Identification of lncRNAs based on different patterns of immune infiltration in gastric cancer
Introduction
Since the last century, gastric cancer (GC) has been one of the most common cancers in the US and worldwide, and is the most common cancer in East Asia. GC is also the second leading cause of cancer-related deaths worldwide (1). Adenocarcinoma accounts for about 90% of all GC cases, originating in the superficial glands or mucous membranes of the stomach. Thus, unless otherwise noted, discussions of GC are primarily concerned with adenocarcinoma (2). However, other kinds of cancer are also derived from the stomach, including leiomyosarcoma (derived from the muscles surrounding the mucous membrane) and mucosa-associated lymphoid tissue lymphoma (derived from gastric lymphoid tissue) (3). Specifically, since the 1970s, the relative 5-year survival rate for GC has markedly increased, rising from 15% in 1975 to 29% in 2009 in the US, though the rate of survival is still low. In the majority of countries, except for Japan, the rate is approximately 20%, and according to reports, the 5-year survival rate of stage I and II GC is more than 70% (4). Surgical treatment is the first choice for advanced GC. For some patients who do not have the opportunity to undergo surgery, extending the survival period and improving the living conditions (5). Therefore, there is an urgent need to find new tumor prognostic indicators to predict the prognosis of GC patients.
At present, tumor immunity has become a research hotspot because of its unique clinical effect on many kinds of cancers. As a new therapeutic method, immunotherapy has achieved good results in the treatment of many tumors (6,7). Endothelial cells, mesenchymal cells, extracellular matrix molecules, and immune cells constitute the tumor microenvironment. There are numerous inflammatory cell infiltrates in GC (8). Different immune cells play different roles in tumors. It has been shown that the density of CD8+ T cells in GC (cytotoxic T cells) is significantly related to the prognosis of GC, and the higher the density, the worse the prognosis (9). Studies have also shown that tumor-associated macrophages in GC are closely related to immune evasion and are strongly associated with GC prognosis (10). However, the specific mechanisms underlying the actions of immune cells in tumors need to be further explored.
In recent decades, molecular biology research has mostly paid attention to protein-coding genes and their critical genomic diversification in GC pathogenesis (11,12). However, protein-coding genes make up less than 2% of the total genome sequence, and the remaining non-coding genes are transcribed as non-coding RNAs (ncRNAs) (13). According to their size, ncRNAs fall into two main categories: long non-coding RNAs (lncRNAs, >200 nucleotides) and small ncRNAs [18–200 nucleotides, such as microRNAs (miRNAs) and small interfering RNAs] (14). According to the latest evidence of the pathogenesis of cancer, ncRNAs exert significant functions, offering a fresh perspective on the biology of GC. LncRNAs are abnormally expressed in GC (15), esophageal cancer (16), liver cancer (17), and other cancers (18). LncRNAs play a significant regulatory role in migration, apoptosis, and cell propagation, thereby attracting much attention. A large number of studies have shown that lncRNA plays a very important role in tumor microenvironment. Wilkerson’s study showed that lncRNA could stimulate T to regulate cell differentiation and promote immune escape of liver cancer cells (19). Another study showed that hypoxic bladder cancer cells can secrete cancer-rich lncRNA in human serum to reshape tumor microenvironment and promote tumor growth and development, which may be used as diagnostic biomarkers for bladder cancer (20).
In terms of GC, a significant number of lncRNAs are specifically expressed, suggesting that they may serve as biomarkers and may predict clinical outcomes. One paper investigated competing endogenous RNA (CeRNA) regulatory axes associated with immune microenvironment in gastric cancer (GC) (21). In this study, we further explored the impact of lncRNA on prognosis and its correlation with clinical features, and constructed a prognostic model.
At present, a method for lncRNA expression profiling using microarray data has been established. The expression data set of lncRNAs in The Cancer Genome Atlas (TCGA) was analyzed in this study. Through ESTIMATE, single-sample gene set enrichment analysis (ssGSEA), Cox regression analyses, and other approaches, lncRNAs related to tumor phenotypes were screened. The main purpose of this study was to uncover immune-related lncRNAs as novel biomarkers for predicting GC prognosis. We present the following article in accordance with the TRIPOD reporting checklist (available at https://jgo.amegroups.com/article/view/10.21037/jgo-21-833/rc).
Methods
The study was conducted in accordance with the Declaration of Helsinki (as revised in 2013).
Cell lines and culture
Human stomach adenocarcinoma (STAD) cell lines (MKNA, AGS, MGC803) and the normal control cell line (GES-1) were purchased from the American Type Culture Collection (ATCC, Rockville, IN, USA). Cells were cultured in RPMI-1640 (Gibco, MA, USA) supplemented with 10% fetal calf serum and 1% streptomycin and penicillin and maintained at 37 °C under a 5% CO2 atmosphere.
RNA extraction and quantitative real-time PCR
Total RNA was extracted from STAD cell lines (MKNA, AGS, MGC803) and the normal control cell line (GES-1) using Trizol reagent (Invitrogen) according to the manufacturer’s instructions. After synthesizing cDNA, real-time fluorescent quantitative PCR was immediately conducted to detect the expression of BMPER, PRUNE2, and RBPMS2. Glyceraldehyde-3-phosphate dehydrogenase (GAPDH) was used as an endogenous reference. The primers used in this experiment were as follows: GAPDH forward, 5'-CACCATGAAGATCAAGATCATTGC-3', GAPDH reverse, 5'-GGCCGGACTCATCGTACTCCTGC-3'; BMPER forward, 5'-GGGTGCGCTGTGTTGTTCATT-3', BMPER reverse, 5'-CTAAGGTGCTGGGGACAGGAG-3'; PRUNE2 forward, 5'-CAGTTCAGTGCTCAGGGTTT-3', PRUNE2 reverse, 5'-CCAAACTTGTCTGTAAATGCTT-3'; RBPMS2 forward, 5'-CTCCCATGCTGCGTTCA-3', RBPMS2 reverse, 5'-GGGTGGTGTCAGAGGAAG-3'. The levels of BMPER, PRUNE2, and RBPMS2 were calculated with the comparative quantification cycle (Cq) method (2-ΔΔCq) (Table S1).
Data retrieval and pre-processing
From the TCGA database, transcriptome data [fragments per kilobase million (FPKM) value] of STAD (32 normal cases and 375 tumor samples) were downloaded and converted into transcripts per kilobase million (TPM) data.
Tumor immune infiltration and cluster analysis
We used ssGSEA to evaluate the enrichment of different types of immune cell-related gene sets in each tumor sample to determine the degree of tumor immune cell infiltration. The enriched components obtained from ssGSEA were used for unsupervised clustering of tumor samples from patients with GC, and two different subtypes were obtained based on immune cell infiltration. We performed the above steps using the ConsensuClusterPlus package in R (19).
We used the ESTIMATE algorithm in R to calculate the ImmuneScore, ESTIMATEScore, and StromalScore of each tumor sample. The difference between PD-L1 (CD274) and CTLA4 in the two subtypes was also calculated. We used ssGSEA to obtain the enriched components of different immune cell gene sets and explored whether the two subtypes were significantly different from each other. According to the degree of immune infiltration, we divided different clusters into the immune-inflammatory type and immune desert type.
Unsupervised clustering of differential lncRNAs
We analyzed the differences in lncRNAs between every 2 clusters, and adjusted P<0.05 and log |fold change| >2 were considered as the thresholds for differential gene expression. A total of 425 lncRNAs were found as the characteristic lncRNAs of different immune subtypes. Then, univariate Cox analysis was performed for these differential lncRNAs. Of these lncRNAs, we found a total of 57 lncRNAs with prognostic value (P<0.05). Based on the expression of the 57 lncRNAs, we applied unsupervised cluster analysis to distinguish these patterns and categorize patients for further analysis.
Construction of a competitive endogenous RNA (ceRNA) network and functional annotation
In order to further explore the biological roles of the differential lncRNAs, we further identified differential mRNAs in the two immune subtypes. We found 1,601 differential genes using adjusted P value <0.05 and |FC| >2 as thresholds. Then, we searched the miRNAs targeted by these different lncRNAs through the miRcode database (http://www.mircode.org/) (20). The mRNAs targeted by these miRNAs in the miRDB (21), miRTarBase (22), and TargetScan (23) databases were intersected with the differential mRNAs of the immune subtypes, and a total of 44 mRNAs were obtained. We used these lncRNAs, miRNAs, and mRNAs to construct a ceRNA network. To determine the functional annotations associated with the 44 mRNAs, we used the Metascape database to analyze the enrichment of these mRNAs (24).
Survival analysis
To evaluate the overall survival (OS) and expression relationship of the 57 differential lncRNAs (univariate Cox, P<0.05), we performed multivariate analysis to construct a prognostic model using the prognostic value. The model for prognostic prediction was developed as described below, using the coefficient and prognostic lncRNA expression level based on multiple regression analysis:
Where for the GC patient, risk score (patient) represented the prognostic risk score, lncRNAi represented the prognostic lncRNA, and expr (lncRNAi) represented the lncRNAi expression level. The coefficient (lncRNAi) represented the lncRNAi contribution to the prognostic risk score, which was acquired by the regression coefficient from multivariate Cox analysis. We divided patients into low and high-risk groups, and used the median score of patients in the training set as the risk threshold. To assess the difference in survival between the two groups, we used the Kaplan-Meier (KM) method. For assessing the independence of the risk score from the other clinical factors, stratified analyses were conducted. To assess the risk score performance, a time-dependent receiver operating characteristic (ROC) curve was generated.
Nomogram
This study applied the Cox regression algorithm to predict the survival rate of patients using indicators such as gender, age, T stage, risk score, and N stage. We also generated the calibration curve of the prediction model to assess the prediction effect of the nomogram.
Statistical analysis
R version 4.0.3 and the R software package “survival” were used for all statistical analyses. This study used the log-rank test and KM analysis to evaluate survival and compare survival differences between clusters and risk groups. P<0.05 was considered as statistically significant.
Results
The landscape of immune cell infiltration in STAD
First, the data of 375 GC samples from TCGA were collected. We then applied ssGSEA to sequencing data from GC samples to assess immune cell infiltration. Lastly, the abundance and types of 23 immune-related cells from GC samples were determined (https://cdn.amegroups.cn/static/public/jgo-21-833-1.xlsx). The STAD cohort was divided into different groups based on the ConsensusClusterPlus R package. When the uniform matrix K value was 2, the crossover between STAD samples was minimal (Figure 1A-1C). As shown in Figure 1D, GC samples, based on immune infiltration, were divided into a low immune cell infiltration cluster (n=160) and a high immune cell infiltration cluster (n=215).
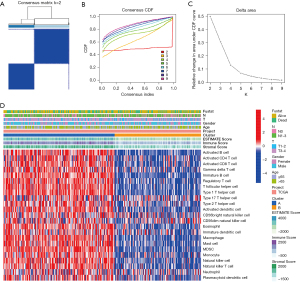
The enriched fractions of different immune cell sets obtained by ssGSEA between the two subtypes were significantly different (Figure 2A). In cluster A, several immune cells were significantly higher compared to cluster B. In order to verify the above clustering results, the ESTIMATEScore, ImmuneScore, and StromalScore were calculated for each tumor sample. The results showed that cluster A had a higher ESTIMATEScore, ImmuneScore, and StromalScore than cluster B (Figure 2B-2D; all P<0.05). Since tumor purity was negatively correlated with ESTIMATEScore, we determined that tumor purity was higher in cluster B. There were also significant differences between PD-L1 (CD274) and CTLA4 expression in the two subtypes (Figure 2E). Therefore, we speculated that cluster A was of the tumor immune-inflammatory type and cluster B was of the immune desert type. These results further suggest that cluster A has a better effect on immunotherapy.
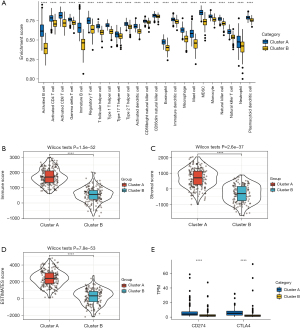
Unsupervised clustering of differential lncRNAs between high and low immune cell infiltration clusters
We analyzed the differentially expressed lncRNAs between every 2 clusters by setting thresholds of adjusted P<0.05 and |FC| >2. There were 425 lncRNAs that were the characteristic lncRNAs of different immune subtypes (Figure 3A). Univariate Cox analysis was conducted for these differential lncRNAs, and 57 lncRNAs were found to have prognostic value (P<0.05). According to the expression of these 57 lncRNAs, we applied unsupervised cluster analysis to confirm these patterns and categorize patients for further analysis. As shown in Figure 3B, we used the unsupervised hierarchical clustering algorithm to separate GC samples into 2 clusters (gene cluster A and B) based on the expression of 57 lncRNAs. Then, we performed prognostic analysis, and the results showed that gene cluster A had a worse prognosis than gene cluster B (Figure 3C; P=0.021). Figure 3D shows the correlations between different clusters and clinical characteristics such as sex, age, T stage, and N stage, among others. The enrichment of different immune cell gene sets between the 2 gene clusters obtained by ssGSEA was also significantly different (Figure 3E). The results showed that gene cluster A had higher enrichment of activated B cells, activated CD8 T cells, gamma delta T cells, immature B cells, regulatory T cells, T follicular helper cells, type I T helper cells, type 2 T helper cells, activated dendritic cells, eosinophils, immature dendritic cells, macrophages, mast cells, myeloid-derived suppressor cells, monocytes, natural killer cells, natural killer T cells, and plasmacytoid dendritic cells, but had lower enrichment of type 17 T helper cells, CD56 dim natural killer cells, and neutrophils than gene cluster B. Furthermore, there were no differences in activated CD4 T cells and CD56 bright natural killer cells between gene cluster A and B. The difference in the distribution of immune cells between gene cluster A and B may reveal the difference in prognosis between these groups.
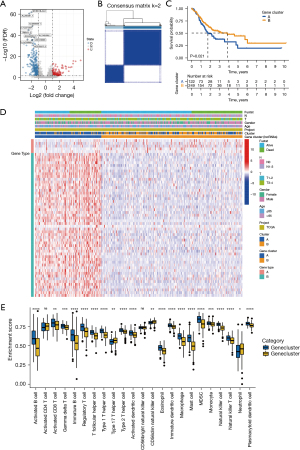
Construction of a ceRNA network and functional annotation
As shown in Figure 4A, we further identified 1,601 differential mRNAs in the two immune subtypes by using adjusted P value <0.05 and |FC| >2 as thresholds. Then, we searched the miRNAs targeted by these different lncRNAs through the miRcode database. The mRNAs targeted by these miRNAs in the miRDB, miRTarBase, and TargetScan databases were intersected with the differential mRNAs, and 44 mRNAs were obtained. We used these lncRNAs, miRNAs, and mRNAs to construct a ceRNA network. There were 44 mRNAs, 27 lncRNAs, and 25 miRNAs in total (Figure 4B). We used the Metascape database to analyze the biological roles of these mRNAs. As shown in Figure 4C, these genes correlated with pathways in cancer, interleukin-4 and interleukin-13 signaling, and negative regulation of BMP signaling pathway.
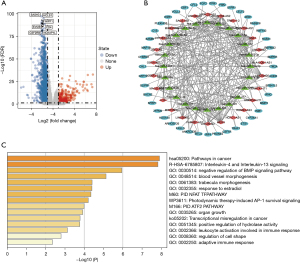
BMPER, PRUNE2, and RBPMS2 expression in STAD cell lines in vitro
We detected the relative mRNA expression levels of BMPER, PRUNE2, and RBPMS2 in 3 human STAD cell lines (MKNA, AGS, and MGC803). We used the normal cell line GES-1 as the control. The mRNA expression levels of BMPER, PRUNE2, and RBPMS2 were significantly decreased in all STAD cell lines compared with the GES-1 cell line (***P<0.001, Figure 5A,5B; **P<0.01, Figure 5C). These findings were consistent with the bioinformatics analysis (Figure 5D-5F).
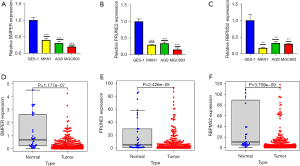
LncRNA signature construction and survival analysis
Univariate regression was used to analyze the prognosis of the TCGA cohort according to the prognostic lncRNAs. We screened 57 prognostic lncRNAs. Then, we performed multivariate analysis to construct a prognostic model using the 57 lnRNAs. The hazard ratio (HR), 95% confidence interval (CI), and P values for 19 lncRNAs in the multivariate analysis are shown in Table 1. The risk score of each patient was calculated according to the following risk formula: risk score = AC129926.1*0.345432553 + MACORIS*0.378648877 + AL355922.1*0.31149596 + AC008808.1*0.600771598 + LINC01980*0.124498202 + AC087521.1*0.684070491 + AC007277.1*-0.676014589 + AC090825.1*0.39490309 + AC037198.2*-0.333425791 + LINC02677*0.238647705 + ZNF667-AS1*-0.349806418 + GACAT3*0.333015708 + AP001189.1*-0.46590347 + AC110995.1*0.643141834 + AC037198.1*0.473234061 + LINC01197*0.528838193 + LINC01614*0.239271261 + LINC00900*-0.870425064 + LINC00968*-0.536139139. According to the median cut-off value of the risk score, we divided the patients into low-risk and high-risk groups. We found that the OS of high-risk patients was significantly lower than that of low-risk patients (Figure 6A, P<0.0001). Furthermore, the 1-year area under the curve (AUC) was 0.71, the 3-year area under the curve (AUC) was 0.76, and the 5-year area under the curve (AUC) was 0.78, demonstrating that in predicting the prognosis of GC, lncRNAs had excellent accuracy (Figure 6B). The nomogram model for predicting OS at 1, 3, and 5 years was then generated (Figure 6C). Through the correlation charts we found that the observed 5-year OS and the predicted OS showed ideal agreement (Figure 6D). We verified the expression of representative lnRNAs in gastric cancer tissues and their role in tumor immunity. We found that there were significant differences in the expression and tumor immune infiltration of two lcRNAs compared with normal tissues (Figure S1).
Table 1
ID | coef | HR | HR.95L | HR.95H | P value |
---|---|---|---|---|---|
AC129926.1 | 0.3454326 | 1.4126008 | 1.1181702 | 1.7845593 | 0.003773 |
MACORIS | 0.3786489 | 1.4603102 | 0.9688083 | 2.2011639 | 0.0705124 |
AL355922.1 | 0.311496 | 1.3654663 | 0.9713673 | 1.9194574 | 0.0730101 |
AC008808.1 | 0.6007716 | 1.8235253 | 1.0051238 | 3.3082935 | 0.0480665 |
LINC01980 | 0.1244982 | 1.13258 | 0.98594 | 1.3010298 | 0.0784396 |
AC087521.1 | 0.6840705 | 1.9819288 | 0.9634832 | 4.0769176 | 0.0630446 |
AC007277.1 | −0.676015 | 0.5086401 | 0.3381935 | 0.7649903 | 0.0011684 |
AC090825.1 | 0.3949031 | 1.4842403 | 1.0211328 | 2.157378 | 0.0384936 |
AC037198.2 | −0.333426 | 0.7164651 | 0.4983392 | 1.0300659 | 0.071854 |
LINC02677 | 0.2386477 | 1.2695312 | 0.9751792 | 1.6527316 | 0.0761936 |
ZNF667-AS1 | −0.349806 | 0.7048245 | 0.5302498 | 0.9368747 | 0.0159955 |
GACAT3 | 0.3330157 | 1.3951692 | 1.0558378 | 1.8435571 | 0.0191756 |
AP001189.1 | −0.465903 | 0.6275679 | 0.3418454 | 1.152104 | 0.1328002 |
AC110995.1 | 0.6431418 | 1.9024487 | 1.1197655 | 3.2322045 | 0.0173943 |
AC037198.1 | 0.4732341 | 1.605177 | 1.1119829 | 2.317116 | 0.0115142 |
LINC01197 | 0.5288382 | 1.6969596 | 0.8989362 | 3.203422 | 0.102825 |
LINC01614 | 0.2392713 | 1.2703231 | 1.0808822 | 1.4929663 | 0.0036853 |
LINC00900 | −0.870425 | 0.4187735 | 0.1717268 | 1.0212224 | 0.0556469 |
LINC00968 | −0.536139 | 0.5850025 | 0.3080866 | 1.1108174 | 0.1012689 |
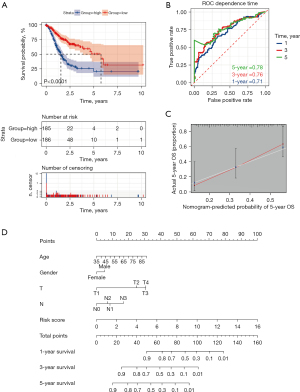
To further determine the independence of the risk score from other clinical characteristics, stratified analysis was performed. We separated the model into high and low-risk groups, and the KM survival curve was drawn for different patients with different clinical states. In different age groups of patients with GC, the survival rate of high-risk patients and low-risk patients was significantly different. At >65 years (Figure 7A, P<0.001) and <65 years (Figure 7B, P<0.001), the survival rate of the low-risk group was significantly higher. The survival rate of females in the low-risk group was significantly higher compared with the high-risk group (Figure 7C, P=0.004), as was the case in men (Figure 7D, P<0.001). In N0 and N1-3 patients at different TNM stages, the survival rate was significantly lower in the high-risk group compared with the low-risk group (Figures 7E,7F, P<0.001), while T1-2 (Figure 7G, P=0.135) showed no statistically significant difference. The survival rate of T3-4 patients in the high-risk group was lower than that of low-risk group (Figure 7H, P<0.001), indicating the high clinical value of this predictive model.
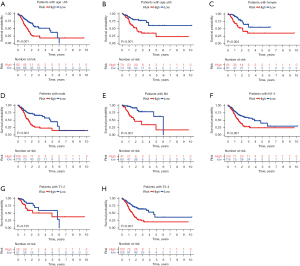
To further clarify the role of the risk scoring model we constructed, we explored the differences among different immune subtypes and genotypes. As shown in Figure 8A,8B, compared with cluster B, the risk score of cluster A was significantly higher (P<0.05). This further explains why gene cluster A had a worse prognosis than gene cluster B. An alluvial diagram was used to visualize the attribute changes of individual patients (Figure 8C).
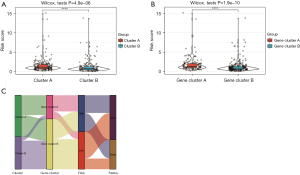
Discussion
As the most frequent and deadly malignant tumor worldwide, GC is highly heterogeneous (25). This heterogeneity is manifested in the tumor cell phenotypes and genotypes and in the microenvironment of the tumor. GC cells and various normal cells constitute GC tissues, such as mesenchymal cells, immune cells, and fibroblasts (26). Therefore, the interaction between tumor-infiltrating immune cells and GC tumor cells is under investigation, which will be useful for studying the mechanism of tumor occurrence and for developing new diagnosis and treatment methods. In this study, transcriptome sequencing data obtained from TCGA and clinicopathological features of GC were used to identify and validate the immune cell infiltration features associated with the 19 prognostic lncRNAs. Many studies have shown that lncRNAs in the tumor microenvironment may help provide new diagnostic and prognostic markers for cancer (21,27). Therefore, lncRNAs have great prospects as prognostic markers in gastric cancer.
The obtained samples were classified into two subtypes, namely cluster A and B, which were shown to have prominent differences in the degree of immune cell infiltration, suggesting that cluster A and cluster B belong to the immune-inflamed type and immune-desert type separately. Compared with cluster B, we found that the enrichment degree of all cluster A immune cells was higher, indicating that cluster A was of the immune-inflammatory type and cluster B was of the immune desert type. Studies have shown that different immune subtypes result in different prognoses (28). We further investigated whether there were significant differences between high and low immune cell infiltrates in terms of StromalScore, ImmuneScore, and ESTIMATEScore. The results showed that all 3 scores were higher in cluster A than in cluster B, indicating that cluster A had higher immune purity and cluster B had higher tumor purity. This suggests that cluster A was more likely to benefit from immunotherapy. PD-L1 and CTLA4, as routine targets for immunotherapy, have made great progress in clinical treatment (29). The findings of this study show that PD-L1 expression and CTLA4 expression in cluster A were higher, suggesting that these patients might be more sensitive to PD-L1 and CTLA4 treatment, which further supported our conclusion.
We divided patients into two groups (gene cluster A and B) based on 57 prognostic lncRNAs. Prognostic analysis showed that gene cluster B had a better prognosis than gene cluster A. The enrichment of different immune cell gene sets obtained by ssGSEA was also significantly different between the two gene clusters. Most of the immune cells were enriched in gene cluster A, which may have contributed to the worse prognosis in gene cluster A. Further enrichment analysis showed that the gene differences are responsible for the negative regulation of tumor pathways, and the interleukin-4, interleukin-13, and BMP signaling pathways.
In recent years, deep transcriptome sequencing research has suggested that most human genome transcripts are non-protein coding genes, containing lncRNAs (30). As a result, we constructed a prognostic model according to the obtained lncRNAs. Based on the model, we divided patients into two groups. The low-risk group showed a significantly higher survival probability. The accuracy of diagnosis suggested by the model was also relatively high and seemed to increase over time, further confirming its reliability. To date, the TNM (tumor-node-metastasis) classification has been the most common guide for the prediction of prognosis, yet a more precise classification is urgently required. In this study, the validation of the prognostic model was verified in groups from different backgrounds, indicating promising potential for clinical use.
At the same time, this study analyzed differentially expressed lncRNAs, mRNAs, and miRNAs in GC and explored their functions. Their interactions were elucidated by constructing a ceRNA network, and three differentially expressed mRNAs of interest were validated by qPCR. The qPCR results were consistent with results of bioinformatics analysis. In a cohort study of 15,651 Chinese patients, Carlevaro-Fita et al. revealed that BMPER can be used as a human longevity gene locus and reduces the risk of many diseases (30). This was consistent with our results, indicating that BMPER can be regarded as a protective factor. In previous studies, a melatonin-attenuated lncRNA was identified as a potential melatonin-regulated oral cancer stimulator (MROS-1). The down-regulation of MROS-1 by melatonin inhibited TPA-induced oral cancer migration by supplementing the protein expression of prune homologue 2 (PRUNE2), which played a tumor inhibitory role in oral cancer. This demonstrated that PRUNE2 is a tumor suppressor gene, which is consistent with our findings (31). GC shows significant biological differences between Asian and non-Asian populations, which makes it difficult to carry out unified prediction measures for all people. Previous researchers have identified new prognostic biomarkers. The prognostic model established by 4 genes (RBPMS2, RGN, PLEKHS, and CT83) was a reliable tool to predict the OS of GC patients in Asia (32), and this was also consistent with our bioinformatics analysis and experimental results.
There are some limitations to our study. Firstly, our prognostic model should be validated in more GC data sets. Secondly, all our results are based on public data sets and need to be verified experimentally.
In summary, this study identified a risk score of 19 lncRNAs as a prognostic marker of GC. These 19 prognostic lncRNAs were related to the infiltration of immune subtypes. In this study, an approach for predicting the prognosis of GC patients was developed, and may provide a new therapeutic target for immunotherapy.
Acknowledgments
Funding: This work was supported by Natural Science Foundation of Liaoning Province (No. 1561449995778) and Hainan Province Clinical Medical Center.
Footnote
Reporting Checklist: The authors have completed the TRIPOD reporting checklist. Available at https://jgo.amegroups.com/article/view/10.21037/jgo-21-833/rc
Data Sharing Statement: Available at https://jgo.amegroups.com/article/view/10.21037/jgo-21-833/dss
Conflicts of Interest: All authors have completed the ICMJE uniform disclosure form (available at https://jgo.amegroups.com/article/view/10.21037/jgo-21-833/coif). The authors have no conflicts of interest to declare.
Ethical Statement: The authors are accountable for all aspects of the work in ensuring that questions related to the accuracy or integrity of any part of the work are appropriately investigated and resolved. The study was conducted in accordance with the Declaration of Helsinki (as revised in 2013).
Open Access Statement: This is an Open Access article distributed in accordance with the Creative Commons Attribution-NonCommercial-NoDerivs 4.0 International License (CC BY-NC-ND 4.0), which permits the non-commercial replication and distribution of the article with the strict proviso that no changes or edits are made and the original work is properly cited (including links to both the formal publication through the relevant DOI and the license). See: https://creativecommons.org/licenses/by-nc-nd/4.0/.
References
- Karimi P, Islami F, Anandasabapathy S, et al. Gastric cancer: descriptive epidemiology, risk factors, screening, and prevention. Cancer Epidemiol Biomarkers Prev 2014;23:700-13. [Crossref] [PubMed]
- Guggenheim DE, Shah MA. Gastric cancer epidemiology and risk factors. J Surg Oncol 2013;107:230-6. [Crossref] [PubMed]
- Song Z, Wu Y, Yang J, et al. Progress in the treatment of advanced gastric cancer. Tumour Biol 2017;39:1010428317714626. [Crossref] [PubMed]
- Correa P. Gastric cancer: overview. Gastroenterol Clin North Am 2013;42:211-7. [Crossref] [PubMed]
- Zhang XY, Zhang PY. Gastric cancer: somatic genetics as a guide to therapy. J Med Genet 2017;54:305-12. [Crossref] [PubMed]
- Gotwals P, Cameron S, Cipolletta D, et al. Prospects for combining targeted and conventional cancer therapy with immunotherapy. Nat Rev Cancer 2017;17:286-301. [Crossref] [PubMed]
- Colli LM, Machiela MJ, Zhang H, et al. Landscape of Combination Immunotherapy and Targeted Therapy to Improve Cancer Management. Cancer Res 2017;77:3666-71. [Crossref] [PubMed]
- Oya Y, Hayakawa Y, Koike K. Tumor microenvironment in gastric cancers. Cancer Sci 2020;111:2696-707. [Crossref] [PubMed]
- Thompson ED, Zahurak M, Murphy A, et al. Patterns of PD-L1 expression and CD8 T cell infiltration in gastric adenocarcinomas and associated immune stroma. Gut 2017;66:794-801. [Crossref] [PubMed]
- Gambardella V, Castillo J, Tarazona N, et al. The role of tumor-associated macrophages in gastric cancer development and their potential as a therapeutic target. Cancer Treat Rev 2020;86:102015. [Crossref] [PubMed]
- Song P, Jiang B, Liu Z, et al. A three-lncRNA expression signature associated with the prognosis of gastric cancer patients. Cancer Med 2017;6:1154-64. [Crossref] [PubMed]
- Sun TT, He J, Liang Q, et al. LncRNA GClnc1 Promotes Gastric Carcinogenesis and May Act as a Modular Scaffold of WDR5 and KAT2A Complexes to Specify the Histone Modification Pattern. Cancer Discov 2016;6:784-801. [Crossref] [PubMed]
- Ferrè F, Colantoni A, Helmer-Citterich M. Revealing protein-lncRNA interaction. Brief Bioinform 2016;17:106-16. [Crossref] [PubMed]
- Sun M, Kraus WL. From discovery to function: the expanding roles of long noncoding RNAs in physiology and disease. Endocr Rev 2015;36:25-64. [Crossref] [PubMed]
- Zhao J, Du P, Cui P, et al. LncRNA PVT1 promotes angiogenesis via activating the STAT3/VEGFA axis in gastric cancer. Oncogene 2018;37:4094-109. [Crossref] [PubMed]
- Zhang H, Hua Y, Jiang Z, et al. Cancer-associated Fibroblast-promoted LncRNA DNM3OS Confers Radioresistance by Regulating DNA Damage Response in Esophageal Squamous Cell Carcinoma. Clin Cancer Res 2019;25:1989-2000. [Crossref] [PubMed]
- Wang H, Huo X, Yang XR, et al. STAT3-mediated upregulation of lncRNA HOXD-AS1 as a ceRNA facilitates liver cancer metastasis by regulating SOX4. Mol Cancer 2017;16:136. [Crossref] [PubMed]
- Huang JZ, Chen M. A Peptide Encoded by a Putative lncRNA HOXB-AS3 Suppresses Colon Cancer Growth. Mol Cell 2017;68:171-184.e6. [Crossref] [PubMed]
- Wilkerson MD, Hayes DN. ConsensusClusterPlus: a class discovery tool with confidence assessments and item tracking. Bioinformatics 2010;26:1572-3. [Crossref] [PubMed]
- Jeggari A, Marks DS, Larsson E. miRcode: a map of putative microRNA target sites in the long non-coding transcriptome. Bioinformatics 2012;28:2062-3. [Crossref] [PubMed]
- Chen Y, Wang X. miRDB: an online database for prediction of functional microRNA targets. Nucleic Acids Res 2020;48:D127-31. [Crossref] [PubMed]
- Huang HY, Lin YC, Li J, et al. miRTarBase 2020: updates to the experimentally validated microRNA-target interaction database. Nucleic Acids Res 2020;48:D148-54. [PubMed]
- Agarwal V, Bell GW, Nam JW, et al. Predicting effective microRNA target sites in mammalian mRNAs. Elife 2015;4:e05005. [Crossref] [PubMed]
- Zhou Y, Zhou B, Pache L, et al. Metascape provides a biologist-oriented resource for the analysis of systems-level datasets. Nat Commun 2019;10:1523. [Crossref] [PubMed]
- Smyth EC, Nilsson M, Grabsch HI, et al. Gastric cancer. Lancet 2020;396:635-48. [Crossref] [PubMed]
- Gao JP, Xu W, Liu WT, et al. Tumor heterogeneity of gastric cancer: From the perspective of tumor-initiating cell. World J Gastroenterol 2018;24:2567-81. [Crossref] [PubMed]
- Liu X, Song Z, Li Y, et al. Integrated genetic analyses revealed novel human longevity loci and reduced risks of multiple diseases in a cohort study of 15,651 Chinese individuals. Aging Cell 2021;20:e13323. [Crossref] [PubMed]
- Zeng D, Li M, Zhou R, et al. Tumor Microenvironment Characterization in Gastric Cancer Identifies Prognostic and Immunotherapeutically Relevant Gene Signatures. Cancer Immunol Res 2019;7:737-50. [Crossref] [PubMed]
- Andrews LP, Yano H, Vignali DAA. Inhibitory receptors and ligands beyond PD-1, PD-L1 and CTLA-4: breakthroughs or backups. Nat Immunol 2019;20:1425-34. [Crossref] [PubMed]
- Carlevaro-Fita J, Liu L, Zhou Y, et al. LnCompare: gene set feature analysis for human long non-coding RNAs. Nucleic Acids Res 2019;47:W523-W529. [Crossref] [PubMed]
- Su SC, Yeh CM, Lin CW, et al. A novel melatonin-regulated lncRNA suppresses TPA-induced oral cancer cell motility through replenishing PRUNE2 expression. J Pineal Res 2021;71:e12760. [Crossref] [PubMed]
- Chen J, Wang A, Ji J, et al. An Innovative Prognostic Model Based on Four Genes in Asian Patient with Gastric Cancer. Cancer Res Treat 2021;53:148-61. [Crossref] [PubMed]
(English Language Editor: J. Gray)