Development and verification of a microsatellite instability-related risk signature for predicting survival and therapy effectiveness in gastric cancer
Introduction
Gastric cancer (GC), which mainly consists of adenocarcinoma, squamous cell carcinoma, adenosquamous carcinoma, and carcinoid tumors, is one of the most common malignancies among digestive system cancers worldwide and is the third major leading cause of death from cancers (1). According to the 2018 global statistics, more than one million people were diagnosed with GC, and 782,685 patients died (1). Although there have been major advances in the treatment of GC using surgical techniques, chemotherapy, and radiotherapy, the survival of GC (especially for patients with advanced GC) remains unsatisfactory due to high mortality rates (2-4). Unfortunately, existing clinical indicators cannot accurately predict the prognosis of GC. Hence, identifying novel and sensitive biomarkers for predicting the prognosis and treatment of GC is of utmost importance.
Microsatellite instability (MSI), a molecular feature of cancer, often occurs when DNA mismatch repair (dMMR) is disrupted (5). Increasing evidence reveals that MSI is common in several cancers, such as esophageal, gastric, colorectal, and endometrial cancers, and is regarded as a promising biomarker for diagnosis and treatment (6). In particular, MSI is considered to be a prognostic factor associated with adjuvant chemotherapy outcomes in colorectal cancer (7). Emerging data reveal that GC patients in The Cancer Genome Atlas (TCGA) can be stratified into different subgroups, including MSI-high (MSI-H) tumors (8,9). More importantly, MSI-H tumors constitute 22% of GC cases in Western countries (8) and have been identified as a separate entity of GC (8,9). However, except for a few prospective data studies, the association between MSI and GC’s clinical features and prognosis remains unexplored (10-12).
Interestingly, recent research has demonstrated that MSI is associated with immunotherapy, especially immune checkpoint blockade (ICB) treatment (13). For example, the dMMR-MSI subtype in colon cancer was shown to benefit from immunotherapy due to a high tumor mutational burden (TMB), infiltration of activated CD8+ cytotoxic T lymphocytes, and activated Th1 cells with (interferon-gamma, IFN-γ) production (14). Moreover, it has been suggested that pembrolizumab can improve the progression-free survival of MSI-H/dMMR metastatic colorectal cancer (15). Furthermore, MSI-H is positively correlated with the expression of PD-L1 (16,17). Microsatellite stability (MSS) colon cancer is not sensitive to ICB treatment due to the lack of immune infiltration and low TMB (16). On the other hand, the tumor microenvironment (TME) of MSI-H in primary colorectal cancer shows a high infiltration of T-helper 1/cytotoxic lymphocytes and a widespread expression of the main immune-checkpoint molecules (18,19). However, the role of MSI in the TME and immunotherapy remains ambiguous.
In this study, we firstly analyzed the correlation between MSI status and survival in GC based on the TCGA database. Next, we assessed the mutation landscape of MSI-H and MSI-L/MSS groups. Then, we constructed an MSI-related risk signature based on the mutated genes in the MSI-H group. Finally, we investigated the association between the MSI-related risk signature and immunotherapeutic and chemotherapeutic responses. We present the following article in accordance with the TRIPOD reporting checklist (available at https://jgo.amegroups.com/article/view/10.21037/jgo-21-808/rc).
Methods
Data acquisition
The study was conducted in accordance with the Declaration of Helsinki (as revised in 2013).
The gene-expression profiles, somatic mutations, and clinical data of GC samples were acquired from the TCGA database. We also extracted the MSI status [MSI-H, MSS, and MSI-low (MSI-L) tumors] of each patient in the TCGA database, as outlined by Bonneville et al. (20). Furthermore, 109 GC samples from the GSE26901 dataset were downloaded from the Gene Expression Omnibus (GEO) database to act as a validation set.
Evaluation of MSI in GC
GC patients in the TCGA database were divided into MSI-H and MSI-L/MSS groups according to their MSI status. We compared the survival data of GC patients in these two groups and also calculated the difference in TMB using the Wilcoxon test.
Mutation landscape differences between the MSI-H and MSI-L/MSS groups
To investigate the mutation landscape differences of patients in the MSI-H and MSI-L/MSS groups, the ‘maftools’ R package was used to analyze and visualize the somatic mutation data of each GC patient (21).
Construction and verification of an MSI-related risk signature in GC
Based on the mutated gene data obtained from GC patients in the TCGA database, we established an MSI-related risk signature by conducting a univariate Cox regression analysis to screen genes with expression levels related to survival. Next, the genes identified as significant by univariate Cox regression analysis were entered into a multivariate Cox regression analysis to remove false-positive genes through the ‘step’ R function. Using this method, an MSI-related risk signature was established based on the expression level of each gene and its corresponding Cox coefficient derived from the multivariate Cox regression analysis; namely, the risk score of each patient was defined as follows: . In this formula, expressi represents the expression level of patient i, and coeffi represents the Cox coefficient of gene i obtained from the multivariate Cox regression analysis. Therefore, patients in the TCGA database were classified into high- and low-risk groups based on the median risk score. Finally, a Kaplan-Meier (K-M) survival analysis was used to compare the difference in overall survival (OS) between the high- and low-risk groups using the log-rank test. Using the ‘survivalROC’ R package, receiver operating characteristic (ROC) analyses were conducted to evaluate the effectiveness of the MSI-related risk signature in predicting the 1-, 3-, and 5-year OS of GC patients (22). Meanwhile, patients in the GSE26901 dataset were also stratified into high- and low-risk groups according to the median risk score obtained by using the above formula. Subsequently, K-M and ROC curves were plotted to further validate the efficacy of the MSI-related risk signature.
Association between the MSI-related risk signature and clinical features
To further explore the association between the MSI-related risk signature and clinical features, including age, gender, pathological T stage, pathological N stage, pathological M stage, pathological tumor stage, TMB status, and MSI status, we compared the distribution of risk scores with different clinical variables using the Wilcoxon test or one-way ANOVA test.
Independent prognostic analysis
Univariate and multivariate Cox regression analyses were performed to investigate whether the MSI-related risk signature could predict the OS of GC patients from the TCGA database independent of other clinical features, such as age, race, gender, pathological T stage, pathological N stage, pathological M stage, pathological tumor stage, TMB status, and MSI status.
Functional enrichment analysis
To further investigate the biological function of the MSI-related risk signature, Gene set enrichment analysis (GSEA, https://www.broadinstitute.org/gsea/index.jsp) was conducted to explore Kyoto Encyclopedia of Genes and Genomes (KEGG) pathways associated with the MSI-related risk signature based on genes in the high- and low-risk groups in the TCGA database (23). Moreover, to investigate the differences in the mutation-related pathways between high- and low-risk groups, we calculated and compared the enrichment scores of the mutation-related pathways [including nucleotide excision repair (NER), mismatch repair (MMR), homologous recombination (HMR), and base excision repair (BER)] between the high- and low-risk groups by using a single sample GSEA (ssGSEA) through the ‘GSVA’ R package (24).
Estimation of immune infiltration in the GC TME
Firstly, we download 29 immune signatures, including immune cells, immune functions, and pathways from the Molecular Signature Database v5.1 (MSigDB) (https://www.broad.mit.edu/gsea/msigdb/) (23). Next, ssGSEA was used to calculate the ssGSEA scores of these 29 immune signatures (24), and the Wilcoxon test was used to detect differences in the 29 ssGSEA scores between the high- and low-risk groups in the TCGA database. Moreover, we also compared the differences in cytolytic activity between the high- and low-risk groups based on the expression of GZMA and PRF1 in the TCGA database (25). Finally, antigen presentation mechanism scores between the high- and low-risk groups were compared based on seven genes (HLA-A/B/C, B2M, TAP1, TAP2, and TAPBP37) in the TCGA database (26).
Assessment of the immunotherapeutic and chemotherapeutic response
A Tumor Immune Dysfunction and Exclusion (TIDE) algorithm was selected to estimate whether the MSI-related risk signature could predict the immunotherapeutic response of patients in the TCGA database as described previously (27). In addition, the ‘pRRophetic’ R package was used to assess the chemotherapeutic response based on the half-maximal inhibitory concentration (the half maximal inhibitory concentration, IC50) for each GC patient on the Genomics of Drug Sensitivity in Cancer (GDSC) website (28,29).
Statistical analyses
All statistical analyses in this study were achieved by R software. The Wilcoxon test was selected to compare the differences between the two groups. Using a two-tailed test of significance, a P value <0.05 was considered statistically significant.
Results
MSI was related to the survival and TMB of GC patients
We firstly downloaded somatic mutation data from 433 GC patients in the TCGA database. After removing the samples without MSI or survival status information, the data from 378 GC patients were used to estimate the correlation between MSI status and survival. The sample information is presented in Table 1. As shown in Figure 1A, the MSI-H group had a relatively higher survival rate. The MSI-H group also showed a higher TMB than the MSI-L/MSS group (Figure 1B,1C). Thus, MSI is related to genetic mutations and the survival of GC patients.
Table 1
Variable | Alive (N=227) | Dead (N=151) | P value | Overall (N=378) |
---|---|---|---|---|
Age | ||||
Mean (SD) | 65.1 (11.1) | 67.1 (10.1) | 0.073 (−1.8) | 65.9 (10.7) |
Median [Min, Max] | 66.0 [35.0, 90.0] | 68.0 [41.0, 90.0] | 67.0 [35.0, 90.0] | |
Missing, n (%) | 4 (1.8) | 0 (0.0) | 4 (1.1) | |
Gender, n (%) | ||||
Female | 91 (40.1) | 46 (30.5) | 0.07 (3.635) | 137 (36.2) |
Male | 136 (59.9) | 105 (69.5) | 241 (63.8) | |
Race, n (%) | ||||
Asian | 55 (24.2) | 19 (12.6) | 0.007 (13.438) | 74 (19.6) |
Black or African American | 3 (1.3) | 8 (5.3) | 11 (2.9) | |
Not reported | 30 (13.2) | 24 (15.9) | 54 (14.3) | |
White | 139 (61.2) | 99 (65.6) | 238 (63.0) | |
Native Hawaiian or other pacific islander | 0 (0.0) | 1 (0.7) | 1 (0.3) | |
AJCC_pathologic_m, n (%) | ||||
M0 | 203 (89.4) | 129 (85.4) | 0.334 (2.259) | 332 (87.8) |
M1 | 12 (5.3) | 14 (9.3) | 26 (6.9) | |
MX | 12 (5.3) | 8 (5.3) | 20 (5.3) | |
AJCC_pathologic_n, n (%) | ||||
N0 | 83 (36.6) | 30 (19.9) | 0.001 (19.57) | 113 (29.9) |
N1 | 55 (24.2) | 42 (27.8) | 97 (25.7) | |
N2 | 45 (19.8) | 31 (20.5) | 76 (20.1) | |
N3 | 31 (13.7) | 43 (28.5) | 74 (19.6) | |
NX | 11 (4.8) | 5 (3.3) | 16 (4.2) | |
Missing | 2 (0.9) | 0 (0.0) | 2 (0.5) | |
AJCC_pathologic_t, n (%) | ||||
T1 | 16 (7.0) | 3 (2.0) | 0.083 (8.37) | 19 (5.0) |
T2 | 56 (24.7) | 28 (18.5) | 84 (22.2) | |
T3 | 94 (41.4) | 74 (49.0) | 168 (44.4) | |
T4 | 58 (25.6) | 42 (27.8) | 100 (26.5) | |
TX | 3 (1.3) | 4 (2.6) | 7 (1.9) | |
AJCC_pathologic_stage, n (%) | ||||
Stage I | 41 (18.1) | 13 (8.6) | <0.001 (17.709) | 54 (14.3) |
Stage II | 77 (33.9) | 35 (23.2) | 112 (29.6) | |
Stage III | 81 (35.7) | 70 (46.4) | 151 (39.9) | |
Stage IV | 16 (7.0) | 23 (15.2) | 39 (10.3) | |
Missing | 12 (5.3) | 10 (6.6) | 22 (5.8) | |
Grade, n (%) | ||||
G1 | 8 (3.5) | 2 (1.3) | 0.326 (3.562) | 10 (2.6) |
G2 | 87 (38.3) | 49 (32.5) | 136 (36.0) | |
G3 | 125 (55.1) | 95 (62.9) | 220 (58.2) | |
GX | 5 (2.2) | 4 (2.6) | 9 (2.4) | |
Missing | 2 (0.9) | 1 (0.7) | 3 (0.8) | |
MSI, n (%) | ||||
MSI-H | 43 (18.9) | 26 (17.2) | 0.676 (0.181) | 69 (18.3) |
MSI-L/MSS | 184 (81.1) | 125 (82.8) | 309 (81.7) |
GC, gastric cancer; TCGA, The Cancer Genome Atlas; MSI, microsatellite instability; MSI-H, MSI-high; MSI-L, MSI-low; MSS, microsatellite stability.
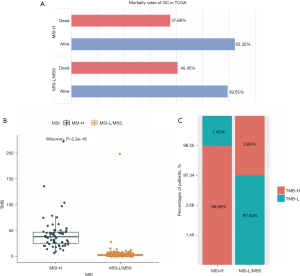
Mutation landscape differences between the MSI-H and MSI-L/MSS groups
To show the mutation landscape differences between the MSI-H and MSI-L/MSS groups, the mutation information of each gene in 378 GC patients was summarized and visualized by the ‘maftools’ R package. It clearly demonstrated that the MSI-H group showed higher mutation rates in 99 of the top 100 mutated genes than the MSI-L/MSS group (Figure 2A,2B). For example, the mutation rate of TTN was 94% in the MSI-H group but 37% in the MSI-L/MSS group. Moreover, in both the MSI-H and MSI-L/MSS groups, the highest variant classification was the missense mutation, the highest variant type was single nucleotide polymorphism (SNP), and C>T was the highest form of SNP. Furthermore, we found the variation frequency of each sample in the MSI-H group was significantly higher than that in the MSI-L/MSS group (Figure 2B-2D). These results further demonstrated that MSI may affect genetic mutations in GC patients. Finally, we also presented the top 10 mutated genes in these two groups with ranked percentages. In the MSI-H group, the top 10 mutated genes were ARID1A (79%), FAT4 (51%), KMT2D (67%), LRP1B (55%), MUC16 (63%), OBSCN (52%), PLEC (52%), and RNF213 (54%), SYNE1 (57%), TTN (94%). The top 10 mutated genes in the MSI-L/MSS group were ARIDIA (12%) CSMD3 (14%), FAT4 (11%), FLG (15%), LRP1B (17%), MUC16 (23%), SPTA1 (11%), SYNE1 (13%), TP53 (46%) and TTN (37%) (Figure 2B,2D).
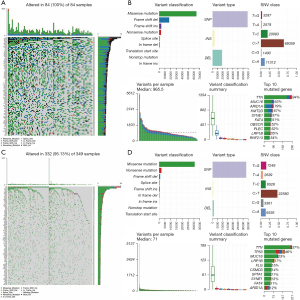
Construction and verification of an MSI-related risk signature in GC
After removing GC samples with a survival time of less than 30 days or without survival information in the TCGA database, the gene expression profiles of 338 GC patients were selected to construct an MSI-related risk signature based on the 99 genes with higher mutation rates in the MSI-H group. Firstly, univariate Cox regression analysis indicated that 31 genes be retained for the multivariate Cox regression analysis (P<0.2, Table S1). Next, the multivariate Cox regression analysis identified 10 robust genes (CUBN, DMD, FAT4, LRP1, MUC16, PXDN, RNF43, RP1, SLC3A2 and SYNE1) that could constitute an MSI-related risk signature (P<0.1, Table 2). Therefore, the MSI-related risk signature was established according to the expression levels of the 10 genes, and its regression coefficient was derived from the multivariate Cox regression analysis. Based on the risk score of each GC patient, 338 GC patients in the TCGA database and 109 GC patients in the GSE26901 dataset were stratified into high- and low-risk groups, respectively. The K-M analysis revealed that patients in the high-risk group of both the TCGA database and GSE26901 dataset exhibited significantly poorer OS than those in the low-risk group (Figure 3A,3B). Moreover, as illustrated by the ROC curves, the area under the curves (AUCs) of the MSI-related risk signature for predicting the 1-, 3-, and 5-year OS of GC patients in the TCGA database were 0.686, 0.707, and 0.720, respectively (Figure 3C), and those in the GSE26091 dataset were 0.645, 0.650, and 0.650, respectively (Figure 3D), indicating that the MSI-related risk signature performed well in predicting the OS of GC patients. Finally, we found that CUBN, DMD, SYNE1, FAT4, LRP1, PXDN, MUC16, and RP1 were significantly more highly expressed in the high-risk group in the TCGA database, while other genes showed lower expressions in the high-risk group (Figure 3E). However, CUBN, LRP1, PXDN, SLC3A2, FAT4, and DMD were significantly more highly expressed in the high-risk group in the GSE26091 dataset, but other genes showed lower expressions in the high-risk group (Figure 3F). These differences may reflect the samples differences between these two datasets.
Table 2
ID | coef | HR | HR.95L | HR.95H | P value |
---|---|---|---|---|---|
CUBN | 1.389242884 | 4.011811498 | 1.672614374 | 9.622440024 | 0.001855963 |
MUC16 | 0.240709835 | 1.272151847 | 0.997266839 | 1.622805711 | 0.052631828 |
RNF43 | −0.330014247 | 0.718913491 | 0.610498793 | 0.846580883 | 7.59E-05 |
LRP1 | 0.446161201 | 1.562303291 | 1.211285968 | 2.015041566 | 0.00058976 |
SYNE1 | −0.915776698 | 0.400205667 | 0.221519424 | 0.723027229 | 0.002408284 |
PXDN | 0.284598749 | 1.329228566 | 1.028178449 | 1.718426003 | 0.02985215 |
FAT4 | −0.466003359 | 0.627505176 | 0.37317874 | 1.055158571 | 0.078836928 |
SLC3A2 | −0.370499216 | 0.690389591 | 0.498202146 | 0.956715645 | 0.026028088 |
RP1 | 1.678261542 | 5.356236281 | 1.244163384 | 23.0590833 | 0.024241584 |
DMD | 0.452524681 | 1.572276676 | 1.127011086 | 2.193460186 | 0.007726009 |
MSI, microsatellite instability; HR, hazard ratio.
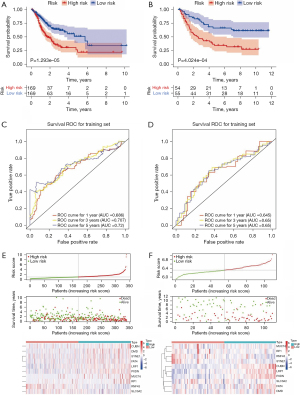
Association between the MSI-related risk signature and clinical features
Meanwhile, we also explored the relationships between the MSI-related risk signature and clinical features in the TCGA database. The results of the Wilcoxon or one-way ANOVA test revealed that the MSI-related risk signature was not correlated with other clinical features, such as age, gender, pathological T stage, pathological N stage, pathological M stage, and pathological tumor stage (Figure 4A-4F). Only related to TMB and MSI status (Figure 4G,4H).
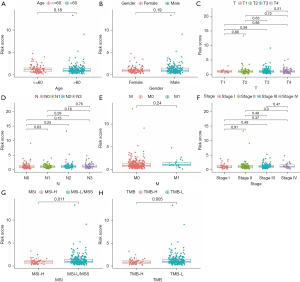
The MSI-related risk signature is an independent prognostic factor
We performed an independent prognostic analysis to explore whether the MSI-related risk signature could predict the OS of GC patients independent of other clinical features. The results of the univariate and multivariate Cox regression analyses suggested that the MSI-related risk signature remained as an independent prognostic factor for GC patients after adjusting for clinical features such as age, race, gender, pathological T stage, pathological N stage, pathological M stage, pathological tumor stage, TMB status, and MSI status (Figure 5A,5B).
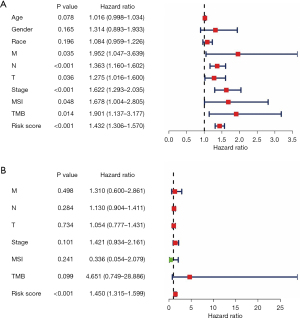
Functional enrichment analysis of the MSI-related risk signature
GSEA was used to explore the KEGG pathways associated with the MSI-related risk signature. As expected, we found that genes involved mainly in the mutation and DNA repair-related pathways differed between the high- and low-risk groups. For example, KEGG pathways related to BER were enriched in the high-risk group, while NER and RNA_degradation were enriched in the low-risk group (Figure 6A). Moreover, we further examined the ssGSEA scores for four DNA repair-related pathways, including NER, MR, HR, and BER. Interestingly, the high-risk group showed lower ssGSEA scores than the low-risk group (Figure 6B). Thus, these results suggested that the MSI-related risk signature may be mainly involved in the DNA repair pathway in the low-risk group, ultimately leading to MSI.
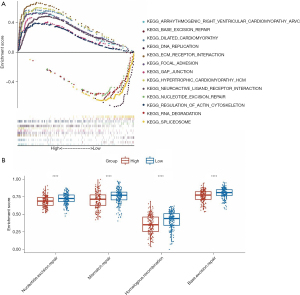
Correlation between the MSI-related risk signature and TME immune cell infiltration in GC
To investigate whether the MSI-related risk signature influenced TME immune cell infiltration in GC, ssGSEA was selected to explore the enrichment scores of 29 immune signatures. The results showed that CD8 T cells, cytolytic activity, inflammation promotion, mast cells, MHC class I, NK cells, pDCs, T cell co-inhibition, T cell co-stimulation, Tfh, Th1 cells, Th2_cells, and type II IFN responses were significantly different between the high- and low-risk groups (Figure 7A), and most of them presented higher enrichment scores in the low-risk group (Figure 7A). Moreover, considering that natural anti-tumor immunity requires a cytolytic immune response, we further compared the immune cell-mediated cytolytic activity between the high- and low-risk groups. Interestingly, we found that the cytolytic scores of patients in the low-risk group were significantly higher than those in the high-risk group (Figure 7B). Furthermore, we calculated the (antigen processing machinery, AMP) between the high- and low-risk groups and found that the low-risk group had higher AMP scores than the high-risk group (Figure 7C). In brief, these results revealed that the MSI-related risk signature may be associated with TME immune cell infiltration and enhances the anti-tumor immune response.
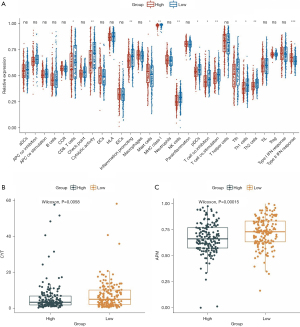
The MSI-related risk signature predicted the clinical response to immunotherapy and chemotherapy
Currently, immunotherapy is a promising choice for treating cancers (30,31), especially ICB targeting CTLA-4 and PD-1 (32). Therefore, we compared the clinical response to ICB (CTLA-4 and PD-1) between the high- and low-risk groups based on the TIDE algorithm. Notably, we found that TIDE scores were remarkably lower in the low-risk group compared with the high-risk group (Figure 8A). Moreover, we also estimated the chemotherapeutic response to chemotherapy using the ‘pRRophetic’ algorithm. Interestingly, we found that patients in the low-risk group were more sensitive to paclitaxel chemotherapy than the high-risk group (Figure 8B). Therefore, the MSI-related risk signature may be used to predict the clinical response to immunotherapy and chemotherapy.
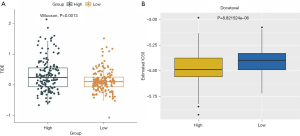
Discussion
Globally, GC is a common digestive system cancer with high malignancy, but especially in Asia, where it causes a significant social burden (1). Unfortunately, although chemotherapy combined with targeted drug therapy has moderately improved the OS of GC, the prognosis remains poor, with a 5-year survival rate of approximately 20% (33,34). Survival predictions can guide the clinical treatment of tumors. Over the past few decades, traditional survival predictions based on pathological information have played a key role in the clinical treatment of cancers (35). However, relying solely on conventional methods to predict tumor survival has greatly limited the development of precision therapy. Therefore, there is an urgent need to identify novel molecular biomarkers for predicting survival in GC. Increasing evidence has suggested that MSI status affects survival and treatment in several cancers, including GC (35,36). However, the role of MSI-related genes in GC remains unknown.
In the present study, we firstly compared survival between the MSI-H and MSI-L/MSS groups. We found that the MSI-H group had a relatively high survival rate (Figure 1A), which indicated that MSI status is related to the survival of GC patients. Moreover, we identified 99 genes with high mutation rates in the MSI-H group. Furthermore, we constructed an MSI-related risk signature based on the expression and corresponding coefficients of 10 mutated genes (CUBN, MUC16, RNF43, LRP1, SYNE1, PXDN, FAT4, SLC3A2, RP1, and DMD). Univariate and multivariate Cox analyses suggested that the MSI-related risk signature predicted the prognosis of GC independent of other factors. Functional enrichment analysis revealed that the MSI-related risk signature was highly associated with mutation and DNA repair-related pathways (Figure 6A,6B), which further demonstrated that the MSI-related risk signature may be a valid representation of MSI status.
CUBN, also known as intestinal intrinsic factor, intrinsic factor-cobalamin receptor, or intrinsic factor-vitamin B12 receptor, has been reported to be involved in the development and progression of cancers. For example, CUBN expression is highly heterogeneous and affects the prognosis of renal cell carcinoma (37,38). Moreover, it has been suggested that CUBN is linked to gastric carcinogenesis by regulating vitamin B12 metabolism (39). Furthermore, the somatic mutation of CUBN changes isoleucine into valine, ultimately influencing the risk of recurrence in osteosarcoma (40). More importantly, mutation in CUBN is associated with the occurrence of GC (41). MUC16, previously known as CA125, has been identified as one of the top three frequently mutated genes in multiple cancers and is related to the growth and metastasis of cancer cells (42). Consistent with our results, MUC16 expression has been linked to prognosis in multiple malignancies, such as pancreatic ductal adenocarcinoma, pancreatic cancer, and epithelial ovarian cancer (43-45). A recent study also found that MUC16 mutation may be associated with TMB, immune response, and survival in GC (46). RNF43 expression affects the DNA damage response in GC (47), and our study also found that the MSI-related risk signature was mainly associated with the DNA repair-related pathway. LRP1 has been revealed to be associated with prognosis and immune modulation in clear-cell renal cell carcinoma (48). SYNE1 mutation can affect immune cell infiltration, TMB, and ICB therapy in clear-cell renal cell carcinoma patients (49). PDXN expression can affect the proliferation, invasion, and migration of ovarian cancer cells by regulating the PI3K/Akt pathway and has been proposed as a potential target for OC therapy (50). It has been suggested that FAT4 regulates the occurrence and development of colorectal cancer by regulating the PI3K/AKT/mTOR and PI3K/AKT/GSK-3β signaling pathways (51). SLC3A2 can affect the migration, invasion, and proliferation of oral squamous cell carcinoma (52). RP1 appears to play an oncogenic role in breast cancer by suppressing p27kip1 (53). DMD mutation has been demonstrated to affect survival in uterine cancer (54). In brief, our study also found that CUBN, MUC16, RNF43, LRP1, SYNE1, PXDN, FAT4, SLC3A2, RP1, and DMD may play key roles in GC.
Interestingly, the results of the ssGSEA based on 29 immune signatures revealed that TME immune cell infiltration was markedly different between the high- and low-risk groups (Figure 7A). Consistent with our results, it has been suggested that MSI status is associated with the TME component in primary colorectal cancer samples (18,19). Moreover, MSI-H colorectal cancer also shows more immune cell infiltration, especially tumor-infiltrating lymphocytes (18). Similarly, our study also revealed that MSI status affects the TME immune cell infiltration of GC, which may contribute to GC treatment. TME immune cell infiltration is considered one of the most important factors in predicting clinical response to immunotherapy in many cancers (55,56). For instance, NK cells and CD8+ T cells can secrete TNF, perforin, and granzyme, leading to cytotoxic effects (57). Considering that the MSI-related risk signature affects TME immune cell infiltration, we further explored whether the MSI-related risk signature could predict the clinical response to immunotherapy. Just as we assumed, we found that the TIDE score was significantly different between the high- and low-risk groups (Figure 8A). Therefore, the MSI-related risk signature could be used to predict the clinical response to immunotherapy. Similarly, a recent study has suggested that MSI status affects the TME and increases sensitivity to ICB (58). Moreover, we also found that the MSI-related risk signature was related to paclitaxel chemotherapy (Figure 8B). MSI has been revealed to have a differentially negative prognostic effect in patients treated with chemotherapy (12). Hence, our study further revealed that MSI status may be related to chemotherapy.
Conclusions
In summary, our study developed an MSI-related risk signature for predicting the OS of GC patients based on 10 robust and MSI-related genes (CUBN, MUC16, RNF43, LRP1, SYNE1, PXDN, FAT4, SLC3A2, RP1, and DMD). Moreover, univariate and multivariate Cox analysis suggested that the MSI-related risk signature was an independent prognostic factor for GC patients. Furthermore, we revealed that the MSI-related risk signature affects the TME immune cell infiltration in GC and can be used to predict the clinical response to immunotherapy. Therefore, these findings may indicate that MSI status plays a key role in GC and may contribute to the clinical treatment of GC. However, further research is needed to verify these findings.
Acknowledgments
We acknowledge TCGA, KEGG, and the GEO database for providing their platforms and the contributors for uploading their meaningful datasets.
Funding: None.
Footnote
Reporting Checklist: The authors have completed the TRIPOD reporting checklist. Available at https://jgo.amegroups.com/article/view/10.21037/jgo-21-808/rc
Conflicts of Interest: All authors have completed the ICMJE uniform disclosure form (available at https://jgo.amegroups.com/article/view/10.21037/jgo-21-808/coif). The authors have no conflicts of interest to declare.
Ethical Statement: The authors are accountable for all aspects of the work in ensuring that questions related to the accuracy or integrity of any part of the work are appropriately investigated and resolved. The study was conducted in accordance with the Declaration of Helsinki (as revised in 2013).
Open Access Statement: This is an Open Access article distributed in accordance with the Creative Commons Attribution-NonCommercial-NoDerivs 4.0 International License (CC BY-NC-ND 4.0), which permits the non-commercial replication and distribution of the article with the strict proviso that no changes or edits are made and the original work is properly cited (including links to both the formal publication through the relevant DOI and the license). See: https://creativecommons.org/licenses/by-nc-nd/4.0/.
References
- Bray F, Ferlay J, Soerjomataram I, et al. Global cancer statistics 2018: GLOBOCAN estimates of incidence and mortality worldwide for 36 cancers in 185 countries. CA Cancer J Clin 2018;68:394-424. [Crossref] [PubMed]
- Joharatnam-Hogan N, Shiu KK, Khan K. Challenges in the treatment of gastric cancer in the older patient. Cancer Treat Rev 2020;85:101980. [Crossref] [PubMed]
- Song Z, Wu Y, Yang J, et al. Progress in the treatment of advanced gastric cancer. Tumour Biol 2017;39:1010428317714626. [Crossref] [PubMed]
- Mehta R, Kommalapati A, Kim RD. The Impact of Ramucirumab Treatment on Survival and Quality of Life in Patients with Gastric Cancer. Cancer Manag Res 2020;12:51-7. [Crossref] [PubMed]
- Boland CR, Goel A. Microsatellite instability in colorectal cancer. Gastroenterology 2010;138:2073-2087.e3. [Crossref] [PubMed]
- de la Chapelle A, Hampel H. Clinical relevance of microsatellite instability in colorectal cancer. J Clin Oncol 2010;28:3380-7. [Crossref] [PubMed]
- Sargent DJ, Marsoni S, Monges G, et al. Defective mismatch repair as a predictive marker for lack of efficacy of fluorouracil-based adjuvant therapy in colon cancer. J Clin Oncol 2010;28:3219-26. [Crossref] [PubMed]
- Cancer Genome Atlas Research Network. Comprehensive molecular characterization of gastric adenocarcinoma. Nature 2014;513:202-9. [Crossref] [PubMed]
- Cristescu R, Lee J, Nebozhyn M, et al. Molecular analysis of gastric cancer identifies subtypes associated with distinct clinical outcomes. Nat Med 2015;21:449-56. [Crossref] [PubMed]
- An JY, Kim H, Cheong JH, et al. Microsatellite instability in sporadic gastric cancer: its prognostic role and guidance for 5-FU based chemotherapy after R0 resection. Int J Cancer 2012;131:505-11. [Crossref] [PubMed]
- Fang WL, Chang SC, Lan YT, et al. Microsatellite instability is associated with a better prognosis for gastric cancer patients after curative surgery. World J Surg 2012;36:2131-8. [Crossref] [PubMed]
- Smyth EC, Wotherspoon A, Peckitt C, et al. Mismatch Repair Deficiency, Microsatellite Instability, and Survival: An Exploratory Analysis of the Medical Research Council Adjuvant Gastric Infusional Chemotherapy (MAGIC) Trial. JAMA Oncol 2017;3:1197-203. [Crossref] [PubMed]
- Germano G, Lamba S, Rospo G, et al. Inactivation of DNA repair triggers neoantigen generation and impairs tumour growth. Nature 2017;552:116-20. [Crossref] [PubMed]
- Ganesh K, Stadler ZK, Cercek A, et al. Immunotherapy in colorectal cancer: rationale, challenges and potential. Nat Rev Gastroenterol Hepatol 2019;16:361-75. [Crossref] [PubMed]
- Le DT, Kim TW, Van Cutsem E, et al. Phase II Open-Label Study of Pembrolizumab in Treatment-Refractory, Microsatellite Instability-High/Mismatch Repair-Deficient Metastatic Colorectal Cancer: KEYNOTE-164. J Clin Oncol 2020;38:11-9. [Crossref] [PubMed]
- Le DT, Durham JN, Smith KN, et al. Mismatch repair deficiency predicts response of solid tumors to PD-1 blockade. Science 2017;357:409-13. [Crossref] [PubMed]
- Le DT, Uram JN, Wang H, et al. PD-1 Blockade in Tumors with Mismatch-Repair Deficiency. N Engl J Med 2015;372:2509-20. [Crossref] [PubMed]
- Llosa NJ, Cruise M, Tam A, et al. The vigorous immune microenvironment of microsatellite instable colon cancer is balanced by multiple counter-inhibitory checkpoints. Cancer Discov 2015;5:43-51. [Crossref] [PubMed]
- Kelderman S, Schumacher TN, Kvistborg P. Mismatch Repair-Deficient Cancers Are Targets for Anti-PD-1 Therapy. Cancer Cell 2015;28:11-3. [Crossref] [PubMed]
- Bonneville R, Krook MA, Kautto EA, et al. Landscape of Microsatellite Instability Across 39 Cancer Types. JCO Precis Oncol 2017;2017:PO.17.00073.
- Mayakonda A, Lin DC, Assenov Y, et al. Maftools: efficient and comprehensive analysis of somatic variants in cancer. Genome Res 2018;28:1747-56. [Crossref] [PubMed]
- Heagerty PJ, Zheng Y. Survival model predictive accuracy and ROC curves. Biometrics 2005;61:92-105. [Crossref] [PubMed]
- Subramanian A, Tamayo P, Mootha VK, et al. Gene set enrichment analysis: a knowledge-based approach for interpreting genome-wide expression profiles. Proc Natl Acad Sci U S A 2005;102:15545-50. [Crossref] [PubMed]
- Rooney MS, Shukla SA, Wu CJ, et al. Molecular and genetic properties of tumors associated with local immune cytolytic activity. Cell 2015;160:48-61. [Crossref] [PubMed]
- Wakiyama H, Masuda T, Motomura Y, et al. Cytolytic Activity (CYT) Score Is a Prognostic Biomarker Reflecting Host Immune Status in Hepatocellular Carcinoma (HCC). Anticancer Res 2018;38:6631-8. [Crossref] [PubMed]
- Linxweiler M, Kuo F, Katabi N, et al. The Immune Microenvironment and Neoantigen Landscape of Aggressive Salivary Gland Carcinomas Differ by Subtype. Clin Cancer Res 2020;26:2859-70. [Crossref] [PubMed]
- Jiang P, Gu S, Pan D, et al. Signatures of T cell dysfunction and exclusion predict cancer immunotherapy response. Nat Med 2018;24:1550-8. [Crossref] [PubMed]
- Geeleher P, Cox NJ, Huang RS. Clinical drug response can be predicted using baseline gene expression levels and in vitro drug sensitivity in cell lines. Genome Biol 2014;15:R47. [Crossref] [PubMed]
- Xu F, Lin H, He P, et al. A TP53-associated gene signature for prediction of prognosis and therapeutic responses in lung squamous cell carcinoma. Oncoimmunology 2020;9:1731943. [Crossref] [PubMed]
- Johnson DB, Sullivan RJ, Menzies AM. Immune checkpoint inhibitors in challenging populations. Cancer 2017;123:1904-11. [Crossref] [PubMed]
- Atkins MB, Clark JI, Quinn DI. Immune checkpoint inhibitors in advanced renal cell carcinoma: experience to date and future directions. Ann Oncol 2017;28:1484-94. [Crossref] [PubMed]
- Postow MA, Callahan MK, Wolchok JD. Immune Checkpoint Blockade in Cancer Therapy. J Clin Oncol 2015;33:1974-82. [Crossref] [PubMed]
- Bang YJ, Van Cutsem E, Feyereislova A, et al. Trastuzumab in combination with chemotherapy versus chemotherapy alone for treatment of HER2-positive advanced gastric or gastro-oesophageal junction cancer (ToGA): a phase 3, open-label, randomised controlled trial. Lancet 2010;376:687-97. [Crossref] [PubMed]
- Fuchs CS, Tomasek J, Yong CJ, et al. Ramucirumab monotherapy for previously treated advanced gastric or gastro-oesophageal junction adenocarcinoma (REGARD): an international, randomised, multicentre, placebo-controlled, phase 3 trial. Lancet 2014;383:31-9. [Crossref] [PubMed]
- Polom K, Marano L, Marrelli D, et al. Meta-analysis of microsatellite instability in relation to clinicopathological characteristics and overall survival in gastric cancer. Br J Surg 2018;105:159-67. [Crossref] [PubMed]
- Mathiak M, Warneke VS, Behrens HM, et al. Clinicopathologic Characteristics of Microsatellite Instable Gastric Carcinomas Revisited: Urgent Need for Standardization. Appl Immunohistochem Mol Morphol 2017;25:12-24. [Crossref] [PubMed]
- Gremel G, Djureinovic D, Niinivirta M, et al. A systematic search strategy identifies cubilin as independent prognostic marker for renal cell carcinoma. BMC Cancer 2017;17:9. [Crossref] [PubMed]
- Ma J, Guan M, Bowden DW, et al. Association Analysis of the Cubilin (CUBN) and Megalin (LRP2) Genes with ESRD in African Americans. Clin J Am Soc Nephrol 2016;11:1034-43. [Crossref] [PubMed]
- Zhao L, Wei Y, Song A, et al. Association study between genome-wide significant variants of vitamin B12 metabolism and gastric cancer in a han Chinese population. IUBMB Life 2016;68:303-10. [Crossref] [PubMed]
- Choy E, Hornicek F, MacConaill L, et al. High-throughput genotyping in osteosarcoma identifies multiple mutations in phosphoinositide-3-kinase and other oncogenes. Cancer 2012;118:2905-14. [Crossref] [PubMed]
- Al-Tassan NA, Whiffin N, Hosking FJ, et al. A new GWAS and meta-analysis with 1000Genomes imputation identifies novel risk variants for colorectal cancer. Sci Rep 2015;5:10442. [Crossref] [PubMed]
- Kim N, Hong Y, Kwon D, et al. Somatic mutaome profile in human cancer tissues. Genomics Inform 2013;11:239-44. [Crossref] [PubMed]
- Thériault C, Pinard M, Comamala M, et al. MUC16 (CA125) regulates epithelial ovarian cancer cell growth, tumorigenesis and metastasis. Gynecol Oncol 2011;121:434-43. [Crossref] [PubMed]
- Shimizu A, Hirono S, Tani M, et al. Coexpression of MUC16 and mesothelin is related to the invasion process in pancreatic ductal adenocarcinoma. Cancer Sci 2012;103:739-46. [Crossref] [PubMed]
- Chen SH, Hung WC, Wang P, et al. Mesothelin binding to CA125/MUC16 promotes pancreatic cancer cell motility and invasion via MMP-7 activation. Sci Rep 2013;3:1870. [Crossref] [PubMed]
- Li X, Pasche B, Zhang W, et al. Association of MUC16 Mutation With Tumor Mutation Load and Outcomes in Patients With Gastric Cancer. JAMA Oncol 2018;4:1691-8. [Crossref] [PubMed]
- Noto JM, Peek RM Jr. RNF43: A Biomarker With Potential Ramifications for Therapeutic Intervention in Gastric Cancer. Cell Mol Gastroenterol Hepatol 2021;11:1202-3. [Crossref] [PubMed]
- Feng C, Ding G, Ding Q, et al. Overexpression of low density lipoprotein receptor-related protein 1 (LRP1) is associated with worsened prognosis and decreased cancer immunity in clear-cell renal cell carcinoma. Biochem Biophys Res Commun 2018;503:1537-43. [Crossref] [PubMed]
- Li P, Xiao J, Zhou B, et al. SYNE1 mutation may enhance the response to immune checkpoint blockade therapy in clear cell renal cell carcinoma patients. Aging (Albany NY) 2020. [Epub ahead of print].
- Zheng YZ, Liang L. High expression of PXDN is associated with poor prognosis and promotes proliferation, invasion as well as migration in ovarian cancer. Ann Diagn Pathol 2018;34:161-5. [Crossref] [PubMed]
- Wei R, Xiao Y, Song Y, et al. FAT4 regulates the EMT and autophagy in colorectal cancer cells in part via the PI3K-AKT signaling axis. J Exp Clin Cancer Res 2019;38:112. [Crossref] [PubMed]
- Liang J, Sun Z. Overexpression of membranal SLC3A2 regulates the proliferation of oral squamous cancer cells and affects the prognosis of oral cancer patients. J Oral Pathol Med 2021;50:371-7. [Crossref] [PubMed]
- Jia X, Shi L, Wang X, et al. KLF5 regulated lncRNA RP1 promotes the growth and metastasis of breast cancer via repressing p27kip1 translation. Cell Death Dis 2019;10:373. [Crossref] [PubMed]
- Luce LN, Abbate M, Cotignola J, et al. Non-myogenic tumors display altered expression of dystrophin (DMD) and a high frequency of genetic alterations. Oncotarget 2017;8:145-55. [Crossref] [PubMed]
- Lee JM, Lee MH, Garon E, et al. Phase I Trial of Intratumoral Injection of CCL21 Gene-Modified Dendritic Cells in Lung Cancer Elicits Tumor-Specific Immune Responses and CD8+ T-cell Infiltration. Clin Cancer Res 2017;23:4556-68. [Crossref] [PubMed]
- Hegde PS, Karanikas V, Evers S. The Where, the When, and the How of Immune Monitoring for Cancer Immunotherapies in the Era of Checkpoint Inhibition. Clin Cancer Res 2016;22:1865-74. [Crossref] [PubMed]
- Lu L, Pan K, Zheng HX, et al. IL-17A promotes immune cell recruitment in human esophageal cancers and the infiltrating dendritic cells represent a positive prognostic marker for patient survival. J Immunother 2013;36:451-8. [Crossref] [PubMed]
- Lin A, Zhang J, Luo P. Crosstalk Between the MSI status and tumor microenvironment in colorectal cancer. Front Immunol 2020;11:2039. [Crossref] [PubMed]
(English Language Editor: D. Fitzgerald)