Identification of a 3-gene signature for predicting the prognosis of stage II colon cancer based on microsatellite status
Introduction
Ranked thirdly in the most common malignant tumor worldwide, colorectal cancer (CRC) is of the second lethal cancer across ages. It was estimated that at 2020 there were more than 1.9 million new cases and 935,000 deaths of CRC all over the world (1). Although combinations neoadjuvant therapy, surgery, adjuvant therapy and immunotherapy have improved survival, approximately 25–30% of stage II CRC patients develop recurrence within 5 years (2-4). The National Comprehensive Cancer Network (NCCN) has recommended adjuvant chemotherapy to improve prognosis in patients with stage II CRC and the following features: T4, <12 lymph nodes investigated, poorly differentiated or undifferentiated histology, perineural invasion, lymphovascular invasion, bowel obstruction and/or perforation, positive resection margins and tumor budding (5).
Factors associated with the prognosis of patients with CRC include TNM stage, perineural invasion, lymphovascular invasion, bowel obstruction, and bowel perforation. Traditional biomarkers such as carcinoembryonic antigen (CEA) concentration (6) and emerging biomarkers such as microsatellite instability (MSI) (7), KRAS, and BRAF mutations (8,9), miRNAs (10), lncRNAs (11) and circRNAs (12) can also affect patient outcomes. However, these clinical biomarkers are unable to precisely stratify patients for personalized treatment. CEA has relatively low sensitivity and specificity (13), whereas assessments of KRAS and BRAF mutations, and miRNAs, lncRNAs, and circRNAs are expense and require advanced technology.
Microsatellites are prone to DNA replication errors owing to the slippage of DNA polymerase during the course of DNA replication and these replication errors can be repaired by DNA mismatch repair (MMR) (7). MSI, which has been detected in approximately 15% of CRCs (14), is thought to play an essential part in the tumorigenesis and development of CRC (15-17). Tumors can be categorized by polymerase chain reaction (PCR) as MSI-high (MSI-H), MSI-low (MSI-L) or microsatellite stable (MSS). Cancers also can be classified as deficient MMR (dMMR) or proficient MMR (pMMR) based on the immunohistochemical results of MLH1, MSH2, MSH6, and PMS2. In general, PCR and immunohistochemical results are in agreement (18), with colon tumors divided into two subtypes: MSI-H/dMMR and MSI-L, MSS/pMMR.
Tumors classified as MSI-H have a lower tendency to metastasize and patients with stage II MSI-H/dMMR may have a more favorable outcome than those with stage II MSI-L/MSS (19-22). The NCCN has therefore recommended that MSI or MMR be tested in every newly diagnosed CC patient. Patients with stage II MSI-H CC do not require adjuvant therapy as they do not benefit from 5-FU adjuvant therapy (21), whereas immunotherapy is recommended to treat patients with stage IV MSI-H CC (23). The molecular mechanisms underlying the association between favorable outcomes and MSI-H in patients with stage II CC remain unelucidated. The present study therefore attempted to identify potential biomarkers associated with the prognosis of MSI-H stage II CC patients in the GEO database. Figure 1 described the workflow of the present study. We present the following article in accordance with the MDAR reporting checklist (available at https://dx.doi.org/10.21037/jgo-21-405).
Methods
Colon cancer microarray datasets and data screening
The GEO database (http://www.ncbi.nlm.nih.gov/) is a public functional genomics database that collects next-generation sequence and high-throughput microarray data with regard to different diseases. These data, which are submitted by researchers worldwide, may also include related prognostic data, such as overall survival (OS), disease-free survival (DFS), and recurrence-free survival (RFS). The colon cancer microarrays GSE143985, GSE39582, and GSE92921 downloaded through the GEO database shared the same platform, GPL570. Stage II patients with known microsatellite status and survival data were enrolled in the present study. The levels of expression of the genes in GSE143985 and GSE92921 were log2 transformed for following analysis. The study was conducted in accordance with the Declaration of Helsinki (as revised in 2013).
Robust rank aggregation (RRA) analysis
Utilizing the limma (linear models for microarray data) package, up-ranked and down-ranked gene lists of GSE143985 and GSE92921 were generated based on fold changes of their expressions between the MSI-H and MSS subgroups. Then the above gene lists were integrated using the “Robust Rank Aggregation” R package to screen for DEGs for further Cox regression analysis. Genes with |log2 fold change (FC)| ≥1 and P-value <0.05 were considered as DEGs.
Construction of a prognostic signature
Forty DEGs obtained from RRA were analyzed by univariate Cox regression analysis, with five of these found to be significantly associated with DFS. These genes were subjected to multivariate Cox stepwise regression analysis to determine the optimal prognostic signature. Ultimately, the prognostic signature was constructed according to the regression coefficients of these genes in the multivariate Cox stepwise regression analysis and the levels of expression of these genes. This signature was utilized to calculate risk scores for each patient. The median risk score was determined, and then the whole was sorted into high and low-risk subgroups based on the median risk score.
Functional annotation
The Metascape database (24) (http://metascape.org/) is a functional and pathway enrichment analysis database that includes the results of various databases such as Gene Ontology (GO) Biological Processes, Reactome Gene Sets, Canonical Pathways and Kyoto Encyclopedia of Genes and Genomes Pathway (KEGG pathway). Metascape was therefore applied to carry out functional annotation with DEGs between high and low-risk subgroups. The DEGs were determined by log2 FC ≥0.5 or log2 FC ≤−0.5 and P value <0.05 using the limma package.
Gene set enrichment analysis (GSEA)
GSEA was performed in GSEA software (version 4.1.0) (25,26) with the h.all.v7.4.symbols.gmt gene sets database to compare tumor hallmarks in the high and low-risk subgroups. The genes were permuted 1,000 times in the software before generated the statistical significance of the enrichment score.
Immune infiltration analysis
Using R package “CIBERSORT”, the 22 immune cells infiltration proportions of each CC patients were calculated based on the expression values of CC patients in the training cohort. Differences in immune cells infiltration were subsequently compared in the different subgroups.
Statistical analysis
Kaplan-Meier survival analysis was performed using “survival” R package and visualized by “survminer” R package to compare the DFS of patients between subgroups with a two-sided log-rank test. Receiver operating characteristic (ROC) curves were plotted with “survivalROC” R package and areas Under ROC curves (AUC) were calculated to evaluate the diagnostic power of the signature for 1-, 3-, and 5-year DFS. Univariate and multivariate Cox regression analyses, which included factors such as KRAS mutation, TP53 mutation and risk score, were performed to determine whether risk score is independently prognostic for survival. Wilcoxon Signed-Rank test was used to compare the levels of expression of MSMB, KRT23, and QPRT and the risk scores between subgroups based on BRAF mutation, KRAS mutation and TP53 mutation. All statistics analyses were conducted in R software (version 4.0.1).
Results
Characteristics of included colon cancer microarrays
Selection of patients with stage II and known microsatellite status and survival outcomes resulted in the inclusion of 322 CC patients, 96 in the training cohort and 226 in the validating cohort. Of these 322 patients, 49 were classified as MSI-H/pMMR and 273 as MSS/dMMR patients. The rates of MSI-H/dMMR varied from 9.3% to 17.3% across datasets. The details were summarized in Table 1.
Table 1
GSE ID | Participants | Tissues | Analysis type | GPL | Year |
---|---|---|---|---|---|
GSE143985 | 5 cases and 49 controls | Colon | Array | GPL570 | 2020 |
GSE92921 | 5 cases and 37 controls | Colon | Array | GPL570 | 2018 |
GSE39582 | 39 cases and 187 controls | Colon | Array | GPL570 | 2013 |
RRA analysis
The RRA method is widely used to integrate data from different sources, aggregate the results of multiple microarray studies and minimize inconsistencies. These properties of RRA analysis are due to four characteristics: its robustness to noise, its capacity to settle incomplete rankings, its provision of significant scores for every object in the final rankings, and its excellent computation efficiency. RRA integrated analysis identified 40 DEGs. Among these, 20 genes (ANXA10, TFF2, CHST6, REG4, TCN1, TM4SF4, SEMG1, TNNT1, TFF1, SDR16C5, VNN1, TRIM7, B3GALT5, GJB5, MSMB, CAPN9, HSPA4L, ZIC2, AGR3 and ISL1) were up-regulated while 20 genes (CEL, FREM2, OLFM4, PPP1R14C, EREG, CHP2, MAP7D2, GPR143, ATP6V1C2, PNLIPRP2, KRT23, RPS4Y1, VAV3, WIF1, PRAP1, ISX, CELP, QPRT, SOSTDC1 and USP9Y) were down-regulated in the MSI-H subgroup (Figure 2A).
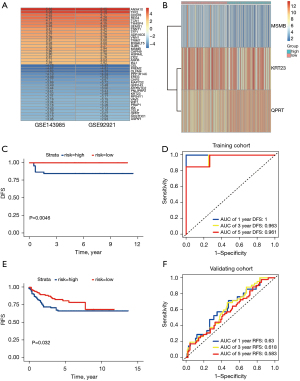
Development of a gene prognostic signature in the training cohort
The 40 dysregulated genes identified by RRA analysis were subjected to univariate Cox regression analysis, which identified five genes, MSMB, CEL, KRT23, WIF1 and QPRT, which were significantly associated with DFS (P-value <0.05 each). Four of these genes, MSMB, CEL, KRT23 and WIF1, were protective genes with hazard ratios (HRs) <1, whereas QPRT was associated with increased risk with an HR >1. Using multivariate Cox stepwise regression analysis, these five genes, were used to develop an integrated prognostic signature. Stepwise regression analysis provided an optimal prognostic signature according to the Akaike Information Criterion (AIC), a type of measure of goodness of fit in statistical models, with a smaller AIC value being associated with a better goodness of fit of the model. The prognostic signature (MKQ signature) was generated based on genes expression levels and regression coefficients, yielding the equation: risk score = (−1.809566) × MSMB + (−1.073572) × KRT23 + 2.477175 × QPRT.
Assessment and validation of the MKQ signature
To assess the prognostic predictive value of the MKQ signature, 96 CC patients were dichotomized into high and low-risk subgroups based on the median risk score. Kaplan-Meier survival analysis (Figure 2) showed that DFS was shorter in the high-risk than in the low-risk subgroup (P=0.0046). ROC analysis of the MKQ signature confirmed its ability to predicting the prognosis of patients with stage II CC. The AUCs of the time-dependent ROC curves of the MKQ signature for 1-, 3-, and 5-year DFS were 1.0, 0.963 and 0.961, respectively (Figure 2). Lower risk was associated with higher MSMB and KRT23 and lower QPRT expression (Figures 2,3). The superiority of the MKQ signature relative to single-gene signature was further accessed by ROC analysis of a model testing MSMB, KRT23, and QPRT (Figures 2,3). Univariate (Table 2) and multivariate (Table 3) Cox regression analyses were performed to assess the prognostic significance of KRAS mutation, TP53 mutation and risk score in the training cohort. These analyses showed that risk score can serve as an independent prognostic factor in patients with stage II CC.
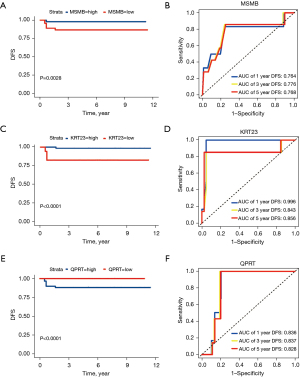
Table 2
Clinicopathological characteristics | HR | 95% CI | P value |
---|---|---|---|
KRAS mutation | 10.848 | 1.305, 90.173 | 0.027 |
TP53 mutation | 0.370 | 0.083, 1.653 | 0.193 |
Risk score | 2.718 | 1.523, 4.852 | <0.001 |
DFS, disease free survival.
Table 3
Clinicopathological characteristics | HR | 95% CI | P value |
---|---|---|---|
KRAS mutation | 0.912 | 0.050, 16.582 | 0.951 |
Risk score | 2.753 | 1.355, 5.596 | 0.005 |
DFS, disease free survival.
To further verify the predictive ability and clinical significance of the MKQ signature, risk scores were calculated for patients in the GSE39582 validating cohort. Based on their median risk scores, these 226 CC patients in the GSE39582 cohort could be divided into high and low-risk CC subgroups, with 39 classified as dMMR and 187 as pMMR. Kaplan-Meier survival analysis (Figure 2) indicated that RFS was significantly longer in patients with lower risk than higher scores in the GSE39582 (P=0.032). The results of the time-dependent ROC curves (Figure 2) showing that the AUCs for 1-, 3-, and 5-year RFS were 0.63, 0.618 and 0.583, respectively, confirmed the predictive property of the 3-gene signature in the validating cohort. A MKQ signature was therefore constructed to predict tumor recurrence in CC patients with stage II CC.
Relationships among MSMB, KRT23, QPRT, risk scores and clinicopathological characteristics of CC
The relationships of MSMB, KRT23, QPRT, and risk scores with the clinicopathological characteristics of CC, such as BRAF mutation, KRAS mutation and TP53 mutation were investigated. MSMB expression differed significantly in patients with wildtype and mutant KRAS (Figure 4A), but did not differ significantly in patients with the wildtype and mutant BRAF (Figure 4B) or in patients with wildtype and mutant TP53 (Figure 4C). The expression of KRT23 was lower in patients with mutant than wildtype BRAF (Figure 4D), did not differ in patients with mutant than wildtype KRAS (Figure 4E) and was higher in patients with mutant than wildtype TP53 (Figure 4F). The levels of expression of QPRT were not significantly affected by BRAF mutation (Figure 4G), KRAS mutation (Figure 4H) and TP53 mutation (Figure 4I). In addition, risk scores, did not differ significantly in patients with wildtype and mutant BRAF (Figure 4J), KRAS (Figure 4K) and TP53 (Figure 4L).
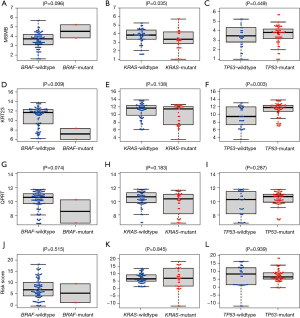
Pathway and process enrichment analysis and GSEA
To comprehend the potential biological effects of the molecular differences between the high and low-risk subgroups, 969 DEGs with log2 FCs ≥0.5 or ≤0.5 and P values <0.05 were identified between the high and low-risk subgroups in the training cohort. The pathway and process enrichment analysis in the Metascape database showed that these DEGs were enriched in the following pathways: inflammatory response, leukocyte chemotaxis, leukocyte activation involved in immune response, regulation of cell adhesion, humoral immune response, regulation of monocyte chemotaxis (GO Biological Processes); NABA matrisome associated, NABA ECM affiliated (Canonical Pathways); interleukin-10 signaling (Reactome Gene Sets); complement and coagulation cascades and staphylococcus aureus infection (KEGG Pathway) (Figure 5A-5C). GSEA demonstrated that KRAS signaling, IL6-JAK-STAT3-signaling, complement, inflammatory response, interferon gamma response, apical junction and other tumor hallmarks were enriched in the low-risk subgroup (Figure 5D). These results may enhance understanding of the molecular pathways associated with the MKQ signature.
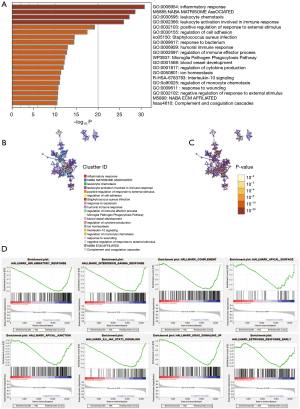
Immune infiltration analysis
As the results in the enrichment analysis showed that the MKQ signature was involved in several immune related pathways, immune infiltration analysis was performed with “CIBERSORT” R package to investigate the 22 immune cells infiltration proportions of each CC patients (Figure 6A). Figure 6B described the relationship between the proportions of infiltrating cells and patients subgroups. Further analysis showed significant infiltration of high-risk CC samples by naive B cells, CD4 naive T cells, resting NK cells and activated dendritic cells, as well as significant infiltration of low-risk CC samples by M1 macrophages, eosinophils, and neutrophils (Figure 6C).
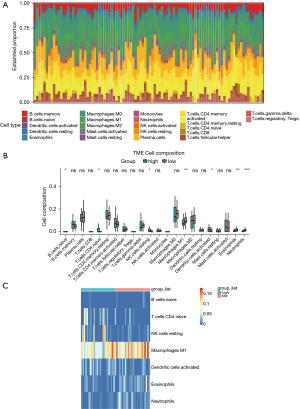
Discussion
Since MSI was described by Aaltonen et al first in hereditary nonpolyposis colorectal cancer in 1993 (27), a great quantity of studies concentrated on MSI have been conducted in colorectal cancer. Similar histopathologic characteristics detected in MSI-H tumors included enrichment of Th1 related cytokines, increased neoantigen formation, and somatic hypermutation (28). Several immune checkpoints, such as PD-1, PD-L1 and CTLA-4, were found to be upregulated in the MSI-H tumor microenvironment (29). High infiltration of immune cells infiltration into MSI-H CRC suggested that immunoregulatory mechanisms were associated with CRC tumorigenesis and appealed thousands of researchers to explore the mechanisms. Furthermore, immune checkpoint blockades have been approved for the treatment of mCRC with MSI.
Although MSI-H is regarded as a protective factor in stage II CC, the molecular mechanisms underlying these associations haven’t been elucidated. Therefore, the present study focused on genes differently expressed in stage II CC patients with and without MSI-H. Three genes, MSMB, KRT23 and QPRT, were found to be associated with DFS in CC patients, with stepwise multivariate Cox regression analysis determining a gene signature involving genes identified by univariate Cox regression analysis. This signature could classify patients with stage II CC and known microsatellite status into groups at high and low risk of disease recurrence in both the training and validating cohorts. Then functional annotation performed in Metascape database and GSEA analysis indicated that several immune related pathways were involved in the enrichment analysis. Besides, immune infiltration analysis showed differences between the high and low-risk subgroups in immune cell infiltration into tumors.
MSMB, also known as microseminoprotein-beta, encoding prostatic secretory protein of 94 amino acids (PSP94), a primary constituent of semen, is a prostate cancer biomarker (30). The function of MSMB in CC has not been determined yet. The present study suggested that MSMB may play a protective role in stage II CC as increased MSMB expression was associated with a longer RFS. Previous studies (31-33) revealed that the level of MSMB expression was found to be higher in normal and benign prostate tissue than in prostate cancer, suggesting that MSMB acted as a tumor-suppressor during the development of prostate cancer (34). MSMB was shown to suppress the synthesis of matrix metalloproteinases, which are involved in tumor metastasis (33) and curb vascularization mediated by vascular endothelial growth factor (35).
KRT23, which encodes Keratin 23, is considered as a gene in type I acute‐phase response. Previous studies (36-38) demonstrated that KRT23 was highly expressed in CC but low in para-cancerous tissues and the expression of KRT23 was associated with tumor stage and lymph node metastasis in CRC (39). A study of tumor samples from 40 patients reported that increased KRT23 expression correlated with promoter hypomethylation (40). The study (40) also pointed out that knockdown of KRT23 inhibited cell proliferation of CC and affected the DNA damage response and repair. Interestingly, the present study showed that high expression of KRT23 prolonged DFS in patients with stage II CC, consistent with the higher expression of KRT23 in the low-risk subgroup and Birkenkamp-Demtroder K et al described the similar result in their study (37). In the same study, they pointed out that the accumulation of KRT23 inhabited the MSI cell lines cellular viability but MSS cell lines did not show the same result. Consistent with the results of the present study, KRT23 was found to be downregulated in MSI tumors while upregulated in MSS tumors (37). Taken together, these findings suggest that KRT23 may be a potential prognostic indicator and therapeutic target in CC. However, in-depth studies are required to determine the function of KRT23 in stage II CC.
QPRT, quinolinate phosphoribosyl transferase, represents as a key enzyme in the kynurenine pathway participating in nicotinamide adenine dinucleotide (NAD+) biosynthesis (41). The level of NAD+ is abnormally high during cancer cell proliferation (42), with NAD+ depletion resulting in cancer cell death. The present study indicated that QPRT might act as an oncogene in CC, as higher QPRT expression was associated with poorer patient outcomes. QPRT was also found to be upregulated in breast cancer (43) and downregulated in renal cell cancer (44). Upregulated QPRT expression was regarded as a harmful factor in breast cancer (45). QPRT was found to enhance breast cancer invasiveness by means of phosphorylation of myosin light chain (45). To the best of our knowledge, however, the role of QPRT has not been investigated in CC.
As the functional annotation and GSEA analysis indicated that several immune related pathways were involved in MKQ signature, differences in the infiltration of 22 types of immune cells were evaluated in the high-risk and low-risk subgroups with the CIBERSORT algorithm. Infiltration of seven types of tumor-infiltrating immune cells was found to differ significantly in these subgroups. The high-risk subgroup showed upregulation of naive B cells, CD4 naive T cells, resting NK cells and activated dendritic cells, but downregulation of M1 macrophages, eosinophils, and neutrophils. These differences might be associated with the immune pathways of the MKQ signature. Therefore, additional studies into the roles of MSMB, KRT23 and QPRT in immune infiltration may enhance the understanding of molecular mechanisms of MSI-H in CC.
The present study described the development and validation a 3-gene MKQ signature that could classify stage II CC patients with known microsatellite status into different risk subgroups for DFS based on GEO databases. The MKQ signature performed well in both the training and validating cohorts. Moreover, the MKQ signature may act as biomarker to facilitate risk stratified management in stage II CC patients. Inevitably, this study had some limitations. First, the mechanisms of action of MSMB and QPRT in the regulation of CC remain unknown, requiring further investigations. Second, large scale prospective clinical trials are needed for further validation of the MKQ signature in stage II CC.
Conclusions
This study identified a 3-gene signature in stage II CC that may enhance our understanding of the molecular mechanisms underlying the better prognosis in patients with MSI-H stage II CC. This signature may provide new therapy biomarkers to target for the treatment of stage II CC.
Acknowledgments
We would like to thank Rachael Clayton for his help in polishing our paper.
Funding: This work was supported by National Natural Science Foundation of China (81871981, 81702902, 8170111484), Natural Science Foundation of Guangdong Province (2019A1515010646) and Medical Scientific Research Foundation of Guangdong Province of China (A2018319).
Footnote
Reporting Checklist: The authors have completed the MDAR reporting checklist. Available at https://dx.doi.org/10.21037/jgo-21-405
Conflicts of Interest: All authors have completed the ICMJE uniform disclosure form (available at https://dx.doi.org/10.21037/jgo-21-405). The authors have no conflicts of interest to declare.
Ethical Statement: The authors are accountable for all aspects of the work in ensuring that questions related to the accuracy or integrity of any part of the work are appropriately investigated and resolved. The study was conducted in accordance the Helsinki Declaration (as revised in 2013). All data in this article is obtained from the public database and no ethical approval has been applied.
Open Access Statement: This is an Open Access article distributed in accordance with the Creative Commons Attribution-NonCommercial-NoDerivs 4.0 International License (CC BY-NC-ND 4.0), which permits the non-commercial replication and distribution of the article with the strict proviso that no changes or edits are made and the original work is properly cited (including links to both the formal publication through the relevant DOI and the license). See: https://creativecommons.org/licenses/by-nc-nd/4.0/.
References
- Sung H, Ferlay J, Siegel RL, et al. Global Cancer Statistics 2020: GLOBOCAN Estimates of Incidence and Mortality Worldwide for 36 Cancers in 185 Countries. CA Cancer J Clin 2021;71:209-49. [Crossref] [PubMed]
- Obrand DI, Gordon PH. Incidence and patterns of recurrence following curative resection for colorectal carcinoma. Dis Colon Rectum 1997;40:15-24. [Crossref] [PubMed]
- Manfredi S, Bouvier AM, Lepage C, et al. Incidence and patterns of recurrence after resection for cure of colonic cancer in a well defined population. Br J Surg 2006;93:1115-22. [Crossref] [PubMed]
- Qaderi SM, Galjart B, Verhoef C, et al. Disease recurrence after colorectal cancer surgery in the modern era: a population-based study. Int J Colorectal Dis 2021;36:2399-410. [Crossref] [PubMed]
- Benson AB 3rd, Schrag D, Somerfield MR, et al. American Society of Clinical Oncology recommendations on adjuvant chemotherapy for stage II colon cancer. J Clin Oncol 2004;22:3408-19. [Crossref] [PubMed]
- Thirunavukarasu P, Sukumar S, Sathaiah M, et al. C-stage in colon cancer: implications of carcinoembryonic antigen biomarker in staging, prognosis, and management. J Natl Cancer Inst 2011;103:689-97. [Crossref] [PubMed]
- Baretti M, Le DT. DNA mismatch repair in cancer. Pharmacol Ther 2018;189:45-62. [Crossref] [PubMed]
- Andreyev HJ, Norman AR, Cunningham D, et al. Kirsten ras mutations in patients with colorectal cancer: the 'RASCAL II' study. Br J Cancer 2001;85:692-6. [Crossref] [PubMed]
- Fariña-Sarasqueta A, van Lijnschoten G, Moerland E, et al. The BRAF V600E mutation is an independent prognostic factor for survival in stage II and stage III colon cancer patients. Ann Oncol 2010;21:2396-402. [Crossref] [PubMed]
- Schetter AJ, Leung SY, Sohn JJ, et al. MicroRNA expression profiles associated with prognosis and therapeutic outcome in colon adenocarcinoma. JAMA 2008;299:425-36. [Crossref] [PubMed]
- Kogo R, Shimamura T, Mimori K, et al. Long noncoding RNA HOTAIR regulates polycomb-dependent chromatin modification and is associated with poor prognosis in colorectal cancers. Cancer Res 2011;71:6320-6. [Crossref] [PubMed]
- Weng W, Wei Q, Toden S, et al. Circular RNA ciRS-7-A Promising Prognostic Biomarker and a Potential Therapeutic Target in Colorectal Cancer. Clin Cancer Res 2017;23:3918-28. [Crossref] [PubMed]
- Koulis C, Yap R, Engel R, et al. Personalized Medicine-Current and Emerging Predictive and Prognostic Biomarkers in Colorectal Cancer. Cancers (Basel) 2020;12:812. [Crossref] [PubMed]
- Boland CR, Goel A. Microsatellite instability in colorectal cancer. Gastroenterology 2010;138:2073-2087.e3. [Crossref] [PubMed]
- Konishi M, Kikuchi-Yanoshita R, Tanaka K, et al. Molecular nature of colon tumors in hereditary nonpolyposis colon cancer, familial polyposis, and sporadic colon cancer. Gastroenterology 1996;111:307-17. [Crossref] [PubMed]
- Jass JR. Classification of colorectal cancer based on correlation of clinical, morphological and molecular features. Histopathology 2007;50:113-30. [Crossref] [PubMed]
- Ogino S, Chan AT, Fuchs CS, et al. Molecular pathological epidemiology of colorectal neoplasia: an emerging transdisciplinary and interdisciplinary field. Gut 2011;60:397-411. [Crossref] [PubMed]
- Hissong E, Crowe EP, Yantiss RK, et al. Assessing colorectal cancer mismatch repair status in the modern era: a survey of current practices and re-evaluation of the role of microsatellite instability testing. Mod Pathol 2018;31:1756-66. [Crossref] [PubMed]
- Ribic CM, Sargent DJ, Moore MJ, et al. Tumor microsatellite-instability status as a predictor of benefit from fluorouracil-based adjuvant chemotherapy for colon cancer. N Engl J Med 2003;349:247-57. [Crossref] [PubMed]
- Guastadisegni C, Colafranceschi M, Ottini L, et al. Microsatellite instability as a marker of prognosis and response to therapy: a meta-analysis of colorectal cancer survival data. Eur J Cancer 2010;46:2788-98. [Crossref] [PubMed]
- Sargent DJ, Marsoni S, Monges G, et al. Defective mismatch repair as a predictive marker for lack of efficacy of fluorouracil-based adjuvant therapy in colon cancer. J Clin Oncol 2010;28:3219-26. [Crossref] [PubMed]
- Klingbiel D, Saridaki Z, Roth AD, et al. Prognosis of stage II and III colon cancer treated with adjuvant 5-fluorouracil or FOLFIRI in relation to microsatellite status: results of the PETACC-3 trial. Ann Oncol 2015;26:126-32. [Crossref] [PubMed]
- Le DT, Uram JN, Wang H, et al. PD-1 Blockade in Tumors with Mismatch-Repair Deficiency. N Engl J Med 2015;372:2509-20. [Crossref] [PubMed]
- Zhou Y, Zhou B, Pache L, et al. Metascape provides a biologist-oriented resource for the analysis of systems-level datasets. Nat Commun 2019;10:1523. [Crossref] [PubMed]
- Mootha VK, Lindgren CM, Eriksson KF, et al. PGC-1α-responsive genes involved in oxidative phosphorylation are coordinately downregulated in human diabetes. Nat Genet 2003;34:267-73. [Crossref] [PubMed]
- Subramanian A, Tamayo P, Mootha VK, et al. Gene set enrichment analysis: a knowledge-based approach for interpreting genome-wide expression profiles. Proc Natl Acad Sci U S A 2005;102:15545-50. [Crossref] [PubMed]
- Aaltonen LA, Peltomäki P, Leach FS, et al. Clues to the pathogenesis of familial colorectal cancer. Science 1993;260:812-6. [Crossref] [PubMed]
- Dudley JC, Lin MT, Le DT, et al. Microsatellite Instability as a Biomarker for PD-1 Blockade. Clin Cancer Res 2016;22:813-20. [Crossref] [PubMed]
- Rizvi NA, Hellmann MD, Snyder A, et al. Mutational landscape determines sensitivity to PD-1 blockade in non-small cell lung cancer. Science 2015;348:124-8. [Crossref] [PubMed]
- Garde SV, Basrur VS, Li L, et al. Prostate secretory protein (PSP94) suppresses the growth of androgen-independent prostate cancer cell line (PC3) and xenografts by inducing apoptosis. Prostate 1999;38:118-25. [Crossref] [PubMed]
- Tsurusaki T, Koji T, Sakai H, et al. Cellular expression of beta-microseminoprotein (beta-MSP) mRNA and its protein in untreated prostate cancer. Prostate 1998;35:109-16. [Crossref] [PubMed]
- Sakai H, Tsurusaki T, Kanda S, et al. Prognostic significance of beta-microseminoprotein mRNA expression in prostate cancer. Prostate 1999;38:278-84. [Crossref] [PubMed]
- Annabi B, Bouzeghrane M, Currie JC, et al. A PSP94-derived peptide PCK3145 inhibits MMP-9 secretion and triggers CD44 cell surface shedding: implication in tumor metastasis. Clin Exp Metastasis 2005;22:429-39. [Crossref] [PubMed]
- Vanaja DK, Cheville JC, Iturria SJ, et al. Transcriptional silencing of zinc finger protein 185 identified by expression profiling is associated with prostate cancer progression. Cancer Res 2003;63:3877-82. [PubMed]
- Lamy S, Ruiz MT, Wisniewski J, et al. A prostate secretory protein94-derived synthetic peptide PCK3145 inhibits VEGF signalling in endothelial cells: implication in tumor angiogenesis. Int J Cancer 2006;118:2350-8. [Crossref] [PubMed]
- Birkenkamp-Demtroder K, Christensen LL, Olesen SH, et al. Gene expression in colorectal cancer. Cancer Res 2002;62:4352-63. [PubMed]
- Birkenkamp-Demtroder K, Mansilla F, Sørensen FB, et al. Phosphoprotein Keratin 23 accumulates in MSS but not MSI colon cancers in vivo and impacts viability and proliferation in vitro. Mol Oncol 2007;1:181-95. [Crossref] [PubMed]
- Broussard EK, Kim R, Wiley JC, et al. Identification of putative immunologic targets for colon cancer prevention based on conserved gene upregulation from preinvasive to malignant lesions. Cancer Prev Res (Phila) 2013;6:666-74. [Crossref] [PubMed]
- Gao X, Yang J. Identification of Genes Related to Clinicopathological Characteristics and Prognosis of Patients with Colorectal Cancer. DNA Cell Biol 2020;39:690-9. [Crossref] [PubMed]
- Birkenkamp-Demtröder K, Hahn SA, Mansilla F, et al. Keratin23 (KRT23) knockdown decreases proliferation and affects the DNA damage response of colon cancer cells. PLoS One 2013;8:e73593. [Crossref] [PubMed]
- Guo J, Lam LT, Longenecker KL, et al. Identification of novel resistance mechanisms to NAMPT inhibition via the de novo NAD+ biosynthesis pathway and NAMPT mutation. Biochem Biophys Res Commun 2017;491:681-6. [Crossref] [PubMed]
- Sahm F, Oezen I, Opitz CA, et al. The endogenous tryptophan metabolite and NAD+ precursor quinolinic acid confers resistance of gliomas to oxidative stress. Cancer Res 2013;73:3225-34. [Crossref] [PubMed]
- Yue Z, Shusheng J, Hongtao S, et al. Silencing DSCAM-AS1 suppresses the growth and invasion of ER-positive breast cancer cells by downregulating both DCTPP1 and QPRT. Aging (Albany NY) 2020;12:14754-74. [Crossref] [PubMed]
- Hornigold N, Dunn KR, Craven RA, et al. Dysregulation at multiple points of the kynurenine pathway is a ubiquitous feature of renal cancer: implications for tumour immune evasion. Br J Cancer 2020;123:137-47. [Crossref] [PubMed]
- Liu CL, Cheng SP, Chen MJ, et al. Quinolinate Phosphoribosyltransferase Promotes Invasiveness of Breast Cancer Through Myosin Light Chain Phosphorylation. Front Endocrinol (Lausanne) 2021;11:621944. [Crossref] [PubMed]