Identification of methyltransferase-like protein 11B as a new prognostic biomarker for colorectal cancer through an analysis of The Cancer Genome Atlas
Introduction
Colorectal cancer (CRC) is a common gastrointestinal tumor that occurs at the junction of the dentate line and rectosigmoid colon, and presents with polypoid and ulcerative foci (1,2). According to estimates released by the World Health Organization’s International Agency for Research on Cancer (IARC), the global incidence of new CRC cases exceeded 1.91 million in 2020, which accounted for 1/10 of all new tumor cases worldwide and made CRC the third highest ranking cancer worldwide (3). Although a number of studies have provided a large number of prognostic schemes and corresponding interventions to improve the prognosis of patients with CRC, the overall survival rate has not improved (4). Therefore, it is particularly important to find simpler and more efficient biomarkers to predict the prognosis of patients with CRC.
Recently, the immune status and nutritional status of cancer cell hosts have been linked to tumor progression (5-7). Representative factors are involved in tumor biological events through indirect or direct interaction with the tumor, and many researchers have assessed their use as biomarkers. To date, many studies have explored predicting the postoperative survival time of patients with CRC using these markers, including the platelet-to-lymphocyte ratio (8), neutrophil-to-lymphocyte ratio (NLR) (9), derived neutrophil-to-lymphocyte ratio (dNLR) (10), serum albumin (11), and serum prealbumin (12). NLR and dNLR are calculated differently. NLR = absolute value of neutrophil count/absolute value of lymphocyte count. dNLR = absolute value of neutrophil count/absolute value of leukocyte count − absolute value of neutrophil count. In recent years, the immune status and nutritional status of cancer cell host have been reported to be related to different tumor progression, and lymphocytes can also reflect the immune status and nutritional status of the host. Therefore, we chose NLR with more factors, which can also conduct more accurate research from more angles. Differentially expressed genes (DEGs) were determined based on the criteria of |log2FC|>2. Using |log2FC|>1 standard that gene screening is only have the differentially expressed genes. By raising |log2FC| value, we found that more differentially expressed genes were screened in the pre-experiment. Therefore, we according to |log2FC|>2 to determine the DEGs, rather than |log2FC|>1.
It is more accurate and efficient to assess the prognosis of patients with cancer from the perspectives of immune status and nutritional status. The neutrophil lymphocyte ratio/prealbumin ratio (NLR/PA) is a combined index of immunological and nutritional factors that has so far only been studied in esophageal cancer (13); it has yet to be analyzed and reported for other cancers.
Human methyltransferase-like (METTL) proteins are part of a big protein family that is defined by the binding domain of S-adenosyl methionine, which is the cosubstrate for methylation reactions (14-16). Although some representatives of this protein family have been identified as DNA, RNA, or protein methyltransferases, the majority of METTL proteins have yet to be identified. Previous studies have shown that while methylation can directly affect the chromatin organization and regulate gene transcription, it does not lead to gene mutations (17,18). Methyltransferase has also been linked to the development of hereditary illnesses, tumors, and metabolic disorders (19-22). METTL11B is a member of the METTL family that has so far been poorly explored and has yet to be investigated in CRC.
Therefore, this study had the goal of investigating whether an association exists between the prognosis of patients with colorectal carcinoma (CRC) and the neutrophil-lymphocyte ratio/prealbumin ratio (NLR/PA) and methyltransferase-like protein 11B (METTL11B) expression. The highlight of this study was mainly to screen the differential genes of colorectal cancer from TCGA database, and to screen the METTL11B with high differential expression significance from the differential genes, At the same time, combining the two aspects of immunity and nutritional status, we selected the serological parameter NLR/PA that is easiest to obtain in our clinical work. The prediction model based on these parameters can help clinicians identify high-risk patients in a timely and accurately after operation, and provide timely and reasonable treatment options. These data have good universality and practicability. We present the following article in accordance with the REMARK reporting checklist (available at https://dx.doi.org/10.21037/jgo-21-781).
Methods
Identification of differentially expressed genes in The Cancer Genome Atlas (TCGA) database
First, gene expression profiles of CRC tissues and para-cancer tissues were downloaded from TCGA, and DEGs were identified. DEGs were determined based on the criteria of |log2FC|>2 and P value <0.05.
Application of the TCGA and GEPIA databases for data analysis
Two databases, GEPIA (Gene Expression Profiling Interactive Analysis; http://gepia.cancer-pku.cn/detail.php) and TCGA (https://tcga-data.nci.nih.gov/), were employed to investigate the expression of METTL11B in CRC. The R 3.1.2 (R Foundation for Statistical Computing, Vienna, Austria) software was used to collect and analyze data. METTL11B gene expression was compared between CRC and normal tissues using the standard method of calculation (23).
Assessment of the immunological response of 24 tumor-infiltrating immune cells (TIICs) in CRC using CIBERSORT
CIBERSORT (24) (http://cibersort.stanford.edu/) is a deconvolution algorithm technique based on gene expression that can analyze variations in the expression of genes that occur in a cluster compared to all other genes in a sample. The concentrations of TIICs may be properly determined using this method. The consistent performance of CIBERSORT has sparked significant interest in cell heterogeneity research (25,26).
In this study, CIBERSORT was used to assess the immune response of 24 TIICs in CRC to determine whether a link exists between survival and molecular subpopulations. Briefly, gene expression values were arranged with standard annotation files and uploaded to the CIBERSORT online website, where the algorithm was performed using its default signature matrix at 1,000 permutations. Using Monte Carlo sampling, CIBERSORT calculated a P value for deconvolution, providing a measure of confidence in the results. To analyze the impact of METTL11B expression, we obtained 396 samples from TCGA containing all genes. The samples were separated into two groups based on the level of METTL11B expression: the high METTL11B expression group and the low METTL11B expression group. Finally, a vioplot was obtained.
Analyzing the relationship between METTL11B and microsatellite instability-related molecules
CRC data were retrieved from the TCGA database for analysis of the relationship between METTL11B and microsatellite instability (MSI)-related molecules.
METTL11B expression phenotype-enriched gene sets
The signaling cascades through which METTL11B may contribute to a poor prognosis of CRC were explored by means of gene enrichment analysis.
Selection of clinical data
The clinical records of 100 patients who underwent CRC surgery in Jiangsu Cancer Hospital between January 2017 and June 2018 were reviewed, and eligible patients were enrolled. The criteria for inclusion were as follows: (I) diagnosed with CRC and underwent surgery; (II) no factors affecting blood test results such as fever within 1 week before surgery; (III) blood tests were taken on the last day before surgery; and (IV) a complete set of data containing clinical, pathological, and follow-up records. Patients meeting any of the following criteria were excluded: (I) patients with a positive pathological margin post-surgery; (II) patients with a history of hematological or autoimmune disorders, or certain malignancies; (III) patients who had heart failure complications, high-risk hypertension; and (IV) patients who experienced serious complications during the perioperative period.
This study comprised 100 patients, including 56 males and 44 females. The study participants ranged in age from 42 to 69 years old, with the mean age being 58.6±7.2 years. All procedures performed in this study involving human participants were in accordance with the Declaration of Helsinki (as revised in 2013). The study was approved by Jiangsu Cancer Hospital’s ethics committee (No. 2016-062-06), and all samples were obtained with signed informed consent from the participants.
Tissue microarray construction and immunohistochemistry
Tissue samples were obtained from 100 patients with CRC who had undergone surgery at Jiangsu Cancer Hospital’s Department of Pathology between January 2017 and June 2018. The tissue microarray was designed by the Department of Pathology. Paraffin blocks of CRC tissue from the patients were stained with hematoxylin and eosin, and the most common characteristics were identified and labeled at fixed points under the microscope. There were fewer than 160 points in each point array. A 3-µm-thick portion was cut from the block and placed onto a slide for ultraviolet crosslinking using a tape transfer technique. During the immunohistochemical (IHC) evaluation, the cell staining intensity and proportion of positive cells were scored. The staining intensity was scored as follows: 0 (negative), 1 (weakly positive), 2 (moderately positive), and 3 (strongly positive). The proportion of positively stained cells was scored as follows: 0 (negative), 1 (≤25%), 2 (25–50%), 3 (51–75%), and 4 (>75%). The final score for each specimen was calculated by multiplying the staining intensity score by the positive cell staining score; the arithmetic means of these score values were then determined. Specimens with a total score of <7 were defined as having low METTL11B expression.
Correlations of NLR/PA and METTL11B with survival prognosis and clinical data parameters in individuals with CRC
The optimal critical value of NLR/PA was determined to be 0.0947 by a receiver operating characteristic (ROC) curve. Using this value as the cut-off, the samples were categorized into two groups based on the patients’ preoperative NLR/PA: the high NLR/PA group (≥0.0947) and the low NLR/PA group (<0.0947). Also, based on the IHC expression of METTL11B, the study participants were differentiated into the low METTL11B expression group and the high METTL11B expression group.
SPSS Version 20.0 software was employed to compare the correlations of clinical data with survival prognosis and clinical parameters between the two groups. The significant independent predictive factors for CRC prognosis were then screened through univariate and multivariate analyses.
Nomograms construction
The independent factors affecting the prognosis of patients with CRC which were obtained by Cox regression analyses were edited using R syntax, and nomograms were subsequently constructed.
Follow-up
Follow-up strategies included collection of readmission clinical information of the patient, outpatient review, and telephone follow-up. Patients were followed up until June 1, 2021. Outcome measures included overall survival (OS) and progression-free survival (PFS). OS was defined as the duration of time from cancer detection to death from any cause or the end of follow-up. PFS was described as the duration of time from the start of therapy to any indication of disease progression during follow-up. At the final data cut-off, data of patients who were still alive and those who had died during follow-up were evaluated.
Statistical analysis
The R (version 3.6.3) software was used for statistical analysis and visualization. The following R packages were used: GEOquery (version 2.54.1) (27) was used for data download; limma (version 3.42.2) (28) was used for variation analysis; and the ggplot2 (version 3.3.3) and ComplexHeatmap (version 2.2.0) (29) were used for heat map visualization. The potential biological mechanism of METTL11B activity was investigated through gene set enrichment analysis (GSEA). Statistical analyses were performed using the SPSS 22.0 (IBM) software. The clinicopathological features of the two treatment groups were compared using the chi-square test. The Kaplan-Meier method was adopted to assess patient survival, and significance testing was conducted using the log-rank statistical approach. Independent prognostic indicators for CRC were identified using a Cox proportional-hazards regression model. The nomograms were drawn and the prediction model was built using the R programming language. A statistically significant difference was indicated by P<0.05.
Results
Determination of DEGs
CRC-related DEGs were identified from the TCGA database. The DEGs were screened out using the criteria log2FC >5 and P<0.05. Finally, METTL11B was verified as a DEG (Figure 1).
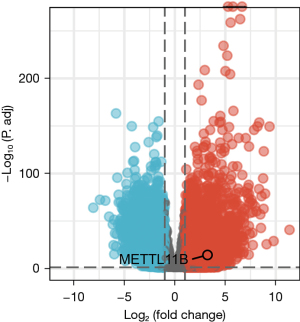
METTL11B expression in the TCGA database
The expression levels of METTL11B were compared between CRC tissues and normal tissues from the TCGA database. The messenger RNA (mRNA) expression of METTL11B was substantially up-regulated in CRC tissues compared to healthy tissues. METTL11B expression was consistent in the databases (Figure 2).
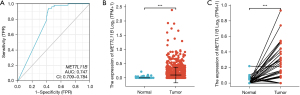
Association between the clinical characteristics of patients with CRC and METTL11B in the TCGA database
Patients exhibiting low METTL11B expression had an improved prognosis compared to patients with high METTL11B expression in the TCGA database. In the subgroup analysis, patients in the node (N) 0, N1, and N2 groups with low METTL11B expression had better OS than those with a high expression. Further, statistically significant differences were observed in the level of carcinoembryonic antigen, the presence of colon polyps, N stage, pathological stage, tumor (T) stage, and weight between the high and low METTL11B expression groups (all P<0.05) (Figure 3).
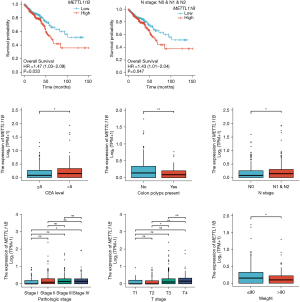
Relationships between METTL11B and MSI-related molecules
METTL11B was found to interact with MSI-related molecules (PMS1, MSH6, PMS2, MLH1, MLH3), and all interactions were statistically significant (P<0.05) (Figure 4).

Immune expression of METTL11B in 24 TIICs in CRC
Next, the relationship between METTL11B expression and immune invasion in CRC was investigated. To this end, the well-known computational tool CIBERSORT was used to examine the gene expression profiles of the obtained CRC samples, and the density of 24 different TIICs was estimated. The CIBERSORT algorithm was first used on 24 immune cell subtypes and then employed to compare the concentrations of these cells in the high and low METTL11B expression groups. At the same time, the percentages of the 24 TIIC subtypes were obtained. T helper 17 (Th17) cells, T helper 1 (Th1) cells, natural killer (NK) cells, CD56bright NK cells, gammadelta T (Tgd) cells, T follicular helper cells (Tfhs), T helper cells, effector memory T (TEMs) cells, central memory T cells (TCMs), mast cells, neutrophils, bright cells, macrophages, interstitial dendritic cells (IDCs), eosinophils, and dendritic cells (DCs) were the important immune cells influenced by METTL11B expression (all P<0.001) (Figure 5).
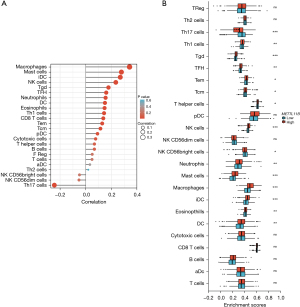
GSEA results
The GSEA analysis results uncovered four potentially statistically significant CRC-related pathways: CALCIUM_SIGNALING_PATHWAY, PATHWAYS_IN_CANCER, REACTOME_PLATELET_ACTIVATION_SIGNALING_AND_AGGREGATION, and WP_PI3KAKT_SIGNALING (Figure 6).
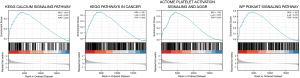
METTL11B expression in IHC
The levels of METTL11B expression were examined in 100 CRC tissues and 14 healthy para-cancer tissues. METTL11B was found to be lowly expressed in 62 (62.0%) CRC tissue samples and highly expressed in 38 (38.0%) CRC tissue samples (Figure 7).
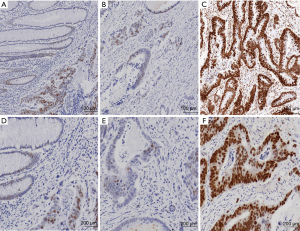
Association between tumor progression and NLR/PA
Figure 1 shows the ROC curve for the association between tumor growth and preoperative NLR/PA in the 100 patients with CRC. The best cut-off value for NLR/PA was 0.0947 (Figure 8).
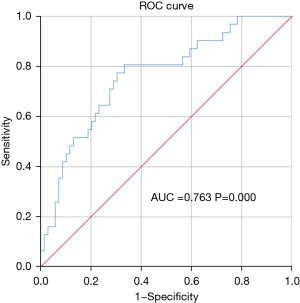
Comparison of PFS and OS between the two groups
All of the patients were tracked for 3 years, at the end of which 69 patients were still alive (69.0%) and 31 patients had died, translating to a mortality rate of 31.0%. Kaplan-Meier survival analysis revealed that patients in the low NLR/PA and low METTL11B expression groups had significantly longer OS and PFS than those in the high NLR/PA and high METTL11B expression groups, respectively (all P<0.05) (Figure 9).

Relationships of clinicopathological data with NLR/PA and METTL11B
No significant statistical differences were detected in clinicopathological data between the low and high NLR/PA expression groups. However, there were statistically significant differences between the high and low METTL11B expression groups in terms of body weight reduction, CA199 levels, serum albumin levels, chemotherapy use, and the incidence of vascular cancer thrombus (all P<0.05) (Table 1).
Table 1
Variable | Total (n=100) | Low NLR/PA (<0.0947) (n=52) | High NLR/PA (≥0.0947) (n=48) | P | Low METTL11B expression (n=62) | High METTL11B expression (n=38) | P |
---|---|---|---|---|---|---|---|
Sex | |||||||
Male | 56 | 29 | 27 | 0.961 | 31 | 25 | 0.123 |
Female | 44 | 23 | 21 | 31 | 13 | ||
Age (years) | |||||||
<64 | 40 | 21 | 19 | 0.935 | 27 | 13 | 0.355 |
≥64 | 60 | 31 | 29 | 35 | 25 | ||
Body mass reduction (kg) | |||||||
<5 | 45 | 26 | 19 | 0.296 | 33 | 12 | 0.035* |
≥5 | 55 | 26 | 29 | 29 | 26 | ||
Body mass index (kg/m2) | |||||||
<18.5 | 20 | 11 | 9 | 0.764 | 12 | 8 | 0.837 |
≥18.5 | 80 | 41 | 39 | 50 | 30 | ||
CEA | |||||||
≤5 | 37 | 19 | 18 | 0.921 | 20 | 17 | 0.210 |
>5 | 63 | 33 | 30 | 42 | 21 | ||
CA199 (U/mL) | |||||||
<37 | 54 | 30 | 24 | 0.441 | 41 | 13 | 0.002* |
≥37 | 46 | 22 | 24 | 21 | 25 | ||
Serum albumin | |||||||
<35 | 65 | 29 | 36 | 0.044 | 34 | 31 | 0.007* |
≥35 | 35 | 23 | 12 | 28 | 7 | ||
Tumor size (cm) | |||||||
<3 | 59 | 31 | 28 | 0.896 | 40 | 19 | 0.152 |
≥3 | 41 | 21 | 20 | 22 | 19 | ||
Tumor differentiation | |||||||
Moderately or highly differentiated | 43 | 25 | 18 | 0.286 | 26 | 17 | 0.784 |
Poorly differentiated | 57 | 27 | 30 | 36 | 21 | ||
Tumor location | |||||||
Medium or high location | 36 | 16 | 20 | 0.257 | 25 | 11 | 0.250 |
Low location | 64 | 36 | 28 | 37 | 27 | ||
TNM stage | |||||||
I–II | 45 | 27 | 18 | 0.148 | 29 | 16 | 0.649 |
III–IV | 55 | 25 | 30 | 33 | 22 | ||
Infiltration depth | |||||||
Early (TIS + T1) | 33 | 17 | 16 | 0.946 | 21 | 12 | 0.813 |
Progression period (T2–T4) | 67 | 35 | 32 | 41 | 26 | ||
Chemotherapy | |||||||
Yes | 61 | 39 | 22 | 0.003* | 45 | 16 | 0.002* |
No | 39 | 13 | 26 | 17 | 22 | ||
Distant metastasis | |||||||
Yes | 14 | 12 | 2 | 0.006* | 10 | 4 | 0.433 |
No | 86 | 40 | 46 | 52 | 34 | ||
Lymph node metastasis | |||||||
Yes | 16 | 11 | 5 | 0.143 | 9 | 7 | 0.605 |
No | 84 | 41 | 43 | 53 | 31 | ||
Nerve invasion | |||||||
Yes | 25 | 17 | 8 | 0.064 | 18 | 7 | 0.234 |
No | 75 | 35 | 40 | 44 | 31 | ||
Vascular tumor thrombus | |||||||
Yes | 10 | 2 | 8 | 0.033* | 3 | 7 | 0.028* |
No | 90 | 50 | 40 | 59 | 31 |
*, P<0.05, the difference was statistically substantial. NLR/PA, neutrophil-lymphocyte ratio/prealbumin ratio; CEA, carcinoembryonic antigen; METTL11B, methyltransferase-like protein 11B; NLR/PA, neutrophil lymphocyte ratio to prealbumin ratio; TNM stage, tumor (T), node (N), metastasis (M) stage; TIS, carcinoma in situ.
Results of univariate analysis
Univariate analysis showed that serum albumin, tumor size, tumor differentiation, chemotherapy, vascular tumor thrombus, NLR/PA, and METTL11B were risk factors affecting OS in patients with CRC (all P<0.05) (Table 2).
Table 2
Variable | HR | 95% CI | P value |
---|---|---|---|
Sex | |||
Male | 1.904 | 0.539–2.220 | 0.804 |
Female | 1 | ||
Age (years) | |||
<64 | 1.110 | 0.538–2.289 | 0.778 |
≥64 | 1 | ||
Body mass reduction (kg) | |||
<5 | 1.708 | 0.817–3.567 | 0.155 |
≥5 | 1 | ||
Body mass index (kg/m2) | |||
<18.5 | 1.903 | 0.841–4.310 | 0.123 |
≥18.5 | 1 | ||
CEA | |||
≤5 | 1.402 | 0.657–2.992 | 0.382 |
>5 | 1 | ||
CA199 (U/mL) | |||
<37 | 0.570 | 0.278–1.166 | 0.124 |
≥37 | 1 | ||
Serum albumin | |||
<35 | 0.235 | 0.082–0.671 | 0.007* |
≥35 | 1 | ||
Tumor size (cm) | |||
<3 | 2.810 | 1.358–5.815 | 0.005* |
≥3 | 1 | ||
Tumor differentiation | |||
Moderately or highly differentiated | 2.345 | 1.073–5.126 | 0.033* |
Poorly differentiated | 1 | ||
Tumor location | |||
Medium or high location | 0.835 | 0.404–1.725 | 0.626 |
Low location | 1 | ||
TNM stage | |||
I–II | 1.843 | 0.866–3.924 | 0.113 |
III–IV | 1 | ||
Infiltration depth | |||
Early (TIS + T1) | 0.649 | 0.318–1.326 | 0.236 |
Progress period (T2–T4) | 1 | ||
Chemotherapy | |||
Yes | 6.053 | 2.698–13.580 | 0.000* |
No | 1 | ||
Distant metastasis | |||
Yes | 5.712 | 0.777–42.001 | 0.087 |
No | 1 | ||
Lymph node metastasis | |||
Yes | 1.326 | 0.463–3.797 | 0.599 |
No | 1 | ||
Nerve invasion | |||
Yes | 1.710 | 0.699–4.186 | 0.240 |
No | 1 | ||
Vascular tumor thrombus | |||
Yes | 0.135 | 0.062–0.293 | 0.000* |
No | 1 | ||
NLR/PA | |||
Low (<0.0947) | 0.181 | 0.074–0.442 | 0.000* |
High (≥0.0947) | 1 | ||
METTL11B expression | |||
Low | 0.102 | 0.039–0.266 | 0.000* |
High | 1 |
*, P<0.05, the difference was statistically substantial. OS, overall survival; CEA, carcinoembryonic antigen; HR, hazard ratio; METTL11B, methyltransferase-like protein 11B; NLR/PA, neutrophil lymphocyte ratio to prealbumin ratio; TNM stage, tumor (T), node (N), metastasis (M) stage; TIS, carcinoma in situ.
Results of multivariate analysis
Risk factors that were statistically significant in the univariate analysis were subsequently integrated into multivariate Cox regression analysis. NLR/PA and METTL11B were found to be independent risk factors for CRC development in this multivariate analysis (P=0.044 and P=0.007, respectively), and tumor size and chemotherapy also had independent effects on CRC development (P=0.006 and P=0.02, respectively) (Table 3).
Table 3
Variable | HR | 95% CI | P |
---|---|---|---|
Tumor size (cm) | |||
<3 | 0.339 | 0.158–0.728 | 0.006* |
≥3 | 1 | ||
Chemotherapy | |||
Yes | 2.622 | 1.164–5.908 | 0.020* |
No | 1 | ||
NLR/PA | |||
Low (<0.0947) | 2.799 | 1.029–7.618 | 0.044* |
High (≥0.0947) | 1 | ||
METTL11B expression | |||
Low | 4.190 | 1.490–11.781 | 0.007* |
High | 1 |
*, P<0.05, the difference was statistically substantial. OS, overall survival; CEA, carcinoembryonic antigen; HR, hazard ratio; METTL11B, methyltransferase-like protein 11B; NLR/PA, neutrophil-lymphocyte ratio/prealbumin ratio.
The nomograms and prediction model
Based on the Cox regression analysis findings, the nomograms were drawn. The C-index for internal verification was 0.8493 (95% CI: 0.7735–0.9251), suggesting that the prediction model was accurate enough (Figure 10).
Discussion
In this study, DEGs in CRC were firstly screened from TCGA data, and the METTL11B gene was selected from among the DEGs. Further data analysis revealed that METTL11B was differentially expressed in CRC tissues and adjacent tissues in both the TCGA database and our clinical data. Moreover, it was found to be linked to the prognosis of patients with CRC. We also demonstrated that preoperative NLR/PA was associated with the prognosis of patients undergoing CRC surgery. Finally, both NLR/PA and METTL11B were found to be independent risk factors for the prognosis of CRC. The prediction model built based on our nomograms can aid clinicians to identify high-risk patients in a timely and accurate manner after surgery, allowing for timely and appropriate treatment to be provided.
Immune and nutritional status factors play a potential role in tumorigenesis, metastasis, and progression. For instance, neutrophils can interact with cancerous cells, and release chemokines and cytokines, boosting cancer growth, metastasis, and angiogenesis (30). Neutrophils have also been investigated as releasers of vascular endothelial growth factors (VEGFs)—which are critical to drug resistance, cancer angiogenesis, and metastasis—into the systemic circulation. In contrast, the function of lymphocytes is to arrest tumor onset and growth via immune surveillance and elimination of cancerous cells. Interestingly, neutrophils may work with lymphocytes in the cancer microenvironment to decrease the anticancer potential of stimulated T cells (31). Therefore, upregulation of the NLR can represent lymphocytopenia and neutrophilia, reflecting an inequity of the immune response. Furthermore, patients with malignant tumors often show symptoms of malnutrition. Serum prealbumin is a negative acute-phase protein produced by the liver that is used to assess the nutritional state of patients with cancer (32,33). It is a persistent tetramer made up of four similar subunits generated by the liver, each of which has 127 amino acids and a molecular weight of 61,000. Serum prealbumin takes its name from the fact that it migrates before albumin in electrophoresis. Prealbumin is found in tiny amounts in normal human serum and has a half-life of only 1.9 days in vivo. It aids in the delivery and control of vitamin A and thyroxine throughout the body. Serum prealbumin has thymic activity, enhances immunity by promoting lymphocyte maturation, and exhibits potent anticancer activity (34-38). Therefore, in this study, combining markers of immune status and nutritional status showed good accuracy and effectiveness for assessing the prognosis of patients with cancer.
METTL11B is a representative of the mammalian METTL family, several members of which have been found to be expressed in tumor tissues and to have prognostic relevance in cancer (39). For instance, elevated expression of METTL7B is associated with a poor prognosis in patients with non-small cell lung cancer (39). However, there have been few studies on METTL11B, and it has not yet been reported on in relation to cancer. Therefore, we researched the role of METTL11B in CRC.
This study evaluated the prognosis of patients with CRC from a multiomics perspective by combining indicators of immune status and nutritional status as well as IHC pathological information. At the beginning of the study, we screened out the CRC-related DEGs based on gene expression profiles in the TCGA database. We then identified the object molecule METTL11B as a DEG based on the conditions of |log2FC|>5 and P<0.05. Afterward, using the GEPIA and TCGA databases, we verified that METTL11B expression was elevated in CRC tissues compared to neighboring tissues. Considering that current tumor treatment is in the immunotherapy era, we explored the gene expression profiles of the retrieved samples using the established computational resource CIBERSORT, and attempted to find out whether METTL11B expression was linked to immune infiltration in CRC. Surprisingly, Th17 cells, Th1 cells, Tgd cells, Tfhs, TEMs, TCNs, CD56bright NK cells, T helper cells, neutrophils, mast cells, NK cells, macrophages, IDCs, eosinophils, and DCs were the important immune cells influenced by METTL11B expression (all P<0.001). Interestingly, METTL11B was found to interact with MSI-related molecules (PMS1, MSH6, PMS2, MLH1, and MLH3), and their expressions were correlated. This finding is consistent with the current treatment guidelines on CRC, which state that the molecular etiology of MSI-high tumors leads to their strong sensitivity to immunotherapy, and also shows that METTL11B not only has good scientific significance but also good clinical value. Through further GSEA, we found that METTL11B may influence or participate in the biological events of CRC by affecting signaling pathways such as the CALCIUM_SIGNALING_PATHWAY, PATHWAYS_IN_CANCER, REACTOME_PLATELET_ACTIVATION_SIGNALING_AND_AGGREGATION, and WP_PI3KAKT_SIGNALING. These predicted signaling pathways may serve as a reference for further basic research in the future. In the IHC analysis of tissues from the patients included in our study, METTL11B expression was elevated in CRC samples compared to para-cancer samples, which was consistent with the expression level observed in the TCGA database. Cox multivariate analysis showed that METTL11B and NLR/PA were independent risk factors for cancer growth (P=0.044 and P=0.007, respectively), and tumor size and chemotherapy also had independent effects on cancer growth (P=0.006 and P=0.02, respectively). After 3 years of follow-up, 69 (69.0%) patients in the study were still alive and 31 (31.0%) patients had died. The OS and PFS of patients in the low NLR/PA group and low METTL11B expression group were significantly greater than those in the high NLR/PA group and high METTL11B expression group, respectively. These results reflect the positive significance of choosing a combined indicator in our study. To provide a better reference for the management of patients with CRC at the clinical level, we created a nomogram based on the findings of Cox regression analysis. During internal verification, the nomogram had a C-index of 0.8493 (95% CI: 0.7735–0.9251), and exhibited strong prognostic reliability and an accurate performance in predicting 1-, 3-, and 5-year survival. Our nomogram has some practical potential for the advancement of clinical treatment.
This study also has some limitations, such as its small sample size. Also, because this is a retrospective study, there is a risk that bias existed in the data selection and analysis. However, despite these limitations, this is the first study to demonstrate the significance of combining preoperative NLR/PA with METTL11B for the postoperative prognostic evaluation of patients harboring CRC, and its findings have positive potential for the implementation of clinical work.
Conclusions
The data of this study clearly show that high NLR/PA and high METTL11B significantly reduce the OS of patients with CRC. Further large-sample prospective investigations involving multiple centers are required to explain the precise mechanisms of action of NLR/PA and METTL11B in CRC.
Acknowledgments
Funding: None.
Footnote
Reporting Checklist: The authors have completed the REMARK reporting checklist. Available at https://dx.doi.org/10.21037/jgo-21-781
Data Sharing Statement: Available at https://dx.doi.org/10.21037/jgo-21-781
Conflicts of Interest: All authors have completed the ICMJE uniform disclosure form (available at https://dx.doi.org/10.21037/jgo-21-781). The authors have no conflicts of interest to declare.
Ethical Statement: The authors are accountable for all aspects of the work in ensuring that questions related to the accuracy or integrity of any part of the work are appropriately investigated and resolved. All procedures performed in this study involving human participants were in accordance with the Declaration of Helsinki (as revised in 2013). The study was approved by Jiangsu Cancer Hospital’s ethics committee (No. 2016-062-06), and all samples were obtained with signed informed consent from the participants.
Open Access Statement: This is an Open Access article distributed in accordance with the Creative Commons Attribution-NonCommercial-NoDerivs 4.0 International License (CC BY-NC-ND 4.0), which permits the non-commercial replication and distribution of the article with the strict proviso that no changes or edits are made and the original work is properly cited (including links to both the formal publication through the relevant DOI and the license). See: https://creativecommons.org/licenses/by-nc-nd/4.0/.
References
- Kuipers EJ, Spaander MC. Personalized screening for colorectal cancer. Nat Rev Gastroenterol Hepatol 2018;15:391-2. [Crossref] [PubMed]
- Schwingshackl L, Schwedhelm C, Hoffmann G, et al. Food groups and risk of colorectal cancer. Int J Cancer 2018;142:1748-58. [Crossref] [PubMed]
- Sung H, Ferlay J, Siegel RL, et al. Global Cancer Statistics 2020: GLOBOCAN Estimates of Incidence and Mortality Worldwide for 36 Cancers in 185 Countries. CA Cancer J Clin 2021;71:209-49. [Crossref] [PubMed]
- Fisseler-Eckhoff A. New TNM classification of malignant lung tumors 2009 from a pathology perspective. Pathologe 2009;30:193-9. [Crossref] [PubMed]
- Provinciali M, Barucca A, Cardelli M, et al. Inflammation, aging, and cancer vaccines. Biogerontology 2010;11:615-26. [Crossref] [PubMed]
- Rodriguez-Vita J, Lawrence T. The resolution of inflammation and cancer. Cytokine Growth Factor Rev 2010;21:61-5. [Crossref] [PubMed]
- Read SA, Douglas MW. Virus induced inflammation and cancer development. Cancer Lett 2014;345:174-81. [Crossref] [PubMed]
- Xiao L, Li L, Chen G, et al. The lymphocyte-to-monocyte ratio could predict the efficacy of PD-1 inhibitors in patients with advanced cancer. Transl Cancer Res 2020;9:4111-20. [Crossref]
- Sunakawa Y, Yang D, Cao S, et al. Immune-related Genes to Dominate Neutrophil-lymphocyte Ratio (NLR) Associated With Survival of Cetuximab Treatment in Metastatic Colorectal Cancer. Clin Colorectal Cancer 2018;17:e741-9. [Crossref] [PubMed]
- Mezquita L, Preeshagul I, Auclin E, et al. Predicting immunotherapy outcomes under therapy in patients with advanced NSCLC using dNLR and its early dynamics. Eur J Cancer 2021;151:211-20. [Crossref] [PubMed]
- Chen S, Han Y, Ouyang Q, et al. Randomized and dose-escalation trials of recombinant human serum albumin /granulocyte colony-stimulating factor in patients with breast cancer receiving anthracycline-containing chemotherapy. BMC Cancer 2021;21:341. [Crossref] [PubMed]
- Cai W, Kong W, Dong B, et al. Pretreatment Serum Prealbumin as an Independent Prognostic Indicator in Patients With Metastatic Renal Cell Carcinoma Using Tyrosine Kinase Inhibitors as First-Line Target Therapy. Clin Genitourin Cancer 2017;15:e437-46. [Crossref] [PubMed]
- Lv Y, Zhang J, Liu Z, et al. A novel inflammation-based prognostic index for patients with esophageal squamous cell carcinoma: Neutrophil lymphocyte ratio/prealbumin ratio. Medicine (Baltimore) 2019;98:e14562. [Crossref] [PubMed]
- Robert MF, Morin S, Beaulieu N, et al. DNMT1 is required to maintain CpG methylation and aberrant gene silencing in human cancer cells. Nat Genet 2003;33:61-5. [Crossref] [PubMed]
- Han J, Wang JZ, Yang X, et al. METTL3 promote tumor proliferation of bladder cancer by accelerating pri-miR221/222 maturation in m6A-dependent manner. Mol Cancer 2019;18:110. [Crossref] [PubMed]
- Gu C, Wang Z, Zhou N, et al. Mettl14 inhibits bladder TIC self-renewal and bladder tumorigenesis through N6-methyladenosine of Notch1. Mol Cancer 2019;18:168. [Crossref] [PubMed]
- Chen X, Li A, Sun BF, et al. 5-methylcytosine promotes pathogenesis of bladder cancer through stabilizing mRNAs. Nat Cell Biol 2019;21:978-90. [Crossref] [PubMed]
- Broche J, Kungulovski G, Bashtrykov P, et al. Genome-wide investigation of the dynamic changes of epigenome modifications after global DNA methylation editing. Nucleic Acids Res 2021;49:158-76. [Crossref] [PubMed]
- Niu C, Wang S, Guo J, et al. BACH1 recruits NANOG and histone H3 lysine 4 methyltransferase MLL/SET1 complexes to regulate enhancer-promoter activity and maintains pluripotency. Nucleic Acids Res 2021;49:1972-86. [Crossref] [PubMed]
- Vallot C, Hérault A, Boyle S, et al. PRC2-independent chromatin compaction and transcriptional repression in cancer. Oncogene 2015;34:741-51. [Crossref] [PubMed]
- Kraus D, Yang Q, Kong D, et al. Nicotinamide N-methyltransferase knockdown protects against diet-induced obesity. Nature 2014;508:258-62. [Crossref] [PubMed]
- Liu F, Zhao X, Perna F, et al. JAK2V617F-mediated phosphorylation of PRMT5 downregulates its methyltransferase activity and promotes myeloproliferation. Cancer Cell 2011;19:283-94. [Crossref] [PubMed]
- Wang Y, Zheng T. Screening of hub genes and pathways in colorectal cancer with microarray technology. Pathol Oncol Res 2014;20:611-8. [Crossref] [PubMed]
- Gentles AJ, Newman AM, Liu CL, et al. The prognostic landscape of genes and infiltrating immune cells across human cancers. Nat Med 2015;21:938-45. [Crossref] [PubMed]
- Ali HR, Chlon L, Pharoah PD, et al. Patterns of Immune Infiltration in Breast Cancer and Their Clinical Implications: A Gene-Expression-Based Retrospective Study. PLoS Med 2016;13:e1002194. [Crossref] [PubMed]
- Bense RD, Sotiriou C, Piccart-Gebhart MJ, et al. Relevance of Tumor-Infiltrating Immune Cell Composition and Functionality for Disease Outcome in Breast Cancer. J Natl Cancer Inst 2016;109:djw192. [Crossref] [PubMed]
- Davis S, Meltzer PS. GEOquery: a bridge between the Gene Expression Omnibus (GEO) and BioConductor. Bioinformatics 2007;23:1846-7. [Crossref] [PubMed]
- Diboun I, Wernisch L, Orengo CA, et al. Microarray analysis after RNA amplification can detect pronounced differences in gene expression using limma. BMC Genomics 2006;7:252. [Crossref] [PubMed]
- Gu Z, Eils R, Schlesner M. Complex heatmaps reveal patterns and correlations in multidimensional genomic data. Bioinformatics 2016;32:2847-9. [Crossref] [PubMed]
- Bindea G, Mlecnik B, Fridman WH, et al. Natural immunity to cancer in humans. Curr Opin Immunol 2010;22:215-22. [Crossref] [PubMed]
- Hanahan D, Weinberg RA. Hallmarks of cancer: the next generation. Cell 2011;144:646-74. [Crossref] [PubMed]
- Lee JL, Oh ES, Lee RW, et al. Serum Albumin and Prealbumin in Calorically Restricted, Nondiseased Individuals: A Systematic Review. Am J Med 2015;128:1023.e1-22. [Crossref] [PubMed]
- Liu X, Sun X, Liu J, et al. Preoperative C-Reactive Protein/Albumin Ratio Predicts Prognosis of Patients after Curative Resection for Gastric Cancer. Transl Oncol 2015;8:339-45. [Crossref] [PubMed]
- Measurement of visceral protein status in assessing protein and energy malnutrition: standard of care. Prealbumin in Nutritional Care Consensus Group. Nutrition 1995;11:169-71. [PubMed]
- Jagoe RT, Goodship TH, Gibson GJ. Nutritional status of patients undergoing lung cancer operations. Ann Thorac Surg 2001;71:929-35. [Crossref] [PubMed]
- Beck FK, Rosenthal TC. Prealbumin: a marker for nutritional evaluation. Am Fam Physician 2002;65:1575-8. [PubMed]
- Moghazy AM, Adly OA, Abbas AH, et al. Assessment of the relation between prealbumin serum level and healing of skin-grafted burn wounds. Burns 2010;36:495-500. [Crossref] [PubMed]
- Miura K, Hamanaka K, Koizumi T, et al. Clinical significance of preoperative serum albumin level for prognosis in surgically resected patients with non-small cell lung cancer: Comparative study of normal lung, emphysema, and pulmonary fibrosis. Lung Cancer 2017;111:88-95. [Crossref] [PubMed]
- Liu D, Li W, Zhong F, et al. METTL7B Is Required for Cancer Cell Proliferation and Tumorigenesis in Non-Small Cell Lung Cancer. Front Pharmacol 2020;11:178. [Crossref] [PubMed]
(English Language Editor: B. Meiser and J. Reylonds)