Nomogram for the prediction of lymph node metastasis and survival outcomes in rectal neuroendocrine tumour patients undergoing resection
Introduction
The worldwide incidence of neuroendocrine neoplasms (NENs) has risen substantially in recent decades (1,2). The rectum is the most frequent site of digestive tract NENs (2). Additionally, the prevalence of rectal neuroendocrine tumours (RNETs) has steadily increased, reaching approximately 1.2/100,000 per year in 2012 (3). RNETs are increasingly diagnosed due to the widespread use of colonoscopy for colorectal tumour screening.
NENs are considered tumours with indolent biological behaviour and a relatively favourable prognosis (4). RNETs are no exception, and small low-grade lesions localized to the submucosa are present in most RNET cases, which leads to an excellent prognosis and a median survival time of approximately 24.6 years (2); however, the survival of RNET patients can worsen if risk factors are present, including large tumour size, lymph node metastasis and distant metastasis (5-7). The tumour size of RNETs is an important factor for survival outcomes and treatment strategies (5-7). Treatment strategies are recommended based on RNET tumour sizes. According to the current National Comprehensive Cancer Network (NCCN) guidelines (8), RNETs >2 cm should be treated with radical resection, while RNETs <1 cm should be treated with transanal or endoscopic excision. However, there is no clear consensus in the NCCN guidelines on the treatment of 1 to 2 cm RNETs. Additional assessment of the depth of invasion and the status of the lymph nodes has been recommended to help guide the type of resection used for 1- to 2-cm RNET patients. Furthermore, the prediction of lymph node metastasis is critical, not only because lymph node metastasis is an important factor affecting the prognosis of RNET patients (9), but also because the evaluation of lymph node metastasis is essential for determining the best surgical strategy, local excision or radical rectal resection. When lymph node metastasis is highly suspected, radical rectal resection with lymph node dissection should be performed. It is necessary to construct predictive models to predict the status of lymph nodes before treatment and survival outcomes after resection in RNET patients, which will be helpful for treatment decision-making and surveillance. To date, few studies have focused on this demand.
In this study, we aimed to identify predictive factors and construct nomograms for lymph node metastasis and survival outcomes in RNET patients using the population-based Surveillance, Epidemiology, and End Results (SEER) database. We present the following article in accordance with the TRIPOD reporting checklist (available at https://jgo.amegroups.com/article/view/10.21037/jgo-21-573/rc).
Methods
Data collection and patient population
Information about the patients enrolled in this study was collected from the Incidence-SEER 18-registry Research Data (10). We extracted data on patients diagnosed with NETs located in the rectum between 2000 and 2018 by using SEER*Stat software (version 8.3.9) (8). The inclusion criteria were as follows: (I) pathologically diagnosed RNETs and (II) underwent surgery. The exclusion criteria were as follows: (I) with another malignant tumour; (II) survival time <1 month; (III) age <18 years; and (IV) incomplete patient information. Overall, 1253 eligible patients were selected for this study (Figure 1). No personal identifying information was used in the study; therefore, we did not require any informed consent. The study was conducted in accordance with the Declaration of Helsinki (as revised in 2013).
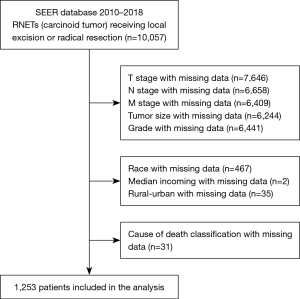
Variable declaration
Clinicopathological variables included age at diagnosis, sex, race, histological grade, T stage (AJCC, 7th ed.), N stage (AJCC, 7th ed.), M stage (AJCC, 7th ed.), tumour size, survival time, cause of death etc. were retrieved. AJCC 7th TNM stage was divided into stages I through IV according to T stage, N stage and M stage. The optimal cut-off value of tumour size for survival was obtained by X-tile analysis. The duration between the date of diagnosis and the date of death due to RNETs was calculated as the cancer-specific survival (CSS) time. The duration between the date of diagnosis and the date of death due to any cause was calculated as the overall survival (OS) time.
Statistical analysis
Continuous variables were expressed as medians [interquartile ranges (IQRs)], whereas categorical variables were presented as counts and percentages. The chi-square test or Fisher’s exact test was used to compare the differences in categorical variables. The optimal cut-off value of tumour size for survival was determined by X-title analysis. Survival comparisons were conducted using Kaplan-Meier analysis and log-rank tests.
Predictors with P<0.10 in the univariate analysis were retained in the multivariate models. A multivariable analysis of the relationships between clinicopathological parameters and lymph node metastasis was performed by logistic regression analysis model. To complete competing the risk analysis, the noncancer-specific death rate was employed as the competing factor, and Grey’s test was used to identify significant differences between groups. The risk factors associated with the survival outcomes of the patients were identified by the Fine-Grey multivariable regression model, and the results were presented as subdistribution hazard ratios (SHRs) and the associated 95% confidence interval (CI). Through the Cox regression model, a multivariable analysis of the OS was performed, which was presented as the HR and 95% CI. P<0.05 was considered statistically significant. All statistically significant risk factors were used to construct the nomogram. The discrimination ability of the model was performed by area under the receiver operating characteristic curve (AUC) and C-index. To evaluate the model calibration, calibration plots and the Hosmer-Lemeshow chi-square test were performed. The Brier score was used to measure the overall performance of the model, covering all aspects of calibration and discrimination. The score ranges from 0 to 1, and the closer the value is to 0, the better the prediction performance of the model. Statistical significance was achieved if the two-tailed P value was <0.05. SPSS Statistics 25.0 (IBM Corporation, Armonk, NY, USA) and R version 3.5.1 (https://www.r-project.org/) were used to perform statistical analyses.
Results
Clinicopathological characteristics
A total of 10,057 patients diagnosed with RNETs from 2010 to 2018 were screened from the SEER database. After eliminating 8,804 patients based on the exclusion criteria, 1,253 RNET patients were included for further analysis (Figure 1). The baseline demographic, clinical and pathological features were summarized in Table 1. Of these patients, 643 (51.32%) were male, and 610 (48.68%) were female. The mean age at diagnosis was 53.79±10.59 years. Ninety-four patients received radical resection, and 1,159 underwent local excision. The median tumour size was 5 [3–8] mm. The tumour grade was I in 1,086 (86.67%) patients, II in 155 (12.37%), III in 9 (0.73%) and IV in 3 (0.23%) patients. The T stage was T1 in 1,169 (93.30%) patients, T2 in 65 (5.19%) patients, T3 in 16 (1.28%) patients and T4 in 3 (0.23%) patients. Twenty-three patients experienced lymph node metastasis, and 14 patients experienced distant organ metastasis. AJCC 7th TNM stage was I in 1,161 (92.66%) patients, II in 64 (5.11%) patients, III in 14 (1.12%) patients and IV in 14 (1.11%) patients.
Table 1
Items | Patients, n (%) |
---|---|
Age (years) | |
≤60 | 924 (73.74) |
>60 | 329 (26.26) |
Sex | |
Female | 610 (48.68) |
Male | 643 (51.32) |
Race | |
White | 687 (54.83) |
Black | 292 (23.30) |
Other | 274 (21.87) |
Year of diagnosis | |
2010–2012 | 412 (32.88) |
2013–2015 | 841 (67.12) |
Population | |
Rural/urban | 94 (7.50) |
Metropolitan | 1,159 (92.50) |
Median income ($) | |
<49,999 | 165 (13.17) |
50,000–64,999 | 459 (36.63) |
≥65,000 | 629 (50.20) |
Treatment type | |
Local excision | 1,159 (92.50) |
Radical resection | 94 (7.50) |
Grade | |
Grade I | 1,086 (86.67) |
Grade II | 155 (12.37) |
Grade III | 9 (0.73) |
Grade IV | 3 (0.23) |
Tumour size (mm), median [IQR] | 5 [3–8] |
T stage | |
T1 | 1,169 (93.30) |
T2 | 65 (5.19) |
T3 | 16 (1.28) |
T4 | 3 (0.23) |
N stage | |
N0 | 1,230 (98.16) |
N1 | 23 (1.83) |
M stage | |
M0 | 1,239 (98.88) |
M1 | 14 (1.12) |
AJCC TNM stage | |
I | 1,161 (92.66) |
II | 64 (5.11) |
III | 14 (1.12) |
IV | 14 (1.11) |
RNETs, rectal neuroendocrine tumours; IQR, interquartile range.
Predictors and construction of a nomogram for the prediction of lymph node metastasis
In the univariate analysis, other race (P=0.004), grade III/grade IV (P<0.001), tumour size ≥12 mm (P<0.001), T3/T4 stage and M1 stage (P<0.001) were significantly associated with the presence of lymph node metastasis. All the abovementioned predictors were included in the multivariate analysis, and tumour size ≥12 mm [odds ratio (OR) =40.401; 95% CI: 8.477–192.543; P<0.001], T3/T4 stage (OR =12.596; 95% CI: 3.505–45.266; P<0.001) and M1 stage (OR =9.185; 95% CI: 2.195–38.433; P=0.001) were independently associated with lymph node metastasis (Table 2). A nomogram with the three independent predictors from the multivariate analysis was constructed (Figure 2). These factors were assigned specific scores as follows: tumour size ≥12 mm, 100; T3/T4 stage, 68; and M stage, 59. The total risk scores of each patient based on the nomogram were calculated, and the total points ranged from 0 to 227. The optimal cut-off value was 84 with a specificity of 0.908 and a sensitivity of 0.913. The performance of the model was acceptable for predicting lymph node metastasis, with an AUC of 0.937 (95% CI: 0.874–1.000) (Figure 3A). Calibration curves and the Hosmer-Lemeshow test revealed desirable model calibration (P=0.99996) (Figure 3B).
Table 2
Items | Univariate analysis | Multivariate analysis | |||
---|---|---|---|---|---|
P | OR (95% CI) | P | OR (95% CI) | ||
Age (years) | |||||
≤60 | Reference | ||||
>60 | 0.620 | 0.777 (0.286–2.109) | |||
Sex | |||||
Female | Reference | ||||
Male | 0.358 | 1.486 (0.639–3.459) | |||
Race | |||||
White | Reference | ||||
Black | 0.372 | 1.692 (0.533–5.377) | |||
Other | 0.004 | 4.063 (1.558–10.593) | |||
Year of diagnosis | |||||
2010–2012 | Reference | ||||
2013–2015 | 0.521 | 0.758 (0.325–1.766) | |||
Population | |||||
Rural/urban | Reference | ||||
Metropolitan | 0.080 | 0.375 (0.125–1.126) | |||
Median income ($) | |||||
<49,999 | Reference | ||||
50,000–64,999 | 0.797 | 0.836 (0.214–3.273) | |||
≥65,000 | 0.840 | 1.140 (0.321–4.047) | |||
Grade | |||||
Grade I | Reference | ||||
Grade II | 0.386 | 0.408 (0.054–3.090) | |||
Grade III and grade IV | <0.001 | 44.916 (12.951–155.778) | |||
Tumour size (mm) | |||||
<12 | Reference | Reference | |||
≥12 | <0.001 | 103.792 (24.026–448.378) | <0.001 | 40.401 (8.477–192.543) | |
T stage | |||||
T1 and T2 | Reference | Reference | |||
T3 and T4 | <0.001 | 140.021 (47.855–409.696) | <0.001 | 12.596 (3.505–45.266) | |
M stage | |||||
M0 | Reference | ||||
M1 | <0.001 | 157.500 (46.801–530.040) | 0.002 | 9.185 (2.195–38.433) |
RNETs, rectal neuroendocrine tumours; OR, odds ratio; CI, confidence interval.
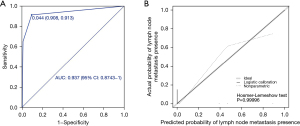
Prognostic factors for CSS and construction of a competing risk nomogram for CSS prediction
The univariable competing risk model analysis showed that radical resection (P<0.001), grade III/grade IV (P<0.001), tumour size ≥12 mm (P<0.001), T2 stage (P<0.001), T3/T4 stage (P<0.001), N1 stage (P<0.001), M1 stage (P<0.001), AJCC TNM stage II (P<0.001), III (P<0.001) and IV (P<0.001) were related to worse CSS outcomes. AJCC TNM stage was constructed based on the T stage, N stage and M stage. To avoid collinearity, AJCC TNM stage was included in the multivariate analysis rather than T stage, N stage and M stage. The multivariate competing risk model analysis showed grade II (SHR =9.981; 95% CI: 1.5124–65.859; P=0.017), tumour size ≥12 mm (SHR =6.034; 95% CI: 1.608–22.642; P=0.007), AJCC TNM stage II (SHR =15.656; 95% CI: 2.6035–94.143; P=0.002), stage III (SHR =55.508; 95% CI: 8.169–377.147; P<0.001) and stage IV (SHR =167.641; 95% CI: 8.619–3,260.394; P<0.001) were significantly associated with worse CSS outcomes (Table 3). The competing risk nomogram model was constructed based on independent prognostic factors (Figure 4). The nomogram showed that the AJCC TNM stage was the most impacted factor relating to prognosis, followed by tumour size and then grade. The estimated probability of survival can be determined at each time point on the total point scale, according to the total points. The time-dependent AUC revealed the good discriminatory ability of the model (survival time from 1 to 107 months, AUC >0.900) (Figure 5A), and the Brier score showed good accuracy of the nomogram (Figure 5B), which was greater than that of the AJCC TNM stage.
Table 3
Items | Univariate analysis | Multivariate analysis | |||
---|---|---|---|---|---|
P | SHR (95% CI) | P | SHR (95% CI) | ||
Age (years) | |||||
≤60 | Reference | ||||
>60 | 0.587 | 1.394 (0.421–4.613) | |||
Sex | |||||
Female | Reference | ||||
Male | 0.112 | 2.888 (0.781–10.690) | |||
Race | |||||
White | Reference | ||||
Black | 0.986 | 0.988 (0.256–3.809) | |||
Other | 0.676 | 0.715 (0.149–3.443) | |||
Year of diagnosis | |||||
2010–2012 | Reference | ||||
2013–2015 | 0.783 | 0.843 (0.249–2.856) | |||
Population | |||||
Rural/urban | Reference | ||||
Metropolitan | 0.246 | 0.406 (0.089–1.860) | |||
Median income ($) | |||||
<49,999 | Reference | ||||
50,000–64,999 | 0.566 | 0.665 (0.165–2.681) | 0.594 | 0.608 (0.098–3.783) | |
≥65,000 | 0.083 | 0.250 (0.052–1.196) | 0.139 | 0.195 (0.022–1.698) | |
Treatment type | |||||
Local excision | Reference | ||||
Radical resection | <0.001 | 11.760 (3.694–37.460) | 0.891 | 1.125 (0.209–6.085) | |
Grade | |||||
Grade I | Reference | ||||
Grade II | 0.058 | 4.059 (0.952–17.310) | 0.017 | 9.981 (1.5124–65.859) | |
Grade III and grade IV | <0.001 | 86.172 (22.696–327.190) | 0.527 | 2.181 (0.194–24.507) | |
Tumour size (mm) | |||||
<12 | Reference | ||||
≥12 | <0.001 | 91.37 (11.87–703.2) | 0.007 | 6.034 (1.608–22.642) | |
T stage | |||||
T1 | Reference | ||||
T2 | <0.001 | 26.87 (4.409–163.7) | |||
T3 and T4 | <0.001 | 281.60 (60.224–1316.7) | |||
N stage | |||||
N0 | Reference | ||||
N1 | <0.001 | 52.78 (17.32–160.8) | |||
M stage | |||||
M0 | Reference | ||||
M1 | <0.001 | 88.13 (31.12–249.6) | |||
AJCC TNM stage | |||||
I | Reference | ||||
II | <0.001 | 57.18 (5.984–546.5) | 0.002 | 15.656 (2.6035– 94.143) | |
III | <0.001 | 155.83 (13.271–1829.9) | <0.001 | 55.508 (8.169– 377.147) | |
IV | <0.001 | 499.99 (63.117–3960.7) | <0.001 | 167.641 (8.619–3,260.394) |
CSS, cancer-specific survival; RNETs, rectal neuroendocrine tumours; SHR, subdistribution hazard ratio; CI, confidence interval.
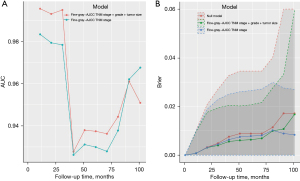
Prognostic factors for OS and construction of a nomogram for OS prediction
In the univariate analysis, age >60 years (P=0.004), median income ≥$65,000 (P=0.015), radical resection (P=0.005), grade III/grade IV (P<0.001), tumour size ≥12 mm (P<0.001), T3/T4 stage (P<0.001), N1 stage (P<0.001), M1 stage (P<0.001), AJCC TNM stage III (P=0.039) and AJCC TNM stage IV (P<0.001) were significantly associated with worse OS. All the abovementioned predictors were included in the multivariate analysis, and age >60 years (HR =2.494; 95% CI: 1.389–4.478; P=0.002), median income ≥$65,000 (HR =0.359; 95% CI: 0.161–0.804; P=0.013), AJCC TNM stage III (HR =4.559; 95% CI: 1.090–19.062; P=0.038) and AJCC TNM stage IV (HR =18.287; 95% CI: 7.564–44.211; P<0.001) were independently associated with worse OS (Table 4). A prognostic nomogram for OS outcomes with point scales for the above independent prognostic factors was constructed (Figure 6). These factors were assigned specific scores as follows: age >60 years, 31; AJCC TNM stage II, 23; AJCC TNM stage III, 52; AJCC TNM stage IV, 100; median income <$49,999, 35; median income $50,000–64,999, 24. The total risk score calculated using the nomogram for each patient ranged from 0 to 166. The nomogram showed that the AJCC TNM stage was the most impacted prognostic factor, followed by income and then age. The C-statistic for OS rate prediction was 0.703 (95% CI: 0.615–0.792), which was significantly better than that of the AJCC TNM stage (0.703 vs. 0.607, P=0.009). A calibration plot for the probability of survival at 1, 3 and 5 years (Figure 7A-7C) demonstrated good calibration.
Table 4
Items | Univariate analysis | Multivariate analysis | |||
---|---|---|---|---|---|
P | HR (95% CI) | P | HR (95% CI) | ||
Age (years) | |||||
≤60 | Reference | ||||
>60 | 0.004 | 2.355 (1.319–4.208) | 0.002 | 2.494 (1.389–4.478) | |
Sex | |||||
Female | Reference | ||||
Male | 0.104 | 1.644 (0.903–2.992) | |||
Race | |||||
White | Reference | ||||
Black | 0.311 | 1.392 (0.734–2.640) | |||
Other | 0.266 | 0.603 (0.247–1.470) | |||
Year of diagnosis | |||||
2010–2012 | Reference | ||||
2013–2015 | 0.517 | 1.248 (0.638–2.439) | |||
Population | |||||
Rural/urban | Reference | ||||
Metropolitan | 0.147 | 0.530 (0.225–1.250) | |||
Median income ($) | |||||
<49,999 | Reference | ||||
50,000–64,999 | 0.326 | 0.685 (0.322–1.457) | 0.418 | 0.731 (0.342–1.560) | |
≥65,000 | 0.015 | 0.369 (0.166–0.822) | 0.013 | 0.359 (0.161–0.804) | |
Treatment type | |||||
Local excision | Reference | ||||
Radical resection | 0.005 | 2.855 (1.377–5.920) | |||
Grade | |||||
Grade I | Reference | ||||
Grade II | 0.507 | 1.317 (0.584–2.966) | |||
Grade III and grade IV | <0.001 | 12.144 (4.312–34.202) | |||
Tumour size (mm) | |||||
<12 | Reference | ||||
≥12 | <0.001 | 3.259 (1.714–6.197) | |||
T stage | |||||
T1 | Reference | ||||
T2 | 0.174 | 2.049 (0.728–5.767) | |||
T3 and T4 | <0.001 | 15.900 (7.039–35.919) | |||
N stage | |||||
N0 | Reference | ||||
N1 | <0.001 | 7.976 (3.375–18.853) | |||
M stage | |||||
M0 | Reference | ||||
M1 | <0.001 | 13.376 (5.661–31.606) | |||
AJCC TNM stage | |||||
I | Reference | ||||
II | 0.127 | 2.240 (0.795–6.314) | 0.206 | 1.956 (0.691–5.533) | |
III | 0.039 | 4.516 (1.081–18.866) | 0.038 | 4.559 (1.090–19.062) | |
IV | <0.001 | 14.779 (6.194–35.262) | <0.001 | 18.287 (7.564–44.211) |
OS, overall survival; RNETs, rectal neuroendocrine tumours; HR, hazard ratio; CI, confidence interval.
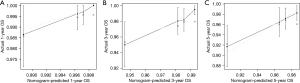
Discussion
This is the first study to construct nomograms for the prediction of lymph node metastasis, CSS and OS outcomes in RNET patients who underwent resection based on a large sample size, which is helpful for treatment decision-making and surveillance for RNET patients.
The prediction of lymph node metastasis is helpful for choosing appropriate treatments for RNET patients, especially for RNETs with a tumour size <20 mm. The assessment of lymph node metastasis is critical for surgeons to determine whether to perform radical resection or local excision. For RNET patients with lymph node metastasis, there is no doubt that radical resection is a better treatment choice, and the survival outcomes could be elevated with radical resection. Local excision cannot achieve the same beneficial effect as radical treatment. For RNETs without lymph node metastasis and other high-risk factors (tumour size ≥20 mm, inherent muscle infiltration, distant organ metastases, etc.), radical resection does not appear to be a good choice. Local excision provides a comparable survival benefit to radical resection, and radical resection can lead to poor functional outcomes—even if patients receive sphincter-sparing surgery for rectal cancer, up to 90% have altered bowel habits (11). Additionally, a substantial number of patients have symptoms of abnormal defecation, such as urgent stools, frequent stools, and incontinence, which severely affects their quality of life (12,13). Therefore, the accurate prediction of lymph node metastasis before resection will provide guidance to clinicians and allow them to develop a treatment strategy that provides the best survival benefit and quality of life. This study not only revealed that tumour size ≥12 mm, T3/T4 stage and M1 stage were independently associated with the presence of lymph node metastasis but also was the first to established a nomogram with a good AUC (0.937) for the prediction of lymph node metastases. This model can help clinicians determine the best surgical strategies for RNET patients.
In a survival analysis of RNET patients, Feng et al. (6) found that TNM stage was an independent prognostic factor, Yu et al. (7) revealed that tumour grade was associated with survival outcomes, and Chagpar et al. (5) found that lymph node metastasis, distant metastasis, the depth of tumour invasion and tumour size were independent prognostic factors. All of the above elements should be taken into consideration by clinicians when evaluating RNET patient survival outcomes. Nomograms are simple graphical linear prediction models that can take these variables into account and are widely used for the prediction of cancer patient prognosis. In this study, after comprehensive consideration of these influencing factors, we established a nomogram for the prediction of survival outcomes, including TNM stage (T: depth of tumour invasion; N: lymph node metastasis; M: distant metastasis), grade (differentiation, Ki-67 and mitotic rate per 10 high-power fields) and tumour size.
The nomograms constructed in this study consist of a competing risk nomogram for the CSS outcome and a nomogram for the OS outcome. The survival outcome of cancer patients may be affected by more than two events; however, only one event typically occurs (14). Competing risk events are events other than those of interest, which are censored in traditional survival analyses and may lead to overestimation of cumulative morbidity. Therefore, these events need to be modified by competitive risk analysis. We first established a competing risk model to reduce the influence of these outcomes. This competing risk nomogram model included grade, tumour size and AJCC TNM stage, which showed not only a good time-dependent AUC value and Brier score but also greater accuracy than that of the AJCC TNM stage classification. The possible effects arising from other lethal factors were eliminated by this simple nomogram, thus providing a reference for clinicians in practice. In addition to the competing risk nomogram model, this study also constructed a nomogram for OS outcomes. The OS outcome events included tumour-related death and nontumour-related death (such as cardiovascular disease, cerebrovascular disease and car accidents). Therefore, clinicians should consider not only the effect of tumour biological behaviour but also the effect of physical functions and economic statuses when considering the factors affecting survival. The variables in our nomogram comprehensively considered the effect of the above factors, including age (physical condition), median income (economic factor) and TNM stage (tumour biological behaviour). Fields et al. (15) reported that old age was an unfavourable factor for OS outcomes in RNET patients based on a study of the NCDB database, which was consistent with our results. Older age is usually accompanied by poor physical function, decreased immunization and increased cardiovascular disease. The relationship between age and OS was expected to some extent. High-income patients tend to enjoy high-quality medical treatment and living conditions. The association between income and OS can be explained by these factors. The direct application of our prognostic nomograms to calculate risk scores may be a practical method to obtain personalized survival prediction for patients with RNETs.
This study has several limitations. First, we only used the SEER database to establish the prognostic models and lacked internal validation and external validation. Because only a small number of patients experienced cancer-specific death (12/1,253, 0.96%), lymph node metastases (23/1,253, 1.83%) and distant organ metastasis (14/1,253, 1.12%), dividing this sample set into a development model and a validation model would have introduced bias. Through accumulating more patients, we will verify our findings and further improve the RNET prognostic model by using a validation database in future. Second, it has been widely reported that the latest WHO classification [G1, G2, G3 and neuroendocrine carcinoma (NEC)] for NET was an important prognostic indicator. However, owing to the limitation of the SEER database, we were unable to obtain classification for the patients enrolled in this study. With advances in molecular and genetic detection techniques, genes and biomarkers have become promising factors to predict survival outcomes. However, biomarker data are not currently available in the SEER database, and more research is needed to explore the effects of these biomarkers on patient prognoses.
In conclusion, the present study established nomograms using a SEER database cohort, and the nomograms displayed comparatively better discrimination and accuracy for the prediction of lymph node metastases, CSS and OS. These nomograms can be used to guide tailored treatment strategies and predict individualized survival outcomes for patients with RNETs, which is helpful for improving prognostic assessments, enhancing patient stratification in clinical trials, and performing prognosis-based decision-making for individual RNET patients.
Acknowledgments
Funding: This study was supported by the National Natural Science Foundation of China (81972311), the Non-Profit Central Research Institution Fund of Chinese Academy of Medical Sciences (2019PT310026) and Sanming Project of Medicine in Shenzhen (No. SZSM 202011010).
Footnote
Reporting Checklist: The authors have completed the TRIPOD reporting checklist. Available at https://jgo.amegroups.com/article/view/10.21037/jgo-21-573/rc
Conflicts of Interest: All authors have completed the ICMJE uniform disclosure form (available at https://jgo.amegroups.com/article/view/10.21037/jgo-21-573/coif). The authors report that the work received funding from the National Natural Science Foundation of China (81972311), the Non-Profit Central Research Institution Fund of Chinese Academy of Medical Sciences (2019PT310026) and Sanming Project of Medicine in Shenzhen (No. SZSM 202011010). The authors have no other conflicts of interest to declare.
Ethical Statement: The authors are accountable for all aspects of the work in ensuring that questions related to the accuracy or integrity of any part of the work are appropriately investigated and resolved. The study was conducted in accordance with the Declaration of Helsinki (as revised in 2013). This study was based on a public SEER database and no personal identifying information was used in the study. Therefore, informed consent and institutional ethical approval was not required.
Open Access Statement: This is an Open Access article distributed in accordance with the Creative Commons Attribution-NonCommercial-NoDerivs 4.0 International License (CC BY-NC-ND 4.0), which permits the non-commercial replication and distribution of the article with the strict proviso that no changes or edits are made and the original work is properly cited (including links to both the formal publication through the relevant DOI and the license). See: https://creativecommons.org/licenses/by-nc-nd/4.0/.
References
- Yao JC, Hassan M, Phan A, et al. One hundred years after "carcinoid": epidemiology of and prognostic factors for neuroendocrine tumors in 35,825 cases in the United States. J Clin Oncol 2008;26:3063-72. [Crossref] [PubMed]
- Dasari A, Shen C, Halperin D, et al. Trends in the Incidence, Prevalence, and Survival Outcomes in Patients With Neuroendocrine Tumors in the United States. JAMA Oncol 2017;3:1335-42. [Crossref] [PubMed]
- de Mestier L, Lorenzo D, Fine C, et al. Endoscopic, transanal, laparoscopic, and transabdominal management of rectal neuroendocrine tumors. Best Pract Res Clin Endocrinol Metab 2019;33:101293. [Crossref] [PubMed]
- Zandee WT, de Herder WW. The Evolution of Neuroendocrine Tumor Treatment Reflected by ENETS Guidelines. Neuroendocrinology 2018;106:357-65. [Crossref] [PubMed]
- Chagpar R, Chiang YJ, Xing Y, et al. Neuroendocrine tumors of the colon and rectum: prognostic relevance and comparative performance of current staging systems. Ann Surg Oncol 2013;20:1170-8. [Crossref] [PubMed]
- Feng X, Wei G, Wang W, et al. Nomogram for individually predicting overall survival in rectal neuroendocrine tumours. BMC Cancer 2020;20:865. [Crossref] [PubMed]
- Yu YJ, Li YW, Shi Y, et al. Clinical and pathological characteristics and prognosis of 132 cases of rectal neuroendocrine tumors. World J Gastrointest Oncol 2020;12:893-902. [Crossref] [PubMed]
- Shah MH, Goldner WS, Halfdanarson TR, et al. NCCN Guidelines Insights: Neuroendocrine and Adrenal Tumors, Version 2.2018. J Natl Compr Canc Netw 2018;16:693-702. [Crossref] [PubMed]
- Sohn B, Kwon Y, Ryoo SB, et al. Predictive Factors for Lymph Node Metastasis and Prognostic Factors for Survival in Rectal Neuroendocrine Tumors. J Gastrointest Surg 2017;21:2066-74. [Crossref] [PubMed]
- NCI. Surveillance, Epidemiology, and End Results (SEER). Available online: https://seer.cancer.gov/
- Keane C, Fearnhead NS, Bordeianou LG, et al. International Consensus Definition of Low Anterior Resection Syndrome. Dis Colon Rectum 2020;63:274-84. [Crossref] [PubMed]
- Liang Z, Ding W, Chen W, et al. Therapeutic Evaluation of Biofeedback Therapy in the Treatment of Anterior Resection Syndrome After Sphincter-Saving Surgery for Rectal Cancer. Clin Colorectal Cancer 2016;15:e101-7. [Crossref] [PubMed]
- Battersby NJ, Bouliotis G, Emmertsen KJ, et al. Development and external validation of a nomogram and online tool to predict bowel dysfunction following restorative rectal cancer resection: the POLARS score. Gut 2018;67:688-96. [PubMed]
- Austin PC, Fine JP. Practical recommendations for reporting Fine-Gray model analyses for competing risk data. Stat Med 2017;36:4391-400. [Crossref] [PubMed]
- Fields AC, Saadat LV, Scully RE, et al. Local Excision Versus Radical Resection for 1- to 2-cm Neuroendocrine Tumors of the Rectum: A National Cancer Database Analysis. Dis Colon Rectum 2019;62:417-21. [Crossref] [PubMed]