Identification and validation of xenobiotic metabolism-associated prognostic signature based on five genes to evaluate immune microenvironment in colon cancer
Introduction
Cancer, including colorectal cancer (CRC), is a metabolic disease. In 2018, CRC was the third most common cancer in the world (1). To delay the progression of the disease and reduce its incidence and mortality, effective prevention and diagnosis and appropriate treatment at an early stage are essential. However, the methods of early diagnosis and treatment monitoring are not perfect. Precise biomarkers need to be identified urgently to improve diagnosis and treatment. Compared to esophageal cancer, gastric cancer, and hepatocellular carcinoma, the burden of CRC is higher in Europe and North America than the rest of the world (2). In addition to geographical and ethnic factors, lifestyle factors, especially eating habits, affect the occurrence and development of CRC; for example, a Mediterranean diet is negatively related to the incidence of CRC, while a Westernized diet may increase the risk of cancer (3,4). The intestinal tract is an important channel by which substances are absorbed in the human body. The colonic epithelium is exposed to a variety of compounds in the diet, including endogenous compounds and xenobiotics (5). There are many kinds of xenobiotics, for example, drugs, chemicals, pesticides, environmental pollutants, and metabolites of other species. The metabolism of xenobiotics in the human body can block or accelerate the progression of CRC to varying degrees (6,7). Thus, the role of xenobiotic metabolism in the development of CRC cannot be ignored. Further, xenobiotic metabolism–related biomarkers may prove effective in the prevention and treatment of CRC.
Xenobiotic metabolism enzymes (XMEs) are important bioactive molecules in the metabolism of xenobiotics . It has been speculated that XMEs are key regulatory factors in the occurrence of CRC (8). At present, XMEs can be divided into 3 categories: (I) cytochrome (CY) P450 (9); (II) glutathione S-transferase (GST) (10); and (III) uridine diphosphate glucuronosyltransferase (UGT) (11). Studies have shown that CYP450 activates proto-oncogenes through oxidation, then transforms them into reactive metabolites, which react irreversibly with macromolecules (12). This process leads to mutation and potential carcinogenesis; however, GST and UGT may inactivate or detoxify these active substances (10-12). Changes in metabolic binding pathways of xenobiotics have been shown to significantly affect drug-induced toxicity and cancer susceptibility. For example, taxane induces its own degradation by inducing CYP450 (13), while UGT1A weakens the cytotoxicity of anticancer drugs by reducing their intracellular exposure, and resulting in metabolic elimination in colon cancer cell lines (14). Thus, the expression of many XMEs in the tissues of normal colon and colon tumor differs, and their correlation with clinicopathological factors is of great significance to understanding the pathogenesis of CRC.
Most exogenous substances are eliminated by a variety of enzymes and pathways. In addition to XMEs, many members of transporter families also play important roles in xenobiotic metabolism. Common transporters include solute carrier transporters (e.g., organic anion transporting polypeptides, organic cation transporters, organic cation/carnitine transporters, organic anion transporters, peptide transporters, concentrative nucleoside transporters, equilibrative nucleoside transporters, and multidrug and toxin extrusion transporters), adenosine triphosphate (ATP)-binding cassette transporters related to drug resistance (e.g., multidrug resistance proteins, multidrug resistance-associated proteins, and breast cancer resistance protein), and bile acid, cholesterol, aminophospholipid, and copper transporters (e.g., sodium taurocholate co-transporting polypeptide, apical sodium-dependent bile acid transporter, bile salt export pump, organic solute transporters, ATP-binding cassette transporter A1, ATP-binding cassette subfamily G members 5 and 8, ATPase copper-transporting β polypeptide, and ATPase class I type 8B member 1) (15). It is necessary to explore the biomarkers of CRC using these substances.
The existence of immune microenvironment suggests that the role of immune system in cancer process can not be ignored. The occurrence of cancer can be considered as the accumulation of a large number of genetic changes. Abnormal expression of genes is easy to induce the production of new antigens, so as to stimulate the immune system and trigger the immune response. Cancer-immunity cycle (CIC) reveals the process that immune cells, as members of tumor immune microenvironment, can identify and kill cancer cells according to a series of steps or procedures, which provides the target for cancer immunotherapy (16). Abnormally expressed genes may be involved in various physiological functions, including xenobiotic metabolism. Therefore, exploring the correlation between xenobiotic metabolism and CIC regulatory factors is helpful to provide new strategies for cancer immunotherapy.
In addition to hereditary factors, environmental exposure plays an important role in the development of the immune system. Xenobiotics in the environment can directly or indirectly activate the aryl hydrocarbon receptor (AhR), which results in the transcription of additional enzymes involved in xenobiotic metabolism, and affects the process of inflammation and cancer through the cytosolic AhR transcriptional pathway (17). It has been suggested that AhR induces epigenetic changes of immune cells and regulates the intestinal immune reaction by the AhR signaling pathway (18). Further, xenobiotic metabolizing enzymes negatively regulate the intestinal AhR pathway based on the consumption of xenobiotics (19). Consequently, xenobiotic metabolism may also be associated with the immune response in CRC. It can be inferred that in the tumor microenvironment, if the expression level of genes or proteins related to xenobiotic metabolism changes, the degree of tumor immune infiltration may also change.
Some XME gene biomarkers in CRC have been found, but other biomarkers related to xenobiotic metabolism that may be useful in the diagnosis and prognosis of CRC are still not very clear. In addition, the correlation between xenobiotic metabolism and immune microenvironment also needs to be explored in depth. As the prognosis of colon cancer may differ to that of rectal cancer, this study was limited to colon cancer. We analyzed differentially expressed xenobiotic metabolism genes (XMGs) in colon tumor tissues and normal colon tissues in The Cancer Genome Atlas (TCGA) database. A prognostic model was then constructed and validated by training cohort and validation cohort, respectively. Additionally, the tumor immune infiltration landscape was explored, and negative regulatory immune checkpoints and immune cytokines of CIC were also analyzed in low- and high-risk groups, in order to explore the mechanism of xenobiotic metabolism affecting the progression of colon cancer from the perspective of immunity. We present the following article in accordance with the TRIPOD reporting checklist (available at https://dx.doi.org/10.21037/jgo-21-655).
Methods
The study was conducted in accordance with the Declaration of Helsinki (as revised in 2013). As this was a retrospective database study that used a public database, approval from the ethics committee was not required.
Online data acquisition
The original transcriptome data and clinical features of a colon cancer data set were downloaded from TCGA database (https://tcga-data.nci.nih.gov/tcga/) on 15 March 2021. The data comprised 452 colon cancer patients, 41 normal colon tissue samples, and 473 colon tumor tissue samples. In addition, data on 200 genes encoding proteins involved in the processing of drugs and other xenobiotics were obtained from the hallmark xenobiotic metabolism set of the Molecular Signatures Database (MSigDB) (http://www.gsea-msigdb.org/gsea/msigdb/index.jsp), and eight xenobiotic metabolism genes (see Table S1), which may be differently expressed between CRC patients and healthy people, were extracted from the literature as supplements (20).
Identification of differentially expressed XMGs and the functional enrichment analysis
The differentially expressed XMGs between the normal samples and colon cancer samples were identified using the “limma” and “pheatmap” packages in R software for which the screening criteria was |log2 fold change (FC)| >0.5 and a false discovery rate (FDR) <0.05. Gene Ontology (GO) and Kyoto Encyclopedia of Genes and Genomes (KEGG) enrichment analyses were performed by the “clusterprofler” package in R software to identify potential molecular mechanisms of the differentially expressed XMGs. The packages of both “ggplot2” and “enrichplot” were applied to visualize the GO and KEGG enrichment analysis results.
Establishment of the XMG prognostic model
Packages of “survival”, “caret”, “glmnet”, “survminer”, and “survivalROC” in R software were used to establish prognostic model. A univariate Cox regression analysis was performed to analyze the XMGs associated with prognosis in colon cancer patients. Subsequently, an XMG prognostic model was constructed by multivariate Cox regression analysis and potential prognostic biomarkers of colon cancer were identified. Further, the risk score of each colon cancer patient was calculated using the following equation: risk score =expression of XMG1 × β1 + expression of XMG2 × β2 +…+ expression of XMGn × βn, where β was the coefficient calculated by a multivariate Cox regression model (21). Finally, colon cancer patients were divided into low- and high-risk groups on the basis of risk score in both the training and validation cohorts.
Evaluation of the XMG prognostic model
For both the training cohort and validation cohort, Kaplan-Meier survival curves were constructed using the “survival” package in R to estimate differences in overall survival (OS) between the two risk groups. The risk plots were constructed through “pheatmap” in R software. Receiver operating characteristic (ROC) curves were constructed to evaluate the accuracy of the prognostic XMG model by the “survivalROC” package in R. The independent prognostic analyses, including the univariate and multivariate Cox regression analyses, were also performed using the “survival” package in R to determine whether the prognostic XMG signature was an independent predictor of OS in colon cancer patients. The covariates were age, gender, stage, tumor (T), node (N), metastasis (M), and risk score.
Tumor immune cell infiltration analysis
The CIBERSORT algorithm (https://cibersort.stanford.edu/) was used to calculate the relative abundances of infiltrated immune cells in the colon cancer tissue samples of the training cohort and validation cohort, and the barplots and boxplots were performed to describe the situation of immune infiltration.
Analysis of genes negatively regulating the CIC
Gene signatures related to CIC were acquired from website of Tracking Tumor Immunophenotype (http://biocc.hrbmu.edu.cn/TIP/index.jsp). The negative regulation genes of CIC were identified, including immune checkpoints and other immune regulation genes. The difference of gene expression between high- and low-risk groups and the correlation between gene expression and risk scores were analyzed in the training cohort and validation cohort.
Statistical analysis
The software R 4.0.2 (https://www.r-project.org/) was used in all statistical analyses, including in the plots, unifactor and multifactor regression analyses, independent prognosis analysis, and correlation analysis. The R package can be downloaded from the Bioconductor platform (https://www.bioconductor.org/). The survival states were accessed using log-rank tests. Wilcoxon tests were conducted for immune cell infiltration analysis. A P value <0.05 was considered statistically significant.
Results
Workflow and clinical features
The study flowchart is shown in Figure 1. In brief, the transcriptome data were downloaded from TCGA database, and XMGs were screened and functionally analyzed. Next, the clinical data of colon cancer patients were also obtained from TCGA. A prognostic model was then constructed based on the XMGs and clinical data, and the prognostic value and accuracy of the prediction model were evaluated in the training cohort and validation cohort. Additionally, the tumor immune cell infiltration and negative regulation genes of the CIC in high- and low-risk groups were analyzed in the two cohorts. In relation to the clinical characteristics of the colon cancer patients, TCGA data set comprised 235 males and 212 females, aged 31–90 years (patients were excluded if they had incomplete data).
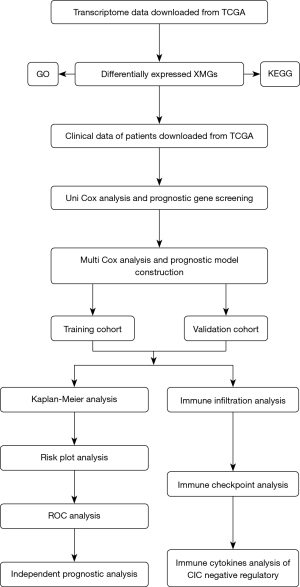
Identification of differentially expressed XMGs
The ribonucleic acid sequencing (RNA-seq) data of the colon cancer data set in TCGA database were standardized using R software. A total of 126 differentially expressed XMGs, including 77 upregulated genes and 49 downregulated genes, were identified in the 41 normal colon tissue samples and 473 colon tumor tissue samples using the following screening criteria; |log2 fold change (FC)| >0.5 and FDR<0.05 (see the volcano and heat maps in Figure 2A,2B).
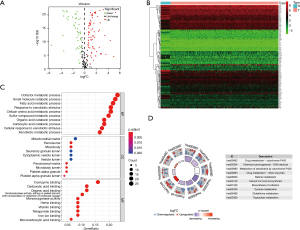
Functional annotations of differentially expressed XMGs
To further explore the biological function of differentially expressed XMGs in colon cancer patients, GO enrichment and KEGG pathway analyses were performed with R software. The results of the GO analysis showed that the XMGs were significantly enriched in the biological processes (BPs) involved in the metabolism of small molecular substances. The BPs included the cofactor metabolic process, small molecule catabolic process, fatty acid metabolic process, response to xenobiotic stimulus, cellular amino acid metabolic process, and so on. The significant cellular components (CCs) for the GO items contained mitochondrial matrix, peroxisome, microbody, secretory granule lumen, cytoplasmic vesicle lumen, and so on. Molecular function (MF) enrichment mainly focused on the binding of small molecular substances, such as coenzyme binding, carboxylic acid binding, organic acid binding, heme binding, monocarboxylic acid binding, and so on (see Figure 2C). The results of the KEGG pathway analysis showed that the differentially expressed XMGs were mainly enriched in signaling pathways related to the metabolism of nutrients and drugs, for example, drug metabolism-CYP450, chemical carcinogenesis-DNA adducts, metabolism of xenobiotics by cytochrome P450, drug metabolism-other enzymes, and retinol metabolism (see Figure 2D).
Construction and evaluation of the XMG prognostic model
The results of the univariate Cox regression analysis showed that 9 differentially expressed XMGs were significantly correlated with the OS of colon cancer patients (see Figure 3A). The detailed expression of the 9 genes in normal and tumor tissues is shown in Figure 3B. Following optimization by a multivariate Cox regression analysis, 5 XMGs (i.e., CYP2W1, GSTM1, TGFB2, MPP2, ACOX1; for the full names, see Table 1) were screened for inclusion in the prognostic prediction model. Among them, the hazard ratios (HRs) for CYP2W1, GSTM1, TGFB2, and MPP2 were >1, but for ACOX1, the HR was <1, which suggests that CYP2W1, GSTM1, TGFB2, and MPP2 are risk genes, while ACOX1 is a protective gene. However, the expression levels of CYP2W1 and TGFB2 were upregulated in colon tumor tissues than normal colon tissues, while those of GSTM1, MPP2, and ACOX1 were downregulated in TCGA data set (Figure 3B). These findings need to be validated in other data sets.
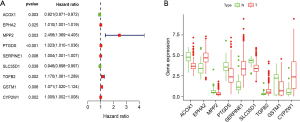
Table 1
Gene | Full name | Coefficient | HR | HR 95% CI (low) | HR 95% CI (high) |
---|---|---|---|---|---|
CYP2W1 | Cytochrome P450 2W1 | 0.0042 | 1.0042 | 0.9999 | 1.0084 |
GSTM1 | Glutathione S-transferase Mu 1 | 0.0913 | 1.0956 | 1.0276 | 1.1680 |
TGFB2 | Transforming growth factor beta-1 | 0.2130 | 1.2373 | 1.0675 | 1.4342 |
MPP2 | MAGUK p55 subfamily member 2 | 0.9292 | 2.5324 | 1.0626 | 6.0353 |
ACOX1 | Peroxisomal acyl-coenzyme A oxidase 1 | -0.1166 | 0.8899 | 0.8165 | 0.9700 |
XMG, xenobiotic metabolism gene; HR, hazard ratio; CI, confidence interval.
The Kaplan-Meier (KM) survival curve analysis indicated that low-risk patients had a better prognosis than high-risk patients in the training cohort (P<0.01; see Figure 4A) and validation cohort (P<0.01; see Figure 4B). As the heatmap shows, the expression levels of CYP2W1, GSTM1, TGFB2, and MPP2 were higher in high-risk group, and the expression of ACOX1 was higher in low-risk group in both the training and validation cohorts (see Figure 4C,4D) Distribution of risk scores indicated that patients in high-risk group had much higher risk scores than those in low-risk group in the training cohort (see Figure 4E), in accordance with that in the validation cohort (see Figure 4F). Distribution of survival status suggested that the high-risk colon cancer population had a relatively higher death toll compared to the low-risk population in the training cohort (see Figure 4G), as well as that in the validation cohort (see Figure 4H). Besides, it was also indicated that mortality was higher in the high-risk group compared to low-risk group in the training cohort (see Figure 4I) and the same is true for the validation cohort (see Figure 4J).
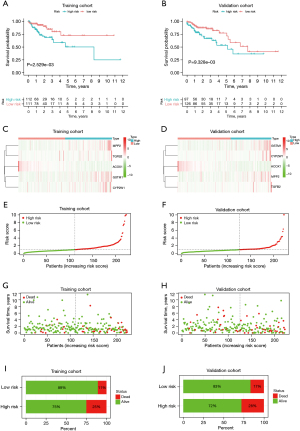
In the training cohort, the areas under the curve (AUCs) of the ROC implied that the model had good efficiency in the prediction of prognosis (AUC =0.724; see Figure 5A); the univariate Cox regression analysis showed that stage (P<0.001, HR =2.524, 95% CI: 1.708 to 3.730), T (P<0.001, HR =3.224, 95% CI: 1.705 to 6.096), M (P<0.001, HR =5.186, 95% CI: 2.602 to 10.338), N (P<0.001, HR =2.239, 95% CI: 1.501 to 3.338), and risk score (P<0.001, HR =1.161, 95% CI: 1.102 to 1.224) were univariate independent prognostic factors of colon cancer (see Figure 5B). The multivariate Cox regression analysis showed that only risk score (P=0.001, HR =1.104, 95% CI: 1.039 to 1.174) was the multivariate independent prognostic factor of colon cancer (see Figure 5C); thus, risk score is an independent prognostic indicator of colon cancer. In the validation cohort, the AUCs of the ROC confirmed the accuracy of this prognostic model (AUC =0.661; see Figure 5D); the univariate Cox regression analysis showed that age (P<0.01, HR =1.044, 95% CI: 1.013 to 1.076), stage (P<0.001, HR =2.064, 95% CI: 1.422 to 2.996), T (P<0.05, HR =2.366, 95% CI: 1.227 to 4.562), M (P<0.001, HR =4.007, 95% CI: 1.994 to 8.049), N (P<0.01, HR =1.820, 95% CI: 1.251 to 2.649), and risk score (P<0.01, HR =1.397, 95% CI: 1.128 to 1.729) were univariate independent prognostic factors of colon cancer (see Figure 5E). The multivariate Cox regression analysis showed that age (P<0.001, HR =1.062, 95% CI: 1.029 to 1.096), and risk score (P<0.001, HR =1.622, 95% CI: 1.291 to 2.040) were multivariate independent prognostic factors of colon cancer (see Figure 5F); thus, age and risk score are independent prognostic indicators of colon cancer. In summary, the risk score of the XMG model can effectively predict the prognosis of colon cancer.
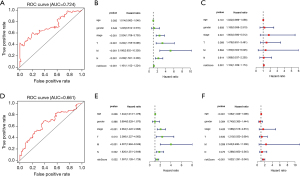
Immune infiltration landscape
Between the low- and high-risk groups of colon cancer patients divided by the risk score, the infiltration of 22 kinds of tumor immune cells was analyzed using the CIBERSORT method. The abundance distribution of each immune cell in each sample in the training cohort is shown in Figure 6A, and the statistical results implied that the abundance of T cells CD4 memory resting was higher in low-risk group compared to high-risk group and that of T cells regulatory (Tregs) was higher in high-risk group compared to low-risk group (see Figure 6B; both P<0.01). In the validation cohort, the abundance distribution of each immune cell in each sample is shown in Figure 6C, and the abundance of T cells CD4 memory resting and Tregs (see Figure 6D; both P<0.01) was similar to that in the training cohort.
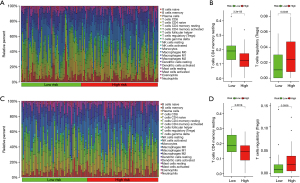
Negative regulation genes of the cancer-immunity cycle
Focusing on negative regulatory genes of CIC in the tracking tumor immunophenotype website, differentially expressed immune genes between low- and high-risk groups were exhibited as heatmaps. There were 16 different genes in the training cohort, including immune checkpoints programmed cell death protein 1 (PDCD1), lymphocyte activation gene 3 protein (LAG3), hepatitis A virus cellular receptor 2 [HAVCR2, also named T-cell immunoglobulin mucin receptor 3 (TIM-3)], and cluster of differentiation 160 (CD160) (see Figure 7A), and 13 different genes in the validation cohort, including immune checkpoints T-cell immunoreceptor with Ig and ITIM domains (TIGIT), PDCD1, and LAG3, respectively (see Figure 7B).
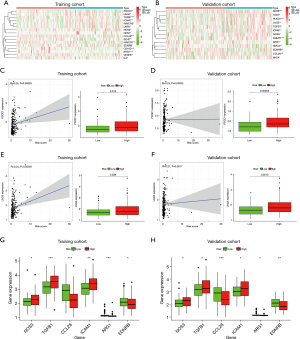
The common immune checkpoints in the training and validation cohorts which were differentially expressed between the low- and high-risk groups were PDCD1 and LAG3. The PDCD1 gene was positively correlated with the risk score, and upregulated in high-risk colon cancer populations compared to low-risk in both the training cohort (see Figure 7C; P<0.05) and validation cohort (see Figure 7D; P<0.001). The correlation with risk score and the expression trends in low- and high-risk groups of LAG3 were close to those of PDCD1 in the training cohort (see Figure 7E; P<0.05), as well as the validation cohort (see Figure 7F, P<0.01).
For the differentially expressed immunomodulatory cytokines between low- and high-risk groups, the expression of nitric oxide synthase 3 (NOS3), transforming growth factor beta-1 proprotein (TGFB1), and intercellular adhesion molecule 1 (ICAM1) was higher in the high-risk group while that of C-C motif chemokine 28 (CCL28), arginase-1 (ARG1), and endothelin receptor type B (EDNRB) was higher in low-risk group in the training cohort (see Figure 7G; all P<0.05), which was similar to the validation cohort (see Figure 7H; all P<0.05).
Discussion
In addition to metabolizing sugars, lipids, and proteins, xenobiotic metabolism is an important part of the BPs related to metabolism in the human body. With the exception of drugs, most exogenous substances are harmful to human body. Xenobiotics usually go through four processes in the human body: absorption, distribution, metabolism, and excretion. The metabolic process is particularly important. Xenobiotics affect the body by producing pharmacological or toxicological effects; however, they are also metabolized by the body. Most lose their pharmacological or toxicological activities through metabolic transformation, becoming highly water soluble substances. In addition, some drugs need to be metabolized to exert their pharmacological effects, and most toxic xenobiotics need to be metabolized to induce cancer. Thus, the metabolism process of xenobiotics is particularly important for human health and disease prognosis. If the human body is overexposed to xenobiotics, abnormal changes in biomolecules or the immune microenvironment related to the xenobiotic metabolism may result. Variations in the biomacromolecules and immune cells involved in the regulation of xenobiotic metabolism need to be monitored to identify biomarkers that can be used in the early diagnosis of diseases.
The occurrence of colon cancer is affected by many factors. In addition to hereditary colon cancer, sporadic colon cancer is closely related to environmental and lifestyle factors. Food is an important source of xenobiotics. Pesticides, environmental pollutants, and other xenobiotics can be indirectly absorbed by the human body through the enrichment of the food chain. The heterocyclic amines, polycyclic aromatic hydrocarbons, and N-nitroso compounds produced in food processing are well-known carcinogens (22). These carcinogens can be activated by XMEs, which increase the risk of CRC. Thus, the abnormal expression of metabolizing enzymes may indicate a risk of colon cancer. At present, studies have shown that XMEs are often dysfunctional in cancers and some XME biomarkers have been identified. In patients with CRC, the expression of CYP3A4, GSTM1, GSTA1, UGT1A8, and UGT1A10 in tumor tissues is significantly lower than that in normal mucosa tissues (7). However, the metabolism of xenobiotics is complex. Similar to XMEs, little is known about the other biological functional molecules or immune cells involved in the xenobiotic metabolic pathways; thus, further study is needed.
Differentially expressed XMGs have a variety of functional roles, and the proteins encoded by them include drug transporters (e.g., canalicular multispecific organic anion transporter 1), XMEs (e.g., CYP450), aquaporin (e.g., Aquaporin-9), dimethylaniline monooxygenase [e.g., eimethylaniline monooxygenase (N-oxide-forming) 3]. The GO and KEGG enrichment analyses suggested that these differentially expressed XMGs are mainly associated with the metabolism of drugs and nutrients. The value and accuracy assessment of the prognostic model in TCGA revealed that among the prognosis-related genes, a combination of 5 genes can effectively predict the outcome of colon cancer.
The CYP450 superfamily can be divided into 18 families and 33 subfamilies, encoding 57 kinds of CYP proteins, a third of which participate in xenobiotics metabolism (23). As a young member, CYP2W1 belongs to family 2 and subfamily W of CYP450 enzymes (24). It has been found to overexpress in CRC (25), and is supposed to be an effective target of drug therapy for CRC (26). Meanwhile, GSTM1, which is a member of the GST family and involved in carcinogen metabolism, may be related to risk and progression of CRC through gene polymorphisms, gene variation, and diet (27,28). The transforming growth factor-β (TGFB) family plays an important role in the process of tumorigenesis by regulating epithelial-mesenchymal transition. Among them, TGFB2 was reported to induce the formation of lipid droplets to promote metastasis of colon cancer cells (29). In addition, TGFB2 combining with HIF1 α contributed to chemoresistance in hypoxic tumor microenvironment in CRC (30). As a kind of tumor suppressor protein, members of the membrane-associated guanylate kinase homologs (MAGUKs) family regulate the proliferation, intracellular junctions, and signal transduction of tumor cells by interacting with the cytoskeleton. The decreased expression of a MAGUK family member, MPP2, is thought to be associated with the development of cancer. It has been reported that MPP2 gene was downregulated in relapse Wilms tumors (31), and may be a biomarker of pituitary tumors (32). The relationship between MPP2 and colon cancer needs to be explored. In vivo and in vitro experiments showed that ACOX1 was closely related to a variety of cancers (33). The activation of the fatty acid oxidation (FAO) pathway induces metastasis in colon cancer (34), and ACOX1 is the rate-limiting enzyme of the FAO pathway, hence, enhancing or restoring the expression of ACOX1 prevents metastasis in colon cancer cells (35). The present study suggests that the cell biology pathway and the fatty acid metabolism pathway are also involved in the process of xenobiotic metabolism, and the 5 genes can be used as diagnostic genes.
Notably, the risk scores related to the XMGs were associated with T cells CD4 memory resting and Tregs, which confirms that xenobiotic metabolism affects the immune microenvironment of colon cancer to a certain extent. The functions of T cells CD4 memory resting and Tregs on CRC have been revealed by bioinformation and experimental studies (36,37). Therefore, immune regulation may be one of the mechanisms of xenobiotic metabolism affecting CRC process.
Negative regulation genes of CIC, including immune checkpoints, were also involved in the immune regulation of xenobiotics metabolism. The concept of CIC was proposed in 2013, referring to the process of tumor cell releasing antigen, tumor antigen presentation, T cell activation, T cell migrating to tumor tissue, T cell infiltration in tumor tissue, T cell recognizing tumor cells, and clearing tumor cells (16,38). The CIC reveals the mechanism of immune system killing tumor cells. These 7 processes need the synergy of corresponding positive and negative regulatory factors to keep the activation of the immune system within the normal range. Negative regulation genes of CIC may affect the inhibition of cancer by the immune system. Programmed cell death 1 ligand 1 (PD-L1) [also named cluster of differentiation 274 (CD274)] and PDCD1 are the first checkpoints to be discovered. The functions of PD-L1 and PDCD1 in the human body are diverse, and protective immunity is restricted by PDCD1 expression in response to chronic pathogens and cancer (39). However, not all cancer patients are responsive to inhibitors of PD-L1 or PDCD1, so other checkpoints are subsequently discovered. The next wave of co-inhibitory receptor targets being explored in clinical trials includes LAG-3, TIM-3, and TIGIT: LAG3 is highly expressed on CD8+ and activated CD4+ T cells, and a subset of natural killer (NK) cells, promoting exhaustion of T cells; TIM-3 is selectively expressed on CD8+ T cytotoxic 1 (Tc1), interferon (IFN)-γ-inducing CD4+ T helper 1 (Th1), Tregs, dendritic cells (DCs), NK cells, and monocytes, indicating dysfunctional or exhausted CD8+ T cells in cancer; TIGIT can be found on activated T cells, NK cells, memory T cells, follicular T helper (Tfh) cells, and a subset of Tregs, directly exerting function on T and NK cells, and indirectly suppressing immune responses (40). Further, CD160 contributes to NK cell activation, T cell inhibition, and mucosal immunity (41). In addition to immune checkpoints, the role of immunosuppressive cytokines in tumor microenvironment should not be ignored. For example, NOS3 can modulate immune responses and inflammatory processes in vivo (42); TGFB1 has been demonstrated to suppress NK cell activity, inhibit DC maturation, alter T-cell cytotoxic properties, and so on (43); as an inflammation biomarker, ICAM1 is expressed in most cell types of tumor microenvironment and affects tumorigenesis and metastasis (44). We have shown that the abundance for checkpoints PDCD1, LAG3, and the expression for immune regulation genes NOS3, TGFB1, and ICAM1 upregulated in the high-risk group in both the training and validation cohorts, which may be the reasons for immune escape in CRC.
In this study, we established and validated a prognostic model related to xenobiotic metabolism for predicting the outcome of colon cancer patients and explored the related characteristics of the tumor immune microenvironment. However, this study had some limitations. First, the model we constructed was only based on TCGA database; thus, the data of diverse races, regions, countries, or nations are needed to improve the general applicability of the prognostic model and the biomarkers. Second, due to time constraints, the latest research data could not be included. Third, due to the close relationship between xenobiotic metabolism and drugs and diet, dietary habits and medication history may affect the prognostic model and genes; however, due to a lack of information, these factors were not considered in this study, which may have led to a bias in the results. To address these issues, multicenter, large-scale clinical cohort studies including eating habits and drugs as correction factors need to be conducted to further validate the prognostic model and biomarkers in the future. This study can provide reference for the prevention and treatment of colon cancer from the perspective of xenobiotic metabolism.
Acknowledgments
Funding: This work was supported by the National Natural Science Foundation of China (81803773) and the Beijing Municipal Administration of Hospitals Incubating Program (PX2020078).
Footnote
Reporting Checklist: The authors have completed the TRIPOD reporting checklist. Available at https://dx.doi.org/10.21037/jgo-21-655
Conflicts of Interest: Both authors have completed the ICMJE uniform disclosure form (available at https://dx.doi.org/10.21037/jgo-21-655). Both authors have no conflicts of interest to declare.
Ethical Statement: The authors are accountable for all aspects of the work in ensuring that questions related to the accuracy or integrity of any part of the work are appropriately investigated and resolved. The study was conducted in accordance with the Declaration of Helsinki (as revised in 2013). As this was a retrospective database study that used a public database, approval from the ethics committee was not required.
Open Access Statement: This is an Open Access article distributed in accordance with the Creative Commons Attribution-NonCommercial-NoDerivs 4.0 International License (CC BY-NC-ND 4.0), which permits the non-commercial replication and distribution of the article with the strict proviso that no changes or edits are made and the original work is properly cited (including links to both the formal publication through the relevant DOI and the license). See: https://creativecommons.org/licenses/by-nc-nd/4.0/.
References
- Bray F, Ferlay J, Soerjomataram I, et al. Global cancer statistics 2018: GLOBOCAN estimates of incidence and mortality worldwide for 36 cancers in 185 countries. CA Cancer J Clin 2018;68:394-424. [Crossref] [PubMed]
- Arnold M, Abnet CC, Neale RE, et al. Global Burden of 5 Major Types of Gastrointestinal Cancer. Gastroenterology 2020;159:335-349.e15. [Crossref] [PubMed]
- Farinetti A, Zurlo V, Manenti A, et al. Mediterranean diet and colorectal cancer: A systematic review. Nutrition 2017;43-44:83-8. [Crossref] [PubMed]
- Saliba W, Rennert HS, Gronich N, et al. Red meat and processed meat intake and risk of colorectal cancer: a population-based case-control study. Eur J Cancer Prev 2019;28:287-93. [Crossref] [PubMed]
- Nogacka AM, Gómez-Martín M, Suárez A, et al. Xenobiotics Formed during Food Processing: Their Relation with the Intestinal Microbiota and Colorectal Cancer. Int J Mol Sci 2019;20:2051. [Crossref] [PubMed]
- Wu JC, Tsai ML, Lai CS, et al. Polymethoxyflavones prevent benzoapyrene/dextran sodium sulfate-induced colorectal carcinogenesis through modulating xenobiotic metabolism and ameliorate autophagic defect in ICR mice. Int J Cancer 2018;142:1689-701. [Crossref] [PubMed]
- Kamiza AB, You JF, Wang WC, et al. Polymorphisms of xenobiotic-metabolizing genes and colorectal cancer risk in patients with lynch syndrome: A retrospective cohort study in Taiwan. Environ Mol Mutagen 2018;59:69-78. [Crossref] [PubMed]
- Lubelska K, Wiktorska K, Mielczarek L, et al. Sulforaphane Regulates NFE2L2/Nrf2-Dependent Xenobiotic Metabolism Phase II and Phase III Enzymes Differently in Human Colorectal Cancer and Untransformed Epithelial Colon Cells. Nutr Cancer 2016;68:1338-48. [Crossref] [PubMed]
- Kwon D, Kim SM, Correia MA. Cytochrome P450 endoplasmic reticulum-associated degradation (ERAD): therapeutic and pathophysiological implications. Acta Pharm Sin B 2020;10:42-60. [Crossref] [PubMed]
- Dong SC, Sha HH, Xu XY, et al. Glutathione S-transferase π: a potential role in antitumor therapy. Drug Des Devel Ther 2018;12:3535-47. [Crossref] [PubMed]
- Tourancheau A, Rouleau M, Guauque-Olarte S, et al. Quantitative profiling of the UGT transcriptome in human drug-metabolizing tissues. Pharmacogenomics J 2018;18:251-61. [Crossref] [PubMed]
- Guengerich FP. Cytochrome p450 and chemical toxicology. Chem Res Toxicol 2008;21:70-83. [Crossref] [PubMed]
- Nallani SC, Genter MB, Desai PB. Increased activity of CYP3A enzyme in primary cultures of rat hepatocytes treated with docetaxel: comparative evaluation with paclitaxel. Cancer Chemother Pharmacol 2001;48:115-22. [Crossref] [PubMed]
- Liu M, Wang Q, Liu F, et al. UDP-glucuronosyltransferase 1A compromises intracellular accumulation and anti-cancer effect of tanshinone IIA in human colon cancer cells. PLoS One 2013;8:e79172. [Crossref] [PubMed]
- Klaassen CD, Aleksunes LM. Xenobiotic, bile acid, and cholesterol transporters: function and regulation. Pharmacol Rev 2010;62:1-96. [Crossref] [PubMed]
- Chen DS, Mellman I. Oncology Meets Immunology: The Cancer-Immunity Cycle. Immunity 2013;39:1-10. [Crossref] [PubMed]
- Tsatsakis A, Petrakis D, Nikolouzakis TK, et al. COVID-19, an opportunity to reevaluate the correlation between long-term effects of anthropogenic pollutants on viral epidemic/pandemic events and prevalence. Food Chem Toxicol 2020;141:111418. [Crossref] [PubMed]
- Lamas B, Natividad JM, Sokol H. Aryl hydrocarbon receptor and intestinal immunity. Mucosal Immunol 2018;11:1024-38. [Crossref] [PubMed]
- Schiering C, Wincent E, Metidji A, et al. Feedback control of AHR signalling regulates intestinal immunity. Nature 2017;542:242-5. [Crossref] [PubMed]
- Beyerle J, Holowatyj AN, Haffa M, et al. Expression Patterns of Xenobiotic-Metabolizing Enzymes in Tumor and Adjacent Normal Mucosa Tissues among Patients with Colorectal Cancer: The ColoCare Study. Cancer Epidemiol Biomarkers Prev 2020;29:460-9. [Crossref] [PubMed]
- Xu X, Lu Y, Wu Y, et al. A signature of seven immune-related genes predicts overall survival in male gastric cancer patients. Cancer Cell Int 2021;21:117. [Crossref] [PubMed]
- Kim E, Coelho D, Blachier F. Review of the association between meat consumption and risk of colorectal cancer. Nutr Res 2013;33:983-94. [Crossref] [PubMed]
- Yin H, Shao J. MicroRNA-643 promotes proliferation and inhibits apoptosis of papillary thyroid carcinoma by down-regulating the cytochrome P450 family member 11B1. Transl Cancer Res 2020;9:1465-75. [Crossref]
- Chung FF, Mai CW, Ng PY, et al. Cytochrome P450 2W1 (CYP2W1) in Colorectal Cancers. Curr Cancer Drug Targets 2016;16:71-8. [Crossref] [PubMed]
- Stenstedt K, Hallstrom M, Johansson I, et al. The expression of CYP2W1: a prognostic marker in colon cancer. Anticancer Res 2012;32:3869-74. [PubMed]
- Travica S, Pors K, Loadman PM, et al. Colon cancer-specific cytochrome P450 2W1 converts duocarmycin analogues into potent tumor cytotoxins. Clin Cancer Res 2013;19:2952-61. [Crossref] [PubMed]
- Huang M, Zeng Y, Zhao F, et al. Association of glutathione S-transferase M1 polymorphisms in the colorectal cancer risk: A meta-analysis. J Cancer Res Ther 2018;14:176-83. [Crossref] [PubMed]
- Ay A, Gulyasar T, Alkanli N, et al. Investigation of the relationship between GSTM1 gene variations and serum trace elements, plasma malondialdehyde levels in patients with colorectal cancer. Mol Biol Rep 2021;48:6911-21. [Crossref] [PubMed]
- Corbet C, Bastien E, Santiago de Jesus JP, et al. TGFβ2-induced formation of lipid droplets supports acidosis-driven EMT and the metastatic spreading of cancer cells. Nat Commun 2020;11:454. [Crossref] [PubMed]
- Tang YA, Chen YF, Bao Y, et al. Hypoxic tumor microenvironment activates GLI2 via HIF-1α and TGF-β2 to promote chemoresistance in colorectal cancer. Proc Natl Acad Sci U S A 2018;115:E5990-9. [Crossref] [PubMed]
- Maschietto M, Piccoli FS, Costa CM, et al. Gene expression analysis of blastemal component reveals genes associated with relapse mechanism in Wilms tumour. Eur J Cancer 2011;47:2715-22. [Crossref] [PubMed]
- Cai T, Xiao J, Wang ZF, et al. Identification of differentially coexpressed genes in gonadotrope tumors and normal pituitary using bioinformatics methods. Pathol Oncol Res 2014;20:375-80. [Crossref] [PubMed]
- Shen S, Faouzi S, Souquere S, et al. Melanoma Persister Cells Are Tolerant to BRAF/MEK Inhibitors via ACOX1-Mediated Fatty Acid Oxidation. Cell Rep 2020;33:108421. [Crossref] [PubMed]
- Wang YN, Zeng ZL, Lu J, et al. CPT1A-mediated fatty acid oxidation promotes colorectal cancer cell metastasis by inhibiting anoikis. Oncogene 2018;37:6025-40. [Crossref] [PubMed]
- Sun LN, Zhi Z, Chen LY, et al. SIRT1 suppresses colorectal cancer metastasis by transcriptional repression of miR-15b-5p. Cancer Lett 2017;409:104-15. [Crossref] [PubMed]
- Li M, Wang H, Li W, et al. Identification and validation of an immune prognostic signature in colorectal cancer. Int Immunopharmacol 2020;88:106868. [Crossref] [PubMed]
- Wang D, Yang L, Yu W, et al. Colorectal cancer cell-derived CCL20 recruits regulatory T cells to promote chemoresistance via FOXO1/CEBPB/NF-κB signaling. J Immunother Cancer 2019;7:215. [Crossref] [PubMed]
- Pio R, Ajona D, Ortiz-Espinosa S, et al. Complementing the Cancer-Immunity Cycle. Front Immunol 2019;10:774. [Crossref] [PubMed]
- Sharpe AH, Pauken KE. The diverse functions of the PD1 inhibitory pathway. Nat Rev Immunol 2018;18:153-67. [Crossref] [PubMed]
- Anderson AC, Joller N, Kuchroo VK. Lag-3, Tim-3, and TIGIT: Co-inhibitory Receptors with Specialized Functions in Immune Regulation. Immunity 2016;44:989-1004. [Crossref] [PubMed]
- Liu W, Garrett SC, Fedorov EV, et al. Structural Basis of CD160:HVEM Recognition. Structure 2019;27:1286-1295.e4. [Crossref] [PubMed]
- Bogdan C. Nitric oxide synthase in innate and adaptive immunity: an update. Trends Immunol 2015;36:161-78. [Crossref] [PubMed]
- Haque S, Morris JC. Transforming growth factor-β: a therapeutic target for cancer. Hum Vaccin Immunother 2017;13:1741-50. [Crossref] [PubMed]
- Bui TM, Wiesolek HL, Sumagin R. ICAM-1: A master regulator of cellular responses in inflammation, injury resolution, and tumorigenesis. J Leukoc Biol 2020;108:787-99. [Crossref] [PubMed]
(English Language Editor: L. Huleatt)