Clinical significance and immunogenomic landscape analysis of glycolysis-associated prognostic model to guide clinical therapy in hepatocellular carcinoma
Introduction
Hepatocellular carcinoma (HCC) has high morbidity and mortality rates and is the most important subtype of primary liver cancer, accounting for about 90% of primary liver cancers in the world (1). In 2021, HCC had the 6th highest mortality rate among all cancers, and in 2020, there were approximately 906,000 new cases of HCC and 830,000 HCC-related deaths (2). Surgery is the main treatment for HCC, but patients’ 5-year survival and overall survival (OS) rates after surgery remain low (3). In recent years, targeted therapy and immunotherapy have been shown to have good therapeutic effect for HCC patients (4,5). Notably, immunotherapy represents a new treatment and provides hope for advanced HCC patients. However, the treatment effect and survival prognosis of patients differ as a result of heterogeneity at the gene level (6). Thus, it is important to study the risk factors for the survival prognosis in the treatment of HCC. A useful biomarker and predictive tool that can effectively evaluate the survival prognosis and therapeutic response of HCC patients urgently needs to be identified, especially for immunotherapy.
Currently, the Barcelona Clinic Liver Cancer (BCLC) and tumor-node-metastasis (TNM) staging systems are the most common tumor staging systems used in the treatment of HCC. As these tumor staging systems mainly depend on hepatic function and tumor histopathology, they are not suitable for the precise diagnosis and treatment of HCC. Besides BCLC and TNM staging systems, numerous prognostic gene signatures have been reported in HCC (7-9). In addition, some predictive biomarkers for response to immunotherapy have been extensively explored, including the expression of programmed death-ligand 1 (PD-L1), tumor mutational burden (TMB), tumor-infiltrating lymphocytes, and immune-related gene signatures (10,11). However, these studies are retrospective analyses with small sample sizes, and the predictive value of them remains unclear. Metabolism reprogramming and the immune microenvironment are important cancer hallmarks, which play a central role in the drug treatment and survival prognosis of HCC patients (12,13). Metabolism reprogramming not only promotes cancer cell growth and proliferation by regulating energy metabolism, but also affects the immune microenvironment by releasing metabolites (14).
It is well known that cancer cells use glycolysis to provide energy to maintain cell proliferation under normal and hypoxic conditions. Notably, the metabolism reprogramming of cancer cells affects antigen presentation, the recognition of immune cells, and their functions (15,16). Immune system dysfunction leads to the failure of immune cells to recognize cancer cells, resulting in tumor immune escape and a poor prognosis. The composition and mechanism of the tumor immune microenvironment are very complex; however, immune cells and immune checkpoints are two important components. Thus, exploring cancer metabolism and the immune microenvironment may have benefits for precision medicine in the treatment of HCC patients.
In this study, we first collected the gene sets of 29 cancer hallmarks and the transcriptome expression data of 810 HCC patients from different databases. Among all the cancer hallmarks, glycolysis was identified as the principal risk factor for the survival prognosis in HCC. We then screened robust candidate genes to build a novel glycolysis-related gene signature (GRS) using various bioinformatic methods. The sensitivity and specificity of the GRS was also confirmed in two independent HCC cohorts (i.e., the GSE14520 and LIRI-JP cohorts) and different clinical feature subgroups. Additionally, we constructed a decision tree and nomogram to improve the predictive accuracy and risk stratification of the HCC patients. Further, we determined the association between the GRS, genomic alterations, and the tumor immune microenvironment to guide the personalized treatment of HCC patients, especially for immunotherapy. The immunotherapy cohort Imvigor210 was also used to predict patient’s responses to anti-PD-L1 therapy. We present the following article in accordance with the TRIPOD reporting checklist (available at https://jgo.amegroups.com/article/view/10.21037/jgo-22-503/rc).
Methods
Data download and analysis
The transcriptome expression profiles and corresponding clinical survival information of 810 HCC patients were collected from different platforms, including The Cancer Genome Atlas–Liver Hepatocellular Carcinoma (TCGA-LIHC), GSE14520, and Liver Cancer–RIKEN JP (LIRI-JP). Specifically, the TCGA-LIHC and LIRI-JP cohort contained the messenger ribonucleic acid (mRNA) sequencing expression data of 346 and 243 HCC patients, respectively. The microarray expression profiles of 221 patients were collected from the GSE14520 cohort. All the mRNA sequencing and microarray expression data were normalized for the subsequent analysis. To ensure the rationality of the survival time, we selected HCC patients whose follow-up survival time was >1 month and deleted HCC patients with missing data. In the present study, the TCGA-LIHC cohort was used to construct a prognostic model for HCC patients, and the GSE14520 and LIRI-JP cohorts were used as the validation cohorts. Additionally, the Imvigor210 cohort (anti-PD-L1 therapy) was used to predict HCC patients’ responses to immunotherapy (17). All the gene sets of the 29 cancer hallmarks were collected from the Molecular Signatures Database (MSigDB) (18,19). The study was conducted in accordance with the Declaration of Helsinki (as revised in 2013).
Candidate gene selection and GRS establishment
First, using a single-sample gene set enrichment analysis (ssGSEA) algorithm, the z-scores of the 29 cancer hallmarks were calculated based on the mRNA sequencing expression data of the HCC patients. According to the z-scores of the cancer hallmarks, we conducted a univariate Cox analysis to determine the role of the 29 cancer hallmarks on survival prognosis. To construct the GRS, candidate genes with a significant survival prognosis were then screened from the glycolysis-related gene sets using R package “survival.” Next, we constructed the gene signature for the HCC patients using a least absolute shrinkage and selection operator (LASSO) Cox regression analysis with “glmnet.” The formula of the gene signature was the expression level of the candidate genes weighted by the LASSO coefficients as follows: GRS = ∑i Coefficient (mRNAi) × Expression (mRNAi). The risk groups were determined based on the appropriate thresholds. Further, the predictive accuracy of the gene signature was evaluated and validated using Kaplan-Meier (K-M) survival curves, and receiver operating characteristic (ROC) analyses were conducted in different HCC cohorts and clinical subgroups.
Tumor immune microenvironment and genomic analysis
The immune cells, genomic alterations and immune checkpoints were examined to analyze the association between the GRS and the tumor immune microenvironment in the HCC patients. In brief, gene sets of 28 tumor-infiltrating immune cells were collected from the Tumor and Immune System Interaction Database (TISIDB) (20). On the basis of these gene sets, the relative content of the immune cells was estimated using the ssGSEA algorithm. Additionally, another Microenvironment Cell Populations-counter (MCP-counter) algorithm was used to further calculate the content of the immune cells and validate the accuracy of the ssGSEA results. The MCP-counter algorithm can accurately distinguish among 10 immune cell types, including B cells, CD8+ T cells, endothelial cells, fibroblasts, macrophages, monocytes, myeloid dendritic cells, neutrophils, natural killer (NK) cells, and T cells (21). Subsequently, the expression difference of 15 immune checkpoints were also analyzed in different GRS groups of HCC patients. Further, the Bioconductor package “maftools” was used to study and visualize the mutant gene profiles and oncogenic signaling pathways (22).
Predicting chemotherapy and immunotherapy responses
Genomics of Drug Sensitivity in Cancer (GDSC) is a database related to pharmacogenomics (23). To examine the effect of drugs in different GRS groups, a half-maximal inhibitory concentration was used to determine the efficacy of the drugs. By mining the drug sensitivity data, the efficacy of the therapeutic drugs among the HCC patients was evaluated using R package “pRRophetic” (24). In this study, we selected 5 test drugs, including 4 chemotherapeutic drugs (i.e., cisplatin, docetaxel, doxorubicin, and gemcitabine) and 1 targeted drug (i.e., sorafenib). Additionally, the Imvigor210 cohort treated with anti-PD-L1 was also used to predict HCC patients’ responses to immunotherapy (17).
Construction of the decision tree and nomogram
Univariate and multivariate Cox regression analyses were conducted to examine the independence of the clinical risk factors using R package “survival.” Based on these independent clinical risk factors, a survival decision tree and nomogram were constructed for risk prediction by R package “rpart” and “rms,” respectively. Additionally, the concordance index (C-index), ROC analysis, and calibration curve analysis were applied to measure the sensitivity and specificity of the nomogram. In general, a model with a larger c-index (>0.70) has a better performance.
Statistical analysis
The statistical analysis was performed using R software v4.1.2. Survival curves and differences were examined using the K-M method and the log-rank test in the R packages “survminer” and “survival.” A Student’s t-test analysis of variance was conducted to analyze the differences the between groups for the variables with a normal distribution. A P value <0.05 was considered statistically significant (two-sided).
Results
Glycolysis was identified as the primary risk factor for survival in HCC
To evaluate the prognostic value of the 29 cancer hallmarks on the survival time of the HCC patients, the enrichment scores and Cox coefficients of the cancer hallmarks were calculated using the ssGSEA algorithm and a univariate Cox analysis, respectively. As Figure 1A shows, 11 cancer hallmarks were significantly correlated with the survival prognosis, and among the 29 cancer hallmarks, glycolysis had the greatest effect on the survival time of the HCC patients (see Table S1). We also found that the glycolysis-related enrichment scores were significantly more increased in the tissues of the HCC and dead patients than the adjacent tissues of the normal and living patients, respectively (P<0.001; see Figure 1B,1C). In the TCGA-LIHC cohort, all the HCC patients were divided into low- and high-score groups according to the median glycolysis score, and the low-score HCC patients had a better OS and disease-free survival (DFS) than the high-score HCC patients (see Figure 1D,1E). We further validated these findings in the GSE14520 and LIRI-JP cohorts, and similarly found that a higher glycolysis-related ssGSEA score was associated with a worse OS and recurrence free survival (RFS) (see Figure 1F-1H). These results suggested that glycolysis was the main risk factor for survival in HCC.
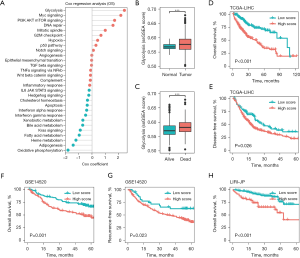
Development of a GRS for prognosis in HCC
Based on the training data set (of the TCGA-LIHC cohort), prognosis-related genes were identified, and used to construct the GRS. We found that a total of 106 promising candidate genes (6 protective and 100 risk genes) were significantly related to the OS of the patients (P<0.05; see Figure 2A). Next, a Lasso Cox regression analysis was conducted to identify the most robust genes for the survival prognosis based on the candidate genes. A total of 12 genes and their Lasso Cox coefficients were identified, which were used to construct the GRS for the HCC patients (see Figure 2B). As Figure 2C shows, the 12 genes and their Lasso Cox coefficients were 0.093*FK506-binding protein 4 (FKBP4), 0.084*steroid 5 alpha reductases type 3 (SRD5A3), 0.073*ATP-binding cassette transporter 6 of subfamily B (ABCB6), 0.070*malic enzyme 2 (ME2), 0.067*glypican-1 (GPC1), 0.058*malic enzyme 1 (ME1), 0.057*heparan sulfate 2-O-sulfotransferase (HS2ST1), 0.047*NAD(P)-dependent steroid dehydrogenase-like protein (NSDHL), 0.040*GDP-mannose pyrophosphorylase A (GMPPA), 0.032*aurora kinase A (AURKA), 0.010*hexokinase 2 (HK2), and –0.181*glutamate oxaloacetate transaminase 2 (GOT2). The HCC patients were divided into 2 groups, and the high-GRS patients had higher risk scores and mortality than the low-GRS patients (see Figure 2D,2E). Additionally, the expression of FKBP4, SRD5A3, ABCB6, ME2, GPC1, ME1, HS2ST1, NSDHL, GMPPA, AURKA, and HK2 tended to be increased in patients in the high-GRS group (see Figure 2F). Notably, the results further suggested that the high-GRS patients had a significantly poorer prognosis than the low-GRS patients (P<0.001; see Figure 2G). The ROC analysis showed that the area under the curves of the GRS were 0.792, 0.723, and 0.717 for 1-, 3-, and 5- years OS, respectively (see Figure 2H).
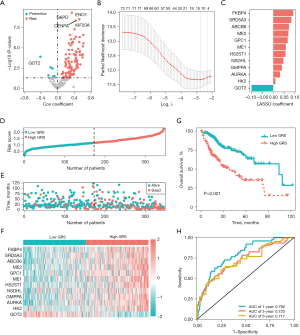
Validation and evaluation of the GRS in different cohorts and clinical subgroups
We further validated the prognostic significance of the GRS in different HCC cohorts (i.e., the GSE14520 and LIRI-JP cohorts) and clinical subgroups. Similar results were also found in the GSE14520 and LIRI-JP cohorts, and our findings demonstrated that the high-GRS HCC patients had a poorer survival prognosis than the low-GRS HCC patients (all P<0.001; see Figure 3A,3B). Additionally, the results also revealed that the low-GRS HCC patients had better RFS and DFS than the high-GRS patients (P<0.05; see Figure 3C,3D). Additionally, a clinical subgroup analysis was conducted to explore the prognostic significance of the GRS in HCC patients with different clinical characteristics, which included the TNM stage, histological grade, gender, and age, and similar results were found (see Figure 3E-3L). Specifically, we found that the low-GRS HCC patients had a longer survival prognosis than the high-GRS patients (all P<0.001). Our findings also showed the prognostic significance of the GRS in different HCC cohorts and clinical subgroups.
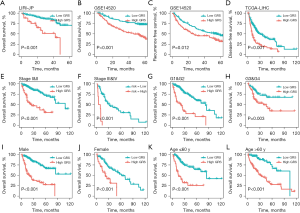
Decision tree and nomogram improved prognostic assessment
To improve the risk stratification of HCC, we established a decision tree based on the clinical characteristics of HCC patients, including age (≥60 or <60 years), gender (male or female), TNM stage (I, II, III, or IV), and GRS (low or high). As Figure 4A shows, only TNM stage and GRS remained in the decision tree. The HCC patients were then classified into 3 different subgroups (low-, intermediate-, and high-risk). The survival analysis showed that there was significant survival differences among the 3 different risk groups (P<0.001; see Figure 4B). Further, the results of the univariate and multivariate Cox analyses suggested that the TNM stage and GRS were the independent clinical factors in the TCGA-LIHC and GSE14520 cohorts (see Table 1). Based on these results, we built a prognostic nomogram that integrated the GRS and traditional TNM stage (see Figure 4C). The calibration and ROC analyses showed that the nomogram was highly accurate at predicting the survival prognosis of HCC patients (see Figure 4D,4E). Additionally, the C-index of the nomogram was 0.74. In summary, these results suggested that our decision tree and nomogram based on the GRS improved the accuracy of prognosis prediction for HCC patients.
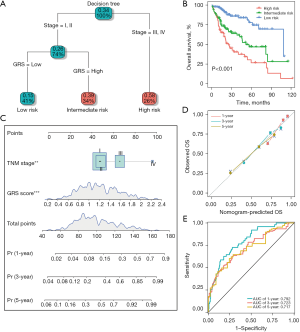
Table 1
Dataset | Variables | Univariate analysis | Multivariate analysis | |||
---|---|---|---|---|---|---|
HR (95% CI) | P | HR (95% CI) | P | |||
TCGA | Age (≥60/<60 years) | 1.119 (0.783–1.599) | 0.537 | 1.127 (0.769–1.653) | 0.539 | |
Gender (Male/Female) | 0.786 (0.547–1.129) | 0.192 | 0.917 (0.619–1.359) | 0.668 | ||
TNM Stage (III+IV/I+II) | 2.781 (1.904–4.062) | <0.001 | 2.506 (1.712–3.669) | <0.001 | ||
GRS score (High/Low) | 2.729 (1.867–3.989) | <0.001 | 2.540 (1.683–3.834) | <0.001 | ||
GSE14520 | Age (≥60/<60 years) | 0.805 (0.453–1.429) | 0.459 | 1.231 (0.670–2.262) | 0.503 | |
Gender (Male/Female) | 1.656 (0.799–3.432) | 0.174 | 1.276 (0.609–2.674) | 0.518 | ||
TNM stage (III+IV/I+II) | 3.415 (2.178–5.356) | <0.001 | 2.999 (1.888–4.764) | <0.001 | ||
GRS score (High/Low) | 2.338 (1.489–3.669) | <0.001 | 2.081 (1.288–3.360) | 0.002 |
HCC, hepatocellular carcinoma; HR, hazard ratio; CI, confidence interval. TNM, tumor-node-metastasis.
Correlations between the GRS and clinical characteristics
We first analyzed the expression of the GRS in HCC tissues, and found that the mRNA expression of ABCB6, AURKA, FKBP4, GMPPA, HK2, HS2ST1, ME1, ME2, NSDHL, and SRD5A3 were significantly increased in the HCC tissues compared to normal tissues (see Figure 5A). Further, we explored the relationship between the GRS and the clinicopathological features in the TCGA-LIHC and GSE14520 cohorts. As Figure 5B,5C show, we found that the HCC patients with stages III–IV had higher GRS risk scores than the HCC patients with stages I–II. Additionally, the GRS risk score was closely associated with the histological grade and BCLC stage (P<0.01; see Figure 5D,5E). Similarly, the GRS risk score also revealed significant differences between the 2 groups of HCC patients in terms of tumor size, tumor multi-nodularity, and AFP (P<0.05; see Figures 5F-5H). However, no significant relationship was found between liver cirrhosis and the GRS risk score in the GSE14520 cohort (see Figure 5I).
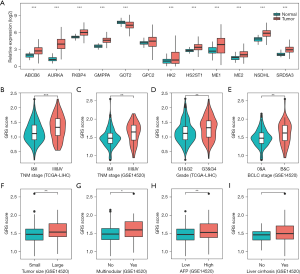
Correlation between the GRS and the tumor immune microenvironment
Immune cells and immune checkpoints were studied to characterize the tumor immune microenvironment of the HCC patients. The relative abundance of 28 immune cells and clinical features in the HCC patients were analyzed using the ssGSEA algorithm. As Figure 6A and Figure 6B show, a significant difference in the immunity signature was observed between the low- and high-GRS groups. Specifically, we found that the HCC patients with high-GRS had a higher abundance of 15 immune cell populations (i.e., Act CD4, Act DC, eosinophil, iDC, Imm B, macrophages, mast, MDSCs, NKT, pDC, Tcm CD4, Tem CD4, Tfh, Th2, and Tregs) than the HCC patients with low-GRS. Additionally, we used the MCP-counter algorithm to calculate the absolute content of the immune cell subsets to confirm the results of the ssGSEA, and similar results were found. Specially, the contents of the CD8+ T cells, fibroblasts, macrophages, monocytes, myeloid dendritic cells, and T cells were more increased in the HCC patients with high-GRS, while the content of the neutrophils was significantly decreased (see Figure 6C). Further, we also examined the association between the GRS and immune checkpoints, and found that the high-GRS patients exhibited higher expression levels for immune checkpoints than the low-GRS patients, especially in the expression of CD86, CXCR4, TGFB1, PDCD1, and CTLA4 (see Figure 6D).
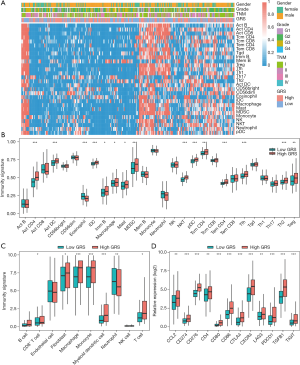
Correlations between the GRS and genomic alterations in HCC
We further examined the association between the genomic alterations and the GRS in the TCGA-LIHC cohort. As Figure 7A,7B show, the top 15 genes with the highest mutation frequencies were analyzed in both the low- and high-GRS cohorts. The top 3 genes with the highest mutation frequencies were CTNNB1 (29%), TTN (23%), and tumor protein p53 (TP53) (16%) in the low-GRS patients, and TP53 (45%), TTN (23%), and CTNNB1 (20%) in the high-GRS patients. Notably, we found that TP53 was the most differentially mutated gene, which suggests that it is significantly correlated to the GRS in HCC patients (P<0.001; see Figure 7C). Additionally, the 10 oncogene-related signaling pathways were also analyzed (see Figure 7D,7E). We found that the WNT- and TP53-related signaling pathways had the highest fraction of samples affected in the low- and high-GRS cohorts, respectively.
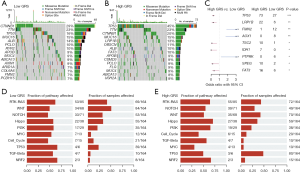
The GRS could guide chemotherapy and immunotherapy in HCC patients
It is widely known that systematic drug therapies (e.g., chemotherapy, targeted therapy, and immunotherapy) improve the prognosis of advanced HCC patients. In this study, we predicted the sensitivity of 4 chemotherapy drugs (i.e., cisplatin, docetaxel, doxorubicin, and gemcitabine) and a targeted drug (i.e., sorafenib) using R package “pRRophetic.” The results showed that the predicted sensitivity of these drugs had significant differences in HCC patients from different GRS groups. Compared to the low-GRS HCC patients, the predicted half maximal inhibitory concentration (IC50) values of cisplatin and docetaxel were significantly decreased in the high-GRS patients, which indicated that the high-GRS patients received a greater clinical benefit (P<0.001; see Figure 8A,8B). However, the low-GRS patients were significantly associated with increased sensitivity to doxorubicin, gemcitabine, and sorafenib relative to the high-GRS HCC patients (P<0.001; see Figure 8C-8E). Further, the IMvigor210 anti-PD-L1 immunotherapy cohort was used to evaluate the value of the GRS in terms of the immunotherapy benefit for HCC patients. For the immunotherapy response, we found that the GRS score in the response patients was significantly more increased than that in the non-response patients (P<0.05; see Figure 8F). In the IMvigor210 cohort, the results also revealed that the high-GRS patients had a poorer prognosis than the low-GRS HCC patients (P=0.003; see Figure 8G). Additionally, our results indicated the rate of objective response to anti-PD-L1 therapy was higher in the high-GRS patients than the low-GRS patients (see Figure 8H). Collectively, these results suggested that the GRS could be used to guide systematic drug therapy for HCC patients.
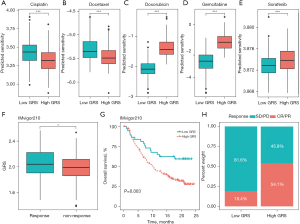
Discussion
This study sought to examine the most important risk factor for the survival prognosis in HCC patients. Using a series of bioinformatics analysis methods, we found that glycolysis was the most important prognostic risk factor for HCC patients. HCC patients with low-glycolysis had a better prognosis and longer survival time than those with high-glycolysis. Glycolysis, which is the most important metabolic feature of solid tumors, refers to a series of reactions that degrade glucose and glycogen into pyruvate accompanied via the production of ATP (25). Compared to normal cells, tumor cells use glycolysis to provide energy to promote their growth and proliferation (26). This may account for the high expression and activity of glycolysis-related enzymes in tumor cells. Several studies have shown that blocking the tumor glycolysis pathway effectively suppresses tumor cell growth and even kills tumors (27-29). Thus, it is of great significance to study the effects of glycolysis on the survival prognosis and treatment responses of HCC patients.
We also examined how to predict the survival prognosis of HCC patients. Currently, the TNM and BCLC staging systems are mainly used in the clinical therapy and prognostic assessments of HCC patients. As HCC is a highly heterogeneous tumor, predictive models based on clinical features cannot accurately predict the survival prognosis of HCC patients. Biomarkers have been shown to improve the accuracy of survival prognosis prediction at the gene level. In this study, the GRS was first constructed to predict the prognosis of HCC patients based on 12 genes (i.e., FKBP4, SRD5A3, ABCB6, ME2, GPC1, ME1, HS2ST1, NSDHL, GMPPA, AURKA, HK2, and GOT2), and further validated in independent cohorts (i.e., the GSE14520 and LIRI-JP cohorts), and different clinical subgroups, including TNM stage, histological grade, gender, and age subgroups. Our data also suggested that high-GRS serves as a risk factor for HCC. Further, all the HCC patients were divided into 3 different risk subgroups by a decision tree based on TNM staging and the GRS. There were significant survival differences among the 3 risk subgroups. Finally, a nomogram with quantification was built based on the GRS to more accurately predict the survival prognosis of patients. These results demonstrated that the GRS improved the risk stratification and prognostic assessment of HCC patients.
We also examined the relationship between glycolysis-related genes and the progression of HCC. We found that the GRS scores were significantly correlated with both the TNM and BCLC stages, and advanced-stage HCC patients had higher GRS scores than early-stage HCC patients. These findings indicated that glycolysis-related genes may contribute to the progression of HCC.ABCB6 is a subtype of the ABC transport family, and the high expression of ABCB6 has been shown to be associated with a worse prognosis (30,31). The biological function of FKBP4 has been reported in many cancers through its interactions with different cellular receptors (32,33). However, to date, the biological role of FKBP4 in HCC has not been examined. Further, ME1 and ME2 are oncogenic genes that have been shown to promote tumor growth and migration in the progression of HCC (34-36). Additionally, AURKA is a serine/threonine kinase that has been shown to be associated with epithelial-mesenchymal transition and cancer stem cells, and plays a critical role in the development of HCC (37,38).
This study also examined how the GRS affects the tumor immune microenvironment. In this study, ssGSEA and MCP-counter algorithms were used to examine the relative content of the immune cells. According to our results, high-GRS HCC patients had a higher percentage of MDSCs, CD4+ T cells, dendritic cells, and macrophages than low-GRS patients. MDSCs are a type of immune cell with high heterogeneity. MDSCs are derived from immature myeloid cells and have immunosuppressive properties. In addition to secreting immunosuppressive cytokines, MDSCs also induce the development of regulatory T cells (39). In this study, the high-GRS HCC patients had higher proportions of MDSC cells, which play a crucial role in the suppression of anti-tumor immunity, than the low-GRS HCC patients. Further, CD4+ T cells have been shown to contribute to the activation of antigen-presenting cells, such as macrophages, dendritic cells, and B cells (40). Regulatory T cells inhibit the activities of CD4+ and CD8+ effector T cells, and NK cells through multiple mechanisms, including the secretion of immunosuppressive cytokines and the production of cytolytic factors (41). These results suggested that high-GRS patients have more immunosuppressive cells, which inhibit anti-tumor immune responses, resulting in a poor prognosis.
This study had several limitations that need to be considered. First, while the prognostic model was validated in two independent cohorts, larger sample sizes and more cohorts are needed to increase the reliability of the results. Second, as a retrospective study, the results of this study may be biased; thus, prospective trials need to be designed and conducted in the future. Third, the prognostic model was established by a series of bioinformatics methods, and its biological role and underlying mechanisms need to be further explored in functional experiments. Thus, future research needs to be conducted to address these issues.
Conclusions
This study developed and validated a glycolysis-related prognostic model to aid in the prediction of the survival and immunotherapeutic response of HCC patients, which may help to guide treatment decisions.
Acknowledgments
Funding: This study was financially supported by the Clinical Research Project of Shanghai Municipal Health Commission (No. 20194Y0357), Project of Shanghai Public Health Clinical Center (No. KY-GW-2021-43), Natural Science Foundation of Shanghai (No. 19ZR1456500), and the Fund of Naval Military Medical University (No. 2018QN17). We also thank for the support of the talent project of Mengchao Wu.
Footnote
Reporting Checklist: The authors have completed the TRIPOD reporting checklist. Available at https://jgo.amegroups.com/article/view/10.21037/jgo-22-503/rc
Conflicts of Interest: All authors have completed the ICMJE uniform disclosure form (available at https://jgo.amegroups.com/article/view/10.21037/jgo-22-503/coif). All authors report that this study was financially supported by the Clinical Research Project of Shanghai Municipal Health Commission (No. 20194Y0357), Project of Shanghai Public Health Clinical Center (No. KY-GW-2021-43), Natural Science Foundation of Shanghai (No. 19ZR1456500), the Fund of Naval Military Medical University (No. 2018QN17), and the support of the talent project of Mengchao Wu. The authors have no other conflicts of interest to declare.
Ethical Statement: The authors are accountable for all aspects of the work in ensuring that questions related to the accuracy or integrity of any part of the work are appropriately investigated and resolved. The study was conducted in accordance with the Declaration of Helsinki (as revised in 2013).
Open Access Statement: This is an Open Access article distributed in accordance with the Creative Commons Attribution-NonCommercial-NoDerivs 4.0 International License (CC BY-NC-ND 4.0), which permits the non-commercial replication and distribution of the article with the strict proviso that no changes or edits are made and the original work is properly cited (including links to both the formal publication through the relevant DOI and the license). See: https://creativecommons.org/licenses/by-nc-nd/4.0/.
References
- Forner A, Reig M, Bruix J. Hepatocellular carcinoma. Lancet 2018;391:1301-14. [Crossref] [PubMed]
- Sung H, Ferlay J, Siegel RL, et al. Global Cancer Statistics 2020: GLOBOCAN Estimates of Incidence and Mortality Worldwide for 36 Cancers in 185 Countries. CA Cancer J Clin 2021;71:209-49. [Crossref] [PubMed]
- Liu CY, Chen KF, Chen PJ. Treatment of Liver Cancer. Cold Spring Harb Perspect Med 2015;5:a021535. [Crossref] [PubMed]
- Pardoll DM. The blockade of immune checkpoints in cancer immunotherapy. Nat Rev Cancer 2012;12:252-64. [Crossref] [PubMed]
- Llovet JM, Montal R, Sia D, et al. Molecular therapies and precision medicine for hepatocellular carcinoma. Nat Rev Clin Oncol 2018;15:599-616. [Crossref] [PubMed]
- Vitale I, Shema E, Loi S, et al. Intratumoral heterogeneity in cancer progression and response to immunotherapy. Nat Med 2021;27:212-24. [Crossref] [PubMed]
- Budhu A, Forgues M, Ye QH, et al. Prediction of venous metastases, recurrence, and prognosis in hepatocellular carcinoma based on a unique immune response signature of the liver microenvironment. Cancer Cell 2006;10:99-111. [Crossref] [PubMed]
- Yang C, Huang X, Liu Z, et al. Metabolism-associated molecular classification of hepatocellular carcinoma. Mol Oncol 2020;14:896-913. [Crossref] [PubMed]
- Nault JC, Villanueva A. Biomarkers for Hepatobiliary Cancers. Hepatology 2021;73:115-27. [Crossref] [PubMed]
- He Y, Lu M, Che J, et al. Biomarkers and Future Perspectives for Hepatocellular Carcinoma Immunotherapy. Front Oncol 2021;11:716844. [Crossref] [PubMed]
- Wang Y, Xie Y, Ma J, et al. Development and validation of a prognostic and immunotherapeutically relevant model in hepatocellular carcinoma. Ann Transl Med 2020;8:1177. [Crossref] [PubMed]
- Du D, Liu C, Qin M, et al. Metabolic dysregulation and emerging therapeutical targets for hepatocellular carcinoma. Acta Pharm Sin B 2022;12:558-80. [Crossref] [PubMed]
- Murciano-Goroff YR, Warner AB, Wolchok JD. The future of cancer immunotherapy: microenvironment-targeting combinations. Cell Res 2020;30:507-19. [Crossref] [PubMed]
- Pavlova NN, Thompson CB. The Emerging Hallmarks of Cancer Metabolism. Cell Metab 2016;23:27-47. [Crossref] [PubMed]
- Bader JE, Voss K, Rathmell JC. Targeting Metabolism to Improve the Tumor Microenvironment for Cancer Immunotherapy. Mol Cell 2020;78:1019-33. [Crossref] [PubMed]
- Vander Heiden MG, DeBerardinis RJ. Understanding the Intersections between Metabolism and Cancer Biology. Cell 2017;168:657-69. [Crossref] [PubMed]
- Mariathasan S, Turley SJ, Nickles D, et al. TGFbeta attenuates tumour response to PD-L1 blockade by contributing to exclusion of T cells. Nature 2018;554:544-8. [Crossref] [PubMed]
- Liberzon A, Birger C, Thorvaldsdóttir H, et al. The Molecular Signatures Database (MSigDB) hallmark gene set collection. Cell Syst 2015;1:417-25. [Crossref] [PubMed]
- Liberzon A, Subramanian A, Pinchback R, et al. Molecular signatures database (MSigDB) 3.0. Bioinformatics 2011;27:1739-40. [Crossref] [PubMed]
- Ru B, Wong CN, Tong Y, et al. TISIDB: an integrated repository portal for tumor-immune system interactions. Bioinformatics 2019;35:4200-2. [Crossref] [PubMed]
- Becht E, Giraldo NA, Lacroix L, et al. Estimating the population abundance of tissue-infiltrating immune and stromal cell populations using gene expression. Genome Biol 2016;17:218. [Crossref] [PubMed]
- Mayakonda A, Lin DC, Assenov Y, et al. Maftools: efficient and comprehensive analysis of somatic variants in cancer. Genome Res 2018;28:1747-56. [Crossref] [PubMed]
- Yang W, Soares J, Greninger P, et al. Genomics of Drug Sensitivity in Cancer (GDSC): a resource for therapeutic biomarker discovery in cancer cells. Nucleic Acids Res 2013;41:D955-61. [Crossref] [PubMed]
- Geeleher P, Cox N, Huang RS. pRRophetic: an R package for prediction of clinical chemotherapeutic response from tumor gene expression levels. PLoS One 2014;9:e107468. [Crossref] [PubMed]
- Feng J, Li J, Wu L, et al. Emerging roles and the regulation of aerobic glycolysis in hepatocellular carcinoma. J Exp Clin Cancer Res 2020;39:126. [Crossref] [PubMed]
- Vander Heiden MG, Cantley LC, Thompson CB. Understanding the Warburg effect: the metabolic requirements of cell proliferation. Science 2009;324:1029-33. [Crossref] [PubMed]
- Liberti MV, Dai Z, Wardell SE, et al. A Predictive Model for Selective Targeting of the Warburg Effect through GAPDH Inhibition with a Natural Product. Cell Metab 2017;26:648-659.e8. [Crossref] [PubMed]
- Ma R, Zhang W, Tang K, et al. Switch of glycolysis to gluconeogenesis by dexamethasone for treatment of hepatocarcinoma. Nat Commun 2013;4:2508. [Crossref] [PubMed]
- Yang J, Jin X, Yan Y, et al. Inhibiting histone deacetylases suppresses glucose metabolism and hepatocellular carcinoma growth by restoring FBP1 expression. Sci Rep 2017;7:43864. [Crossref] [PubMed]
- Tsunedomi R, Iizuka N, Yoshimura K, et al. ABCB6 mRNA and DNA methylation levels serve as useful biomarkers for prediction of early intrahepatic recurrence of hepatitis C virus-related hepatocellular carcinoma. Int J Oncol 2013;42:1551-9. [Crossref] [PubMed]
- Zhang J, Zhang X, Li J, et al. Systematic analysis of the ABC transporter family in hepatocellular carcinoma reveals the importance of ABCB6 in regulating ferroptosis. Life Sci 2020;257:118131. [Crossref] [PubMed]
- Zong S, Jiao Y, Liu X, et al. FKBP4 integrates FKBP4/Hsp90/IKK with FKBP4/Hsp70/RelA complex to promote lung adenocarcinoma progression via IKK/NF-kappaB signaling. Cell Death Dis 2021;12:602. [Crossref] [PubMed]
- Mangé A, Coyaud E, Desmetz C, et al. FKBP4 connects mTORC2 and PI3K to activate the PDK1/Akt-dependent cell proliferation signaling in breast cancer. Theranostics 2019;9:7003-15. [Crossref] [PubMed]
- Mihara Y, Akiba J, Ogasawara S, et al. Malic enzyme 1 is a potential marker of combined hepatocellular cholangiocarcinoma, subtype with stem-cell features, intermediate-cell type. Hepatol Res 2019;49:1066-75. [Crossref] [PubMed]
- Lee D, Zhang MS, Tsang FH, et al. Adaptive and Constitutive Activations of Malic Enzymes Confer Liver Cancer Multilayered Protection Against Reactive Oxygen Species. Hepatology 2021;74:776-96. [Crossref] [PubMed]
- Zhang S, Cheng ZM, Yu JL, et al. Malic enzyme 2 promotes the progression of hepatocellular carcinoma via increasing triglyceride production. Cancer Med 2021;10:6795-806. [Crossref] [PubMed]
- Chen C, Song G, Xiang J, et al. AURKA promotes cancer metastasis by regulating epithelial-mesenchymal transition and cancer stem cell properties in hepatocellular carcinoma. Biochem Biophys Res Commun 2017;486:514-20. [Crossref] [PubMed]
- Dauch D, Rudalska R, Cossa G, et al. A MYC-aurora kinase A protein complex represents an actionable drug target in p53-altered liver cancer. Nat Med 2016;22:744-53. [Crossref] [PubMed]
- Groth C, Hu X, Weber R, et al. Immunosuppression mediated by myeloid-derived suppressor cells (MDSCs) during tumour progression. Br J Cancer 2019;120:16-25. [Crossref] [PubMed]
- Savage PA, Klawon DEJ, Miller CH, Regulatory T. Cell Development. Annu Rev Immunol 2020;38:421-53. [Crossref] [PubMed]
- Shevyrev D, Tereshchenko V. Treg Heterogeneity, Function, and Homeostasis. Front Immunol 2019;10:3100. [Crossref] [PubMed]