Prognostic prediction and gene regulation network of EIF2S2 in hepatocellular carcinoma based on data mining
Introduction
Globally, liver cancer is ranked the sixth most common cancer with the third highest mortality rate, of which hepatocellular carcinoma (HCC) accounts for 75–85% of cases (1). HCC has a poor prognosis, short survival outcome, and no clear curative strategies (2), plaguing many medical researchers (1,3,4). Therefore, it is necessary to find new treatment targets by screening the interaction network of genes related to the prognosis of HCC patients.
Compelling evidence has shown that RNA-binding proteins (RBPs) play critical roles in regulating gene expression in many diseases, especially tumors (5,6). In recent years, several RBPs closely associated with cancers have been discovered. For example, the RNA binding protein FXR1 degrades p21-mRNA via the stability of miR301a-3p in oral cancer (7). RBP Musashi2 increases the stabilization of the androgen receptor pathway by binding to the 3'-untranslated region of its mRNA, promoting prostate cancer progression (8). EIF2B5 has been proved to participate in cancer progression and has become a biomarker for the prognosis of patients with liver cancer (9).
Furthermore, in recent years eukaryotic translation initiation factor 2 subunit beta (EIF2S2) has been identified as a novel gene within the RBP family. EIF2S2 is mainly involved in trait genetics in the normal physiological process of humans and animals, such as skin color (10), pigmentation (11), and hair fibers (12). The most interesting aspect now lies in its promising role in clinical diseases, such as ovarian cancer (13), testicular cancer (14), breast cancer (15), ovarian insufficiency (16), and lung adenocarcinoma (17). Several studies have indicated that EIF2S2 may have significant potential in predicting cancer stage and prognosis, but the specific mechanism remains unclear. One report confirms that EIF2S2 is highly expressed in gastrointestinal cancers and promotes cell proliferation, migration, and invasion of colorectal cancer via the EIF2S2-LINC01600-MYC axis (18). However, the prognostic relationship between EIF2S2 and HCC has not been established.
This study explored the expression level of EIF2S2 in HCC tissues, the associations between EIF2S2 and the clinical features of HCC patients, and the effect of EIF2S2 gene mutations on the prognosis of patients. Using bioinformatics analyses, we also constructed gene networks and explored the predictive function of gene sets to elucidate the prognostic role and interaction networks of EF2S2 in HCC, hoping to provide new directions for prognostic prediction and future therapeutic interventions. We present the following article in accordance with the REMARK reporting checklist (available at https://dx.doi.org/10.21037/jgo-21-748).
Methods
Oncomine database
The Oncomine database (www.oncomine.org) is a powerful online tool that integrates and unifies high-throughput cancer research profiling data (DNA and RNA), helping researchers rapidly assess target expressions across a large volume of cancer types, subtypes, and experiments (19). The study was conducted in accordance with the Declaration of Helsinki (as revised in 2013). We acquired the EIF2S2 mRNA levels from the Oncomine database. The Student’s t-test was used to compare the difference in EIF2S2 mRNA levels between HCC vs. normal tissues. The following parameters were selected: P value: 0.05; fold change: 1.5; gene rank: 10%; and data type: mRNA.
Ualcan database
Ualcan (http://Ualcan.path.uab.edu) provides easy access to The Cancer Genome Atlas (TCGA) (20). In this study, it was used to analyze the relative expression of EIF2S2 in different grades and TNM-stages of HCC. The Student’s t-test was employed in the statistical analysis of EIF2S2 transcription, where P<0.01 was considered statistically significant.
TCGA
TCGA has collected 2.5 PB of data, including genome, epigenome, transcriptome, and proteome data to obtain a comprehensive understanding of the genomic alterations that underlie all major cancers. It has revealed the characteristics of cancers from the gene and molecular perspective and has improved the diagnosis, treatment, and prevention of cancers (21). R software (for statistical computing and graphics, version 3.6.3) was used to analyze the RNAseq data and clinicopathological characteristics of liver hepatocellular carcinoma (LIHC) patients in TCGA. Using the median expression of EIF2S2 as the dividing line, the correlation between the expression of EIF2S2 and the clinical characteristics of patients was analyzed. The data without clinical information was filtered out.
Kaplan-Meier plotter
The Kaplan-Meier plotter (http://kmplot.com/analysis/) integrates gene expression and clinical data and has been used to determine the prognostic value of specific genes in liver cancer, breast cancer, ovarian cancer, lung cancer, and gastric cancer (22-25). The Kaplan-Meier curves were selected to compare the differences in overall survival (OS), relapse-free survival (RFS), progression-free survival (PFS), and disease-specific survival (DSS) between the low-EIF2S2 mRNA group and the high-EIF2S2 mRNA group.
Cox proportional hazards regression model
The Cox regression model of survival package (R version 3.6.3) was applied to analyze the correlations among T stage, pathological stage, tumor status, residual tumor, histological grade, AFP (ng/mL), vascular invasion, EIF2S2, and OS.
cBioPortal
The cBioPortal for Cancer Genomics is an open-access, open-source resource for interactive retrieval, visualization, and analysis of multidimensional cancer genomic data sets. It provides rapid, intuitive, and high-quality access to molecular profiles and clinical attributes from large-scale cancer genomics projects (26). In this study, we retrieved the EIF2S2 genomic profile, which contained mutations, putative copy-number alterations from GISTIC (Genomic Identification of Significant Targets in Cancer), and mRNA expression z-scores (RNASeq V2 RSEM) with a z-score threshold ±2.0. The EIF2S2 mutations were shown by tracts, and their effect on OS, disease-free survival, PFS, and DSS of HCC patients was demonstrated by Kaplan-Meier curves. The statistical significance between the survival curves was analyzed using the log-rank test, where P<0.05 was considered statistically significant.
GEPIA2 and Metascape
The Gene Expression Profiling Interactive Analysis (GEPIA2, http://gepia2.cancer-pku.cn) is an online interactive database (27), which allowed us to explore 50 similar genes related to EIF2S2. Subsequently, we used the enrichment module of Metascape (28) to analyze the Gene Ontology (GO) and Kyoto Encyclopedia of Genes and Genomes (KEGG) pathways to construct a protein-protein interaction network of 50 similar genes related to EIF2S2.
Gene set enrichment analysis (GSEA)
The LinkedOmics database (http://www.linkedomics.org) contains multi-omics data of 32 malignant tumors in TCGA database and also includes mass spectrometry-based proteomics data generated by the Clinical Proteomics Tumor Analysis Consortium (CPTAC) for TCGA breast, colorectal, and ovarian tumors (29). It offers an online platform for biologists and clinicians to retrieve, analyze, and compare cancer multi-omics data within and across tumor types. The LinkInterpreter module was used to perform a GSEA, including GO (cellular components, molecular functions, and biological processes) and KEGG pathways, kinase-target enrichment, miRNA-target enrichment, and transcription factor-target enrichment.
GeneMANIA analysis
GeneMANIA (http://www.genemania.org) has a user-friendly interface, which allowed us to build a protein-protein interaction network, analyze gene functions to generate hypotheses, and prioritize genes for functional assays (30). In this study, GeneMANIA provided the ability to visualize gene networks and predict the function of GSEA gene sets enriched in HCC: kinase MAPK1, miRNA-144, and transcription factor.
Statistical analysis
The data were expressed as the Mean ± SD. The Student’s t-test was used to compare the difference in EIF2S2 mRNA levels between HCC vs normal tissues on the Oncomine and Ualcan database. Basic R package of R software (for statistical computing and graphics, version 3.6.3; https://cran.r-project.org/bin) was used to analyze the RNAseq data and clinicopathological characteristics of liver hepatocellular carcinoma (LIHC) patients in TCGA. The long rank test was applied to analyze the Kaplan-Meier survival curve. The Cox regression model of survival package (R version 3.6.3) was applied to analyze the correlations among T stage, pathological stage, tumor status, residual tumor, histological grade, AFP (ng/mL), vascular invasion, EIF2S2, and OS. P<0.05 was considered statistically significant.
Results
Correlations between EIF2S2 expression and clinicopathological characteristics in HCC
Firstly, the transcriptional level of EIF2S2 was evaluated in three HCC studies from TCGA and the Gene Expression Omnibus (GEO) (see Figure 1). The data from the Oncomine database showed that EIF2S2 mRNA levels and DNA copy number variations (CNVs) were significantly higher in HCC tissues than in normal liver tissues (P<0.001) (Figure 1A). Although the fold-change (1.997) was <2 (Figure 1A), EIF2S2 ranked in the top 4% for mRNA expression and the top 10% according to DNA CNVs (Figure 1B,1C). Based on the TCGA subgroup analysis, which included sex, age, race, tumor grade, and stage, high transcription levels of EIF2S2 were also observed in 371 LIHC samples, which showed a significant difference compared with healthy individuals (Figure 2). The correlations between EIF2S2 expression and clinicopathological characteristics for LIHC patients in TCGA are listed in Table 1. EIF2S2 mRNA upregulation was significantly associated with T stage of tumor (P=0.002), pathologic stage (P=0.002), histologic grade (P<0.001), AFP (P<0.001), vascular invasion (P=0.008), and residual tumor (P=0.025). Most importantly, these findings indicate that overexpressed EIF2S2 participates in the progression of HCC, which also implies EIF2S2 may be a potential prognostic indicator for HCC.
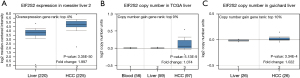
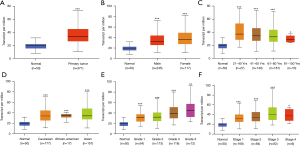
Table 1
Characteristics | Low expression of EIF2S2 | High expression of EIF2S2 | P1 |
---|---|---|---|
n | 187 | 187 | |
T stage, n (%) | 0.002* | ||
T1 | 109 (29.4) | 74 (19.9) | |
T2 | 37 (10.0) | 58 (15.6) | |
T3 | 34 (9.2) | 46 (12.4) | |
T4 | 4 (1.1) | 9 (2.4) | |
N stage, n (%) | 0.622 | ||
N0 | 126 (48.8) | 128 (49.6) | |
N1 | 1 (0.4) | 3 (1.2) | |
M stage, n (%) | 1.000 | ||
M0 | 131 (48.2) | 137 (50.4) | |
M1 | 2 (0.7) | 2 (0.7) | |
Pathologic stage, n (%) | 0.002* | ||
Stage I | 104 (29.7) | 69 (19.7) | |
Stage II | 36 (10.3) | 51 (14.6) | |
Stage III | 33 (9.4) | 52 (14.9) | |
Stage IV | 3 (0.9) | 2 (0.6) | |
Tumor status, n (%) | 0.083 | ||
Tumor free | 111 (31.3) | 91 (25.6) | |
With tumor | 69 (19.4) | 84 (23.7) | |
Gender, n (%) | 0.122 | ||
Female | 53 (14.2) | 68 (18.2) | |
Male | 134 (35.8) | 119 (31.8) | |
Race, n (%) | 0.670 | ||
Asian | 76 (21.0) | 84 (23.2) | |
Black or African American | 7 (1.9) | 10 (2.8) | |
White | 94 (26.0) | 91 (25.1) | |
Age, n (%) | 0.133 | ||
≤60 | 81 (21.7) | 96 (25.7) | |
>60 | 106 (28.4) | 90 (24.1) | |
Histologic grade2, n (%) | <0.001* | ||
G1 | 36 (9.8) | 19 (5.1) | |
G2 | 101 (27.4) | 77 (20.9) | |
G3 | 45 (12.2) | 79 (21.4) | |
G4 | 3 (0.8) | 9 (2.4) | |
AFP (ng/mL), n (%) | <0.001* | ||
≤400 | 129 (46.1) | 86 (30.7) | |
>400 | 18 (6.4) | 47 (16.8) | |
Albumin (g/dL), n (%) | 0.421 | ||
<3.5 | 40 (13.3) | 29 (9.7) | |
≥3.5 | 119 (39.7) | 112 (37.3) | |
Child-Pugh grade, n (%) | 0.906 | ||
A | 115 (47.7) | 104 (43.2) | |
B | 10 (4.1) | 11 (4.6) | |
C | 1 (0.4) | 0 (0) | |
Vascular invasion, n (%) | 0.008* | ||
No | 119 (37.4) | 89 (28.0) | |
Yes | 45 (14.2) | 65 (20.4) | |
Fibrosis Ishak score, n (%) | 0.635 | ||
0 | 45 (20.9) | 30 (14.0) | |
1/2 | 17 (7.9) | 14 (6.5) | |
3/4 | 13 (6.0) | 15 (7.0) | |
5/6 | 43 (20) | 38 (17.7) | |
Residual tumor, n (%) | 0.025* | ||
R0 | 171 (49.6) | 156 (45.2) | |
R1 | 4 (1.2) | 13 (3.8) | |
R2 | 1 (0.3) | 0 (0) | |
Age, median [IQR] | 62 [53–69] | 60 [51–68] | 0.224 |
1, significance of EIF2S2 overexpression correlated with clinicopathological characteristics was calculated using chi-square test. *, P<0.05 is considered statistically significant. 2, Edmonson-Steiner histological grade. EIF2S2, eukaryotic translation initiation factor 2 subunit beta; AFP, α-fetoprotein.
Correlations between EIF2S2 gene mutations and the prognosis of HCC patients
We observed the influence of EIF2S2 gene mutations on the prognosis of patients, using cBioPortal according to the RNA-seq data from LIHC patients in TCGA database. Changes in EIF2S2 were found in 39 (11%) LIHC patients (n=366), of which 38 (10.4%) were mRNA upregulated and one (0.003%) was amplified (Figure 3A). Thus, EIF2S2 mRNA upregulation was the most common type of gene mutation in HCC. Interestingly, the relationship between genetic alterations and OS (Figure 3B, P=0.15), disease-free survival (Figure 3C, P=0.463), PFS (Figure 3D, P=0.855), and DSS (Figure 3E, P=0.338) in HCC patients revealed that there was no correlation between genetic mutations and various survival periods.
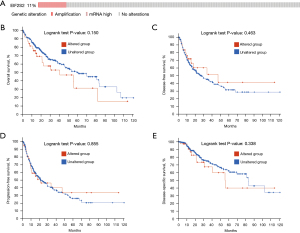
Overexpressed EIF2S2 indicated poor prognosis in HCC patients
We used the Kaplan Meier plotter database to display the relationships between EIF2S2 and HCC prognosis by combining OS, disease-free survival, and RFS with DSS. OS [Figure 4A, hazard ratio (HR) =1.8 (1.27−2.55), logrank P=0.00076], disease-free survival [Figure 4B, HR =1.37 (1.02−1.84), logrank P=0.034], RFS [Figure 4C, HR =1.45 (1.04−2.01), logrank P=0.026] and DSS [Figure 4D, HR =2.09 (1.25−3.51), logrank P=0.0042] were reduced in HCC patients with upregulated EIF2S2 expression.
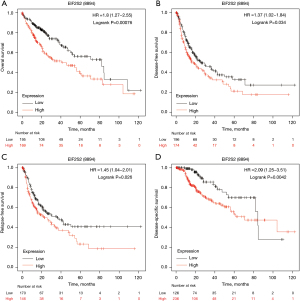
Furthermore, univariate and multivariate Cox proportional hazards regression models were applied to evaluate the correlation between EIF2S2 and clinical prognosis (Table 2). The univariate Cox regression statistical results indicated that patients with overexpressed EIF2S2 had a poor OS (P<0.001) in TCGA-LIHC, together with several clinicopathological features, including tumor stage (P<0.001), pathologic stage (P<0.001), and tumor status (P<0.001). Based on the multivariate analysis, pathologic stage [HR (95% CI) =1.787 (1.094–2.919), P=0.020], and tumor status [HR (95% CI) =1.743 (1.162–2.615), P=0.007] had adverse effects on OS. In TCGA-LIHC, EIF2S2 overexpression was an independent risk factor related to poor prognosis [HR (95% CI) =1.962 (1.320–2.915), P<0.001]. Clearly, upregulation of EIF2S2 serves as an independent high-risk factor underlying poor clinical prognosis.
Table 2
Characteristics | Total (N) | Univariate analysis | Multivariate analysis | |||
---|---|---|---|---|---|---|
Hazard ratio (95% CI) | P value | Hazard ratio (95% CI) | P value | |||
T stage (T2 & T3 & T4 vs. T1) | 370 | 2.126 (1.481–3.052) | <0.001* | 1.257 (0.763–2.071) | 0.369 | |
Pathologic stage (stage III & stage IV vs. stage I & stage II) | 349 | 2.504 (1.727–3.631) | <0.001* | 1.787 (1.094–2.919) | 0.020* | |
Tumor status (with tumor vs. tumor free) | 354 | 2.317 (1.590–3.376) | <0.001* | 1.743 (1.162–2.615) | 0.007* | |
Residual tumor (R1 & R2 vs. R0) | 344 | 1.604 (0.812–3.169) | 0.174 | |||
Histologic grade (G4 & G2 & G3 vs. G1) | 368 | 1.188 (0.721–1.958) | 0.499 | |||
AFP (ng/mL) (>400 vs. ≤400) | 279 | 1.075 (0.658–1.759) | 0.772 | |||
Vascular invasion (yes vs. no) | 317 | 1.344 (0.887–2.035) | 0.163 | |||
EIF2S2 (high vs. low) | 373 | 2.031 (1.423–2.897) | <0.001* | 1.962 (1.320–2.915) | <0.001* |
*, indicates statistically significant, P<0.05. EIF2S2, eukaryotic translation initiation factor 2 subunit beta; HCC, hepatocellular carcinoma; AFP, α-fetoprotein.
EIF2S2 biological interaction network in HCC
GO and KEGG pathway analysis of 50 similar genes correlated with EIF2S2 in HCC
We further analyzed 50 similar genes significantly associated with EIF2S2 using the GEPIA2 database and constructed an integrated network with Metascape (https://metascape.org). The 50 similar genes are shown in Table S1. Metascape was used to enrich the GO and KEGG terms. The top 19 clusters with their representative enriched terms are shown in Figure 5A. In addition, based on the enriched GO and KEGG terms, a protein-protein interaction network is shown in Figures 5B,5C. The top three best P value GO terms were as follows: go: 1900182 was involved in the positive regulation of protein localization to the nucleus; go: 1903829 participated in the positive regulation of cellular protein localization; and go: 0008022 played a part in protein C-terminus binding.
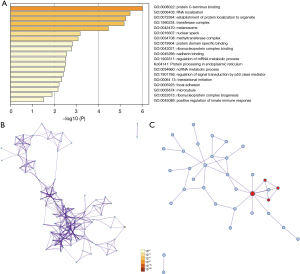
Moreover, the LinkedOmics function module was applied to analyze the mRNA sequencing data of 371 LIHC patients in TCGA database. In the LinkFinder module, the volcano plot (Figure 6A) shows 5,123 genes (dark red dot) to be significantly positively correlated with EIF2S2, while 4,690 genes (dark green dot) were significantly negatively correlated with EIF2S2 [false discovery rate (FDR) <0.01]. The heat map illustrates the top 50 genes that were positively (Figure 6B) and negatively (Figure 6C) related to EIF2S2. We also found that prefoldin 4 (PFDN4), histocompatibility minor 13 (HM13), and small nuclear ribonucleoprotein D1 polypeptide (SNRPD1) were the top three genes significantly and positively correlated to EIF2S2, as shown in Figure S1. These results indicate that EIF2S2 has a widespread effect on gene transcription.
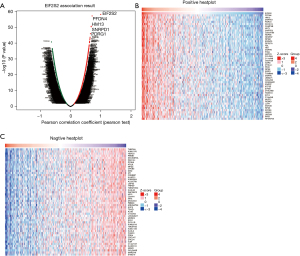
In the LinkInterpreter module, the top GO-term analysis by GSEA revealed that genes enriched in association with EIF2S2 were located mainly in the ribosome, mitochondrial protein complex, and cytosolic part (Figure 7A, Table S2), where they were primarily involved in the structural constituent of ribosome, unfolded protein binding, and rRNA binding (Figure 7B, Table S3) and participated in ribonucleoprotein complex biogenesis, translational initiation, and the rRNA metabolic process (Figure 7C, Table S4). The KEGG pathway was enriched in the ribosome, oxidative phosphorylation, spliceosome, and proteasome pathways (Figure 7D,7E, and Table S5).
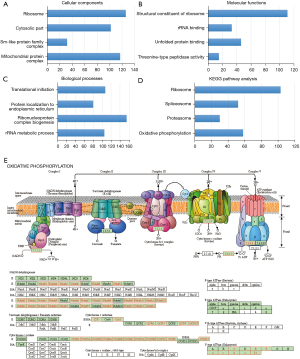
The EIF2S2 kinase, miRNA, and transcription factor target networks in HCC
The target networks of EIF2S2 were thoroughly explored using the GSEA tool in LinkedOmics. The top five kinase targets (P<0.05) were mitogen-activated protein kinase 1 (MAPK1), mitogen-activated protein kinase kinase kinase 6 (MAP3K6), dual specificity tyrosine phosphorylation regulated kinase 1A (DYRK1A), casein kinase 2 alpha 1 (CSNK2A1), and Pim-1 proto-oncogene, serine/threonine kinase (PIM1) (Table 3 and Table S6). The top five miRNA targets were related to (ATACTGT) MIR-144; (TAGGTCA) MIR-192, MIR-215; (TGTTTAC) MIR-30A-5P, MIR-30C, MIR-30D, MIR-30B, MIR-30E-5P; (TACTTGA) MIR-26A, MIR-26B; and (GCTTGAA) MIR-498 (Table 3 and Table S7). The top five transcription factor targets were associated with GGAANCGGAANY_UNKNOWN, V$FREAC2_01, YNGTTNNNATT_UNKNOWN, V$FREAC4_01, and V$FOXO4_02 (Table 3 and Table S8).
Table 3
Enriched category | Geneset | LeadingEdgeNum | P value | FDR |
---|---|---|---|---|
Kinase target | Kinase_MAPK1 | 54 | 0 | 0.31931 |
Kinase_MAP3K6 | 1 | 0.0042918 | 0.30758 | |
Kinase_DYRK1A | 7 | 0.0043668 | 0.35358 | |
Kinase_CSNK2A1 | 84 | 0.0068493 | 0.63526 | |
Kinase_PIM1 | 8 | 0.011765 | 0.29544 | |
miRNA target | ATACTGT, MIR-144 | 59 | 0 | 0 |
TAGGTCA, MIR-192, MIR-215 | 17 | 0 | 0 | |
TGTTTAC, MIR-30A-5P, MIR-30C, MIR-30D, MIR-30B, MIR-30E-5P | 184 | 0 | 0 | |
TACTTGA, MIR-26A, MIR-26B | 82 | 0 | 0 | |
GCTTGAA, MIR-498 | 33 | 0 | 0 | |
Transcription factor target | GGAANCGGAANY_UNKNOWN | 38 | 0 | 0 |
V$FREAC2_01 | 60 | 0 | 0 | |
YNGTTNNNATT_UNKNOWN | 79 | 0 | 0 | |
V$FREAC4_01 | 42 | 0 | 0.000724 | |
V$FOXO4_02 | 75 | 0 | 0.000905 |
V$, the annotation found in Molecular Signatures; Database (MSigDB) for transcription factors (TF). EIF2S2, eukaryotic translation initiation factor 2 subunit beta; HCC, hepatocellular carcinoma; LeadingEdgeNum, the number of leading edge genes; FDR, false discovery rate from Benjamini and Hochberg from gene set enrichment analysis (GSEA).
The protein-protein interaction network generated with GeneMANIA demonstrated relationships among genes relating to the kinases MAPK1, miRNA-144, and GGAANCGGAANY_UNKNOWN. The genes enriched for kinase MAPK1 participated in RNA polymerase II-specific DNA-binding transcription factor binding, positive regulation of transcription by RNA polymerase II, and DNA-templated transcription, initiation (Figure 8). The enriched genes for miRNA-144 were mainly involved in ligand-activated transcription factor activity, regulation of cytoplasmic translation, RNA polymerase II-specific DNA-binding transcription factor binding, and DNA-templated transcription, initiation (Figure S2). The enriched genes for transcription factor play a part in ribosome, translation factor activity, RNA binding, ribosomal subunit, translation regulator activity, nucleic acid binding, translational termination, and protein modification by small protein removal (Figure S3).
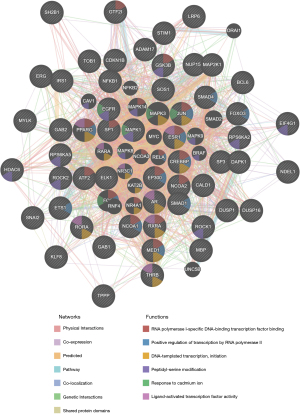
Discussion
The present study primarily focused on exploring the prognostic role and interaction networks of EIF2S2 in HCC using bioinformatics data. High EIF2S2 mRNA levels were expressed in HCC and were positively correlated with clinically malignant features. Furthermore, we proved that EIF2S2 was an independent prognostic marker for HCC and played a critical role in HCC gene-regulating networks.
Over the last decade, RNA binding proteins (RBPs) have attracted growing attention in multiple diseases (31), particularly in tumors such as triple-negative breast cancer (32), clear cell renal cell carcinoma (33), and pancreatic cancer (34). RBPs also play a role in HCC (35-38). As a result, an increasing number of genes related to the RBP family have now been identified in various types of cancers, including RBP-Nudt21 (39), SRSF10 (40), IGF2BP3 (41), CELF2 (42), MEX3A (43), RBM47 (44), and SERBP1 (45). As a novel member of the RBP family, EIF2S2 has recently been linked to tumor prognosis. In the current study, EIF2S2 mRNA levels in HCC tissues were overexpressed and were significantly correlated with clinicopathological features. The upregulation of EIF2S2 mRNA in HCC was in agreement with previous studies of other tumors (18). Importantly, our results suggested that high EIF2S2 expression in HCC patients was associated with a significantly shorter survival period than low EIF2S2 expression. But the underlying mechanism involved in the overexpression of EIF2S2 mRNA needs further exploration.
Next generation sequencing has identified EIF2S2 as one of the 18 known “variant” genes in primary ovarian insufficiency (POI), which implies that an EIF2S2 genetic abnormality increases the risk of POI (16). In this study, the mutation rate of EIF2S2 remained quite low in HCC. The finding that there was no correlation between EIF2S2 genetic mutation and HCC survival periods indirectly suggests that EIF2S2 might be a crucial prognostic factor for HCC survival.
RBPs play multiple biological roles through diverse mechanisms (31,35,46), and this is consistent with our GSEA-based findings. In the following paragraphs, we discuss EIF2S2-related genes and possible signal pathways. Using LinkedOmics, we screened the top three genes (PFDN4, HM13, and SNRPD1) that were strongly positively correlated with EIF2S2 and which are known to play important roles in various tumors (47-58). Overexpressed PFDN4 in colorectal cancer predicts a better prognosis (47), but the opposite was found in HCC, probably due to tissue specificity. HM13 expression has been revealed to be closely related to prognosis and survival in glioblastoma (52). And SNRPD1, a member of the SNRP nuclear protein family, was shown to be significantly overexpressed in invasive neuroblastoma, providing a promising biomarker for clinical prognosis (58). In HCC, EIF2S2 may affect the prognosis of HCC patients via PFDN4, HM13, or SNRPD1.
Subsequently, we established a protein-protein interaction network of 50 similar gene-related GO and KEGG terms in HCC, which were mainly concentrated in ribosome, oxidative phosphorylation, spliceosome, and proteasome. EIF2S2 may play a role in the occurrence and progression of tumors through the KEGG pathways related to ribosome (59-62), oxidative phosphorylation (63-65), spliceosome (66-68), and proteasome (69,70). In addition, the data from the GO terms demonstrated that EIF2S2 participated in protein localization, synthesis, and mRNA maturation, as well as in the development of various tumors. In consideration of strong positive correlation between PFDN4, HM13, SNRPD1 and EIF2S2, the mRNA maturation, protein synthesis and localization of the above three genes may be the underlying mechanism that EIF2S2 promotes the HCC progression. We have shown that EIF2S2 in HCC is related to a network of kinases, of which the top five most highly correlated are MAPK1, MAP3K6, DYRK1A, CSNK2A1, and PIM1. Here, we only discuss the relationship between kinase MAPK1 and EIF2S2. It is well established that the MAPK1 signaling pathway participates in the occurrence and development of many tumors, including HCC. Kinase MAPK1 (71-74) plays multiple roles in HCC. For example, lncRNA H19 was shown to be involved in HCC aggressiveness through the miR-193b/MAPK1 axis, bridged the crosstalk between HCC and the immunological microenvironment, and resulted in poor clinical outcome (74). The activation of MAPK/ERK may play a vital catalytic role in the multistep development of HCC (71). Therefore, we assume that EIF2S2 might regulate the progression of HCC via the MAPK1 kinase.
Similarly, our data also distinguished the top five target miRNAs associated with EIF2S2. It is well known that miRNAs play an important role in tumor development. The target miRNA ranking first in the correlation with EIF2S2 was Mir-144. But Mir-144 inhibited the development of HCC (75-80), displaying an opposite function compared with EIF2S2. We will need to further verify whether EIF2S2 works through Mir-144. The first-ranked predicted transcription factor target (GGAANCGGAANY_UNKNOWN) has not been reported previously. Both the predicted miRNA and transcription factor targets need to be further investigated. And Zhang reports that EIF2S2 accelerates cell proliferation, migration, and invasion of colorectal cancer via the EIF2S2-LINC01600-MYC axis (18). We need to further explore the same or similar molecular mechanisms in HCC.
In our current study, data mining based on R and online tools was used to analyze tumor data from public databases. This strategy affords high efficiency, a large sample size, low cost, great credibility, and makes the direction of follow-up scientific research clearer. However, TCGA database also has some limitations. The biological functions are performed only in the transcription levels, not in the protein and protein activity levels. The genetic background and etiology of tumors in the three ethnic groups of TCGA LIHC samples are different and the number of patients with stage 4 tumors in the LIHC sample size is small, which may be attributed to the low surgical rate in patients with advanced stage and the relatively high difficulty in obtaining specimens.
In summary, our study has provided multidimensional evidence for prognostic values and gene regulation networks of EIF2S2 in HCC. All the findings from the bioinformatics analyses indicated that EIF2S2 may be a powerful prognostic factor in HCC. EIF2S2 can be developed as a prognostic biomarker for HCC and the development of EIF2S2-specific inhibitors is a good opinion to prevent HCC. However, the interactions of EIF2S2-related genes and biological interaction networks need verification by future studies.
Conclusions
In brief, by using a prognostic value and gene interaction network analysis, our integrative study reveals that EIF2S2 is closely related to HCC prognosis. Therefore, EIF2S2 can be considered as a promising prognostic biomarker or therapeutic target for HCC.
Acknowledgments
Funding: This work was supported by the National Natural Science Foundation of China (82101321), the Yantai Science and Technology Planning Project (2021YD066), and the Shandong Provincial Natural Science Foundation (ZR2021MH249, ZR2018PH017).
Footnote
Reporting Checklist: The authors have completed the REMARK reporting checklist. Available at https://dx.doi.org/10.21037/jgo-21-748
Conflicts of Interest: All authors have completed the ICMJE uniform disclosure form (available at https://dx.doi.org/10.21037/jgo-21-748). The authors have no conflicts of interest to declare.
Ethical Statement: The authors are accountable for all aspects of the work in ensuring that questions related to the accuracy or integrity of any part of the work are appropriately investigated and resolved. The study was conducted in accordance with the Declaration of Helsinki (as revised in 2013).
Open Access Statement: This is an Open Access article distributed in accordance with the Creative Commons Attribution-NonCommercial-NoDerivs 4.0 International License (CC BY-NC-ND 4.0), which permits the non-commercial replication and distribution of the article with the strict proviso that no changes or edits are made and the original work is properly cited (including links to both the formal publication through the relevant DOI and the license). See: https://creativecommons.org/licenses/by-nc-nd/4.0/.
References
- Sung H, Ferlay J, Siegel RL, et al. Global Cancer Statistics 2020: GLOBOCAN Estimates of Incidence and Mortality Worldwide for 36 Cancers in 185 Countries. CA Cancer J Clin 2021;71:209-49. [Crossref] [PubMed]
- Golabi P, Fazel S, Otgonsuren M, et al. Mortality assessment of patients with hepatocellular carcinoma according to underlying disease and treatment modalities. Medicine (Baltimore) 2017;96:e5904. [Crossref] [PubMed]
- Siegel RL, Miller KD, Jemal A. Cancer statistics, 2019. CA Cancer J Clin 2019;69:7-34. [Crossref] [PubMed]
- Bray F, Ferlay J, Soerjomataram I, et al. Global cancer statistics 2018: GLOBOCAN estimates of incidence and mortality worldwide for 36 cancers in 185 countries. CA Cancer J Clin 2018;68:394-424. [Crossref] [PubMed]
- Eberhardt W, Doller A. Modulation of mRNA stability as a novel therapeutic approach. Pharmacol Ther 2007;114:56-73. [Crossref] [PubMed]
- Schoenberg DR, Maquat LE. Regulation of cytoplasmic mRNA decay. Nat Rev Genet 2012;13:246-59. [Crossref] [PubMed]
- Majumder M, Palanisamy V. RNA binding protein FXR1-miR301a-3p axis contributes to p21WAF1 degradation in oral cancer. PLoS Genet 2020;16:e1008580. [Crossref] [PubMed]
- Zhao J, Zhang Y, Liu XS, et al. RNA-binding protein Musashi2 stabilizing androgen receptor drives prostate cancer progression. Cancer Sci 2020;111:369-82. [Crossref] [PubMed]
- Jiao Y, Fu Z, Li Y, et al. High EIF2B5 mRNA expression and its prognostic significance in liver cancer: a study based on the TCGA and GEO database. Cancer Manag Res 2018;10:6003-14. [Crossref] [PubMed]
- Liu F, Visser M, Duffy DL, et al. Genetics of skin color variation in Europeans: genome-wide association studies with functional follow-up. Hum Genet 2015;134:823-35. [Crossref] [PubMed]
- Aydin B, Arga KY. Co-expression Network Analysis Elucidated a Core Module in Association With Prognosis of Non-functioning Non-invasive Human Pituitary Adenoma. Front Endocrinol (Lausanne) 2019;10:361. [Crossref] [PubMed]
- Mendoza MN, Raudsepp T, Alshanbari F, et al. Chromosomal Localization of Candidate Genes for Fiber Growth and Color in Alpaca (Vicugna pacos). Front Genet 2019;10:583. [Crossref] [PubMed]
- Li N, Qian S, Li B, et al. Quantitative analysis of the human ovarian carcinoma mitochondrial phosphoproteome. Aging (Albany NY) 2019;11:6449-68. [Crossref] [PubMed]
- Heaney JD, Michelson MV, Youngren KK, et al. Deletion of eIF2beta suppresses testicular cancer incidence and causes recessive lethality in agouti-yellow mice. Hum Mol Genet 2009;18:1395-404. [Crossref] [PubMed]
- Gatza ML, Silva GO, Parker JS, et al. An integrated genomics approach identifies drivers of proliferation in luminal-subtype human breast cancer. Nat Genet 2014;46:1051-9. [Crossref] [PubMed]
- Eskenazi S, Bachelot A, Hugon-Rodin J, et al. Next Generation Sequencing Should Be Proposed to Every Woman With "Idiopathic" Primary Ovarian Insufficiency. J Endocr Soc 2021;5:bvab032.
- Liang KH, Wang ML. Deep proteogenomic investigations elucidate the NRF2 antioxidant mechanism as a major driving mechanism of lung adenocarcinoma in Asia. J Chin Med Assoc 2021;84:766-71. [Crossref] [PubMed]
- Zhang J, Li S, Zhang L, et al. RBP EIF2S2 Promotes Tumorigenesis and Progression by Regulating MYC-Mediated Inhibition via FHIT-Related Enhancers. Mol Ther 2020;28:1105-18. [Crossref] [PubMed]
- Rhodes DR, Yu J, Shanker K, et al. ONCOMINE: a cancer microarray database and integrated data-mining platform. Neoplasia 2004;6:1-6. [Crossref] [PubMed]
- Chandrashekar DS, Bashel B, Balasubramanya SAH, et al. UALCAN: A Portal for Facilitating Tumor Subgroup Gene Expression and Survival Analyses. Neoplasia 2017;19:649-58. [Crossref] [PubMed]
- Tomczak K, Czerwińska P, Wiznerowicz M. The Cancer Genome Atlas (TCGA): an immeasurable source of knowledge. Contemp Oncol (Pozn) 2015;19:A68-77. [Crossref] [PubMed]
- Szász AM, Lánczky A, Nagy Á, et al. Cross-validation of survival associated biomarkers in gastric cancer using transcriptomic data of 1,065 patients. Oncotarget 2016;7:49322-33. [Crossref] [PubMed]
- Györffy B, Lanczky A, Eklund AC, et al. An online survival analysis tool to rapidly assess the effect of 22,277 genes on breast cancer prognosis using microarray data of 1,809 patients. Breast Cancer Res Treat 2010;123:725-31. [Crossref] [PubMed]
- Gyorffy B, Lánczky A, Szállási Z. Implementing an online tool for genome-wide validation of survival-associated biomarkers in ovarian-cancer using microarray data from 1287 patients. Endocr Relat Cancer 2012;19:197-208. [Crossref] [PubMed]
- Győrffy B, Surowiak P, Budczies J, et al. Online survival analysis software to assess the prognostic value of biomarkers using transcriptomic data in non-small-cell lung cancer. PLoS One 2013;8:e82241. [Crossref] [PubMed]
- Gao J, Aksoy BA, Dogrusoz U, et al. Integrative analysis of complex cancer genomics and clinical profiles using the cBioPortal. Sci Signal 2013;6:pl1. [Crossref] [PubMed]
- Tang Z, Kang B, Li C, et al. GEPIA2: an enhanced web server for large-scale expression profiling and interactive analysis. Nucleic Acids Res 2019;47:W556-60. [Crossref] [PubMed]
- Zhou Y, Zhou B, Pache L, et al. Metascape provides a biologist-oriented resource for the analysis of systems-level datasets. Nat Commun 2019;10:1523. [Crossref] [PubMed]
- Vasaikar SV, Straub P, Wang J, et al. LinkedOmics: analyzing multi-omics data within and across 32 cancer types. Nucleic Acids Res 2018;46:D956-63. [Crossref] [PubMed]
- Franz M, Rodriguez H, Lopes C, et al. GeneMANIA update 2018. Nucleic Acids Res 2018;46:W60-4. [Crossref] [PubMed]
- Castello A, Fischer B, Eichelbaum K, et al. Insights into RNA biology from an atlas of mammalian mRNA-binding proteins. Cell 2012;149:1393-406. [Crossref] [PubMed]
- Kim SJ, Ju JS, Kang MH, et al. RNA-binding protein NONO contributes to cancer cell growth and confers drug resistance as a theranostic target in TNBC. Theranostics 2020;10:7974-92. [Crossref] [PubMed]
- Li CX, Chen J, Xu ZG, et al. The expression and prognostic value of RNA binding proteins in clear cell renal cell carcinoma. Transl Cancer Res 2020;9:7415-31. [Crossref]
- Xu YF, Xu X, Gin A, et al. SRSF1 regulates exosome microRNA enrichment in human cancer cells. Cell Commun Signal 2020;18:130. [Crossref] [PubMed]
- Dang H, Takai A, Forgues M, et al. Oncogenic Activation of the RNA Binding Protein NELFE and MYC Signaling in Hepatocellular Carcinoma. Cancer Cell 2017;32:101-114.e8. [Crossref] [PubMed]
- Yang X, Qu S, Wang L, et al. PTBP3 splicing factor promotes hepatocellular carcinoma by destroying the splicing balance of NEAT1 and pre-miR-612. Oncogene 2018;37:6399-413. [Crossref] [PubMed]
- Wang M, Huang S, Chen Z, et al. Development and validation of an RNA binding protein-associated prognostic model for hepatocellular carcinoma. BMC Cancer 2020;20:1136. [Crossref] [PubMed]
- Wang Q, Guo Y, Wang W, et al. RNA binding protein DAZAP1 promotes HCC progression and regulates ferroptosis by interacting with SLC7A11 mRNA. Exp Cell Res 2021;399:112453. [Crossref] [PubMed]
- Brumbaugh J, Di Stefano B, Wang X, et al. Nudt21 Controls Cell Fate by Connecting Alternative Polyadenylation to Chromatin Signaling. Cell 2018;172:106-120.e21. [Crossref] [PubMed]
- Liu X, Shen S, Zhu L, et al. SRSF10 inhibits biogenesis of circ-ATXN1 to regulate glioma angiogenesis via miR-526b-3p/MMP2 pathway. J Exp Clin Cancer Res 2020;39:121. [Crossref] [PubMed]
- Li K, Huang F, Li Y, et al. Stabilization of oncogenic transcripts by the IGF2BP3/ELAVL1 complex promotes tumorigenicity in colorectal cancer. Am J Cancer Res 2020;10:2480-94. [PubMed]
- Guo Q, Wu Y, Guo X, et al. The RNA-Binding Protein CELF2 Inhibits Ovarian Cancer Progression by Stabilizing FAM198B. Mol Ther Nucleic Acids 2021;23:169-84. [Crossref] [PubMed]
- Panzeri V, Manni I, Capone A, et al. The RNA-binding protein MEX3A is a prognostic factor and regulator of resistance to gemcitabine in pancreatic ductal adenocarcinoma. Mol Oncol 2021;15:579-95. [Crossref] [PubMed]
- Shen DJ, Jiang YH, Li JQ, et al. The RNA-binding protein RBM47 inhibits non-small cell lung carcinoma metastasis through modulation of AXIN1 mRNA stability and Wnt/β-catentin signaling. Surg Oncol 2020;34:31-9. [Crossref] [PubMed]
- Kosti A, de Araujo PR, Li WQ, et al. The RNA-binding protein SERBP1 functions as a novel oncogenic factor in glioblastoma by bridging cancer metabolism and epigenetic regulation. Genome Biol 2020;21:195. [Crossref] [PubMed]
- Gerstberger S, Hafner M, Tuschl T. A census of human RNA-binding proteins. Nat Rev Genet 2014;15:829-45. [Crossref] [PubMed]
- Miyoshi N, Ishii H, Mimori K, et al. Abnormal expression of PFDN4 in colorectal cancer: a novel marker for prognosis. Ann Surg Oncol 2010;17:3030-6. [Crossref] [PubMed]
- Urabe F, Kosaka N, Sawa Y, et al. miR-26a regulates extracellular vesicle secretion from prostate cancer cells via targeting SHC4, PFDN4, and CHORDC1. Sci Adv 2020;6:eaay3051. [Crossref] [PubMed]
- Sadeghi H, Nazemalhosseini-Mojarad E, Sahebi U, et al. Novel long noncoding RNAs upregulation may have synergistic effects on the CYP24A1 and PFDN4 biomarker role in human colorectal cancer. J Cell Physiol 2021;236:2051-7. [Crossref] [PubMed]
- Wang D, Zhu ZZ, Jiang H, et al. Multiple genes identified as targets for 20q13.12-13.33 gain contributing to unfavorable clinical outcomes in patients with hepatocellular carcinoma. Hepatol Int 2015;9:438-46. [Crossref] [PubMed]
- Goovaerts T, Steyaert S, Vandenbussche CA, et al. A comprehensive overview of genomic imprinting in breast and its deregulation in cancer. Nat Commun 2018;9:4120. [Crossref] [PubMed]
- Wei JW, Cai JQ, Fang C, et al. Signal Peptide Peptidase, Encoded by HM13, Contributes to Tumor Progression by Affecting EGFRvIII Secretion Profiles in Glioblastoma. CNS Neurosci Ther 2017;23:257-65. [Crossref] [PubMed]
- Yang W, Xia Y, Qian X, et al. Co-expression network analysis identified key genes in association with mesenchymal stem cell osteogenic differentiation. Cell Tissue Res 2019;378:513-29. [Crossref] [PubMed]
- Li Y, Fong KW, Tang M, et al. Fam118B, a newly identified component of Cajal bodies, is required for Cajal body formation, snRNP biogenesis and cell viability. J Cell Sci 2014;127:2029-39. [PubMed]
- Little JT, Jurica MS. Splicing factor SPF30 bridges an interaction between the prespliceosome protein U2AF35 and tri-small nuclear ribonucleoprotein protein hPrp3. J Biol Chem 2008;283:8145-52. [Crossref] [PubMed]
- Quidville V, Alsafadi S, Goubar A, et al. Targeting the deregulated spliceosome core machinery in cancer cells triggers mTOR blockade and autophagy. Cancer Res 2013;73:2247-58. [Crossref] [PubMed]
- Kim YD, Lee J, Kim HS, et al. The unique spliceosome signature of human pluripotent stem cells is mediated by SNRPA1, SNRPD1, and PNN. Stem Cell Res 2017;22:43-53. [Crossref] [PubMed]
- Batra R, Harder N, Gogolin S, et al. Time-lapse imaging of neuroblastoma cells to determine cell fate upon gene knockdown. PLoS One 2012;7:e50988. [Crossref] [PubMed]
- Derenzini M, Montanaro L, Trerè D. Ribosome biogenesis and cancer. Acta Histochem 2017;119:190-7. [Crossref] [PubMed]
- Bustelo XR, Dosil M. Ribosome biogenesis and cancer: basic and translational challenges. Curr Opin Genet Dev 2018;48:22-9. [Crossref] [PubMed]
- Pelletier J, Thomas G, Volarević S. Ribosome biogenesis in cancer: new players and therapeutic avenues. Nat Rev Cancer 2018;18:51-63. [Crossref] [PubMed]
- Catez F, Dalla Venezia N, Marcel V, et al. Ribosome biogenesis: An emerging druggable pathway for cancer therapeutics. Biochem Pharmacol 2019;159:74-81. [Crossref] [PubMed]
- Boukalova S, Hubackova S, Milosevic M, et al. Dihydroorotate dehydrogenase in oxidative phosphorylation and cancer. Biochim Biophys Acta Mol Basis Dis 2020;1866:165759. [Crossref] [PubMed]
- Ashton TM, McKenna WG, Kunz-Schughart LA, et al. Oxidative Phosphorylation as an Emerging Target in Cancer Therapy. Clin Cancer Res 2018;24:2482-90. [Crossref] [PubMed]
- Vander Heiden MG, Cantley LC, Thompson CB. Understanding the Warburg effect: the metabolic requirements of cell proliferation. Science 2009;324:1029-33. [Crossref] [PubMed]
- Matera AG, Wang Z. A day in the life of the spliceosome. Nat Rev Mol Cell Biol 2014;15:108-21. [Crossref] [PubMed]
- Wang E, Aifantis I. RNA Splicing and Cancer. Trends Cancer 2020;6:631-44. [Crossref] [PubMed]
- Lee SC, Abdel-Wahab O. Therapeutic targeting of splicing in cancer. Nat Med 2016;22:976-86. [Crossref] [PubMed]
- Chen Y, Zhang Y, Guo X. Proteasome dysregulation in human cancer: implications for clinical therapies. Cancer Metastasis Rev 2017;36:703-16. [Crossref] [PubMed]
- Mofers A, Pellegrini P, Linder S, et al. Proteasome-associated deubiquitinases and cancer. Cancer Metastasis Rev 2017;36:635-53. [Crossref] [PubMed]
- Ito Y, Sasaki Y, Horimoto M, et al. Activation of mitogen-activated protein kinases/extracellular signal-regulated kinases in human hepatocellular carcinoma. Hepatology 1998;27:951-8. [Crossref] [PubMed]
- Fu X, Zhang J, He X, et al. Circular RNA MAN2B2 promotes cell proliferation of hepatocellular carcinoma cells via the miRNA-217/MAPK1 axis. J Cancer 2020;11:3318-26. [Crossref] [PubMed]
- Wang H, Ke J, Guo Q, et al. Long non-coding RNA CRNDE promotes the proliferation, migration and invasion of hepatocellular carcinoma cells through miR-217/MAPK1 axis. J Cell Mol Med 2018;22:5862-76. [Crossref] [PubMed]
- Ye Y, Guo J, Xiao P, et al. Macrophages-induced long noncoding RNA H19 up-regulation triggers and activates the miR-193b/MAPK1 axis and promotes cell aggressiveness in hepatocellular carcinoma. Cancer Lett 2020;469:310-22. [Crossref] [PubMed]
- Wu M, Huang C, Huang X, et al. MicroRNA-144-3p suppresses tumor growth and angiogenesis by targeting SGK3 in hepatocellular carcinoma. Oncol Rep 2017;38:2173-81. [Crossref] [PubMed]
- Gu J, Liu X, Li J, et al. MicroRNA-144 inhibits cell proliferation, migration and invasion in human hepatocellular carcinoma by targeting CCNB1. Cancer Cell Int 2019;19:15. [Crossref] [PubMed]
- Bao H, Li X, Li H, et al. MicroRNA-144 inhibits hepatocellular carcinoma cell proliferation, invasion and migration by targeting ZFX. J Biosci 2017;42:103-11. [Crossref] [PubMed]
- Ma Y, She XG, Ming YZ, et al. MicroRNA-144 suppresses tumorigenesis of hepatocellular carcinoma by targeting AKT3. Mol Med Rep 2015;11:1378-83. [Crossref] [PubMed]
- Yu M, Lin Y, Zhou Y, et al. MiR-144 suppresses cell proliferation, migration, and invasion in hepatocellular carcinoma by targeting SMAD4. Onco Targets Ther 2016;9:4705-14. [Crossref] [PubMed]
- Cao T, Li H, Hu Y, et al. miR-144 suppresses the proliferation and metastasis of hepatocellular carcinoma by targeting E2F3. Tumour Biol 2014;35:10759-64. [Crossref] [PubMed]
(English Language Editor: D. Fitzgerald)