ANTXR1 as a potential prognostic biomarker for hepatitis B virus-related hepatocellular carcinoma identified by a weighted gene correlation network analysis
Introduction
Liver cancer is the 6th most common type of cancer and the 4th leading cause of cancer-related death (1). Hepatocellular carcinoma (HCC) is the main type of primary liver cancer. HCC is highly malignant, and its characteristics include an insidious onset, and rapid invasive growth. It has high recurrence and mortality rates. Except for patients in Taiwan and Japan, ≥60% of patients in China, Korea, North America, and Europe with HCC are diagnosed in the intermediate or advanced stages (2). These patients often have a poor prognosis. There is increasing evidence that early detection could significantly improve the overall survival (OS) of HCC patients (3). Thus, it is essential to explore novel diagnostic and therapeutic biomarkers to reduce the high mortality of HCC.
Hepatitis B virus (HBV) infection is one of the most attributable causes of HCC (4). In China, liver cancer is associated with HBV infection in 85% of patients (5). It has been estimated that approximately 291,992,000 people are infected with HBV worldwide. Among them, only 29 million have been diagnosed and only 4.8 million have received antiviral therapy, which only appears to ameliorate (and not eliminate) HBV (6). Chronic carriers with persistent virus or subviral particles in their blood for >6 months develop progressive chronic liver diseases that eventually manifest as hepatic inflammation, fibrosis, cirrhosis, and even HCC (7). However, little is known about the precise molecular mechanism underlying HBV-related hepatocarcinogenesis.
With advancements in next-generation sequencing and genomic analysis technology, growing cancer biomarkers, such as oncogenes, micro ribonucleic acids (RNAs), specific peptides and deoxyribonucleic acid (DNA) methylation have been identified as having the potential to detect HCC early (8-11). HBV-related hepatocarcinogenesis possesses its own specific characteristics. Some prognostic biomarkers have been identified through proteogenomic analyses, which have greatly expanded understandings of HBV-related HCC (12). However, the development of HCC is a complex process involving multiple factors. Thus, there is an urgent need to identify novel diagnostic and prognostic biomarkers for HBV-related HCC.
Weighted gene co-expression network analyses (WGCNAs) have been widely used to analyze correlations between clinical features and gene modules (13). In our study, we examined two key gene modules associated with HBV-related HCC using a WGCNA. Based on the protein-protein interaction (PPI) network of the genes in the key modules, the top 10 hub genes were identified. Further, the survival-related hub genes were analyzed. This study provides further insights into the complex mechanisms underlying HBV-related HCC and identifies novel hub genes that could have potential value as diagnostic and therapeutic biomarkers for HBV-related HCC. We present the following article in accordance with the REMARK reporting checklist (available at https://dx.doi.org/10.21037/jgo-21-764).
Methods
Data information
The HCC RNA-sequencing data were retrieved from The Cancer Genome Atlas (TCGA)-Liver Hepatocellular Carcinoma database (https://portal.gdc.cancer.gov/). This study comprised 424 HCC patients (57 of whom had hepatitis B Surface Antigen positive status and 127 who did not have a HBV infection). The patients’ clinical information was also downloaded, including their height, weight, race, gender, age, tumor, node, metastasis (TNM) stage, pathological stage, vital status, new tumor event status, cancer status, and HBV status (see available online: https://cdn.amegroups.cn/static/public/jgo-21-764-01.pdf). The study was conducted in accordance with the Declaration of Helsinki (as revised in 2013).
WGCNA
As genes with little variation in expression usually represent noise, the most variant genes were used for the network construction. The expression levels of genes were measured as fragments per kilobase of transcript per million (FPKM) mapped reads. Based on FPKM, the genes were first ranked from largest to smallest, and the top 5,000 genes were then chosen for cluster analysis using the hClust function in R (13). After filtering out the outliers in the data set, a WGCNA was conducted to build a scale-free network. First, a soft-thresholding power β was determined to ensure that the co-expression network was a “scale-free” network that was biologically close to reality. To determine the best soft threshold power, the scale independence and average connectivity degree of the modules with different power values were calculated using the gradient method. Next, the adjacency was transformed into a topological overlap matrix and the corresponding dissimilarity was calculated. A hierarchical clustering dendrogram of the topological overlap matrix was then constructed by the average distance with a minimum size threshold of 30, and a merge cut height of 0.25 to classify the similar gene expression profiles into different gene modules. The interaction relationships among different co-expression modules were visualized on a heat map with 400 genes randomly selected.
Identification of modules associated with clinical traits
The correlations between the module eigengenes (MEs) and clinical traits were calculated by a module-trait relationship analysis. Next, gene significance (GS) was defined as the log10 transformation of the P value (GS = lg P) in the linear regression between gene expression and clinical characteristics. For each module, we also defined a quantitative measure of module membership (MM) as the correlations between the MEs and the gene expression profiles. The correlations between GS and MM were visualized in a scatter plot. The most significant relevant modules were selected as the key modules.
Functional enrichment analysis
To explore potential the biological functions and pathways of genes in the modules, the clusterprofiler package in R (14) was used to perform the Gene ontology (GO) and Kyoto Encyclopedia of Genes and Genomes (KEGG) pathway enrichment analyses. The GO terms include biological process (BP), cellular component (CC), and molecular function (MF).
Construction of the PPI network and identification of hub genes
A PPI network of the genes in the key modules was constructed using Cytoscape (15). The top 10 genes with the highest maximal clique centrality were identified as hub genes using the CytoHubba plugin in Cytoscape (16). Further, the core subnetworks were constructed based on the PPI network through the MCODE plugin in Cytoscape (17).
Survival analysis
The survival package in R was used to evaluate the OS-related genes based on the 58 HCC patients who were infected by HBV (18). Hazard ratios (HRs) and 95% confidence intervals were calculated using a Cox regression model.
Statistical analysis
All data were presented as mean ± SE through three independent experiments. Unpaired t-test and chi-square test were used for statistical analysis. The statistical data were performed by SPSS 21.0 software (IBM Corp., NY, USA), and diagrammed by GraphPad Prism 6.0 (GraphPad Software Inc., CA, USA). P<0.05 was considered to be indicative of statistical significance.
Results
WGCNA
The 5,000 most variant genes according to the FPKM values were selected for the hcluster analysis to detect outliers. As Figure 1A shows, 1 outlier sample was excluded. The soft-thresholding power parameter β is an important parameter for the scale-free topology. Thus, we performed a network topology analysis for thresholding powers from 1 to 20 and identified relatively balanced scale independence and mean connectivity (see Figure 1B). When β=5 was selected as the soft threshold power, scale free R2=0.88 (Figure 1C,1D). The co-expression network exhibits a scale-free topology. Further, the merge cut height was set as 0.25 to merge similar modules. Finally, 10 modules were identified through the cluster dendrogram (see Figure 2A). Each module was assigned a unique color as an identifier. The number of genes in the modules ranged from 59 to 1,070 (see Table 1). After randomly selecting 400 of the 5,000 genes, the relationships between the 10 modules were analyzed, and the network heatmap was plotted (see Figure 2B). The results showed that the modules were independent of each other; that is, there was a high degree of independence among the modules and the gene expression in each module was relative independent.
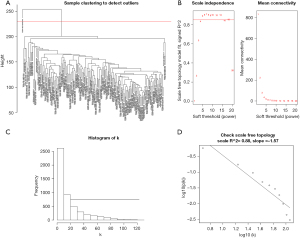
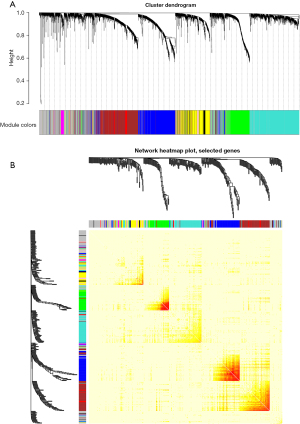
Table 1
Module | No. of gene |
---|---|
Black | 120 |
Blue | 869 |
Brown | 780 |
Green | 479 |
Grey | 857 |
Magenta | 59 |
Pink | 82 |
Red | 157 |
Turquoise | 1,070 |
Yellow | 527 |
Identification of clinical trait-related modules
To explore the clinical trait significance of each module, correlations between MEs and clinical traits, including height, weight, race, gender, age, TNM stage, pathologic stage, vital status, new tumor event status, cancer status, and HBV status were analyzed. A P value <0.05 was considered statistically significantly. In the modules, the yellow and brown modules were significantly associated with both HBV and race. Further, the yellow module was correlated with weight (see Figure 3A). We then plotted a scatter plot of GS versus MM for the selected modules. The yellow module (cor =0.39, P=1.4e−20) and the brown module (cor =0.43, P=1.9e−36) were identified as the modules to the virus infection status of HBV. Figure 3B and Figure 3C show the correlations between MM and GS in the yellow and brown modules, respectively.
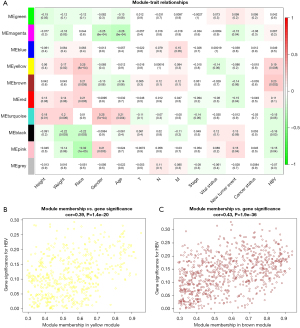
Functional enrichment analysis
To examine the functions of genes in the yellow and brown modules, the genes in the two modules were analyzed by clusterProfile in R for the GO and KEGG pathway enrichment analyses. As Figure 4A shows, the GO enrichment analysis results revealed that the yellow module was significantly related to the cell-cell junction (CC), laminin binding, and extracellular matrix binding (MF). However, no KEGG pathway was significantly enriched. The GO enrichment analysis results also showed that the genes in the brown module were mainly enriched in BP (e.g., extracellular matrix organization and extracellular structure organization), the CC (e.g., the collagen-containing extracellular matrix), and MF (e.g., the extracellular matrix structural constituent and glycosaminoglycan binding) (see Figure 4B). According to the KEGG enrichment analysis results, 10 pathways were significantly enriched, including the phosphatidylinositol-3-kinase and protein kinase b (PI3K-Akt) signaling pathway, focal adhesion, human papillomavirus infection, the Rap1 signaling pathway, extracellular matrix-receptor interaction, the cGMP-PKG signaling pathway, protein digestion and absorption, the relaxin signaling pathway, vascular smooth muscle contraction, and the AGE-RAGE signaling pathway (see Figure 4C). Most of these pathways have been confirmed to be closely related with HCC.
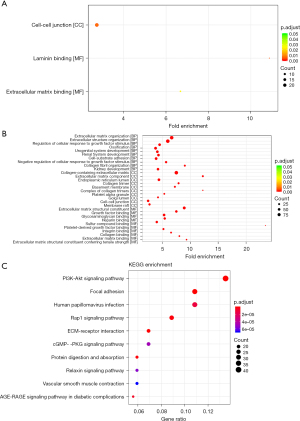
Construction of PPI network and identification of hub genes
In this study, an interaction score ≥0.25 and 0.15 was set as the cut-off criterion for the brown module and the yellow module, respectively. The results were visualized using Cytoscape. There were 428 PPI pairs in the PPI network of the brown module (see Figure 5A), and 527 interaction pairs in the yellow module (see Figure 5B). We identified hub genes in the two modules according to Mathew correlation coefficient (MCC) using the cytohubba plugin in Cytoscape. 10 hub genes [i.e., Collagen Type III Alpha 1 Chain (COL3A1), Anthrax-toxin receptor 1 (ANTXR1), Collagen Type XIV Alpha 1 Chain (COL14A1), Thrombospondin 2 (THBS2), ADAM Metallopeptidase With Thrombospondin Type 1 Motif 2 (ADAMTS2), AE Binding Protein 1 (AEBP1), Proline And Arginine Rich End Leucine Rich Repeat Protein (PRELP), Elastin Microfibril Interfacer 1 (EMILIN1), Decorin (DCN), and Podocan (PODN)] were identified in the brown module (see Figure 6A), and 10 hub genes [i.e., Ubiquitin Specific Peptidase 34 (USP34), SEC24 Homolog C, COPII Coat Complex Component (SEC24C), Zinc Finger Protein 770 (ZNF770), Stromal Antigen 1 (STAG1), Thiosulfate Sulfurtransferase Like Domain Containing 2 (TSTD2), Polycystin 1, Transient Receptor Potential Channel Interacting Pseudogene 6 (PKD1P6), Cyclin K (CCNK), General Transcription Factor IIi (GTF2I), 5'-Nucleotidase, Cytosolic II (NT5C2), and SMG6 Nonsense Mediated MRNA Decay Factor (SMG6)] were identified in the yellow module (see Figure 6B). We also constructed core subnetworks for the brown and yellow modules using the MCDOE plugin in Cytoscape. As expected, the above-mentioned hub genes were all in the 2 core subnetworks (see Figure 7A,7B).
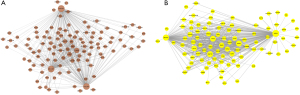
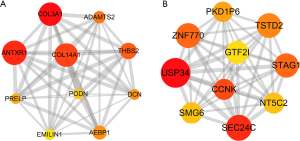
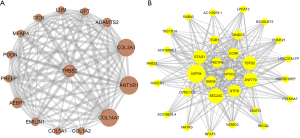
Identification of the survival-related hub genes
A survival analysis was performed to evaluate the effect of all genes on OS based on the 58 HBV infection-related HCC patients. The patients were divided into two groups based on the median value of each gene. The results showed that 469 genes were significantly associated with the prognosis of HBV-related HCC patients (see available online: https://cdn.amegroups.cn/static/public/jgo-21-764-02.pdf). Among them, there were 90 genes in the brown module and 30 genes in the yellow module. Further, 1 hub gene (i.e., ANTXR1) was significantly associated with HBV-related HCC patients’ OS [see Figure 8; HR =0.303 (0.125–0.738), P=0.00778].
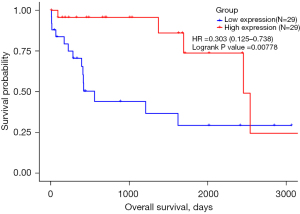
Discussion
For decades, researchers have sought to find better and valuable prognostic or predictive biomarkers for HCC patients. The WGCNA possesses many outstanding advantages over other methods, which makes the analysis results more reliable and more biologically significant. Some biomarkers associated with HBV-related HCC have been identified by WGCNAs in previous studies; for example, Protein Regulator of Cytokinesis 1 (PRC1) and DNA Topoisomerase II Alpha (TOP2A) were found to be significantly associated with poor clinical outcomes among patients with HBV-related HCC (19). However, little is known about how aberrant genes drive cancer phenotypes in HBV-related HCC.
Unlike other WGCNA studies that focused on discovering differentially expressed genes between HBV (+) HCC tumor tissues and HBV (+) normal liver tissues, we examined the association between co-expression modules and clinical traits based on the gene expression profiles of HCC tissues. In our study, we conducted a WGCNA to determine the clinical trait modules of HCC and identified two key modules (brown and yellow) that were significantly associated with HBV infection. Notably, we found that the brown and yellow modules were also significantly related to race. Previous studies have reported significant geographic and ethnic differences in HCC (20,21). From the perspective of genomics, it may become possible to analyze HBV infection susceptibility based on race. In this study, the data sets included 5 races (i.e., Asian, White, Black or African American and unknown). However, this classification is sketchy, and a more detailed study of the association between races and HBV-related HCC needs to be conducted.
Functional and pathway enrichment analyses are important to understand the functions and potential molecular mechanisms of a gene in a disease. In this study, our results revealed that the genes in the brown module were mainly involved in the PI3K-Akt signaling pathway, the Rap1 signaling pathway, focal adhesion, and the cGMP-PKG signaling pathway. Among these, the PI3K-Akt signaling pathway plays a pivotal role in the pathogenesis of HCC induced by HBV infection (22-25). The Rap1 signaling pathway is also associated with the development of HBV-related HCC. As previously shown, HBV infection elevates the expression of Rap1b by inhibiting miR-101-3p, and thereby promoting the proliferation and migration of HCC cells (26). In another study, HBV infection was shown to suppress Rap1a expression by up-regulating miR-203a, thereby mediating the PI3K/ERK/p38/NFκB pathway, and eventually inducing hepatitis inflammation (24). The interactions between the above two pathways are complex (27). These findings show the credibility of the data we obtained.
Focal adhesion kinase, a tyrosine kinase, is overexpressed in a variety of human tumors, including HCC (28). Many previous studies have reported that focal adhesion kinase overexpression is closely related to the pathological stage and clinical outcomes of patients with HCC (29). Notably, Wang demonstrated that notch activated by iNOS/NO/sGC/cGMP/PKG-dependent TACE may be an important mechanism in the promotion of liver cancer stem cell enrichment and function in HCC (30). To date, no research appears to have been conducted on the role of focal adhesion and the cGMP-PKG signaling pathway in HBV-related HCC patients. Notably, human papillomavirus infection was also found to be involved in the genes in the brown module, which suggests that different viral infections may share the same pathways. Further investigations should examine these aspects.
In the PPI network, 20 hub genes were identified (i.e., COL3A1, ANTXR1, COL14A1, THBS2, ADAMTS2, AEBP1, PRELP, EMILIN1, DCN, and PODN in the brown module, and USP34, SEC24C, ZNF770, STAG1, TSTD2, PKD1P6, CCNK, GFT2I, NT5C2, and SMG6 in the yellow module). Among hub genes, ANTXR1 was significantly correlated with HBV-related HCC patients’ OS. ANTXR1, also known as tumor endothelial marker 8 (TEM8), is a highly conserved single-pass cell-surface protein that was originally identified in endothelial cells derived from the blood vessels of human malignant colorectal tissues (31). It is also expressed in the endothelial cells (32,33) and stromal cells of tumors (34-36).
There is increasing evidence that ANTXR1 plays an important role in tumor angiogenesis (35). ANTXR1 could also mediate the receptor activator of nuclear factor kappa B ligand-induced osteoclast differentiation and bone resorption without affecting osteoblast differentiation (37). ANTXR1 knockdown may suppress tumor growth in multiple cancer types (34,38). ANTXR1 was confirmed to be a downstream molecule of miR-26b-3p in glioma (39) and miR-493 in HCC (40). Molecular mechanistic investigation indicated that ANTXR1 exerted its promoting effects through activation of the Wnt/beta-catenin signaling pathway in lung adenocarcinoma (41) and HCC (40). The PI3K/AKT/mTOR signaling pathway can also be activated by ANTXR1 in gastric cancer (42). Further, the disruption of ANTXR1 gene in mice cannot affect physiological angiogenesis and wound healing (33). This study suggests that ANTXR1 is necessary for tumor rather than physiological angiogenesis.
ANTXR1 is also an anthrax-toxin receptor, which binds to the nontoxic protective antigen component secreted by Bacillus anthracis, and mediates the enzymatic toxins into the cytoplasm of host target cells (43). The systemic anti-tumor activity of the anthrax lethal toxin has been observed in a range of human solid tumors attributed to ANTXR1 (44-47). Based on these features, TEM8 antagonists and the TEM8-mediated transport of anticancer drugs may have potential as effective cancer therapeutics. TEM8-Fc is an antibody-like molecule comprising the protective antigen-binding domain of human TEM8 linked to the Fc portion of human immunoglobulin G1, which has been shown to suppress the growth and metastasis of xenograft human tumors in athymic nude mice (48). TEM8 CAR T cells were used to kill triple-negative breast cancer cells in local and metastatic murine models (49). High ANTXR1 expression was found in gastric adenocarcinoma cells (50). ANTXR1 is also considered a probable target in CAR T-cell therapy for gastric adenocarcinoma (51). Anti-TEM8 antibody-drug conjugate treatment has been shown to induce regression and eradicate multiple solid tumor types, block metastatic growth, and prolong OS through a surprising killing mechanism called DAaRTS (drug activation and release through stroma) (36). Thus, the expression level of ATNXR1 in tumors might be used as a predictor of the treatment efficacy of ANTXR1-targeted cancer therapy.
A xenogeneic DNA vaccine encoding human TEM8 carried by attenuated Salmonella typhimurium could overcome peripheral immune tolerance and produce TEM8-specific CD8 cytotoxic T-cell responses (52). Yang (53) reported that dendritic cells transduced with TEM8 recombinant adenovirus prevent angiogenesis and inhibit cell growth of HCC. Recent research suggests that ANTXR1 is an essential receptor for the Seneca Valley virus infection (54), which is a prototype member of the Seneca virus genus in the Picornaviridae family (55). In our study, ANTXR1 was found to be significantly associated with HBV infection in HCC patients. Further, the KEGG analysis revealed that the genes in the brown module were significantly enriched in human papillomavirus infection, a virus infected pathway. These findings suggest that ANTXR1 is both an anthrax-toxin receptor and may be a virus receptor for many kinds of mammalian viruses. To date, no association between ANTXR1 and HBV infection has been reported; however, ANTXR1 may be a cellular receptor for HBV.
ANTXR1 might play a dual role in the pathogenesis and treatment of HBV-related HCC. First, it may act as a cellular receptor for HBV that assists HBV infection and promotes tumor growth. Second, ATNXR1 may transport certain unknown anticancer drugs into tumor cells to exert a killing mechanism, or some effective therapy induced by ANTXR1 overexpression. ANTXR1 expression is upregulated in endothelial cells of the gastric adenocarcinoma regions with a history of neoadjuvant therapy (50), and no therapeutic information is supplied in TCGA database. This may explain why HBV-related HCC patients with high ANTXR1 expression had a significantly longer OS time in our analysis. However, this study was limited by the small number of HBV (+) samples. The expression and function of ANTXR1 in HBV-related HCC tumorigenesis are still unclear, and need to be further validated by large-scale studies.
Conclusions
Our study suggests that ANTXR1, which regulates tumor angiogenesis and participates in transmembrane transport, is a potential therapeutic target for HCC. Further, it might play an important role in HBV infection and HBV-related HCC. These findings provide novel insights into the molecular mechanisms of HBV-related HCC.
Acknowledgments
Funding: This study was supported by funding from the National Natural Science Foundation of China (No. 82060856), and the National Natural Science Foundation of Guangxi (No. 2020JJA140194).
Footnote
Reporting Checklist: The authors have completed the REMARK reporting checklist (available at https://dx.doi.org/10.21037/jgo-21-764).
Conflicts of Interest: All authors have completed the ICMJE uniform disclosure form (available at https://dx.doi.org/10.21037/jgo-21-764). The authors have no conflicts of interest to declare.
Ethical Statement: The authors are accountable for all aspects of the work in ensuring that questions related to the accuracy or integrity of any part of the work are appropriately investigated and resolved. The study was conducted in accordance with the Declaration of Helsinki (as revised in 2013).
Open Access Statement: This is an Open Access article distributed in accordance with the Creative Commons Attribution-NonCommercial-NoDerivs 4.0 International License (CC BY-NC-ND 4.0), which permits the non-commercial replication and distribution of the article with the strict proviso that no changes or edits are made and the original work is properly cited (including links to both the formal publication through the relevant DOI and the license). See: https://creativecommons.org/licenses/by-nc-nd/4.0/.
References
- Yang JD, Hainaut P, Gores GJ, et al. A global view of hepatocellular carcinoma: trends, risk, prevention and management. Nat Rev Gastroenterol Hepatol 2019;16:589-604. [Crossref] [PubMed]
- Park JW, Chen M, Colombo M, et al. Global patterns of hepatocellular carcinoma management from diagnosis to death: the BRIDGE Study. Liver Int 2015;35:2155-66. [Crossref] [PubMed]
- Singal AG, Pillai A, Tiro J. Early detection, curative treatment, and survival rates for hepatocellular carcinoma surveillance in patients with cirrhosis: a meta-analysis. PLoS Med 2014;11:e1001624. [Crossref] [PubMed]
- Sartorius K, Sartorius B, Aldous C, et al. Global and country underestimation of hepatocellular carcinoma (HCC) in 2012 and its implications. Cancer Epidemiol 2015;39:284-90. [Crossref] [PubMed]
- Qin W, Han C, Mai R, et al. Establishment of a prognostic model for predicting short-term disease-free survival in cases of hepatitis B-related hepatocellular carcinoma with the TP53 249Ser mutation in southern China. Transl Cancer Res 2020;9:4517-33. [Crossref]
- Vasuri F, Visani M, Acquaviva G, et al. Role of microRNAs in the main molecular pathways of hepatocellular carcinoma. World J Gastroenterol 2018;24:2647-60. [Crossref] [PubMed]
- Roberts LR, Gores GJ. Hepatocellular carcinoma: molecular pathways and new therapeutic targets. Semin Liver Dis 2005;25:212-25. [Crossref] [PubMed]
- Kisiel JB, Dukek BA. Hepatocellular Carcinoma Detection by Plasma Methylated DNA: Discovery, Phase I Pilot, and Phase II Clinical Validation. Hepatology 2019;69:1180-92. [Crossref] [PubMed]
- Lin XJ, Chong Y, Guo ZW, et al. A serum microRNA classifier for early detection of hepatocellular carcinoma: a multicentre, retrospective, longitudinal biomarker identification study with a nested case-control study. Lancet Oncol 2015;16:804-15. [Crossref] [PubMed]
- Shang S, Plymoth A, Ge S, et al. Identification of osteopontin as a novel marker for early hepatocellular carcinoma. Hepatology 2012;55:483-90. [Crossref] [PubMed]
- Schulze K, Nault JC, Villanueva A. Genetic profiling of hepatocellular carcinoma using next-generation sequencing. J Hepatol 2016;65:1031-42. [Crossref] [PubMed]
- Gao Q, Zhu H, Dong L, et al. Integrated Proteogenomic Characterization of HBV-Related Hepatocellular Carcinoma. Cell 2019;179:561-577.e22. [Crossref] [PubMed]
- Langfelder P, Horvath S. WGCNA: an R package for weighted correlation network analysis. BMC Bioinformatics 2008;9:559. [Crossref] [PubMed]
- Yu G, Wang LG, Han Y, et al. clusterProfiler: an R package for comparing biological themes among gene clusters. OMICS 2012;16:284-7. [Crossref] [PubMed]
- Shannon P, Markiel A, Ozier O, et al. Cytoscape: a software environment for integrated models of biomolecular interaction networks. Genome Res 2003;13:2498-504. [Crossref] [PubMed]
- Chin CH, Chen SH, Wu HH, et al. cytoHubba: identifying hub objects and sub-networks from complex interactome. BMC Syst Biol 2014;8:S11. [Crossref] [PubMed]
- Bader GD, Hogue CW. An automated method for finding molecular complexes in large protein interaction networks. BMC Bioinformatics 2003;4:2. [Crossref] [PubMed]
- Therneau TM, T. Lumley. Package ‘ survival ’. R topics documented. 2015:128.
- Liao X, Yu T, Yang C, et al. Comprehensive investigation of key biomarkers and pathways in hepatitis B virus-related hepatocellular carcinoma. J Cancer 2019;10:5689-704. [Crossref] [PubMed]
- Le MH, Yeo YH, Cheung R, et al. Chronic Hepatitis B Prevalence Among Foreign-Born and U.S.-Born Adults in the United States, 1999-2016. Hepatology 2020;71:431-43. [Crossref] [PubMed]
- McMahon BJ, Holck P, Bulkow L, et al. Serologic and clinical outcomes of 1536 Alaska Natives chronically infected with hepatitis B virus. Ann Intern Med 2001;135:759-68. [Crossref] [PubMed]
- Chung W, Kim M, de la Monte S, et al. Activation of signal transduction pathways during hepatic oncogenesis. Cancer Lett 2016;370:1-9. [Crossref] [PubMed]
- Liu YC, Lu LF, Li CJ, et al. Hepatitis B Virus X Protein Induces RHAMM-Dependent Motility in Hepatocellular Carcinoma Cells via PI3K-Akt-Oct-1 Signaling. Mol Cancer Res 2020;18:375-89. [Crossref] [PubMed]
- Wu A, Chen H, Xu C, et al. miR-203a is involved in HBx-induced inflammation by targeting Rap1a. Exp Cell Res 2016;349:191-7. [Crossref] [PubMed]
- Zhu M, Li W, Lu Y, et al. HBx drives alpha fetoprotein expression to promote initiation of liver cancer stem cells through activating PI3K/AKT signal pathway. Int J Cancer 2017;140:1346-55. [Crossref] [PubMed]
- Su H, Yang JR, Xu T, et al. MicroRNA-101, down-regulated in hepatocellular carcinoma, promotes apoptosis and suppresses tumorigenicity. Cancer Res 2009;69:1135-42. [Crossref] [PubMed]
- Ma XL, Shen MN, Hu B, et al. CD73 promotes hepatocellular carcinoma progression and metastasis via activating PI3K/AKT signaling by inducing Rap1-mediated membrane localization of P110beta and predicts poor prognosis. J Hematol Oncol 2019;12:37. [Crossref] [PubMed]
- Golubovskaya VM. Targeting FAK in human cancer: from finding to first clinical trials. Front Biosci (Landmark Ed) 2014;19:687-706. [Crossref] [PubMed]
- Panera N, Crudele A, Romito I, et al. Focal Adhesion Kinase: Insight into Molecular Roles and Functions in Hepatocellular Carcinoma. Int J Mol Sci 2017;18:99. [Crossref] [PubMed]
- Wang R, Li Y, Tsung A, et al. iNOS promotes CD24+CD133+ liver cancer stem cell phenotype through a TACE/ADAM17-dependent Notch signaling pathway. Proc Natl Acad Sci U S A 2018;115:E10127-36. [Crossref] [PubMed]
- St Croix B, Rago C, Velculescu V, et al. Genes expressed in human tumor endothelium. Science 2000;289:1197-202. [Crossref] [PubMed]
- Carson-Walter EB, Watkins DN, Nanda A, et al. Cell surface tumor endothelial markers are conserved in mice and humans. Cancer Res 2001;61:6649-55. [PubMed]
- Cullen M, Seaman S, Chaudhary A, et al. Host-derived tumor endothelial marker 8 promotes the growth of melanoma. Cancer Res 2009;69:6021-6. [Crossref] [PubMed]
- Chaudhary A, Hilton MB, Seaman S, et al. TEM8/ANTXR1 blockade inhibits pathological angiogenesis and potentiates tumoricidal responses against multiple cancer types. Cancer Cell 2012;21:212-26. [Crossref] [PubMed]
- Nanda A, Carson-Walter EB, Seaman S, et al. TEM8 interacts with the cleaved C5 domain of collagen alpha 3(VI). Cancer Res 2004;64:817-20. [Crossref] [PubMed]
- Szot C, Saha S, Zhang XM, et al. Tumor stroma-targeted antibody-drug conjugate triggers localized anticancer drug release. J Clin Invest 2018;128:2927-43. [Crossref] [PubMed]
- Baek JM, Kwak SC, Yoon KH, et al. Role of ANTXR1 in the regulation of RANKL-induced osteoclast differentiation and function. Biochem Biophys Res Commun 2019;510:296-302. [Crossref] [PubMed]
- Fernando S, Fletcher BS. Targeting tumor endothelial marker 8 in the tumor vasculature of colorectal carcinomas in mice. Cancer Res 2009;69:5126-32. [Crossref] [PubMed]
- Geng F, Lu GF, Ji MH, et al. MicroRNA-26b-3p/ANTXR1 signaling modulates proliferation, migration, and apoptosis of glioma. Am J Transl Res 2019;11:7568-78. [PubMed]
- Xu Y, Ge K, Lu J, et al. MicroRNA-493 suppresses hepatocellular carcinoma tumorigenesis through down-regulation of anthrax toxin receptor 1 (ANTXR1) and R-Spondin 2 (RSPO2). Biomed Pharmacother 2017;93:334-43. [Crossref] [PubMed]
- Ding C, Liu J, Zhang J, et al. Tumor Endothelial Marker 8 Promotes Proliferation and Metastasis via the Wnt/beta-Catenin Signaling Pathway in Lung Adenocarcinoma. Front Oncol 2021;11:712371. [Crossref] [PubMed]
- Cai C, Dang W, Liu S, et al. Anthrax toxin receptor 1/tumor endothelial marker 8 promotes gastric cancer progression through activation of the PI3K/AKT/mTOR signaling pathway. Cancer Sci 2020;111:1132-45. [Crossref] [PubMed]
- Bradley KA, Mogridge J, Mourez M, et al. Identification of the cellular receptor for anthrax toxin. Nature 2001;414:225-9. [Crossref] [PubMed]
- Abi-Habib RJ, Singh R, Leppla SH, et al. Systemic anthrax lethal toxin therapy produces regressions of subcutaneous human melanoma tumors in athymic nude mice. Clin Cancer Res 2006;12:7437-43. [Crossref] [PubMed]
- Rouleau C, Menon K, Boutin P, et al. The systemic administration of lethal toxin achieves a growth delay of human melanoma and neuroblastoma xenografts: assessment of receptor contribution. Int J Oncol 2008;32:739-48. [PubMed]
- Liu S, Aaronson H, Mitola DJ, et al. Potent antitumor activity of a urokinase-activated engineered anthrax toxin. Proc Natl Acad Sci U S A 2003;100:657-62. [Crossref] [PubMed]
- Liu S, Liu J, Ma Q, et al. Solid tumor therapy by selectively targeting stromal endothelial cells. Proc Natl Acad Sci U S A 2016;113:E4079-87. [Crossref] [PubMed]
- Duan HF, Hu XW, Chen JL, et al. Antitumor activities of TEM8-Fc: an engineered antibody-like molecule targeting tumor endothelial marker 8. J Natl Cancer Inst 2007;99:1551-5. [Crossref] [PubMed]
- Byrd TT, Fousek K, Pignata A, et al. TEM8/ANTXR1-Specific CAR T Cells as a Targeted Therapy for Triple-Negative Breast Cancer. Cancer Res 2018;78:489-500. [Crossref] [PubMed]
- Sotoudeh M, Shakeri R, Dawsey SM, et al. ANTXR1 (TEM8) overexpression in gastric adenocarcinoma makes the protein a potential target of immunotherapy. Cancer Immunol Immunother 2019;68:1597-603. [Crossref] [PubMed]
- Sotoudeh M, Shirvani SI, Merat S, et al. MSLN (Mesothelin), ANTXR1 (TEM8), and MUC3A are the potent antigenic targets for CAR T cell therapy of gastric adenocarcinoma. J Cell Biochem 2019;120:5010-7. [Crossref] [PubMed]
- Ruan Z, Yang Z, Wang Y, et al. DNA vaccine against tumor endothelial marker 8 inhibits tumor angiogenesis and growth. J Immunother 2009;32:486-91. [Crossref] [PubMed]
- Yang X, Zhu H, Hu Z. Dendritic cells transduced with TEM8 recombinant adenovirus prevents hepatocellular carcinoma angiogenesis and inhibits cells growth. Vaccine 2010;28:7130-5. [Crossref] [PubMed]
- Miles LA, Burga LN, Gardner EE, et al. Anthrax toxin receptor 1 is the cellular receptor for Seneca Valley virus. J Clin Invest 2017;127:2957-67. [Crossref] [PubMed]
- Reddy PS, Burroughs KD, Hales LM, et al. Seneca Valley virus, a systemically deliverable oncolytic picornavirus, and the treatment of neuroendocrine cancers. J Natl Cancer Inst 2007;99:1623-33. [Crossref] [PubMed]