2009;10:25-34. [Crossref] [PubMed]
- Wang H, Wang H, Yu Z, et al. Alternative treatment strategies to sorafenib in patients with advanced hepatocellular carcinoma: a meta-analysis of randomized Phase III trials. Onco Targets Ther 2018;11:5195-201. [Crossref] [PubMed]
- Ni JY, Liu SS, Sun HL, et al. Transcatheter hepatic arterial infusion chemotherapy vs sorafenib in the treatment of patients with hepatocellular carcinoma of Barcelona Clinic Liver Cancer stage C: a meta-analysis of Asian population. Onco Targets Ther 2018;11:7883-94. [Crossref] [PubMed]
- Tang W, Chen Z, Zhang W, et al. The mechanisms of sorafenib resistance in hepatocellular carcinoma: theoretical basis and therapeutic aspects. Signal Transduct Target Ther 2020;5:87. [Crossref] [PubMed]
- Méndez-Blanco C, Fondevila F, García-Palomo A, et al. Sorafenib resistance in hepatocarcinoma: role of hypoxia-inducible factors. Exp Mol Med 2018;50:1-9. [Crossref] [PubMed]
- Zhu YJ, Zheng B, Wang HY, et al. New knowledge of the mechanisms of sorafenib resistance in liver cancer. Acta Pharmacol Sin 2017;38:614-22. [Crossref] [PubMed]
- Niu L, Liu L, Yang S, et al. New insights into sorafenib resistance in hepatocellular carcinoma: Responsible mechanisms and promising strategies. Biochim Biophys Acta Rev Cancer 2017;1868:564-70. [Crossref] [PubMed]
- Zhou L, Du Y, Kong L, et al. Identification of molecular target genes and key pathways in hepatocellular carcinoma by bioinformatics analysis. Onco Targets Ther 2018;11:1861-9. [Crossref] [PubMed]
- Huang D, Yuan W, Li H, et al. Identification of key pathways and biomarkers in sorafenib-resistant hepatocellular carcinoma using bioinformatics analysis. Exp Ther Med 2018;16:1850-8. [Crossref] [PubMed]
- Lv J, Zhu B, Zhang L, et al. Detection and screening of small molecule agents for overcoming Sorafenib resistance of hepatocellular carcinoma: a bioinformatics study. Int J Clin Exp Med 2015;8:2317-25. [PubMed]
- Morey JS, Ryan JC, Van Dolah FM. Microarray validation: factors influencing correlation between oligonucleotide microarrays and real-time PCR. Biol Proced Online 2006;8:175-93. [Crossref] [PubMed]
- Tulpan D, Davey ML, aflamme M, Quantitative Reverse Transcriptase Polymerase Chain Reaction for Validating Microbial Microarray Data. Quantitative Real-time PCR in Applied Microbiology 2012:181-32.
- Bader GD, Hogue CW. An automated method for finding molecular complexes in large protein interaction networks. BMC Bioinformatics 2003;4:2. [Crossref] [PubMed]
- Fu Q, Yang F, Zhao J, et al. Bioinformatical identification of key pathways and genes in human hepatocellular carcinoma after CSN5 depletion. Cell Signal 2018;49:79-86. [Crossref] [PubMed]
- Lavi O, Skinner J, Gottesman MM. Network features suggest new hepatocellular carcinoma treatment strategies. BMC Syst Biol 2014;8:88. [Crossref] [PubMed]
- Wang F, Wang R, Li Q, et al. A transcriptome profile in hepatocellular carcinomas based on integrated analysis of microarray studies. Diagn Pathol 2017;12:4. [Crossref] [PubMed]
- Zhang C, Peng L, Zhang Y, et al. The identification of key genes and pathways in hepatocellular carcinoma by bioinformatics analysis of high-throughput data. Med Oncol 2017;34:101. [Crossref] [PubMed]
- Chen J, Jin R, Zhao J, et al. Potential molecular, cellular and microenvironmental mechanism of sorafenib resistance in hepatocellular carcinoma. Cancer Lett 2015;367:1-11. [Crossref] [PubMed]
- Sun T, Liu H, Ming L. Multiple Roles of Autophagy in the Sorafenib Resistance of Hepatocellular Carcinoma. Cell Physiol Biochem 2017;44:716-27. [Crossref] [PubMed]
- Mir N, Jayachandran A, Dhungel B, et al. Epithelial-to-Mesenchymal Transition: A Mediator of Sorafenib Resistance in Advanced Hepatocellular Carcinoma. Curr Cancer Drug Targets 2017;17:698-706. [Crossref] [PubMed]
- Dazert E, Colombi M, Boldanova T, et al. Quantitative proteomics and phosphoproteomics on serial tumor biopsies from a sorafenib-treated HCC patient. Proc Natl Acad Sci U S A 2016;113:1381-6. [Crossref] [PubMed]
- Chen Y, Ramjiawan RR, Reiberger T, et al. CXCR4 inhibition in tumor microenvironment facilitates anti-programmed death receptor-1 immunotherapy in sorafenib-treated hepatocellular carcinoma in mice. Hepatology 2015;61:1591-602. [Crossref] [PubMed]
- Chen Y, Reiberger T, Ramjiawan R, et al. CXCR4 inhibition facilitates anti-PD-1 immunotherapy by reprogramming the tumor microenvironment during sorafenib treatment in hepatocellular carcinoma. Cancer Immunology Research 2015; Available online: https://cancerimmunolres.aacrjournals.org/content/3/10_Supplement/B79
- Zhou SL, Zhou ZJ, Hu ZQ, et al. Tumor-Associated Neutrophils Recruit Macrophages and T-Regulatory Cells to Promote Progression of Hepatocellular Carcinoma and Resistance to Sorafenib. Gastroenterology 2016;150:1646-1658.e17. [Crossref] [PubMed]
- Yao W, Ba Q, Li X, et al. A Natural CCR2 Antagonist Relieves Tumor-associated Macrophage-mediated Immunosuppression to Produce a Therapeutic Effect for Liver Cancer. EBioMedicine 2017;22:58-67. [Crossref] [PubMed]
- Reiberger T, Yunching C, Ramjiawan RR, et al. CXCR4 inhibition reverts immunosuppressive tumor microenvironment and facilitates anti-PD-1 immunotherapy in sorafenib-treated hepatocellular carcinoma. J Hepatol 2015;62:S416. [Crossref]
- Reiberger T, Ramjiawan RR, Chen Y, et al. CXCR4 inhibition in combination with anti-PD1 immunotherapy results in an effective immune response during treatment with sorafenib in hepatocellular carcinoma. Hepatology 2014;60:644A.
- Omar HA, Tolba MF, Hung JH, et al. OSU-2S/Sorafenib Synergistic Antitumor Combination against Hepatocellular Carcinoma: The Role of PKCδ/p53. Front Pharmacol 2016;7:463. [Crossref] [PubMed]
- Joerger AC, Fersht AR. The p53 Pathway: Origins, Inactivation in Cancer, and Emerging Therapeutic Approaches. Annu Rev Biochem 2016;85:375-404. [Crossref] [PubMed]
- Stiewe T, Haran TE. How mutations shape p53 interactions with the genome to promote tumorigenesis and drug resistance. Drug Resist Updat 2018;38:27-43. [Crossref] [PubMed]
- Li S, Gao M, Li Z, et al. p53 and P-glycoprotein influence chemoresistance in hepatocellular carcinoma. Front Biosci (Elite Ed) 2018;10:461-8. [PubMed]
- Zhang H, Wang Q, Liu J, et al. Inhibition of the PI3K/Akt signaling pathway reverses sorafenib-derived chemo-resistance in hepatocellular carcinoma. Oncol Lett 2018;15:9377-84. [Crossref] [PubMed]
- Ersahin T, Tuncbag N, Acar A, et al. Combination Therapy for Hepatocellular Carcinoma: A Systems Biology Perspective on the Synergistic Antitumor Activity of Sorafenib with Pi3k/Akt Pathway Inhibitors. J Hepatol 2015;62:S464. [Crossref]
- Han P, Li H, Jiang X, et al. Dual inhibition of Akt and c-Met as a second-line therapy following acquired resistance to sorafenib in hepatocellular carcinoma cells. Mol Oncol 2017;11:320-34. [Crossref] [PubMed]
- Qu Z, Wu J, Wu J, et al. Exosomes derived from HCC cells induce sorafenib resistance in hepatocellular carcinoma both in vivo and in vitro. J Exp Clin Cancer Res 2016;35:159. [Crossref] [PubMed]
- Ray EM, Sanoff HK. Optimal therapy for patients with hepatocellular carcinoma and resistance or intolerance to sorafenib: challenges and solutions. J Hepatocell Carcinoma 2017;4:131-8. [Crossref] [PubMed]
- Makarova AS, Lazarevich NL. Deregulation of signaling pathways involved in sorafenib resistance of hepatocellular carcinoma. Klin Lab Diagn 2013;(10):66-8, 34-7.
(English Language Editor: J. Jones)