Combined screening analysis of aberrantly methylated-differentially expressed genes and pathways in hepatocellular carcinoma
Introduction
Liver hepatocellular carcinoma (HCC) is a common and fatal malignant tumor that seriously threatens human life and health (1). In recent years, the incidence of HCC has increased in most countries and regions around the world (2). Despite constant advancement in the screening, diagnosis, and treatment of HCC, the prognosis of this cancer remains poor (3). There has been almost no change in the survival rate of HCC over a 20-year period in most countries (4). Many patients are at the final stage of the disease or have distant metastases when diagnosed. Therefore, there is an urgent need to identify more sensitive and specific biomarkers related to the occurrence and progression of HCC for the accurate early diagnosis and prognosis of patients.
Studies have shown that the occurrence of HCC is largely determined by the combined effects of genetic and environmental factors (5). The occurrence and progression of HCC are usually characterized by genetic and genomic changes; however, in recent years, various studies have pointed out that HCC is also associated with epigenetics (6). DNA methylation (7), the most common epigenetic modification, is catalyzed by DNA methyltransferases (DNMTs). The active methyl group is transferred from S-adenosyl methionine (SAM) to the 5-position carbon of cytosine to form 5-methylcytosine (5MC) without altering the DNA sequence. This causes changes in DNA conformation, DNA stability, and the manner in which DNA interacts with proteins, thus controlling gene expression. The abnormal methylation status includes hypermethylation and hypomethylation, which are closely related to the occurrence and development of tumors (8). In tumor cells, the hypermethylation of the promoters of the tumor suppressor gene hinders the expression of tumor suppressor gene, leading to the occurrence of tumor. In addition, the hypomethylation of the specific gene can cause oncogene activation and promote tumor growth and metastasis.
Abnormal DNA methylation plays important roles in the occurrence and development of HCC. Abnormal methylation changes the spatial structure of chromatin by recruiting methylation binding proteins and related complexes, and makes it difficult for transcription factors to approach DNA double strand, and then prevents gene transcription, leading to the formation of HCC (9). At present, it has been found that a series of the genes have abnormal methylation changes in HCC (10). The functions of these genes are related to cell cycle regulation, apoptosis regulation, DNA repair, cell signal transduction, etc. The study of genes with abnormal methylation can help us to understand the regulation of tumor gene expression and may provide a new theoretical basis for the diagnosis and treatment of HCC.
In recent years, microarray chip technology has been widely used in the fields of medicine and biology research, as it provides a large amount of high-throughput data for genes and plays an important role in the study of tumor gene expression profiles and the search for key genes associated with cancer (11). The emergence of bioinformatics and its massive data resources can aid in exploring and identifying valuable gene network maps and functional pathways related to HCC (12). Our study analyzed the gene expression profiles and methylation chip microarray data of HCC using a series of bioinformatic tools. With this approach, we hope to identify aberrantly methylated genes and pathways and elucidate their underlying molecular mechanisms in HCC.
Methods
Identification of differently methylated-differentially expressed genes (DEGs) in HCC
In this study, we selected the gene expression profiling dataset GSE101685 and gene methylation profiling dataset GSE44909 from the Gene Expression Omnibus (GEO) of the National Center for Biotechnology Information (https://www.ncbi.nlm.nih.gov/geo/). The study was conducted in accordance with the Declaration of Helsinki (as revised in 2013).
The GSE101685 dataset included 24 HCC and 8 normal liver specimens (platform: GPL570 Affymetrix Human Genome U133 Plus 2.0 Array, Thermo Fisher Scientific, Waltham, MA, USA). For the gene methylation profiling microarray, the GSE44909 dataset included a total of 12 HCC samples and 8 normal samples (platform: GPL8490 HumanMethylation27_270596_v.1.2). In the GSE44909 dataset, The Illumina Infinium 27k Human DNA methylation Beadchip v1.2 was used to obtain DNA methylation profiles across approximately 27,000 CpGs.
The GEO2R software (https://www.ncbi.nlm.nih.gov/geo/geo2r/) was used to analyze the DEGs and differentially methylated genes (DMGs) in the microarray data from GSE101685 and GSE44909, using P<0.05, and t >2 as the cut-off criteria. Subsequently, hypomethylation-high expression genes (Hypo-HGs) were obtained by overlapping the hypomethylation and upregulated genes. Hypermethylation-low expression genes (Hyper-LGs) were obtained by overlapping the hypermethylation and downregulated genes (http://bioinformatics.psb.ugent.be/webtools/Venn/).
Functional and pathway enrichment analysis
After obtaining the Hypo-HGs and Hyper-LGs, Gene Ontology (GO) analysis and Kyoto Encyclopedia of Genes and Genomes (KEGG) pathway enrichment analysis were performed using the Database for Annotation, Visualization, and Integrated Discovery (DAVID, https://david.ncifcrf.gov/). Statistical significance was set at P<0.05.
Protein-protein interaction (PPI) network analysis and the Molecular Complex Detection (MCODE)
In this study, the Search Tool for the Retrieval of Interacting Genes database (STRING, https://www.string-db.org/) was used to analyze the functional PPI network of Hypo-HGs and Hyper-LGs, with the aim of interpreting the molecular mechanisms of key cellular activities in HCC. An interaction score of 0.4 was regarded as the cut-off criterion.
Subsequently, MCODE was performed using the Cytoscape software (13) to screen modules (MCODE score >3 and number of nodes >4) within the PPI network. We also analyzed the functional enrichment of the genes in the screened modules using DAVID (P<0.05). Afterwards, we selected the hub genes (connection degree >10) using the cytoHubba app in the Cytoscape software.
Verification of the hub genes in The Cancer Genome Atlas (TCGA) database
We then verified the mRNA expression levels and methylation status of the selected hub genes in the TCGA database. Additionally, we studied the survival curves of hub genes using the Kaplan–Meier method (http://ualcan.path.uab.edu/).
Results
Identification of the Hypo-HGs and Hyper-LGs in HCC
DEGs and DMGs were screened from the microarray data of GSE101685 and GSE44909 using the GEO2R software online. A total of 80 Hypo-HGs were obtained by overlapping 2,305 hypomethylation genes and 907 upregulated genes. Contrastingly, a total of 189 Hyper-LGs were obtained by overlapping 3,758 hypermethylation genes and 1,260 downregulated genes (Figure 1).
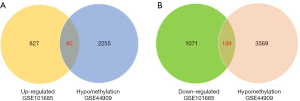
GO and KEGG pathway analysis
The top 5 significant terms of the GO enrichment analysis of 80 Hypo-HGs and 189 Hyper-LGs in DAVID are separately illustrated in Tables 1 and 2, respectively.
Table 1
Go analysis | Term | Count | % | P value |
---|---|---|---|---|
GOTERM_BP | DNA replication | 7 | 8.8 | 7.8E-5 |
GOTERM_BP | DNA replication initiation | 4 | 5.0 | 4.2E-4 |
GOTERM_BP | Response to unfolded protein | 4 | 5.0 | 9.4E-4 |
GOTERM_BP | Mitotic nuclear division | 7 | 8.8 | 9.7E-4 |
GOTERM_BP | Regulation of signal transduction by p53 class mediator | 5 | 6.2 | 2.5E-3 |
GOTERM_CC | Nucleus | 46 | 57.5 | 2.5E-7 |
GOTERM_CC | Nucleoplasm | 30 | 37.5 | 1.8E-6 |
GOTERM_CC | Nuclear chromosome, telomeric region | 7 | 8.8 | 2.0E-5 |
GOTERM_CC | Nucleosome | 6 | 7.5 | 5.1E-5 |
GOTERM_CC | Senescence-associated heterochromatin focus | 3 | 3.8 | 1.1E-4 |
GOTERM_MF | Protein binding | 57 | 71.2 | 4.8E-5 |
GOTERM_MF | Protein kinase binding | 8 | 10.0 | 1.4E-3 |
GOTERM_MF | ATP binding | 15 | 18.8 | 5.6E-3 |
GOTERM_MF | DNA binding | 16 | 20.0 | 6.2E-3 |
GOTERM_MF | Single-stranded DNA binding | 4 | 5.0 | 8.2E-3 |
Hypo-HGs, hypomethylation-high expression genes; HCC, hepatocellular carcinoma; ATP, adenosine triphosphate.
As shown in Table 1, a total of 80 Hypo-HGs were enriched in the biological processes (BPs) of DNA replication, DNA replication initiation, response to unfolded protein, mitotic nuclear division, and regulation of signal transduction by the p53 class mediator. As for the molecular function (MF), these genes showed enrichment in protein binding, protein kinase binding, adenosine triphosphate (ATP) binding, DNA binding, and single-stranded DNA binding. The cell component (CC) indicated enrichment predominantly in the nucleus, nucleoplasm, nuclear chromosome, telomeric region, nucleosome, and senescence-associated heterochromatin foci.
As shown in Table 2, a total of 189 Hyper-LGs were enriched in the BPs, including cell adhesion, positive regulation of inflammatory response, immune response, metabolic process, and positive regulation of nuclear factor kappa B (NF-kappaB) transcription factor activity. MF enrichment indicated receptor activity, receptor for advanced glycation endproducts (RAGE) receptor binding, pyridoxal phosphate binding, carbohydrate binding, and oxidoreductase activity. In addition, the CC displayed the plasma membrane, extracellular region, integral component of plasma membrane, mitochondrial matrix, and extracellular space.
Table 2
GO analysis | Term | Count | % | P value |
---|---|---|---|---|
GOTERM_BP | Cell adhesion | 16 | 8.5 | 1.0E-4 |
GOTERM_BP | Positive regulation of inflammatory response | 7 | 3.7 | 1.2E-4 |
GOTERM_BP | Immune response | 15 | 7.9 | 1.4E-4 |
GOTERM_BP | Metabolic process | 9 | 4.8 | 4.0E-4 |
GOTERM_BP | Positive regulation of NF-kappaB transcription factor activity | 8 | 4.2 | 5.1E-4 |
GOTERM_CC | Plasma membrane | 67 | 35.4 | 2.8E-5 |
GOTERM_CC | Extracellular region | 34 | 18.0 | 6.7E-5 |
GOTERM_CC | Integral component of plasma membrane | 31 | 16.4 | 8.3E-5 |
GOTERM_CC | Mitochondrial matrix | 13 | 6.9 | 1.3E-4 |
GOTERM_CC | Extracellular space | 29 | 15.3 | 2.0E-4 |
GOTERM_MF | Receptor activity | 11 | 5.8 | 1.0E-4 |
GOTERM_MF | RAGE receptor binding | 4 | 2.1 | 1.8E-4 |
GOTERM_MF | pyridoxal phosphate binding | 5 | 2.6 | 3.0E-3 |
GOTERM_MF | Carbohydrate binding | 8 | 4.2 | 4.7E-3 |
GOTERM_MF | Oxidoreductase activity | 8 | 4.2 | 5.2E-3 |
Hyper-LGs, hypermethylation-low expression genes; HCC, hepatocellular carcinoma; NF-kappaB, nuclear factor kappa B; RAGE, receptor for advanced glycation endproducts.
The KEGG pathway enrichment analysis results showed that a total of 80 Hypo-HGs were significantly enriched in pathways such as DNA replication, cell cycle, systemic lupus erythematosus, alcoholism, viral carcinogenesis, and spliceosome (Table 3 and Figure 2A).
Table 3
Pathway name | Genes | Count | % | P value |
---|---|---|---|---|
DNA replication | RFC5, FEN1, PRIM1, MCM3, MCM4 | 5 | 6.2 | 5.0E-5 |
Cell cycle | CDKN2B, PTTG1, CDKN2A, CHEK1, MCM3, MCM4, E2F3 | 7 | 8.8 | 7.1E-5 |
Systemic lupus erythematosus | H2AFZ, HIST1H2AE, HIST1H3F, HIST1H2BH, HIST2H2BE, HIST1H3E, SNRPB | 7 | 8.8 | 1.1E-4 |
Alcoholism | H2AFZ, HIST1H2AE, HIST1H3F, HIST1H2BH, HIST2H2BE, HIST1H3E | 6 | 7.5 | 3.4E-3 |
Viral carcinogenesis | CDKN2B, CDKN2A, CHEK1, HIST1H2BH, HIST2H2BE | 5 | 6.2 | 3.0E-2 |
Spliceosome | SF3B4, HSPA2, LSM4, SNRPB | 4 | 5.0 | 4.2E-2 |
KEGG, Kyoto Encyclopedia of Genes and Genomes; Hypo-HGs, hypomethylation-high expression genes; HCC, hepatocellular carcinoma.
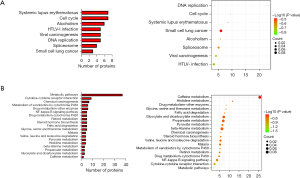
As shown in Table 4 and Figure 2B, a total of 189 Hyper-LGs were significantly enriched in metabolic pathways; drug metabolism; enzymes; chemical carcinogenesis; glycine, serine, and threonine metabolism; fatty acid degradation; histidine metabolism; metabolism of xenobiotics by cytochrome P450; steroid hormone biosynthesis; retinol metabolism; drug metabolism—cytochrome P450; pyruvate metabolism; cytokine–cytokine receptor interaction; valine leucine and isoleucine degradation; malaria; and NF-kappaB signaling.
Table 4
Pathways | Genes | Count | % | P value |
---|---|---|---|---|
Metabolic pathways | CDA, ALAS1, NNMT, B4GALT1, GDA, ADH1A, ADK, UGT2B28, PLA2G5, COMT, CYP3A4, CSAD, CYP3A5, CNDP1, ACAT1, HSD11B1, ALDH2, ACADL, KYNU, PLCG2, MAN1C1, ENPP1, UPP2, ACADM, AASS, AGXT2, GALNT2, MUT, GSTZ1, GRHPR, GATM, CYP2A6, HAL, BDH2, NAT1, SARDH, ASPA | 37 | 19.6 | 3.8E-5 |
Drug metabolism – other enzymes | CDA, CYP2A6, NAT1, UGT2B28, UPP2, CYP3A4 | 6 | 3.2 | 6.6E-4 |
Chemical carcinogenesis | HSD11B1, CYP2A6, NAT1, ADH1A, UGT2B28, CYP3A4, CYP3A5 | 7 | 3.7 | 1.4E-3 |
Glycine, serine, and threonine metabolism | GRHPR, GATM, ALAS1, AGXT2, SARDH | 5 | 2.6 | 2.9E-3 |
Fatty acid degradation | ACADL, ALDH2, ADH1A, ACADM, ACAT1 | 5 | 2.6 | 3.8E-3 |
Histidine metabolism | HAL, ALDH2, ASPA, CNDP1 | 4 | 2.1 | 4.4E-3 |
Metabolism of xenobiotics by cytochrome P450 | HSD11B1, CYP2A6, ADH1A, UGT2B28, CYP3A4, CYP3A5 | 6 | 3.2 | 5.5E-3 |
Steroid hormone biosynthesis | HSD11B1, UGT2B28, COMT, CYP3A4, CYP3A5 | 5 | 2.6 | 1.2E-2 |
Retinol metabolism | CYP2A6, ADH1A, UGT2B28, CYP3A4, CYP3A5 | 5 | 2.6 | 1.7E-2 |
Drug metabolism – cytochrome P450 | CYP2A6, ADH1A, UGT2B28, CYP3A4, CYP3A5 | 5 | 2.6 | 2.0E-2 |
Pyruvate metabolism | GRHPR, ALDH2, LDHD, ACAT1 | 4 | 2.1 | 2.3E-2 |
Cytokine-cytokine receptor interaction | CX3CR1, TSLP, IL2RB, CCL4, TNFSF11, LIFR, CCL19, CXCL14, IL18R1 | 9 | 4.8 | 3.3E-2 |
Valine, leucine, and isoleucine degradation | ALDH2, ACADM, MUT, ACAT1 | 4 | 2.1 | 3.5E-2 |
Malaria | KLRB1, HBB, SELE, TLR4 | 4 | 2.1 | 3.9E-2 |
NF-κB signaling pathway | CCL4, PLCG2, TNFSF11, CCL19, TLR4 | 5 | 2.6 | 4.5E-2 |
KEGG, Kyoto Encyclopedia of Genes and Genomes; Hyper-LGs, hypermethylation-low expression genes; HCC, hepatocellular carcinoma.
PPI network construction, module analysis, and hub gene selection
The PPI network analysis of Hypo-HGs and Hyper-LGs was performed using the STRING database, and MCODE analysis was performed using the Cytoscape software.
The results of the PPI network of Hypo-HGs are shown in Figure 3A, and the top 2 modules are displayed in Figure 3B. The genes in the significant core modules were enriched in pathways of DNA replication and systemic lupus erythematosus, as shown in Table 5.
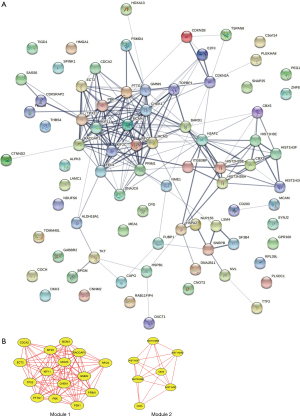
Table 5
Term ID | Term description | FDR | Nodes |
---|---|---|---|
hsa03030 | DNA replication | 1.29e-09 | TPX2, MCM3, MCM4, PBK, GMNN, PRIM1, KIF2C, FEN1, RACGAP1, KIF11, PTTG1, CHEK1, ECT2, RFC5, CDCA2 |
hsa05322 | Systemic lupus erythematosus | 0.0015 | CBX3, HIST1H3E, HIST1H3F, HIST2H2BE, HIST1H2AE, HIST1H2BH, CBX5 |
Hypo-HG, hypomethylation-high expression gene; FDR, false discovery rate.
The results of the PPI network of the Hyper-LGs are illustrated in Figure 4A, and the top 4 modules are displayed in Figure 4B. Significantly enriched pathways included the interleukin-17 (IL-17) signaling pathway; steroid hormone biosynthesis; the prolactin signaling pathway; and glycine, serine, and threonine metabolism (Table 6).
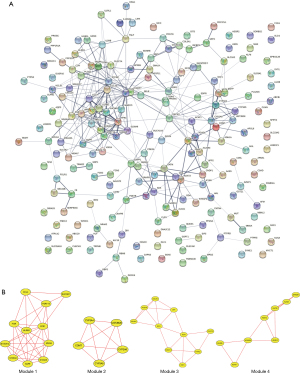
Table 6
Term ID | Term description | FDR | Nodes |
---|---|---|---|
hsa04657 | IL-17 signaling pathway | 0.0171 | P2RY13, S100A8, SUCNR1, AQP9, MNDA, FPR1, S100A9, S100A12, CCL4, LILRB2, FGR |
hsa00140 | Steroid hormone biosynthesis | 6.83e-09 | CYP3A5, CYP2A6, CYP3A4, COMT, UGT2B28 |
hsa04917 | Prolactin signaling pathway | 0.0246 | ESR1, TLR4, COL6A1, POSTN, PTPRC, TNFSF11, COL3A1, DPT, CLEC7A, BGN |
hsa00260 | Glycine, serine, and threonine metabolism | 1.68e-08 | ACADM, GATM, ALDH2, AGXT2, SARDH, ALAS1, ACAT1, ACADL |
Hypo-HG, hypomethylation-high expression gene; FDR, false discovery rate.
The top 5 hub genes selected for Hypo-HGs were minichromosome maintenance complex component 3 (MCM3), checkpoint kinase 1 (CHEK1), kinesin family member 11 (KIF11), PDZ binding kinase (PBK), and Rac GTPase activating protein 1 (RACGAP1; Figure 5A).
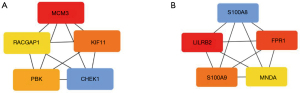
The top 5 hub genes of Hyper-LGs included leukocyte immunoglobulin like receptor B2 (LILRB2), formyl peptide receptor 1 (FPR1), S100 calcium binding protein A9 (S100A9), S100 calcium binding protein A8 (S100A8), and myeloid cell nuclear differentiation antigen (MNDA; Figure 5B).
Verification of the hub genes in the TCGA database
The expression of the hub genes of Hypo-HGs and Hyper-LGs were then verified in the TCGA database. As shown in Figures 6,7, our results were consistent with the mRNA expression and methylation status of MCM3, CHEK1, KIF11, PBK, FPR1, and S100A9 (P<0.05), but not with those of RACGAP1, LILRB2, S100A8, and MNDA.
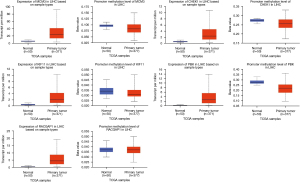
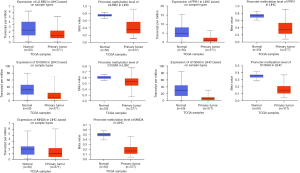
The Kaplan–Meier method was used to evaluate the relationship between these hub genes and the prognosis of patients with HCC. The results showed that the abnormal expression of MCM3, CHEK1, KIF11, PBK, RACGAP1, and S100A9 was significantly correlated with the prognosis of patients with HCC (P<0.05, Figure 8).
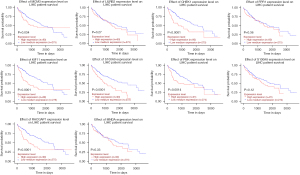
Discussion
With the continuous development of techniques, an increasing number of biomarkers for the early diagnosis of HCC have been discovered, including the pathogenesis of cancer, early diagnosis, disease monitoring, and prognosis evaluation based on genes (14). Previous studies have shown that abnormal methylated DNA detected in tissues, blood, feces, urine, and other sites in the bodies of patients with HCC may be a biomarker for early diagnosis (15). Recently, an increasing number of studies have used chip technology to identify abnormal methylation genes in tissues to help improve the prognosis of HCC (16). We selected and analyzed gene expression microarrays (GSE101685) and gene methylation microarrays (GSE44909) in the GEO database, screened aberrantly methylated–DEGs, studied the important biological functions and pathways in HCC, and verified the core genes, aiming to provide new clues for exploring new tumor markers and therapeutic targets of HCC. In particular, Cai et al. (17) also studied the aberrantly methylated-DEGs and pathways in HCC using the similar method in their study. However, they used normal liver tissue sample or adjacent non-tumor samples as the control in their study. But we think the molecular biological behavior in the normal liver tissue sample and adjacent non-tumor sample are different. In our study, we specially chose normal liver tissue as control, not the adjacent non-tumor sample, to assure the standardization and preciseness of the study. Besides, in our study, we screened out different hub-genes compared to the study of Cai et al. This suggested the complexity of molecular biological behavior of liver cancer.
A total of 80 Hypo-HGs were screened in this study. The results of the GO analysis suggest that Hypo-HGs are associated with BPs such as DNA replication, DNA replication initiation, response to unfolded proteins, mitotic division, and regulation of signal transduction by p53 mediators. The KEGG analysis revealed that Hypo-HGs were associated with DNA replication, cell cycle, viral carcinogenesis, and spliceosome pathways. During cell division, the precise transmission of epigenetic information to the next generation, such as DNA methylation and histone modification, requires the participation of DNA replication elements to ensure genome stability (18). Abnormal DNA methylation leads to disorders of the DNA replication process, eventually resulting in reduced genomic stability and the occurrence of cancer (19). Abnormal regulation of the cell cycle and mitosis can lead to excessive cell proliferation and the occurrence and development of malignant tumors. This suggests that these hypomethylated and overexpressed genes may play an important role in regulating the growth of HCC cells by influencing the cell cycle and mitosis (20). Although the causes of HCC are complex, the most common is hepatitis virus infection, including hepatitis B (HBV) and hepatitis C (HCV). Long-term chronic viral infection can lead to hepatitis cirrhosis, which eventually develops into HCC (21). Various studies have found that abnormal methylation plays an important regulatory role in the occurrence and development of HCC caused by viral hepatitis and could be an independent risk factor for HCC recurrence (22). The spliceosome is a complex ribosomal protein complex that is responsible for the splicing of the precursor mRNA (23). Recently, studies on the regulation mechanism of aberrant splicing and related diseases have found that splicing abnormalities are common in cancer processes, particularly in the migration and metabolism of cancer cells, regulation of cell growth, induction of angiogenesis, and escape from growth inhibitory factors (24).
In this study, we identified 5 core genes in Hypo-HGs, including MCM3, CHEK1, KIF11, PBK, and RACGAP1. As an important factor in the process of cell DNA replication, MCM3 can be used to directly reflect the proliferation state of tumor cells. It is associated with the occurrence of a variety of tumors, and the level of MCM3 can be used to diagnose malignant tumors and determine their prognosis (25). CHEK1, an important modulator of the DNA repair response, is overexpressed in a variety of tumor cells, suggesting that tumor cells are dependent on CHEK1 to alleviate the damage caused by stress during replication. Accordingly, CHEK1 could be used as a target to attack tumor cells (26). KIF11 is a member of the kinesin family and plays a key role in spindle bipolarity. Overexpression of KIF11 can lead to abnormal cell division and genomic instability, which are closely related to tumorigenesis (27). Studies have found that abnormal expression of KIF11 is closely related to HCC progression and prognosis, indicating that it could be a biomarker for the prognosis and treatment of HCC (28). PBK is a T cell-derived protein kinase, belonging to the serine/threonine protein kinase, with high expression in the testis and thymus and low expression in normal cells (29). However, studies have found that PBK expression is upregulated in tumor cells, which could promote the occurrence and development of cancer by phosphorylation of downstream target genes (30). RACGAP1 is highly expressed in HCC and correlated with TNM stage, pathological grade, tumor size, and poor prognosis of patients, suggesting that RACGAP1 can promote the occurrence and progression of HCC (31). It can be used as a valuable tumor marker and therapeutic target for HCC.
We screened 189 Hyper-LGs, and the results of the GO analysis indicated that the Hyper-LGs were related to cell adhesion, positive regulation of inflammatory response, immune response, metabolic process, and positive regulation of NF-kappaB transcription factor activity. The KEGG analysis revealed that hypermethylated and underexpressed genes were mainly associated with amino acid metabolism, cytochrome P450 metabolism, the IL-17 signaling pathway, and the NF-kappaB signaling pathway. Amino acid metabolism plays a decisive role in tumor growth and progression, and it is disordered in HCC cells (32). HCC cells secrete specific enzymes to regulate the metabolism of amino acids, and the infinite growth and proliferation of tumor cells require strong metabolic functions (33). The abnormal activity of the serine and glycine signaling pathways provides the main guarantee for satisfying the abundant nutrient requirements of tumor cells (34). In addition, the serine signaling pathway can provide biological precursors for many substances, such as proteins, nucleic acids, fatty acids, and cell membranes for tumor cell proliferation (35). Some studies have shown that abnormal methylation in tumor cells may affect the gene regulation process of this pathway (36). The key genes identified in our KEGG analysis could help us to understand the mechanism of epigenetic methylation regulation of amino acid metabolism in HCC cells. Cytochrome P450 (CYP) is involved in the metabolism of endogenous and exogenous substances, and any changes in its activity directly affect the transformation of toxic substances, leading eventually to the accumulation of toxic substances in the liver (37). Various studies have shown that changes in the CYP metabolic pathway are associated with HCC susceptibility (38). IL-17 plays an important role in the occurrence and development of HCC. It can promote the metastasis of HCC by inducing the secretion of matrix metallopeptidase 2 (MMP2) and matrix metallopeptidase 9 (MMP9) via the NF-kappaB pathway (39).
In this study, we identified 5 core genes in Hyper-LGs, including LILRB2, FPR1, S100A9, S100A8, and MNDA. In the recent years, an increasing number of studies have shown that LILRB2 is a cancer-promoting molecule which is overexpressed in various tumor cells (40). Previous studies have also found that LILRB2 is highly expressed in HCC and that it enhances the proliferation, migration, and invasion abilities of HCC cells and is associated with poor prognosis (41) FPR1 is abnormally expressed in a variety of cancers and is significantly correlated with patient prognosis (42) S100A9, a calcium-binding protein, is a member of the S100 protein family and often forms a heterodimer with S100A8. Studies have shown that S100A8 and S100A9 are closely related to the malignancy degree and prognosis of patients with HCC (43).
In addition, we verified 10 screened core genes in the TCGA database. The results showed that the methylation states and expression differences of MCM3, CHEK1, KIF11, PBK, FPR1, and S100A9 were consistent with our results. The results of the Kaplan–Meier survival analysis in the TCGA database revealed that the expression levels of MCM3, CHEK1, KIF11, PBK, RACGAP1, and S100A9 were significantly correlated with patient prognosis. Combined with the results of the microarray and TCGA database analysis, we finally determined that the Hub genes, including MCM3, CHEK1, KIF11, PBK, and S100A9, could be used as the important biomarkers for the prognosis of HCC in patients with HCC.
Nevertheless, the exact regulatory epigenetic mechanism, particularly the correlation between DNA methylation and clinical manifestations, still lacks sufficient evidence. As there is a certain distance between our study results and the actual diagnosis and treatment of patients in clinical practice, and validating the expressions and functions of representative hub genes by real world data may better for elucidating the epigenetic mechanism in HCC, our findings need to be confirmed by further basic research and large-scale clinical studies.
Conclusions
Combined screening of aberrantly methylated–DEGs based on bioinformatic analysis may provide new clues for elucidating the epigenetic mechanism in HCC. Hub genes, including MCM3, CHEK1, KIF11, PBK, and S100A9, may serve as biomarkers for the precise diagnosis of HCC.
Acknowledgments
We thank the researchers of the GSE101685, GSE44909, and TCGA datasets who shared all these data to the public.
Funding: None.
Footnote
Conflicts of Interest: All authors have completed the ICMJE uniform disclosure form (available at https://jgo.amegroups.com/article/view/10.21037/jgo-21-866/coif). The authors have no conflicts of interest to declare.
Ethical Statement: The authors are accountable for all aspects of the work in ensuring that questions related to the accuracy or integrity of any part of the work are appropriately investigated and resolved. The study was conducted in accordance with the Declaration of Helsinki (as revised in 2013).
Open Access Statement: This is an Open Access article distributed in accordance with the Creative Commons Attribution-NonCommercial-NoDerivs 4.0 International License (CC BY-NC-ND 4.0), which permits the non-commercial replication and distribution of the article with the strict proviso that no changes or edits are made and the original work is properly cited (including links to both the formal publication through the relevant DOI and the license). See: https://creativecommons.org/licenses/by-nc-nd/4.0/.
References
- Habibollahi P, Sheth RA, Cressman ENK. Histological Correlation for Radiofrequency and Microwave Ablation in the Local Control of Hepatocellular Carcinoma (HCC) before Liver Transplantation: A Comprehensive Review. Cancers (Basel) 2020;13:104. [Crossref] [PubMed]
- Berman ZT, Newton I. Diagnosis, Staging, and Patient Selection for Locoregional Therapy to Treat Hepatocellular Carcinoma. Semin Intervent Radiol 2020;37:441-7. [Crossref] [PubMed]
- Damiris K, Abbad H, Pyrsopoulos N. Cellular based treatment modalities for unresectable hepatocellular carcinoma. World J Clin Oncol 2021;12:290-308. [Crossref] [PubMed]
- Péneau C, Zucman-Rossi J, Nault JC. Genomics of Viral Hepatitis-Associated Liver Tumors. J Clin Med 2021;10:1827. [Crossref] [PubMed]
- Pan Y, Chen H, Yu J. Biomarkers in Hepatocellular Carcinoma: Current Status and Future Perspectives. Biomedicines 2020;8:576. [Crossref] [PubMed]
- Joseph DB, Strand DW, Vezina CM. DNA methylation in development and disease: an overview for prostate researchers. Am J Clin Exp Urol 2018;6:197-218. [PubMed]
- Vukic M, Daxinger L. DNA methylation in disease: Immunodeficiency, Centromeric instability, Facial anomalies syndrome. Essays Biochem 2019;63:773-83. [Crossref] [PubMed]
- Brittain WJ, Brandsetter T, Prucker O, et al. The Surface Science of Microarray Generation-A Critical Inventory. ACS Appl Mater Interfaces 2019;11:39397-409. [Crossref] [PubMed]
- Villanueva A, Portela A, Sayols S, et al. DNA methylation-based prognosis and epidrivers in hepatocellular carcinoma. Hepatology 2015;61:1945-56. [Crossref] [PubMed]
- Fan G, Tu Y, Chen C, et al. DNA methylation biomarkers for hepatocellular carcinoma. Cancer Cell Int 2018;18:140. [Crossref] [PubMed]
- Lee ECS, Elhassan SAM, Lim GPL, et al. The roles of circular RNAs in human development and diseases. Biomed Pharmacother 2019;111:198-208. [Crossref] [PubMed]
- Chandrasekaran S, Stahlberg E. Computational approaches for Cancer 2017 workshop overview. BMC Bioinformatics 2018;19:487. [Crossref] [PubMed]
- Shannon P, Markiel A, Ozier O, et al. Cytoscape: a software environment for integrated models of biomolecular interaction networks. Genome Res 2003;13:2498-504. [Crossref] [PubMed]
- Tai D, Choo SP, Chew V. Rationale of Immunotherapy in Hepatocellular Carcinoma and Its Potential Biomarkers. Cancers (Basel) 2019;11:1926. [Crossref] [PubMed]
- Nishida N, Kudo M. Alteration of Epigenetic Profile in Human Hepatocellular Carcinoma and Its Clinical Implications. Liver Cancer 2014;3:417-27. [Crossref] [PubMed]
- Nakamura M, Chiba T, Kanayama K, et al. Epigenetic dysregulation in hepatocellular carcinoma: an up-to-date review. Hepatol Res 2019;49:3-13. [Crossref] [PubMed]
- Cai C, Wang W, Tu Z. Aberrantly DNA Methylated-Differentially Expressed Genes and Pathways in Hepatocellular Carcinoma. J Cancer 2019;10:355-66. [Crossref] [PubMed]
- Zion EH, Chandrasekhara C, Chen X. Asymmetric inheritance of epigenetic states in asymmetrically dividing stem cells. Curr Opin Cell Biol 2020;67:27-36. [Crossref] [PubMed]
- Wang J, Yang J, Li D, et al. Technologies for targeting DNA methylation modifications: Basic mechanism and potential application in cancer. Biochim Biophys Acta Rev Cancer 2021;1875:188454. [Crossref] [PubMed]
- Kim JE. Bookmarking by histone methylation ensures chromosomal integrity during mitosis. Arch Pharm Res 2019;42:466-80. [Crossref] [PubMed]
- Wangensteen KJ, Chang KM. Multiple Roles for Hepatitis B and C Viruses and the Host in the Development of Hepatocellular Carcinoma. Hepatology 2021;73:27-37. [Crossref] [PubMed]
- Mak LY, Wong DK, Pollicino T, et al. Occult hepatitis B infection and hepatocellular carcinoma: Epidemiology, virology, hepatocarcinogenesis and clinical significance. J Hepatol 2020;73:952-64. [Crossref] [PubMed]
- Dumesic PA, Madhani HD. The spliceosome as a transposon sensor. RNA Biol 2013;10:1653-60. [Crossref] [PubMed]
- Agrawal AA, Yu L, Smith PG, et al. Targeting splicing abnormalities in cancer. Curr Opin Genet Dev 2018;48:67-74. [Crossref] [PubMed]
- Wen QL, Zhu SM, Jiang LH, et al. Expression and prognostic significance of MCM-3 and MCM-7 in salivary adenoid cystic carcinoma. Int J Clin Exp Pathol 2018;11:5359-69. [PubMed]
- Dominguez-Valentin M, Nakken S, Tubeuf H, et al. Results of multigene panel testing in familial cancer cases without genetic cause demonstrated by single gene testing. Sci Rep 2019;9:18555. [Crossref] [PubMed]
- Zhou Y, Yang L, Xiong L, et al. KIF11 is upregulated in colorectal cancer and silencing of it impairs tumor growth and sensitizes colorectal cancer cells to oxaliplatin via p53/GSK3β signaling. J Cancer 2021;12:3741-53. [Crossref] [PubMed]
- Hu ZD, Jiang Y, Sun HM, et al. KIF11 Promotes Proliferation of Hepatocellular Carcinoma among Patients with Liver Cancers. Biomed Res Int 2021;2021:2676745. [Crossref] [PubMed]
- Kwon CH, Park HJ, Choi YR, et al. PSMB8 and PBK as potential gastric cancer subtype-specific biomarkers associated with prognosis. Oncotarget 2016;7:21454-68. [Crossref] [PubMed]
- Park JH, Park SA, Lee YJ, et al. PBK attenuates paclitaxel-induced autophagic cell death by suppressing p53 in H460 non-small-cell lung cancer cells. FEBS Open Bio 2020;10:937-50. [Crossref] [PubMed]
- Lawson CD, Der CJ. Filling GAPs in our knowledge: ARHGAP11A and RACGAP1 act as oncogenes in basal-like breast cancers. Small GTPases 2018;9:290-6. [Crossref] [PubMed]
- Sivanand S, Vander Heiden MG. Emerging Roles for Branched-Chain Amino Acid Metabolism in Cancer. Cancer Cell 2020;37:147-56. [Crossref] [PubMed]
- Sakai H, Shirakami Y, Shimizu M. Chemoprevention of obesity-related liver carcinogenesis by using pharmaceutical and nutraceutical agents. World J Gastroenterol 2016;22:394-406. [Crossref] [PubMed]
- Gorska-Ponikowska M, Perricone U, Kuban-Jankowska A, et al. 2-methoxyestradiol impacts on amino acids-mediated metabolic reprogramming in osteosarcoma cells by its interaction with NMDA receptor. J Cell Physiol 2017;232:3030-49. [Crossref] [PubMed]
- Kim H, Park YJ. Links between Serine Biosynthesis Pathway and Epigenetics in Cancer Metabolism. Clin Nutr Res 2018;7:153-60. [Crossref] [PubMed]
- Zeng JD, Wu WKK, Wang HY, et al. Serine and one-carbon metabolism, a bridge that links mTOR signaling and DNA methylation in cancer. Pharmacol Res 2019;149:104352. [Crossref] [PubMed]
- Guengerich FP, Waterman MR, Egli M. Recent Structural Insights into Cytochrome P450 Function. Trends Pharmacol Sci 2016;37:625-40. [Crossref] [PubMed]
- Kwon YJ, Shin S, Chun YJ. Biological roles of cytochrome P450 1A1, 1A2, and 1B1 enzymes. Arch Pharm Res 2021;44:63-83. [Crossref] [PubMed]
- Zarogoulidis P, Katsikogianni F, Tsiouda T, et al. Interleukin-8 and interleukin-17 for cancer. Cancer Invest 2014;32:197-205. [Crossref] [PubMed]
- Chen HM, van der Touw W, Wang YS, et al. Blocking immunoinhibitory receptor LILRB2 reprograms tumor-associated myeloid cells and promotes antitumor immunity. J Clin Invest 2018;128:5647-62. [Crossref] [PubMed]
- Li X, Wei X, Xu H, et al. Expression of leukocyte immunoglobulin-like receptor B2 in hepatocellular carcinoma and its clinical significance. J Cancer Res Ther 2018;14:1655-9. [Crossref] [PubMed]
- Vacchelli E, Le Naour J, Kroemer G. The ambiguous role of FPR1 in immunity and inflammation. Oncoimmunology 2020;9:1760061. [Crossref] [PubMed]
- Wei R, Zhu WW, Yu GY, et al. S100 calcium-binding protein A9 from tumor-associated macrophage enhances cancer stem cell-like properties of hepatocellular carcinoma. Int J Cancer 2021;148:1233-44. [Crossref] [PubMed]