Integrated omics analysis: the relationship between significantly increased Klebsiella post-hepatectomy and decreased hub-metabolite 3-methyl-2-oxobutanoic acid is associated with induced liver failure
Introduction
Hepatocellular carcinoma (HCC) is the 6th most prevalent type of cancer in the world (1), and the 2nd leading cause of cancer-related deaths (2). Generally, HCC is the result of chronic liver diseases, including hepatitis B or C viral infections (3,4), fungal aflatoxins, excessive drinking, and non-alcoholic steatohepatitis (5). China has the largest population of liver cancer cases in the world for which hepatitis B virus (HBV) functions as the main pathogenic factor (6). Regional approaches, such as hepatectomies, liver transplantation, radiofrequency ablation, radiotherapy, and transcatheter arterial chemoembolization, are commonly used to combat HCC in its early or middle stages, and help to achieve radical or palliative treatment (7). In advanced cases, tyrosine kinase inhibitors, conventional treatments, newly developed immune checkpoint inhibitors, and other systemic treatments are considered as treatment options, but have a limited therapeutic response (8). Despite the increasing and diverse treatment options available for liver cancer, hepatectomy still remains the primary and most effective option (9). Due to advances in surgical techniques and the growing use of perioperative management, the mortality and morbidity of complications faced by post-hepatectomy liver cancer patients have been greatly reduced (10). However, post-hepatectomy liver failure (PHLF) is a frequently developing and fatal issue that requires attention (11). At present, the commonly recognized definition of PHLF is that proposed by the International Study Group of Liver Surgery (ISGLS) (12), but it is relatively subjective. A more objective and comprehensive evaluation of PHLF could help to reduce its incidence and improve the therapeutic efficacy of treatments, and thus is a vital goal that needs to be achieved.
In recent years, numerous studies have indicated that the gut microbiome participates in host liver metabolism and have found associations between multiple liver diseases and liver injury characterized by microbial dysbiosis (13,14). A study reported that gut microbiota interruptions in patients suffering from non-alcoholic fatty liver disease (NAFLD) are a cause of increased intestinal permeability to bacterial metabolites, and that the activated the Toll-like receptor 4 (TLR4)/NLRP3 inflammasome pathway by microbiome-derived lipopolysaccharide (LPS) is a risk factor for liver pathological changes, which are characterized by increased liver fat and inflammatory responses (15). In animal studies, the reduction of intestinal microbial metabolites (i.e., tryptamine and indole-3-acetic acid) from a high-fat diet was found to be involved in liver inflammation and cytokine-mediated fat production; thus, these 2 metabolites could serve as potential drug targets for NAFLD (16,17). Additionally, experiments on mice with alcoholic liver disease (ALD) revealed that human-to-mouse transfer of pro-inflammatory signals associated with gut microbial imbalance in ALD patients by fecal microbiota transplantation (FMT) could result in disease transfer, this was accomplished by gut microbiotas transformation of bile acids produced by the liver. Thus, the potential relationship between microbiota imbalance and alcohol-related liver diseases could be the result of alterations in the microbiome’s metabolic functions (18,19).
Research has shown that long-term HBV-induced liver injury can lead to an increased risk of cirrhosis and liver cancer (20). In developing countries, HBV-infected patients are rich in several opportunistic bacteria, including Fusobacterium, Clostridium difficile, Veillonella, and Escherichia coli (21). In cases of progressions to liver fibrosis, cirrhosis, or cancer (22), the gut microbiota is greatly affected, which is mainly reflected in a microbial imbalance, decreased microbial diversity, and the conversion of some beneficial bacteria to pathogenic types, such as Bifidobacterium (23,24). Gut microbes and their metabolites can cross the intestinal epithelial barrier (25,26) to participate in intestinal homeostasis (24) assisted by the liver-gut axis (27). Consequently, relevant metabolic disorders are induced accordingly (21), increasing the risk of endotoxemia, liver injury, and increasing evidence also indicates that endotoxemia produced by gut microbiota is involved in the tumorigenesis of HCC (28,29).
Abnormal serum metabolites also increase the risk of liver diseases. Aromatic amino acid metabolic disorders (30,31) have been shown to affect liver tissue functions, making them a key part in the pathogenesis of chronic liver diseases, such as liver fibrosis, cirrhosis, and HCC. Further, studies of liver transplantation and gut microbiota in mouse models of hepatectomy revealed that gut microbial metabolites are associated with aggravated ischemia-reperfusion injury, and slow liver regeneration post-operation, which in turn may lead to severe post-operative complications (32,33). Thus, interruptions to gut microbiota can be observed during the occurrence and development of liver diseases and are closely associated with metabolic disturbances.
Despite a high risk of liver failure post-operation, surgery remains the primary option for managing HBV-related liver cancer. Recent research has extended understandings of the relationship between liver disease and gut microbiota, but there is still a dearth of clinical studies on PHLF investigating this relationship. Our study examined 29 patients with HBV-related HCC (B-HCC) and found significant alterations in gut microbial abundance before and after hepatectomy. Further, Klebsiella (1 of the top 10 gut microbiota) was observed to be greatly enriched in patients who developed PHLF post-operation. In addition, fecal and serum metabolites in patients with PHLF were observed before and after by non-targeted liquid chromatography-mass spectrometry (LS-MS/MS), which was conducted to identify the association between gut microbes and the host metabolite 3-methyl-2-oxobutanoic acid. This study examined the relationship between PHLF and gut microbiota under specific metabolic pathways. Our findings may inform future treatments by reducing PHLF incidence and improving therapeutic outcomes. We present the following article in accordance with the MDAR reporting checklist (available at https://jgo.amegroups.com/article/view/10.21037/jgo-21-906/rc).
Methods
Patient recruitment and sample collection
Twenty-nine B-HCC patients who underwent continuous hepatectomy at the Department of Hepatobiliary Surgery of the Guangxi Medical University Cancer Hospital (Nanning, China) from September to December 2020 were recruited for this study. To be eligible for inclusion in this study, the patients had to meet the following inclusion criteria: (I) have an initial diagnoses of HBV infection-related primary HCC and have been treated only by surgery; (II) be Child-Pugh Class A; (III) have no gastrointestinal symptoms, such as abdominal pain, constipation, or diarrhea; (IV) have no history of alcohol addiction, asthma, or drug allergy; (V) have taken no acid suppressants, pro-gastrointestinal prokinetic agents, probiotics (biostime probiotics), or antibiotics for at least 4 weeks before surgery; (VI) have no inflammatory bowel diseases, metabolic diseases (e.g., diabetes or hypertension), non-alcoholic fatty liver, or gut microbiota-associated diseases; (VII) have no malignant tumors in sites other than the liver; and (VIII) have taken no drugs for intestinal tract cleaning in the preoperative preparation.
The 29 patients were divided into a before-operation (bo) group and after-operation (ao), group and then allocated to either Group A (n=11) or B (n=18) based on the presence or absence of PHLF, which was defined according to the consensus definition and severity grading reported by the ISGLS. Patients in Group A were then sub-divided into the before-operation (bo.PHLF) group and after-operation (ao.PHLF) group, while patients in Group B were sub-divided into the nbo.PHLF and nao.PHLF groups. Fecal and serum samples were collected from 8:00 AM to 9:00 AM 1 day before the operation, and on the 5th day after operation. The fecal samples were stored at −80 °C immediately after collection, and the serum samples were prepared from the brachial venous blood, and immediately stored at −80 °C.
The study followed the ethical guidelines of the Helsinki Declaration (as revised in 2013), and was approved by the Research Ethics Committee of Guangxi Medical University Cancer Hospital (No. KY2019009). All patients who met our experimental conditions were informed of the research contents and signed informed consent forms.
Fecal DNA extraction, microbial sequencing, analysis, and function prediction
Fecal bacterial deoxyribonucleic acid (DNA) extracted using the cetyltrimethylammonium bromide (CTAB) method was used for DNA library construction. Polymerase chain reaction (PCR) was run with the V3–V4 region of the small subunit gene of bacterial 16S ribosomal ribonucleic acid (rRNA) as the target, and the primers were designed as forward of 5'-CCTAYGGGRBGCASCAG-3' and reverse of 5'-GACTACHVGGTATCTAATCC-3'. The PCR products were purified using the GeneJET gel extraction kit (Thermo Science). The DNA library was then constructed and index coded using the TruSeq®DNA PCR-Free Sample Preparation Kit (Illumina, San Diego, CA, USA). The quality control check for the library was completed by the Qubit@2.0 Fluorimeter (Thermo Science) and Agilent BioAnalyzer 2100 System. 16S rRNA sequencing was performed on the Illumina Novaseq6000 platform (Beijing Nuohe Zhiyuan Technology Co., Ltd.). The original FASTQ file was strictly quality-filtered by FLASH (V1.2.7, http://ccb.jhu.edu/software/FLASH/) (34) plus Qiime (V1.9.1, http://qiime.org/scripts/split_libraries_fastq.html) (35), and the chimeric tag sequences were removed (https://github.com/torognes/vsearch/) (36). Using the Uparsev7.0.1001 software (http://www.drive5.com/uparse/) (37), operational taxonomic units (OTUs) were obtained at a 97% sequence homology, then annotated for further taxonomic analysis (confidence threshold = 80%) using the Mothur method and the SS UrRNA (16SrRNA) database (38) of SILVA138 (http://www.arb-silva.de/) (39). The relative abundance of OTUs was reflected at the phylum, class, order, family, genus, and species levels, based on which α and β diversity were calculated. For α diversity, the Shannon and Simpson indices were compared, while for β diversity, a principal coordinate analysis was performed with the weighted UniFrac metric and an analysis of similarities (ANOSIM) similarity analysis was carried out for comparison. The PICRUST2 (40) on the Omicsmart microbial online analysis platform (GENE DENOVO) was applied to output functional information from Intergrated Microbial Genome (IMG) microbial genome data to predict metabolic pathways based on the Kyoto Encyclopedia of Genes and Genomes (KEGG) database. The abundance data for all predicted paths were expressed as the relative value (%).
Metabolite extraction from feces and serum, analytical measurements, metabolite annotation, and metabolic pathway analysis
Fecal and serum samples were taken for the metabolite analysis by LC-MS/MS (41). Chromatographic separation was performed on an ultra-performance liquid-chromatography system (SCIEX, UK) and a Hypesil Goldcolumn (C18) column (100 mm × 2.1 mm, 1.9 µm, Thermo Fisher, USA). The molecular characteristic peaks were detected by Q ExActive™ HF (Thermo Fisher, Germany) and matched with the mzCloud, mzvault, and MassList data. The MS data obtained were processed by Component Discoverer 3.1 (CD3.1, Thermo Fisher) software for the metabolite qualitative analysis and relative quantitation. Metabolite annotation was run on the KEGG, Human Metabolome Database, and LIPIDMaps databases, and the differential metabolites were statistically analyzed, and then subjected to a KEGG pathway enrichment analysis.
Integrated omics analysis
The fecal and serum metabolite data were comprehensively analyzed to identify the overlapping metabolites in both the intestinal and host metabolite profiles. The Spearman correlations between the relative concentration of differential fecal metabolites and the relative abundance of differential microbiomes were calculated.
Statistical analysis
Fisher’s precise test and the t-test were used for between-group comparisons in relation to the preoperative demographic and clinical characteristics of patients, the amount of intraoperative blood loss, and the range of surgical resection. The results are expressed as median values (ranges). QIIME (version 1.9.1) and R (version 2.15.3) were used for the analysis of α and β diversity. The t-test or Mann-Whitney rank-sum test was performed on R (version 2.15.3) software to compare the relative abundance of the intestinal microbiome. A P value <0.05 was considered statistically significant. PICRUST2 was run to uncover the putative functions of the gut microbiome. Statistical software R (R-3.4.3), Python (Python 2.7.6), and CentOS (CentOS version 6.6) were used to conduct the statistical analysis of the qualitative and relative quantitative results of the metabolites. Metax software was used for the partial least-squares discrimination analysis (PLS-DA) of the metabolomics data, and t-tests were performed to identify any significant differences (P value). Metabolites meeting Variable Importance in the Projection (VIP) >1.0, Fold Change (FC) >1.2, or FC <0.833 and P value <0.05 were considered differential metabolites, which were statistically analyzed by R (R-3.4.3) and Python (Python-3.5.0), and then underwent a KEGG pathway enrichment analysis. Correlations between variables were calculated using a Spearman rank correlation analysis, and the P value obtained were multi-checked and adjusted using the Benjamini-Hochberg method; a P value <0.05 was set as the significant threshold.
Results
General information and clinical characteristics
Twenty-nine patients diagnosed with B-HCC at first admission were included in this study. The demographic characteristics and clinical manifestations of all the patients before hepatectomy are detailed in Table 1, and their intraoperative bleeding volume and surgical resection region are also presented. Of all the parameters between Groups A and B, only the severity grading of liver failure was found to be statistically significant.
Table 1
Characteristic | Overall (n=29) | Group A (n=11) | Group B (n=18) | P value |
---|---|---|---|---|
Age (year) | 47.62±10.80 | 44.91±8.47 | 49.28±11.93 | 0.299 |
<45, n (%) | 11 (37.9) | 5 (45.5) | 6 (33.3) | 0.696 |
≥45, n (%) | 18 (62.1) | 6 (54.5) | 12 (66.7) | |
Sex, n (%) | >0.999 | |||
Female | 6 (20.7) | 2 (18.2) | 4 (22.2) | |
Male | 23 (79.3) | 9 (81.8) | 14 (77.8) | |
BMI (kg/m2), n (%) | 0.432 | |||
≤24 | 19 (65.5) | 6 (54.5) | 13 (72.2) | |
>24 | 10 (34.5) | 5 (45.5) | 5 (27.8) | |
PLT (109/L), n (%) | 0.622 | |||
100–300 | 24 (82.8) | 10 (90.9) | 14 (77.8) | |
<100 or >300 | 5 (17.2) | 1 (9.1) | 4 (22.2) | |
TBIL (μmol/L), n (%) | 0.694 | |||
≤17.1 | 20 (69.0) | 7 (63.6) | 13 (72.2) | |
>17.1 | 9 (31.0) | 4 (36.4) | 5 (27.8) | |
ALB (g/L), n (%) | 0.060 | |||
<35 | 14 (48.3) | 8 (72.7) | 6 (33.3) | |
≥35 | 15 (51.7) | 3 (27.3) | 12 (66.7) | |
PA (mg/L) | 188.38±53.72 | 169.81±50.30 | 199.73±53.90 | 0.149 |
ALT (U/L), n (%) | >0.999 | |||
≤40 | 19 (65.5) | 7 (63.6) | 12 (66.7) | |
>40 | 10 (34.5) | 4 (36.4) | 6 (33.3) | |
AST (U/L), n (%) | >0.999 | |||
≤40 | 16 (55.2) | 6 (54.5) | 10 (55.6) | |
>40 | 13 (44.8) | 5 (45.5) | 8 (44.4) | |
HBsAg (ng/mL) | 543.20±327.91 | 657.42±317.33 | 473.40±322.90 | 0.146 |
HBV-DNA, n (%) | >0.999 | |||
≤103 | 8 (27.6) | 3 (27.3) | 5 (27.8) | |
>103 | 21 (72.4) | 8 (72.7) | 13 (72.2) | |
PT (s), n (%) | 0.237 | |||
≤13 | 20 (69.0) | 6 (54.5) | 14 (77.8) | |
>13 | 9 (31.0) | 5 (45.5) | 4 (22.2) | |
INR, n (%) | >0.999 | |||
≤1.5 | 29 (100.0) | 11 (100.0) | 18 (100.0) | |
>1.5 | 0 (0.0) | 0 (0.0) | 0 (0.0) | |
AFP (ng/mL), n (%) | >0.999 | |||
≤400 | 14 (48.3) | 5 (45.5) | 9 (50.0) | |
>400 | 15 (51.7) | 6 (54.5) | 9 (50.0) | |
Ascites, n (%) | >0.999 | |||
No | 28 (96.6) | 11 (100.0) | 17 (94.4) | |
Yes | 1 (3.4) | 0 (0.0) | 1 (5.6) | |
Smoking, n (%) | >0.999 | |||
No | 20 (69.0) | 8 (72.7) | 12 (66.7) | |
Yes | 9 (31.0) | 3 (27.3) | 6 (33.3) | |
Drinking, n (%) | >0.999 | |||
No | 27 (93.1) | 10 (90.9) | 17 (94.4) | |
Yes | 2 (6.9) | 1 (9.1) | 1 (5.6) | |
CT tumor size before operation (cm) | 7.30±4.23 | 7.09±5.02 | 7.42±3.82 | 0.842 |
Portal hypertension, n (%) | 0.646 | |||
No | 23 (79.3) | 8 (72.7) | 15 (83.3) | |
Yes | 6 (20.7) | 3 (27.3) | 3 (16.7) | |
Tumor number | 1.59±1.02 | 1.64±0.92 | 1.56±1.10 | 0.840 |
With or without envelope, n (%) | >0.999 | |||
No | 5 (17.2) | 2 (18.2) | 3 (16.7) | |
Yes | 24 (82.8) | 9 (81.8) | 15 (83.3) | |
Is the envelope intact, n (%) | 0.696 | |||
No | 11 (37.9) | 5 (45.5) | 6 (33.3) | |
Yes | 18 (62.1) | 6 (54.5) | 12 (66.7) | |
Microvascular tumor thrombus, n (%) | 0.710 | |||
No | 14 (48.3) | 6 (54.5) | 8 (44.4) | |
Yes | 15 (51.7) | 5 (45.5) | 10 (55.6) | |
Liver resection involves more than 3 segments, n (%) | 0.710 | |||
No | 15 (51.7) | 5 (45.5) | 10 (55.6) | |
Yes | 14 (48.3) | 6 (54.5) | 8 (44.4) | |
Intraoperative bleeding (mL), n (%) | 0.646 | |||
≤500 | 23 (79.3) | 8 (72.7) | 15 (83.3) | |
>500 | 6 (20.7) | 3 (27.3) | 3 (16.7) | |
BCLC stage, n (%) | 0.597 | |||
A | 16 (55.2) | 6 (54.5) | 10 (55.6) | |
B | 8 (27.6) | 4 (36.4) | 4 (22.2) | |
C | 5 (17.2) | 1 (9.1) | 4 (22.2) | |
Liver failure grade, n (%) | <0.001 | |||
No | 18 (62.1) | 0 (0.0) | 18 (100.0) | |
1 | 6 (20.7) | 6 (54.5) | 0 (0.0) | |
2 | 4 (13.8) | 4 (36.4) | 0 (0.0) | |
3 | 1 (3.4) | 1 (9.1) | 0 (0.0) |
The P value is based on a Fisher’s exact test and t-test. Group A and Group B: patients were assigned to Group A (n=11) and Group B (n=18) based on the presence or absence of PHLF, which was defined according to the consensus definition and severity grading in the ISGLS report. BMI, body mass index; PLT, platelet; TBIL, total bilirubin; ALB, albumin; PA, prealbumin; ALT, alanine aminotransferase; AST, aspartate aminotransferase; HBsAg, hepatitis B surface antigens; PT, prothrombin time; INR, international normalized ratio; AFP, alpha fetoprotein; BCLC stage, Barcelona clinic liver cancer stage.
OTUs and microbial diversity analysis
The collected fecal contents were processed for the 16S rRNA metagenomic analysis, and the OTUs were taken as a parameter to reflect different microbial taxonomies. In total, 2,645 OTUs were shared by the ao and bo groups (see Figure 1A). Of which, 1,182 OTUs were observed in bo.PHLF vs. nbo.PHLF, 1,722 in ao.PHLF vs. bo.PHLF, 1,624 in nao.PHLF vs. nbo.PHLF, and 1,403 in ao.PHLF vs. nao.PHLF (see Figure 1B). In relation to α diversity, no significant differences in richness and evenness were found in the all-group comparative analysis (see Figure S1). For β diversity, ao vs. bo (see Figure 1C) indicated significant statistical difference. bo.PHLF vs. nbo.PHLF showed no such statistically significant difference (see Figure 1D). ao.PHLF vs. bo.PHLF (see Figure 1E), and nao.PHLF vs. nbo.PHLF (see Figure 1F), presented statistically significant differences.
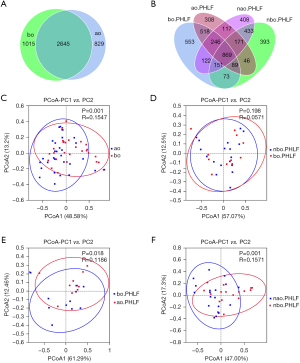
Gut microbial abundance analysis based on taxonomy
The top 10 differential bacteria were analyzed by taxonomy based on the phylum, class, order, family, and genus levels between the ao and bo groups (see Figure 2A and Figure S2A). At the phylum level, the abundance of proteobacteria was significantly higher in the ao group than the bo group. At the class level, Clostridia was less abundant while bacilli and Gammaproteobacteria were increased. At the order level, Lachnospirales and Oscillospirales showed a downward trend while Lactobacillales, Enterobacterales, and Christensenellales showed an upward trend, and their families, including Enterococcaceae, Enterobacteriaceae, Erwiniaceae, and Christensenellaceae, were observed to be highly abundant in the ao group (see Figure S2B). At the genus level, Prevotella, Bacteroides, Akkermansia, enterococcus, Escherichia-Shigella, Klebsiella, Streptococcus, Lactobacillus, Blautia, and Faecalibacterium were observed to be the top 10 bacteria of differential relative abundance in the ao vs. bo groups (see Figure 2B). Thus, gut microbial abundance is greatly affected after hepatectomy, and the abundance of Klebsiella is notably elevated.
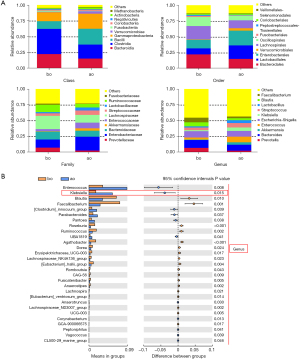
Klebsiella changes in the PHLF group
There was no significant alteration in the abundance of Klebsiella in the bo.PHLF vs. nbo.PHLF (baseline control) groups (see Figure 3A). Additionally, Klebsiella was highly abundant in the ao.PHLF vs. bo.PHLF group (see Figure 3B), but there was no significant difference between the nao.PHLF vs. nbo.PHLF groups (see Figure 3C). The abundance of Klebsiella was still listed as 1 of the top 10 genera (see Figure 3D). A PICRUST2 analysis was performed to identify the pathways related to differential gut microbes in ao.PHLF vs. bo.PHLF at the genus level (see Figure 3E). Notably, Klebsiella acted on a total of 13 amino acid-related pathways, and had a significant effect on the branched-chain amino acid (BCAA) metabolic pathway (see Table 2). In relation as previously described relationship and as evidenced by significantly weakened BCAA functions in the ao.PHLF group, Klebsiella was increased in the PHLF population.
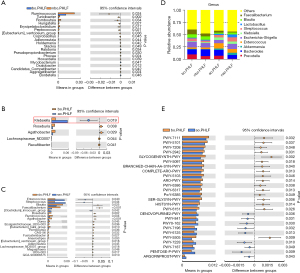
Table 2
Pathway ID | Altered pathway | P value |
---|---|---|
PWY-7111 | Pyruvate fermentation to isobutanol (engineered) | 0.032 |
PWY-5101 | L-isoleucine biosynthesis II | 0.037 |
PWY-2942 | L-lysine biosynthesis III | 0.031 |
PWY-5097 | L-lysine biosynthesis VI | 0.018 |
BRANCHED-CHAIN-AA-SYN-PWY | Superpathway of branched amino acid biosynthesis | 0.040 |
COMPLETE-ARO-PWY | Superpathway of aromatic amino acid biosynthesis | 0.013 |
PWY-5103 | L-isoleucine biosynthesis III | 0.046 |
PWY-6386 | UDP-N-acetylmuramoyl-pentapeptide biosynthesis II (lysine-containing) | 0.038 |
SER-GLYSYN-PWY | Superpathway of L-serine and glycine biosynthesis I | 0.006 |
HISTSYN-PWY | L-histidine biosynthesis | 0.014 |
PWY-6151 | S-adenosyl-L-methionine cycle I | 0.026 |
PWY-5505 | L-glutamate and L-glutamine biosynthesis | 0.009 |
ARGORNPROST-PWY | Arginine, ornithine and proline interconversion | 0.043 |
Metabolomic analysis in PHLF patients
We identified fecal and serum metabolites in ao.PHLF vs. bo.PHLF. From the fecal metabolites, 859 characteristics of negative ion mode (NIM) and 1,802 of positive ion mode (PIM) were identified. Of these, the 156 (20 upregulated and 136 downregulated) and 430 (54 upregulated and 376 downregulated) types of metabolites of NIM and PIM, respectively, differed significantly (see Table S1). In relation to the serum metabolites under NIM and PIM, we identified 431 characteristics with 160 metabolite types (45 upregulated and 115 downregulated) and 765 characteristics with 201 metabolite types (92 upregulated and 109 downregulated), respectively (see Table S2). For the NIM and the PIM, the differential metabolites from both the feces and serum are shown in Volcano plots (see Figure 4A,4B, and Figure S3A,S3B). From the orthogonal PLS-DA, the metabolic data of both feces and serum showed significant differences in ao.PHLF vs. bo.PHLF under both NIM and PIM (see Figure 4C,4D, and Figure S3C,S3D). The KEGG analysis of differential metabolites in ao.PHLF vs. bo.PHLF indicated that, under the NIM, the most activated BCAA metabolic pathways were involved in valine, leucine, and isoleucine degradation and biosynthesis (see Figure 4E,4F), which were rich in metabolite 3-methyl-2-oxobutanoic acid (see Table 3). A Venn diagram plot of both the fecal and serum differential metabolites identified 13 overlaps, among which 3-methyl-2-oxobutanoic acid showed a downward trend in the ao.PHLF group (see Figure 5A). Conversely, no BACC pathway enrichment was observed in cases of PIM (see Figure S3E,S3F). Collectively, the results showed that the BACC metabolic pathway was affected by hepatectomy, and hub-metabolite 3-methyl-2-oxobutanoic acid was significantly suppressed in both the feces and serum.
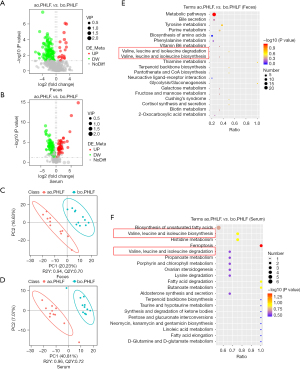
Table 3
Pathway | Fecal metabolites | Serum metabolites |
---|---|---|
Valine, leucine and isoleucine biosynthesis | 3-methyl-2-oxobutanoic acid (down) | 3-methyl-2-oxobutanoic acid (down) |
2-Oxobutyric acid (down) | ||
CCitraconic acid (up) | ||
Valine, leucine and isoleucine degradation | 3-methyl-2-oxobutanoic acid (down) | 3-methyl-2-oxobutanoic acid (down) |
Acetoacetate (down) |
NIM, Negative Ion Mode.
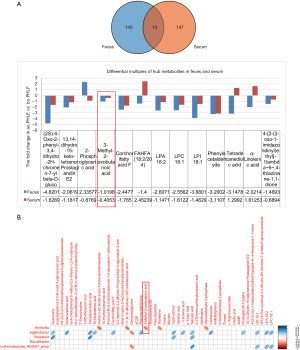
Relationship between 3-methyl-2-oxobutanoic acid and Klebsiella
A Spearman correlation analysis was then conducted to discover the top 20 differential metabolites, hub metabolites, pathway-enriched differential metabolites, and the 5 differential gut microbial taxonomies at the genus level. The results showed that significantly enriched 3-methyl-2-oxobutanoic acid on the BCAA pathway was negatively linked to Klebsiella under the NIM (P=0.02, r=0.51; see Figure 5B).
Discussion
There is growing evidence of the correlation between gut microbiota and multiple liver diseases. However, changes in the intestinal flora of B-HCC patients before and after surgery, and the changes effect on the disease, had not previously been examined. In the present study, PHLF was found to be associated with Klebsiella conditions, while Klebsiella appears to acts on liver functions via 3-methyl-2-oxobutanoic acid on the BCAA metabolic pathway.
We found that the gut microbial species in B-HCC patients did not differ greatly before or after hepatectomy, while microbial abundance at different levels showed significant changes, especially at the genus level. This reflects the imbalance of the gut microbiome post-hepatectomy. Notably, the imbalance affected microbial abundance rather than community diversity, which might be related to the short time intervals of fecal sample collecting. To some extent, the bacterial genus of superior abundance is irreplaceable in disease development (33). Our findings are consistent with those of previous studies using mouse models of hepatectomy (32,33). Previous research has reported that long-term exposure to exogenous chemical materials or drug stimulations may increases the risk of microbial imbalance, which is largely reflected in elevated Bacteroides genera and is accompanied by damaged intestinal mucosal barrier functions—a cause of aggravated liver impairment and even liver failure (42,43). Acute liver failure by decompensated cirrhosis has been reported as a result of abundant enterococcus and Peptostreptococcus (22). In the present study, unlike previous research, we found that based on the phenomenon of the change of intestinal flora before and after surgery, the correlation between PHLF and a significantly increasing Klebsiella. We also explored PHLF further.
We found that Klebsiella, which is 1 of the top 10 bacteria that significantly changed, was highly abundant in all 29 patients after hepatectomy. Before hepatectomy, there was no significant difference in Klebsiella between Groups A and B. Post-operation, both groups showed elevated Klebsiella abundance, and the elevation in Group A reached statistical significance. Klebsiella belongs to the Enterobacteriaceae family, which is associated with multiple clinical diseases, including pneumonia, pyogenic infection, meningitis, and liver abscesses (44,45). It is characterized by a strong pathogenicity (46), which is a risk factor for severe inflammatory responses (47). Thus, we reasoned that PHLF may be associated with the remarkable alteration of Klebsiella. Gut microbiota was once studied as a potential non-invasive biomarker for liver cancer diagnosis (48,49). The considerable changes of the Klebsiella genus may indicate the occurrence of liver failure post-operation; thus, it has the potential to predict the risk of surgery-induced liver failure. Additionally, there were no statistical differences in relation to the clinical baseline characteristics and microbiome diversity before surgery between Groups A and B. However, diverse outcomes in the presence and absence of PHLF resulted, which might be associated with various mechanisms; thus, the role of gut microbes is highly important and cannot be negated (50). In the future, the mechanism underlying Klebsiella changes and the occurrence of PHLF needs to be further investigated.
Gut microbiome and metabolites, other signals and nutrients by the presence of single bacterium, the bacterium-host axis can interact with each other, or by the microbial metabolite-host axis to be delivered to the liver under portal circulation (gut-liver axis) to further act on liver functions (51,52). Our research found that Klebsiella abundance differed greatly in patients with PHLF before and after hepatectomy, and was significantly associated with multiple amino-acid pathways and the BCAA metabolic pathway. As reported, as a result of decreased oxidative stress and inflammatory responses BCAA may benefit liver regeneration, and improve nutrient status and liver cell damage repair (32). In the present study, the functions of the BCAA metabolic pathway were weakened in patients with PHLF after surgery, who also had a greatly increased abundance of Klebsiella, which suggests Klebsiella is involved in the suppression of BCAA metabolic pathways.
When detecting the metabolite information in feces and blood, the comprehensive omics analysis found that compared with ao.PHLF vs. bo.PHLF group, in the NIM, Klebsiella and 3-methyl-2-oxobutanoic acid (pivotal metabolites with the host feces, and blood) is negatively correlated in the branched-chain amino acid pathway; that is, when Klebsiella increased, 3-methyl-2-oxobutanoic acid significantly decreased. This indicates that the functions of Klebsiella in PHLF pathogenesis are potentially realized by participation in fecal and serum metabolism in the BCAA pathway. 3-methyl-2-oxobutanoic acid is a kind of bifunctional compound and a type of branched-chain ketones acid (BCKA) (53,54) with strong reactivity, and can be used as an active intermediate product to function on BCAA metabolism (55,56). 3-methyl-2-oxobutanoic acid and its calcium salt derivative could be applied in medicine to combat chronic renal failure, as they produce a variety of benefits, including decreasing the accumulation of urea nitrogen and uremia toxicity and improving uremia symptoms, by the assembly of necessitate amino acid following transamination (57). As reported, BCAA and its metabolite BCKA are both key mediators during metabolism. They can be mutually transferred due to a reversible ammonia reaction (55,58) by a specific reversible enzyme with high reactivity (59). Additionally, the intake of all types of BCKA is completed in the liver where metabolism circulation occurs (60). Moreover, BCAA transaminase was observed to be highly activated in rats with acute liver injury (61). Given the above findings, we hypothesized that a significant increase of Klebsiella on the BCAA metabolic pathway potentially affects the activities of key enzymes or key enzyme genes related to 3-methyl-2-oxobutanoic acid. Additionally, Klebsiella metabolites may degrade 3-methyl-2-oxobutanoic acid or suppress transamination, causing poor BCAA transformation, which may weaken the protection against post-operative liver injury, and lead to an increased risk of liver failure. So alterations in the Klebsiella may explain its role in the pathogenesis of PHLF. In future studies, we will explore the specific mechanisms of how Klebsiella and 3-methyl-2-oxobutanoic acid on the BCAA metabolic pathway affects PHLF.
This study had a number of limitations. First, it did not examine the severity grading of patients with PHLF. Second, it was a single-center study. Multi-center studies need to be conducted with more subjects to determine the generalizability of the present findings. Targeted metabonomics, metagenomics, tissue total transcriptomics, and FMT need to be further investigated and the correlation between Klebsiella and liver failure needs to be validated. Moreover, a time-based comparative analysis will be conducted of fecal/serum samples from an earlier period post-hepatectomy, in hopes of finding Klebsiella changes in early samples and determine if it is reliable predictive marker for PHLF. The relationship between Klebsiella and 3-methyl-2-oxobutanoic acid will be also validated with bacterial strains, which may help in the application of 3-methyl-2-oxobutanoic acid and its derivative in the treatment of PHLF.
Conclusions
In sum, this study examined the relationship between gut microbiota and metabolites in patients with PHLF and proposed a potential mechanism by which Klebsiella aggravates liver injury by alterations in 3-methyl-2-oxobutanoic acid shared by fecal and serum metabolites. This is the first study to propose that Klebsiella plays a vital role in PHLF and to note the potential of 3-methyl-2-oxobutanoic acid as a therapeutic target.
Acknowledgments
Funding: This study was supported by the National Nature Science Foundation of China (Grant/Award Number: 81960534), the National Nature Science Foundation of China (Grant/Award Number: 81972306), the Key laboratory of High-Incidence-Tumor Prevention and Treatment (Guangxi Medical University), and the Ministry of Education (GKE-ZZ202008).
Footnote
Reporting Checklist: The authors have completed the MDAR reporting checklist. Available at https://jgo.amegroups.com/article/view/10.21037/jgo-21-906/rc
Data Sharing Statement: Available at https://jgo.amegroups.com/article/view/10.21037/jgo-21-906/dss
Conflicts of Interest: All authors have completed the ICMJE uniform disclosure form (available at https://jgo.amegroups.com/article/view/10.21037/jgo-21-906/coif). The authors have no conflicts of interest to declare.
Ethical Statement: The authors are accountable for all aspects of the work in ensuring that questions related to the accuracy or integrity of any part of the work are appropriately investigated and resolved. The study followed the ethical guidelines of the Helsinki Declaration (as revised in 2013). The protocol for this study was approved by the ethics committee of the Guangxi Medical University Cancer Hospital (No. KY2019009). All patients signed informed consent forms and agreed to their anthropometric data being used in the analysis.
Open Access Statement: This is an Open Access article distributed in accordance with the Creative Commons Attribution-NonCommercial-NoDerivs 4.0 International License (CC BY-NC-ND 4.0), which permits the non-commercial replication and distribution of the article with the strict proviso that no changes or edits are made and the original work is properly cited (including links to both the formal publication through the relevant DOI and the license). See: https://creativecommons.org/licenses/by-nc-nd/4.0/.
References
- Anwanwan D, Singh SK, Singh S, et al. Challenges in liver cancer and possible treatment approaches. Biochim Biophys Acta Rev Cancer 2020;1873:188314. [Crossref] [PubMed]
- Wallace MC, Preen D, Jeffrey GP, et al. The evolving epidemiology of hepatocellular carcinoma: a global perspective. Expert Rev Gastroenterol Hepatol 2015;9:765-79. [Crossref] [PubMed]
- Tan AT, Schreiber S. Adoptive T-cell therapy for HBV-associated HCC and HBV infection. Antiviral Res 2020;176:104748. [Crossref] [PubMed]
- Tang A, Hallouch O, Chernyak V, et al. Epidemiology of hepatocellular carcinoma: target population for surveillance and diagnosis. Abdom Radiol (NY) 2018;43:13-25. [Crossref] [PubMed]
- Anstee QM, Reeves HL, Kotsiliti E, et al. From NASH to HCC: current concepts and future challenges. Nat Rev Gastroenterol Hepatol 2019;16:411-28. [Crossref] [PubMed]
- Tanaka M, Katayama F, Kato H, et al. Hepatitis B and C virus infection and hepatocellular carcinoma in China: a review of epidemiology and control measures. J Epidemiol 2011;21:401-16. [Crossref] [PubMed]
- Craig AJ, von Felden J, Garcia-Lezana T, et al. Tumour evolution in hepatocellular carcinoma. Nat Rev Gastroenterol Hepatol 2020;17:139-52. [Crossref] [PubMed]
- Couri T, Pillai A. Goals and targets for personalized therapy for HCC. Hepatol Int 2019;13:125-37. [Crossref] [PubMed]
- Chen H, Jia W. Progress in hepatectomy for hepatocellular carcinoma and peri-operation management. Genes Dis 2020;7:320-7. [Crossref] [PubMed]
- Aramaki O, Takayama T, Higaki T, et al. Decreased blood loss reduces postoperative complications in resection for hepatocellular carcinoma. J Hepatobiliary Pancreat Sci 2014;21:585-91. [Crossref] [PubMed]
- Mullen JT, Ribero D, Reddy SK, et al. Hepatic insufficiency and mortality in 1,059 noncirrhotic patients undergoing major hepatectomy. J Am Coll Surg 2007;204:854-64. [Crossref] [PubMed]
- Rahbari NN, Garden OJ, Padbury R, et al. Posthepatectomy liver failure: a definition and grading by the International Study Group of Liver Surgery (ISGLS). Surgery 2011;149:713-24. [Crossref] [PubMed]
- Mitev K, Taleski V. Association between the Gut Microbiota and Obesity. Open Access Maced J Med Sci 2019;7:2050-6. [Crossref] [PubMed]
- Cani PD, Van Hul M, Lefort C, et al. Microbial regulation of organismal energy homeostasis. Nat Metab 2019;1:34-46. [Crossref] [PubMed]
- Lee G, You HJ, Bajaj JS, et al. Distinct signatures of gut microbiome and metabolites associated with significant fibrosis in non-obese NAFLD. Nat Commun 2020;11:4982. [Crossref] [PubMed]
- Krishnan S, Ding Y, Saedi N, et al. Gut Microbiota-Derived Tryptophan Metabolites Modulate Inflammatory Response in Hepatocytes and Macrophages. Cell Rep 2018;23:1099-111. [Crossref] [PubMed]
- Fei N, Bruneau A, Zhang X, et al. Endotoxin Producers Overgrowing in Human Gut Microbiota as the Causative Agents for Nonalcoholic Fatty Liver Disease. mBio 2020;11:03263-19. [Crossref] [PubMed]
- Ciocan D, Voican CS, Wrzosek L, et al. Bile acid homeostasis and intestinal dysbiosis in alcoholic hepatitis. Aliment Pharmacol Ther 2018;48:961-74. [Crossref] [PubMed]
- Tilg H, Mathurin P. Altered intestinal microbiota as a major driving force in alcoholic steatohepatitis. Gut 2016;65:728-9. [Crossref] [PubMed]
- Burns GS, Thompson AJ. Viral hepatitis B: clinical and epidemiological characteristics. Cold Spring Harb Perspect Med 2014;4:a024935. [Crossref] [PubMed]
- Wang J, Wang Y, Zhang X, et al. Gut Microbial Dysbiosis Is Associated with Altered Hepatic Functions and Serum Metabolites in Chronic Hepatitis B Patients. Front Microbiol 2017;8:2222. [Crossref] [PubMed]
- Solé C, Guilly S, Da Silva K, et al. Alterations in Gut Microbiome in Cirrhosis as Assessed by Quantitative Metagenomics: Relationship With Acute-on-Chronic Liver Failure and Prognosis. Gastroenterology 2021;160:206-218.e13. [Crossref] [PubMed]
- Chen Z, Xie Y, Zhou F, et al. Featured Gut Microbiomes Associated With the Progression of Chronic Hepatitis B Disease. Front Microbiol 2020;11:383. [Crossref] [PubMed]
- Wang R, Tang R, Li B, et al. Gut microbiome, liver immunology, and liver diseases. Cell Mol Immunol 2021;18:4-17. [Crossref] [PubMed]
- Zheng R, Wang G, Pang Z, et al. Liver cirrhosis contributes to the disorder of gut microbiota in patients with hepatocellular carcinoma. Cancer Med 2020;9:4232-50. [Crossref] [PubMed]
- Nolan JP. The role of intestinal endotoxin in liver injury: a long and evolving history. Hepatology 2010;52:1829-35. [Crossref] [PubMed]
- Tripathi A, Debelius J, Brenner DA, et al. The gut-liver axis and the intersection with the microbiome. Nat Rev Gastroenterol Hepatol 2018;15:397-411. [Crossref] [PubMed]
- Tao X, Wang N, Qin W. Gut Microbiota and Hepatocellular Carcinoma. Gastrointest Tumors 2015;2:33-40. [Crossref] [PubMed]
- Fukui H. Role of Gut Dysbiosis in Liver Diseases: What Have We Learned So Far? Diseases 2019;7:58. [Crossref] [PubMed]
- Huang H, Sun Z, Pan H, et al. Serum metabolomic signatures discriminate early liver inflammation and fibrosis stages in patients with chronic hepatitis B. Sci Rep 2016;6:30853. [Crossref] [PubMed]
- Oh TG, Kim SM, Caussy C, et al. A Universal Gut-Microbiome-Derived Signature Predicts Cirrhosis. Cell Metab 2020;32:878-888.e6. [Crossref] [PubMed]
- Cornide-Petronio ME, Álvarez-Mercado AI, Jiménez-Castro MB, et al. Current Knowledge about the Effect of Nutritional Status, Supplemented Nutrition Diet, and Gut Microbiota on Hepatic Ischemia-Reperfusion and Regeneration in Liver Surgery. Nutrients 2020;12:284. [Crossref] [PubMed]
- Bao Q, Yu L, Chen D, et al. Variation in the gut microbial community is associated with the progression of liver regeneration. Hepatol Res 2020;50:121-36. [Crossref] [PubMed]
- Magoč T, Salzberg SL. FLASH: fast length adjustment of short reads to improve genome assemblies. Bioinformatics 2011;27:2957-63. [Crossref] [PubMed]
- Caporaso JG, Kuczynski J, Stombaugh J, et al. QIIME allows analysis of high-throughput community sequencing data. Nat Methods 2010;7:335-6. [Crossref] [PubMed]
- Rognes T, Flouri T, Nichols B, et al. VSEARCH: a versatile open source tool for metagenomics. PeerJ 2016;4:e2584. [Crossref] [PubMed]
- Haas BJ, Gevers D, Earl AM, et al. Chimeric 16S rRNA sequence formation and detection in Sanger and 454-pyrosequenced PCR amplicons. Genome Res 2011;21:494-504. [Crossref] [PubMed]
- Wang Q, Garrity GM, Tiedje JM, et al. Naive Bayesian classifier for rapid assignment of rRNA sequences into the new bacterial taxonomy. Appl Environ Microbiol 2007;73:5261-7. [Crossref] [PubMed]
- Edgar RC. UPARSE: highly accurate OTU sequences from microbial amplicon reads. Nat Methods 2013;10:996-8. [Crossref] [PubMed]
- Douglas GM, Maffei VJ, Zaneveld JR, et al. PICRUSt2 for prediction of metagenome functions. Nat Biotechnol 2020;38:685-8. [Crossref] [PubMed]
- Want EJ, Masson P, Michopoulos F, et al. Global metabolic profiling of animal and human tissues via UPLC-MS. Nat Protoc 2013;8:17-32. [Crossref] [PubMed]
- Wang G, Pan R, Liang X, et al. Perfluorooctanoic acid-induced liver injury is potentially associated with gut microbiota dysbiosis. Chemosphere 2021;266:129004. [Crossref] [PubMed]
- Chen Q, Wang Y, Jiao F, et al. Betaine inhibits Toll-like receptor 4 responses and restores intestinal microbiota in acute liver failure mice. Sci Rep 2020;10:21850. [Crossref] [PubMed]
- Lin YC, Lu MC, Lin C, et al. Activation of IFN-γ/STAT/IRF-1 in hepatic responses to Klebsiella pneumoniae infection. PLoS One 2013;8:e79961. [Crossref] [PubMed]
- Zhang S, Zhang X, Wu Q, et al. Clinical, microbiological, and molecular epidemiological characteristics of Klebsiella pneumoniae-induced pyogenic liver abscess in southeastern China. Antimicrob Resist Infect Control 2019;8:166. [Crossref] [PubMed]
- Siu LK, Yeh KM, Lin JC, et al. Klebsiella pneumoniae liver abscess: a new invasive syndrome. Lancet Infect Dis 2012;12:881-7. [Crossref] [PubMed]
- Atarashi K, Suda W, Luo C, et al. Ectopic colonization of oral bacteria in the intestine drives TH1 cell induction and inflammation. Science 2017;358:359-65. [Crossref] [PubMed]
- Ren Z, Li A, Jiang J, et al. Gut microbiome analysis as a tool towards targeted non-invasive biomarkers for early hepatocellular carcinoma. Gut 2019;68:1014-23. [Crossref] [PubMed]
- Huang H, Ren Z, Gao X, et al. Integrated analysis of microbiome and host transcriptome reveals correlations between gut microbiota and clinical outcomes in HBV-related hepatocellular carcinoma. Genome Med 2020;12:102. [Crossref] [PubMed]
- Jama HA, Fiedler A, Tsyganov K, et al. Manipulation of the gut microbiota by the use of prebiotic fibre does not override a genetic predisposition to heart failure. Sci Rep 2020;10:17919. [Crossref] [PubMed]
- Usami M, Miyoshi M, Yamashita H. Gut microbiota and host metabolism in liver cirrhosis. World J Gastroenterol 2015;21:11597-608. [Crossref] [PubMed]
- Chen P. Gut Microbiota and Pathogenesis of Organ Injury. Adv Exp Med Biol 2020; [Crossref]
- de la Plaza M, Fernández de Palencia P, Peláez C, et al. Biochemical and molecular characterization of alpha-ketoisovalerate decarboxylase, an enzyme involved in the formation of aldehydes from amino acids by Lactococcus lactis. FEMS Microbiol Lett 2004;238:367-74. [PubMed]
- Yudkoff M, Daikhin Y, Nissim I, et al. Brain amino acid requirements and toxicity: the example of leucine. J Nutr 2005;135:1531S-8S. [Crossref] [PubMed]
- Harper AE, Miller RH, Block KP. Branched-chain amino acid metabolism. Annu Rev Nutr 1984;4:409-54. [Crossref] [PubMed]
- Koketsu K, Mitsuhashi S, Tabata K. Identification of homophenylalanine biosynthetic genes from the cyanobacterium Nostoc punctiforme PCC73102 and application to its microbial production by Escherichia coli. Appl Environ Microbiol 2013;79:2201-8. [Crossref] [PubMed]
- Miller RH, Harper AE. Metabolism of valine and 3-methyl-2-oxobutanoate by the isolated perfused rat kidney. Biochem J 1984;224:109-16. [Crossref] [PubMed]
- Schauder P, Schroder K, Herbertz L, et al. Oral administration of alpha-ketoisovaleric acid or valine in humans: blood kinetics and biochemical effects. J Lab Clin Med 1984;103:597-605. [PubMed]
- Sivanand S, Vander Heiden MG. Emerging Roles for Branched-Chain Amino Acid Metabolism in Cancer. Cancer Cell 2020;37:147-56. [Crossref] [PubMed]
- Ten Have GAM, Jansen L, Schooneman MG, et al. Metabolic flux analysis of branched-chain amino and keto acids (BCAA, BCKA) and β-hydroxy β-methylbutyric acid across multiple organs in the pig. Am J Physiol Endocrinol Metab 2021;320:E629-E640. [Crossref] [PubMed]
- Kobayashi Y, Miyazawa H, Sasanuma A, et al. Changes in activity levels and isozyme patterns of isoleucine aminotransferase in response to experimentally induced hepatic lesion. Biochem Pharmacol 1995;49:1331-4. [Crossref] [PubMed]