Tumor mutation burden determined by a 645-cancer gene panel and compared with microsatellite instability and mismatch repair genes in colorectal cancer
Introduction
Colorectal cancer (CRC) is the fourth leading cause of cancer-related death in China (1-3). With the improvement of surgical methods and the combination of chemotherapy drugs and other medical technology, the treatment level of CRC has improved, while the overall prognosis of patients has not significantly improved. Over the past 2 decades, many drugs, including targeted drugs such as antibodies targeting vascular endothelial growth factor (bevacizumab) and the epidermal growth factor receptor (EGFR; cetuximab and panitumumab), and immunotherapy drugs have been approved for the treatment of metastatic CRC (mCRC). The survival period of advanced CRC patients has increased from less than 1 to 3 years, and even 20% of patients can survive for more than 5 years (4-7). However, the optimal combination of these drugs is likely dependent on many factors, including the mutational status of the tumor cells. With the continuous development of genome sequencing, targeted therapy and immunotherapy for CRC have made great progress in recent years. Scientists have found several groups of biomarkers such as gene mutations (KRAS, NRAS, BRAF, HER2, NTRK), tumor mutation burden (TMB), and microsatellite instability/mismatch repair (MSI/MMR) which can be used as prognostic indicators of targeted therapy and immunotherapy (8-14).
MSI are DNA elements composed of repeating motifs that occur as alleles of variable lengths. It was first found in hereditary non-polyposis colorectal cancer (HNPCC) (15), and was then identified in a variety of sporadic tumors (such as gastric cancer, lung cancer, and endometrial cancer) (16). MSI has been associated with improved prognosis and immune checkpoint inhibitors (ICIs). Some evidence has shown that MSI-high (MSI-H) mCRC patients who received nivolumab and nivolumab + ipilimumab had a better response rate and survival time (17,18). MSI increases the probability of somatic mutation. The incidence of somatic mutation was 10–50 times higher than that of MMR proficiency (pMMR) (19). As the increase of mutation frequency would lead to the enhancement of tumor immunogenicity (20), patients with MMR deficiency (dMMR) had higher sensitivity to immunotherapy. Recent studies suggest that dMMR may also be a marker for predicting the efficacy of immunotherapy (21). Solid tumor patients with MSI-H/dMMR usually develop immunogenicity and extensive T-cell infiltration, which results in a high response to ICI treatment. These findings indicate that MSI/MMR gene deletion may predict the efficacy of immunotherapy, however, the incidence of dMMR/MSI-H in CRC is only about 10–15% (22). Therefore, more molecular markers are needed to predict the efficacy of immunotherapy.
TMB measured by whole-exome sequencing (WES) is a novel prognostic biomarker for ICI therapy in cancers (12,23). However, TMB is difficult to popularize because of the cost, timeliness, and bioinformatics challenges of WES in the clinical setting (24,25).
Hence, in this study, 645 cancer-related genes and 5 MSI loci (BAT-25, BAT-26, NR-21, NR-24, MONO-27) were obtained for developing a panel for TMB estimation (panel-TMB) and predicting the efficacy of targeted therapy and immunotherapy in CRC. In this study, somatic and genetic mutations of patients were detected in the same experimental species, and TMB, HRR, MMR and MSI of patients were analyzed at the same time, which could provide patients with a better comprehensive treatment plan including targeted drugs, genetic and immunotherapy. The correlation between TMB, MMR and MSI was also analyzed, and multiple tests were combined in one experiment to shorten the detection cycle and cost. In addition, this study clinical samples of the department and TCGA database were comprehensively analyzed to verify the accuracy of the process and shorten the overall smooth testing development cycle. We present the following article in accordance with the REMARK reporting checklist (available at https://dx.doi.org/10.21037/jgo-21-572).
Methods
Patient samples
A total of 41 tumor biopsies and whole blood samples were collected from newly diagnosed patients at Jiangsu Cancer Hospital (JCH) between November 29, 2017 and March 18, 2020 for targeted sequencing using the 645 cancer gene panel (GP645). All procedures performed in this study involving human participants were in accordance with the Declaration of Helsinki (as revised in 2013). The study was approved by the Ethics Committee of Jiangsu Cancer Hospital (No. 2016-062-06). All participants provided written informed consent.
Library construction
Genomic DNA was extracted from tumor biopsies and whole blood. Libraries were constructed by the KAPA Hyper DNA Library Prep Kit (KAPA Biosystem). Finally, dual-index libraries were cleaned up with purification beads (AxyPrep Fragment Select-I kit, Corning). The concentration and quality of these libraries were measured using the Qubit 3.0 system (Invitrogen) and Bioanalyzer 2100 (Agilent HS DNA Reagent, Agilent), respectively.
Hybrid selection and ultra-deep next-generation sequencing (NGS)
5'-biotinylated probes for targeted sequencing covered exons, selected introns, MSI, MMR-related genes, and genetic genes in the 645 cancer-related genes, and were designed and synthesized by the Targetseq Enrichment Kit (Gensmile and iGeneTech, China) (Table S1) in a cohort of 41 patients. These libraries were hybridized to the GP645 to capture targeted fragments according to the manufacturer's protocol. Then, these fragments were sequenced by the NovaSeq 6000 System (Illumina, USA), and the depth of sequencing was 1,000× for germline mutations and 5,000× for somatic mutations.
Acquisition of mutation data
The Cancer Genome Atlas (TCGA) database of CRC was obtained from the Genomic Data Commons (GDC) website (https://portal.gdc.cancer.gov/) using the University of California Santa Cruz (UCSC) Xena platform (https://xenabrowser.net/datapages/) (26), including CRC mutation data and clinical information, such as age, sex, AJCC-TNM stages, pathological stages, tumor stages, and survival outcomes. The statistical results of somatic mutations were visualized with the maftools software.
Correlation analysis between TMB and overall survival (OS)
First, we screened the TCGA dataset for dbSNP and ExAC-annotated germline mutations using GP645. Meanwhile, we filtered out the germline mutations via blood cell mutations for the JCH dataset using the GP645. Then, we calculated the panel-TMB for each sample as the total amount of coding variants/exons length (38 million) based on the number of non-synonymous somatic mutations, including frameshift deletion mutation, in-frame deletion mutation, frameshift insertion mutation, in-frame insertion mutation, missense mutation, nonsense mutation, nonstop mutation, and silent mutation. Using median as the threshold, patients were divided into high TMB group and low TMB group (27).
MSI status determined by NGS
Five microsatellite loci (BAT40, BAT26, BAT25, NR27, NR21) were used to identify MSI in the GP645. The number of microsatellite loci was counted for each of the JCH patients. Only insertions or deletions that increased or decreased the number of repeats were considered. Samples with 2 or more MSIs were identified as MSI-H, samples with one MSI were classified as MSI-low (MSI-L), and samples without MSI were classified as microsatellite stable (MSS). In the outcome analysis, MSI-L samples were grouped with MSS tumors. We further identified MMR status by functional loss mutation of MLH1, MSH2, MSH6, PMS1, PMS2, MSH3, and MLH3.
Statistical analyses
All statistical analyses were performed using R software (Version 3.5.2). The Benjamini-Hochberg method was used to conduct multiple test adjustments for P values based on false discovery rate (FDR), and P value <0.05 was considered statistically significant. Differential analysis and normalization were mainly carried out using the “limma” package of R software (version 3.5.2). Kaplan-Meier analysis with the log-rank test or Cox regression model was performed using the “survival” package. Student’s t-test was used for continuous variables, while χ2 test was used for categorical variables.
Results
The mutation profiling of the GP645 in CRC
The somatic and germline mutation data of CRC patients from the JCH and TCGA datasets were processed as shown in Figure 1 and their clinical information is presented in Table 1. The mean age was 58.32 years, and 12 (29.3%) women and 29 (70.7%) men were included. Utilizing maftools software, we classified these mutations into various groups and depicted mutation groups in box plots using various colors (Figure 1). We compared the mutation profiling of the JCH and TCGA datasets using the GP645 developed by us and found that the most common type was missense mutation (Figure 1). Single nucleotide polymorphism occurred more frequently than deletion or insertion (INS) (Figure 1), and C>T transition was the most common form of single nucleotide variants in both the JCH and TCGA datasets (Figure 1). The mutation categories are shown in box plots. We further found that the mutation frequencies of APC, TP53, KRAS, PIK3CA, LRP1B, FAT3, FBXW7, ATM, KMT2D, SMAD4, SOX9, BRAF, SPTA1, AMER1, FAT1, ARID1A, ZFHX3, KMT2B, DYNC2H1, and PTPRT (Figure 1) were greater than 10% in both the JCH and TCGA datasets. Besides, the GP645 genes were distributed on 21 chromosomes (Figure S1). The co-occurrences and exclusive associations between mutated genes of the JCH and TCGA databases are shown in Figure 2A (TCGA dataset) and Figure 2B (JCH dataset).
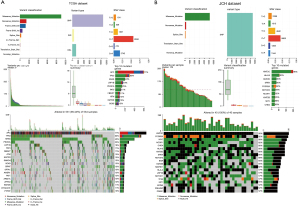
Table 1
Level | JCH dataset | TCGA dataset |
---|---|---|
N | 41 | 629 |
Age [median (IQR)] | 58.32 | 61.00 |
Gender (%) | ||
Female | 12 (29.3) | 335 (53.3) |
Male | 29 (70.7) | 294 (46.7) |
Status (%) | ||
Alive | NA | 473 (75.2) |
Dead | NA | 124 (19.7) |
Not reported | NA | 32 (5.0) |
Pathologic_T (%) | ||
T1 | 0 (0) | 20 (3.2) |
T2 | 3 (7.3) | 109 (17.3) |
T3 | 11 (26.8) | 427 (67.9) |
T4 | 10 (24.3) | 70 (10.6) |
TX | 17 (43.4) | 1 (0.2) |
Pathologic_N (%) | ||
N0 | 9 (21.9) | 356 (56.6) |
N1 | 10 (24.3) | 151 (24.0) |
N2 | 4 (9.7) | NA |
NX | 18 (43.9) | 118 (18.6) |
Pathologic_M (%) | ||
M0 | 12 (29.2) | 466 (74.1) |
M1 | 15 (36.5) | 75 (11.9) |
M | 1 (2.4) | NA |
MX | 0 (31.7) | 64 (10.2) |
Pathologic_stage (%) | ||
Stage I | NA | 109 (17.3) |
Stage II | NA | 229 (36.4) |
Stage III | NA | 181 (28.8) |
Stage IV | NA | 90 (14.3) |
CRC, colorectal cancer; JCH, Jiangsu Cancer Hospital; TCGA, The Cancer Genome Atlas.
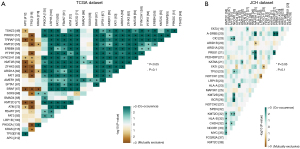
Next, the pathways of the GP645 genes were investigated in both the JCH and TCGA datasets. As shown Figure 3, the genes in the GP645 were involved in 10 pathways in both the JCH and TCGA databases, including RTK-RAS, PI3K, cell cycle, NOTCH, WNT, Hippo, TGF-Beta, MYC, TP53, and NRF2, and, respectively, the number of genes with mutations in each category was 46, 20, 13, 12, 10, 7, 6, 6, 5, and 3 in TCGA dataset (Figure 3A) and the number of samples with gene mutations in each category was 401, 236, 49, 172, 474, 147, 145, 66, 366, and 23 in JCH dataset (Figure 3C). Meanwhile, the number of genes in each pathway was 45, 18, 12, 11, 10, 5, 6, 5, 4, and 2 in the JCH dataset (Figure 3B), and the number of samples with gene mutations in each category was 40, 36, 26, 40, 35, 33, 16, 35, 29, and 11 in TCGA dataset respectively (Figure 3D). These results suggested that the GP645 genes are primarily involved in important processes in tumor progression.
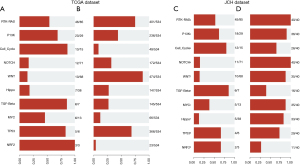
The relationship between the panel‑TMB database and TMB estimated by TCGA database
To evaluate whether the panel-TMB could reflect TMB estimated by WES (wTMB), we calculated the number of TMB per million bases for 531 CRC patients in TCGA dataset and analyzed the correlation between panel-TMB and wTMB. Non-synonymous mutations (NsMs) derived from WES and the GP645 were relatively consistent in CRC (Figure 4A). Furthermore, panel-TMB and wTMB had a significant positive correlation (R=0.75, P<0.001, 95% CI: 0.75 to 0.82, Figure 4B). These results suggested that the panel-TMB of the GP645 could represent wTMB and might be a potential predictor of prognostic stratification for CRC patients.
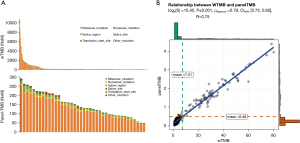
Higher TMB estimated by the panel-TMB is associated with improved OS
We determined the median value as the threshold for panel-TMB to assess the impact of panel-TMB on the OS of CRC. Kaplan-Meier survival analysis indicated that patients with a higher panel-TMB had improved OS in TCGA dataset (P=0.0062) (Figure 5A). Moreover, the low panel-TMB group had a longer 3-year restricted mean survival time (RMST) than the high panel-TMB group in TCGA dataset [2,944.97 (95% CI: 2,574 to 3,315) vs. 2,315.8 (95% CI: 1,926 to 2,705) days] (Figure 5B). Unfortunately, there was not enough clinical data to analyze the survival curve of panel-TMB in the JCH dataset.
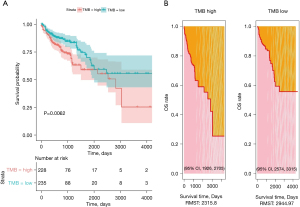
Panel-TMB subgroup analysis in the JCH dataset
We calculated the number of TMB per million bases for 41 samples of the JCH dataset and classified them into high-TMB and low-TMB groups (Table 2, Figure 6), and also classified MSI status and MMR gene mutations for each of the 41 samples. A total of 15 patients (34.2%) were classified into the high-TMB group, and 26 (65.7%) were classified into the low-TMB group (Figure 6). Only 3 patients (7.3%) were identified as having dMMR, and the 3 patients (7.3%) were also classified as MSI-H (Figure 6). The proportion of patients with pMMR was about 92.6% (Figure 6). Moreover, we analyzed the MMR and MSI of the high-TMB and low-TMB groups in the JCH dataset. We found that 3 patients were identified as MSI-H and dMMR, and 2 of them had a high TMB value (Table 2). Furthermore, dMMR status was identified in 3 cases (7.3%) (Table 3), while MSI-H was identified in the same patients (7.3%), and high-TMB was identified in 15 cases (34.2%).
Table 2
Sample | TMB | TMB-group | MSI | MMR |
---|---|---|---|---|
GS645-171130-01 | 6.9 | L | MSS | pMMR |
GS645-171214-02 | 2.3 | L | MSS | pMMR |
GS645-171226-01 | 0.26 | L | MSS | pMMR |
GS645-180131-01 | 5.38 | L | MSI-H | dMMR |
GS645-180319-04 | 5.38 | L | MSI-L | pMMR |
GS645-180428-01 | 3.85 | L | MSS | pMMR |
GS645-180606-01 | 4.62 | L | MSS | pMMR |
GS645-180621-03 | 13.85 | H | MSS | pMMR |
GS645-180621-01 | 8.46 | L | MSI-L | pMMR |
GS645-180711-03 | 11.54 | H | MSS | pMMR |
GS645-180711-03 | 10 | H | MSS | pMMR |
GS645-180716-02 | 5.38 | L | MSS | pMMR |
GS645-180815-01 | 11.54 | H | MSS | pMMR |
GS645-180912-02 | 8.92 | L | MSS | pMMR |
GS645-181019-01 | 4.12 | L | MSS | pMMR |
GS645-181107-02 | 25.81 | H | MSS | pMMR |
GS645-181112-02 | 5.62 | L | MSS | pMMR |
GS645-181128-02 | 72.76 | H | MSI-H | dMMR |
GS645-181229-02 | 2.75 | L | MSS | pMMR |
GS645-190107-02 | 3.43 | L | MSS | pMMR |
GS645-190107-07 | 1.42 | L | MSS | pMMR |
GS645-190117-02 | 22.65 | H | MSS | pMMR |
GS645-190129-04 | 24.02 | H | MSI-L | pMMR |
GS645-190214-01 | 6.18 | L | MSS | pMMR |
GS645-190228-03 | 5.49 | L | MSS | pMMR |
GS645-190304-03 | 4.8 | L | MSS | pMMR |
GS645-190304-04 | 23.34 | H | MSS | pMMR |
GS645-190313-03 | 10.13 | H | MSI-L | pMMR |
GS645-190319-04 | 12.76 | H | MSS | pMMR |
GS645-190322-05 | 2.06 | L | MSS | pMMR |
GS645-190329-03 | 30.2 | H | MSS | pMMR |
GS645-190404-05 | 4.12 | L | MSS | pMMR |
GS645-190408-03 | 5.49 | L | MSS | pMMR |
GS645-190412-05 | 4.8 | L | MSS | pMMR |
GS645-190426-03 | 6.29 | L | MSS | pMMR |
GS645-190428-02 | 12.76 | H | MSS | pMMR |
GS645-190620-01 | 5.49 | L | MSS | pMMR |
GS645-190628-04 | 4.12 | L | MSS | pMMR |
GS645-190729-05 | 85.8 | H | MSI-H | dMMR |
GS645-200224-04 | 10.13 | H | MSS | pMMR |
GS645-200318-07 | 8.81 | L | MSS | pMMR |
TMB, tumor mutation burden; MSI, microsatellite instability; MMR, mismatch repair; JCH, Jiangsu Cancer Hospital; L, low-TMB group; H, high-TMB group; MSI-H, high-MSI group; MSI-L, low-MSI group; MMS, MSI-stability group; pMMR, mismatch repair proficiency; dMMR, mismatch repair deficiency.
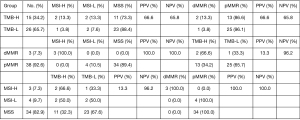
Table 3
Sample | Somatic mutation | Germline mutation | ||||
---|---|---|---|---|---|---|
Gene | Loci | Gene | Loci | Frequency (%) | ||
GS645-180131-01 | MSH6 | p.y394 | MLH3 | p.N932Y | 18.9 | |
GS645-181128-02 | MSH6 | p.R1068* | MSH3 | p.K383Rfs*32 | 27.5 | |
GS645-190729-05 | NA | NA | MSH3 | p.K383Rfs*32 | 11.6 |
MMR, mismatch repair.
Compared with MMR cases, MSI had a positive predictive value (PPV) of 100.0% and a negative predictive value (NPV) of 100.0%, and TMB had a PPV of 13.6% and an NPV of 96.2%. Compared with TMB, MSI had a PPV of 66.6% and an NPV of 65.8%, and MMR had a PPV of 66.6% and an NPV of 65.8%. Compared with MSI cases, TMB had a PPV of 13.6% and an NPV of 96.2%, and MMR had a PPV of 100.0% and an NPV of 100.0%. These results showed that patients with dMMR were associated with MSI-H, and patients with low-TMB were associated with pMMR and MSS. Meanwhile, patients with high-TMB were not associated with MSI status and MMR status.
Discussion
To established a prognostic system for cancer patients, cancer-related genes have been used to develop cancer panels in lung cancer (28), malignant lymphoma (29), melanoma (30), gastric cancer (31), and other cancers (32). In non-small cell lung cancer (27), TMB quantified by a gene panel was significantly correlated with WES results (P=0.81), and panel/WES TMB could effectively predict the efficacy of immunotherapy in the high-TMB population. Meanwhile, using a cancer panel, the dynamic monitoring of ctDNA could indicate the efficacy of immunotherapy for gastric cancer, and showed potential clinical value in the analysis of drug resistance mechanisms and the prediction of immune-related side effects (31). In this study, to construct a prediction system for Chinese CRC patients, we also developed a 2.1-Mb GP645 which includes 5 MSI loci, 7 MMR genes, and 645 cancer-related genes distributed on 21 chromosomes. We found a positive correlation between the panel-TMB and the wTMB. These results suggest that the panel-TMB measured by the GP645 is an accurate and clinically available tool for measuring TMB and represents the genomic instability in CRC patients, and can replace wTMB in evaluating prognosis. These results are in accordance with those in non-small cell lung cancer (33).
Furthermore, we performed a survival analysis of the panel-TMB measured by the GP645 using TCGA database and found that high-TMB patients were strongly associated with poor OS in CRC. Previous studies confirmed that TMB measured by a cancer-related gene panel (CRGP) could be used for prognosis and to predict the benefits of immunotherapy (30-32). Thus, these findings indicated that higher panel-TMB might be an adverse prognostic factor for CRC. However, the present study accounted for less than 40% of cases with high TMB in the JCH dataset.
Understanding genomic instability is also important to carcinogenesis and progression. MSI status has clear guiding significance for CRC patients of different stages. In addition, among CRC patients in China, the incidence of MSI-H/dMMR in right colon cancer is 20.5%, 9.2% in left colon cancer, and 5.1% in rectal cancer. Therefore, MSI/MMR should be tested for left/right colon cancer and rectal cancer (34). Both domestic and international guidelines and consensus recommend that all CRC patients be tested for MMR or MSI. This information is of great significance for patient prognosis, drug efficacy prediction and lynch syndrome screening (35). dMMR/MSI-H is an important molecular marker guiding immunotherapy in advanced patients. For early resectable CRC patients, dMMR/MSI-H patients generally have a good prognosis, but are less likely to benefit from 5-FU-based adjuvant chemotherapy (36). We identified MSI status and MMR genes which are markers of genomic instability to establish a prognostic system in CRC. Solid tumors with MSI-H/dMMR are usually immunogenic and have extensive T-cell infiltration, and are highly responsive to ICIs. Patients with MSI-H benefited from bevacizumab, while only 5% of mCRC patients with MSI-H benefited from ICIs (8,37). The NICHE clinical trial showed that patients with dMMR benefited from ICIs for early-stage colon cancers, and that neoadjuvant immunotherapy may be a potential defined standard for treating CRC patients (38). In this study, the proportions of MSI-H (7.3%) and dMMR (7.3%) accounted for less than 10% of CRC, and the vast majority of MSI-H/dMMR samples also had high TMB (PPV =66.6%). However, the converse was not true, as only 13.3% (PPV =13.3%) of samples with high TMB were classified as MSI-high/dMMR. In addition, patients with low-TMB were associated with MSS/pMMR (96.2%), and these results are consistent with earlier studies (8,32,37).
In summary, we analyzed TMB, MSI/MMR, and gene mutations and found that these biomarkers for clinical detection can provide new classifications for precision medicine in CRC, predict the prognosis of patients with CRC, and improve treatment methods to improve the survival rate of patients with CRC. The panel-TMB measured by the GP645 targeting ~2.1 Mb of MSI loci, MMR genes, and cancer-related genes could replace wTMB, and higher panel-TMB is associated with poor OS. MSI-H/dMMR and high-TMB was fairly common but MSI-high was very uncommon in CRC. Panel-TMB and MSI/MMR might be potential prognostic indexes in Chinese CRC patients.
Acknowledgments
Funding: This study was supported by Medical Research Project of Jiangsu Provincial Health Commission in 2021 (No. Z2021056).
Footnote
Reporting Checklist: The authors have completed the REMARK reporting checklist. Available at https://dx.doi.org/10.21037/jgo-21-572
Data Sharing Statement: Available at https://dx.doi.org/10.21037/jgo-21-572
Conflicts of Interest: All authors have completed the ICMJE uniform disclosure form (available at https://dx.doi.org/10.21037/jgo-21-572). The authors have no conflicts of interest to declare.
Ethical Statement: The authors are accountable for all aspects of the work in ensuring that questions related to the accuracy or integrity of any part of the work are appropriately investigated and resolved. All procedures performed in this study involving human participants were in accordance with the Declaration of Helsinki (as revised in 2013). The study was approved by the Ethics Committee of Jiangsu Cancer Hospital (No. 2016-062-06). All participants provided written informed consent.
Open Access Statement: This is an Open Access article distributed in accordance with the Creative Commons Attribution-NonCommercial-NoDerivs 4.0 International License (CC BY-NC-ND 4.0), which permits the non-commercial replication and distribution of the article with the strict proviso that no changes or edits are made and the original work is properly cited (including links to both the formal publication through the relevant DOI and the license). See: https://creativecommons.org/licenses/by-nc-nd/4.0/.
References
- Siegel RL, Miller KD, Jemal A. Cancer statistics, 2015. CA Cancer J Clin 2015;65:5-29. [Crossref] [PubMed]
- Chen W, Zheng R, Baade PD, et al. Cancer statistics in China, 2015. CA Cancer J Clin 2016;66:115-32. [Crossref] [PubMed]
- Bray F, Ferlay J, Soerjomataram I, et al. Global cancer statistics 2018: GLOBOCAN estimates of incidence and mortality worldwide for 36 cancers in 185 countries. CA Cancer J Clin 2018;68:394-424. [Crossref] [PubMed]
- Yao JC, Phan A, Hoff PM, et al. Targeting vascular endothelial growth factor in advanced carcinoid tumor: a random assignment phase II study of depot octreotide with bevacizumab and pegylated interferon alpha-2b. J Clin Oncol 2008;26:1316-23. [Crossref] [PubMed]
- Rawla P, Barsouk A, Hadjinicolaou AV, et al. Immunotherapies and Targeted Therapies in the Treatment of Metastatic Colorectal Cancer. Med Sci (Basel) 2019;7:83. [Crossref] [PubMed]
- Tol J, Punt CJ. Monoclonal antibodies in the treatment of metastatic colorectal cancer: a review. Clin Ther 2010;32:437-53. [Crossref] [PubMed]
- Feng QY, Wei Y, Chen JW, et al. Anti-EGFR and anti-VEGF agents: important targeted therapies of colorectal liver metastases. World J Gastroenterol 2014;20:4263-75. [Crossref] [PubMed]
- Innocenti F, Ou FS, Qu X, et al. Mutational Analysis of Patients With Colorectal Cancer in CALGB/SWOG 80405 Identifies New Roles of Microsatellite Instability and Tumor Mutational Burden for Patient Outcome. J Clin Oncol 2019;37:1217-27. [Crossref] [PubMed]
- De Roock W, Claes B, Bernasconi D, et al. Effects of KRAS, BRAF, NRAS, and PIK3CA mutations on the efficacy of cetuximab plus chemotherapy in chemotherapy-refractory metastatic colorectal cancer: a retrospective consortium analysis. Lancet Oncol 2010;11:753-62. [Crossref] [PubMed]
- Taieb J, Le Malicot K, Shi Q, et al. Prognostic Value of BRAF and KRAS Mutations in MSI and MSS Stage III Colon Cancer. J Natl Cancer Inst 2016;109:djw272. [Crossref] [PubMed]
- Price TJ, Hardingham JE, Lee CK, et al. Impact of KRAS and BRAF Gene Mutation Status on Outcomes From the Phase III AGITG MAX Trial of Capecitabine Alone or in Combination With Bevacizumab and Mitomycin in Advanced Colorectal Cancer. J Clin Oncol 2011;29:2675-82. [Crossref] [PubMed]
- Romero D. TMB is linked with prognosis. Nat Rev Clin Oncol 2019;16:336. [Crossref] [PubMed]
- Vanderwalde A, Spetzler D, Xiao N, et al. Microsatellite instability status determined by next-generation sequencing and compared with PD-L1 and tumor mutational burden in 11,348 patients. Cancer Med 2018;7:746-56. [Crossref] [PubMed]
- Xiao J, Li XY, Huang T, et al. A next-generation sequencing-based strategy combining microsatellite instability and tumor mutation burden for comprehensive molecular diagnosis of advanced colorectal cancer. BMC Cancer 2021;21:282. [Crossref] [PubMed]
- de la Chapelle A, Hampel H. Clinical relevance of microsatellite instability in colorectal cancer. J Clin Oncol 2010;28:3380-7. [Crossref] [PubMed]
- Hause RJ, Pritchard CC, Shendure J, et al. Classification and characterization of microsatellite instability across 18 cancer types. Nat Med 2016;22:1342-50. [Crossref] [PubMed]
- Topalian SL, Hodi FS, Brahmer JR, et al. Safety, activity, and immune correlates of anti-PD-1 antibody in cancer. N Engl J Med 2012;366:2443-54. [Crossref] [PubMed]
- Ribas A, Camacho LH, Lopez-Berestein G, et al. Antitumor activity in melanoma and anti-self responses in a phase I trial with the anti-cytotoxic T lymphocyte-associated antigen 4 monoclonal antibody CP-675,206. J Clin Oncol 2005;23:8968-77. [Crossref] [PubMed]
- Xiao Y, Freeman GJ. The microsatellite instable subset of colorectal cancer is a particularly good candidate for checkpoint blockade immunotherapy. Cancer Discov 2015;5:16-8. [Crossref] [PubMed]
- Gubin MM, Zhang X, Schuster H, et al. Checkpoint blockade cancer immunotherapy targets tumour-specific mutant antigens. Nature 2014;515:577-81. [Crossref] [PubMed]
- Meng X, Huang Z, Teng F, et al. Predictive biomarkers in PD-1/PD-L1 checkpoint blockade immunotherapy. Cancer Treat Rev 2015;41:868-76. [Crossref] [PubMed]
- Farchoukh L, Kuan SF, Dudley B, et al. MLH1-deficient Colorectal Carcinoma With Wild-type BRAF and MLH1 Promoter Hypermethylation Harbor KRAS Mutations and Arise From Conventional Adenomas. Am J Surg Pathol 2016;40:1390-9. [Crossref] [PubMed]
- Wu Y, Xu J, Xu J, et al. The predictive value of tumor mutation burden for immune checkpoint inhibitors therapy in non-small cell lung cancer is affected by patients' age. Biomark Res 2020;8:9. [Crossref] [PubMed]
- Paradiso V, Garofoli A, Tosti N, et al. Diagnostic Targeted Sequencing Panel for Hepatocellular Carcinoma Genomic Screening. J Mol Diagn 2018;20:836-48. [Crossref] [PubMed]
- Johnson DB, Frampton GM, Rioth MJ, et al. Targeted Next Generation Sequencing Identifies Markers of Response to PD-1 Blockade. Cancer Immunol Res 2016;4:959-67. [Crossref] [PubMed]
- Chen C, Liang C, Wang S, et al. Expression patterns of immune checkpoints in acute myeloid leukemia. J Hematol Oncol 2020;13:28. [Crossref] [PubMed]
- Liu S, Tang Q, Huang J, et al. Prognostic analysis of tumor mutation burden and immune infiltration in hepatocellular carcinoma based on TCGA data. Aging (Albany NY) 2021;13:11257-80. [Crossref] [PubMed]
- Zeng Y, Li N, Chen R, et al. Screening of hub genes associated with prognosis in non-small cell lung cancer by integrated bioinformatics analysis. Transl Cancer Res 2020;9:7149-64. [Crossref]
- Sun P, Chen C, Xia Y, et al. Mutation Profiling of Malignant Lymphoma by Next-Generation Sequencing of Circulating Cell-Free DNA. J Cancer 2019;10:323-31. [Crossref] [PubMed]
- Zhuang W, Ma J, Chen X, et al. The Tumor Mutational Burden of Chinese Advanced Cancer Patients Estimated by a 381-cancer-gene Panel. J Cancer 2018;9:2302-7. [Crossref] [PubMed]
- Jin Y, Chen DL, Wang F, et al. The predicting role of circulating tumor DNA landscape in gastric cancer patients treated with immune checkpoint inhibitors. Mol Cancer 2020;19:154. [Crossref] [PubMed]
- Zhu Y, Sun L, Yu J, et al. Identification of biomarkers in colon cancer based on bioinformatic analysis. Transl Cancer Res 2020;9:4879-95. [Crossref]
- Wang Z, Duan J, Cai S, et al. Assessment of Blood Tumor Mutational Burden as a Potential Biomarker for Immunotherapy in Patients With Non-Small Cell Lung Cancer With Use of a Next-Generation Sequencing Cancer Gene Panel. JAMA Oncol 2019;5:696-702. [Crossref] [PubMed]
- Battaglin F, Naseem M, Lenz HJ, et al. Microsatellite instability in colorectal cancer: overview of its clinical significance and novel perspectives. Clin Adv Hematol Oncol 2018;16:735-45. [PubMed]
- Le DT, Kim TW, Van Cutsem E, et al. Phase II Open-Label Study of Pembrolizumab in Treatment-Refractory, Microsatellite Instability-High/Mismatch Repair-Deficient Metastatic Colorectal Cancer: KEYNOTE-164. J Clin Oncol 2020;38:11-9. [Crossref] [PubMed]
- Sargent DJ, Marsoni S, Monges G, et al. Defective mismatch repair as a predictive marker for lack of efficacy of fluorouracil-based adjuvant therapy in colon cancer. J Clin Oncol 2010;28:3219-26. [Crossref] [PubMed]
- Oliveira AF, Bretes L, Furtado I. Review of PD-1/PD-L1 Inhibitors in Metastatic dMMR/MSI-H Colorectal Cancer. Front Oncol 2019;9:396. [Crossref] [PubMed]
- Chalabi M, Fanchi LF, Dijkstra KK, et al. Neoadjuvant immunotherapy leads to pathological responses in MMR-proficient and MMR-deficient early-stage colon cancers. Nat Med 2020;26:566-76. [Crossref] [PubMed]
(English Language Editor: C. Betlzar)