Bioinformatics analysis of the prognostic and immunotherapeutic significance of NPRL2 in stomach adenocarcinoma
Introduction
Stomach adenocarcinoma (STAD) is the most predominant type of gastric malignancy. Over the past few decades, adjuvant chemotherapy and surgery have improved the survival of patients with early gastric cancer. However, gastric cancer is still the fourth leading contributor to cancer mortality (1). The development of comprehensive management involving radiotherapy, combinations of chemotherapy, targeted therapy, and immunotherapy has significantly improved the survival and quality of life of patients with advanced gastric cancer (2). Research on biomarkers, such as HER2, tumour-infiltrating immune cells (TICS), and microsatellite instability (MSI), has not only provided predictors for the prognosis of advanced gastric cancer but also identified biological targets for individualized treatments (3). However, it is common for HER-2-positive patients to turn negative after trastuzumab treatment, often leading to treatment failure, while anti-HER-2 therapy does not benefit HER-2-negative patients or those with HER-2 gene amplification (2). Patients with high MSI are thought to be well responded to immunotherapy; however, the incidence of MSI in gastric cancer is low and the number of analyses of MSI gastric cancers remains limited (3). In addition, due to the heterogeneity of cancer, changes in different markers can occur in different subsets of patients (2). Since individual cancer marker lacks the sensitivity or specificity for broad application (4), further exploration of other markers is needed for better combinatorial detection and individualised treatment.
GATOR complex protein NPRL2, also known as TUSC4, is a 380aa highly conserved protein containing a nitrogen permease regulator 2 domain (5). NPRL2 is an important component in the mechanistic target of the rapamycin (mTOR) signalling pathway. NPRL2 interacts with DEPDC5 and NPRL3 to form the GATOR1 complex, which acts as a negative regulator of mTOR complex 1 and participates in the regulation of various downstream cellular functions, including cell growth, proliferation, and metabolism (6). In recent years, the role of NPRL2 in human diseases, especially in cancers, has been characterised (7). NPRL2 expression is down-regulated and acts as a tumour suppressor gene in a variety of cancers, including lung, renal, breast and colorectal cancers (8-11). NPRL2 overexpression in non-small cell lung cancer cells can inhibit tumour growth and metastasis and induce apoptosis (8). In addition, upregulation of NPRL2 can reverse cisplatin resistance and increase chemosensitivity by promoting apoptosis, increasing the kinase activity of CHK1 and CHK2, and activating the DNA damage checkpoint pathway (12). In digestive cancers, NPRL2 also exerts a potential anti-cancer role. NPRL2 was found to be an independent prognostic factor in patients with liver cancer, and high expression of NPRL2 was found significantly associated with higher survival rates of patients (13). By contrast, in colon cancers, reduced expression of NPRL2 in the blood was strongly associated with poor prognosis, and thus, NPRL2 could be a biomarker for the early diagnosis of colon cancer (14). Liu et al. revealed that NPRL2 can regulate the PI3K/AKT/mTOR signalling and enhance the sensitivity of colon cancer cells to 5-fluorouracil treatment (10). Given the importance of NPRL2 in various cancer types, a comprehensive analysis of NPRL2 in gastric adenocarcinoma may provide insights into the identification of new markers for gastric adenocarcinoma.
In this study, we applied various bioinformatic methods to comprehensively analyse the expression of NPRL2 and its role in STAD from the perspective of associations to patient prognosis, genomic methylation, functional enrichment, drug resistance, immunity, and survival risk models. We found that NPRL2 and its related genes could be new prognostic and immunotherapeutic biomarkers for gastric cancer and provided insights for risk assessment and the development of individualized therapeutic targets for gastric cancer patients. We present the following article in accordance with the TRIPOD reporting checklist (available at https://jgo.amegroups.com/article/view/10.21037/jgo-22-115/rc).
Methods
Study design
This is a bioinformatics analysis study based on publicly available databases of gastric adenocarcinoma transcriptomic data and clinical information. This study was conducted in accordance with the Declaration of Helsinki (as revised in 2013). The research process was presented as a flow chart in Figure 1.
Data acquisition and correlation analysis
The expression profile of NPRL2 in pan-cancer and immunohistochemical staining of NPRL2 protein in gastric cancer were obtained on the Human Protein Atlas (https://www.proteinatlas.org/) (15). The differential expression of NPRL2 in STAD compared with normal tissue across different studies was presented with the Oncomine database (https://www.oncomine.org/) (16). Transcriptome expression, survival prognosis and other clinical covariates (age, gender, tumour grade and tumour stage) of 407 samples (375 STAD and 32 normal tissues) were downloaded from The Cancer Genome Atlas (TCGA, https://portal.gdc.cancer.gov/). The gene expression and clinical data of the Gene Expression Omnibus (GEO) validation cohort were acquired from the GSE84437 dataset (17). Samples without complete survival information or target gene expression data were discarded.
Prognostic value analysis
Patients in TCGA cohort were divided into 2 groups based on the optimal cut-off value generated by the “surv_cutpoint” function of the “survminer” R package (18). Kaplan-Meier method and log-rank test were used in R 3.6.3 (RRID: SCR_001905) to assess the effect of factors on the survival of patients. The Kaplan-Meier Plotter (KM plotter, https://kmplot.com/analysis/) (19) was employed to validate the impact of NPRL2 on the clinical outcomes of patients. The correlations between NPRL2 and tumour stage or grade were estimated on TISIDB (20). The expression of NPRL2 in patients with different stage cancers in TCGA was compared with one way ANOVA on GEPIA2 (https://gepia2.cancer-pku.cn/#index) (21).
Genomic methylation analysis
Methylation raw data of STAD in the GSE30601 dataset (22) were imported and normalized with the “minfi” R package (23). The methylation profile of NPRL2 were downloaded from UALCAN (https://ualcan.path.uab.edu/) (24) and DiseaseMeth 2.0 (https://bio-bigdata.hrbmu.edu.cn/diseasemeth/) (25). Finally, the correlations among gene expression, methylation value and clinical data were analysed in MEXPRESS (26).
Drug-protein interaction analysis
The drug-protein docking model was constructed with the Dock module in the molecular operating environment (MOE 4.5.0) (Chemical Computing Group, Montreal, QC, Canada). The molecular structures of paclitaxel and GATOR1 were first obtained from PubChem (https://pubchem.ncbi.nlm.nih.gov) and the Protein Data Bank database (https://www.rcsb.org/), respectively. The energy of drug molecules and protein crystal structures was minimized by using the Energy minimize and Protonate 3D modules with the default settings, while the Ramachandran diagrams were generated with the “Phi-Psi plot” module in MOE.
NPRL2-related gene and functional analysis
The protein-protein interaction (PPI) network for NPRL2 was constructed in STRING (https://string-db.org/) (27) with the following parameters: active interaction sources-Experiments, minimum required interaction score-low confidence (0.150). The interaction between proteins in the PPI network was quantified as degree and binding scores using cytoHubba in CytoScape (3.8.2). Genes co-expressed with the NPRL2 gene were analysed in the “Similar Genes Detection” module of GEPIA 2 (21). Functional enrichment analysis including Kyoto Encyclopedia of Genes and Genomes (KEGG) analysis and gene ontology (GO) analysis was performed with the “clusterProfiler” package in R (28).
Immune infiltration and immunotherapy analysis
The ImmuneScore, StromalScore and ESTIMATEScore of STAD were calculated using the “ESTIMATE” R package (29) based on the transcriptomic data from the TCGA cohort. The responsiveness of each sample to immunotherapy was predicted using Immune Cell Abundance Identifier (ImmuCellAI, https://bioinfo.life.hust.edu.cn/ImmuCellAI#!/) (30). The relationship between NPRL2 and 28 types of immune cells was estimated on TISIDB (20). In addition, the sensitivity of STAD samples to immunotherapy was predicted by Tumour Immune Dysfunction and Exclusion (TIDE, https://tide.dfci.harvard.edu/) (31) based on multiple published transcriptomic biomarkers and the expression profile of the tumour and presented as TIDE Score. The SangerBox online tool (https://sangerbox.com/Tool) was employed to perform the correlation analysis of NPRL2 with immunotherapeutic markers.
Construction of prognostic risk model
The expression data of NPRL2-related genes and clinical data of STAD in the TCGA cohort and GEO validation cohort were used to construct the prognostic risk model. Transcription data were normalized and batch effects in different cohorts were eliminated in R program. Genes that had significant effects (P<0.05) on the prognosis were identified using the univariate Cox analysis and further screened using the least absolute shrinkage and selection operator (LASSO). The prognosis-related genes obtained were used to construct Cox regression prognostic risk models and to generate risk scores for each sample. High and low-risk groups were distinguished based on the risk score of each sample. Risk score = ∑ (gene expression × coefficient). The impact of risk scores on patient prognosis was analysed with the Kaplan-Meier curve and univariate and multivariate Cox regression analyses. And the predictive performance of the risk model was assessed by generating nomograms for the prediction of survival probabilities and plotting the receiver operating characteristic (ROC) curves and calibration curves using the clinical information and risk scores. Area under ROC curve (AUC) value >0.5 indicates that the prognostic model has a predictive effect.
Statistical analysis
Propensity score matching by inverse probability of treatment weighting (IPTW) was performed to reduce selection bias and balance covariates between patients predicted to respond to immunotherapy and those who did not (32,33). Characteristics matched by propensity score included age, gender, tumour grade and tumour stage. Differential analyses between two groups of data were performed by the t-test. The correlation analyses were performed with Spearman’s rank correlation. All statistical tests were two-sided and P<0.05 was considered to be statistically significant.
Results
The expression of NPRL2 was downregulated in human STAD and associated with methylation.
RNA sequencing data on the Human Protein Atlas revealed that NPRL2 had a relatively low expression in STAD among pan-cancer (Figure 2A) (15). Analysis of several RNA sequencing studies in the Oncomine database suggested that NPRL2 mRNAs were significantly downregulated in different subtypes of STAD, including intestinal type, diffuse and mixed STAD (Figure 2B,2C), compared to normal gastric tissue. Immunohistochemistry images from the Human Protein Atlas also confirmed the downregulation of NPRL2 expression at the protein level in gastric cancer (Figure 2D) (15). The occurrence and development of gastric cancer were associated with abnormal methylation of tumour suppressor genes (34). Methylation data of 297 samples from the GSE30601 dataset (22) (including 203 STAD samples and 94 normal samples) were used to further verify differential methylation between STAD and normal tissue (Figure S1A). The average methylation level of NPRL2 in STAD was much higher than that in normal tissues (Figure 3A, P<0.001), consistent with the methylation data of cg16470957 and cg27239147 probes (Figure S1B). The data from the DiseaseMeth database also verified that the methylation level of NPRL2 was relatively low in normal samples (Figure 3B,3C). Using the UALCAN database (24), we found that patients with Grade 3 STAD had significantly higher levels of methylation at the NPRL2 promoter than patients with Grade 2 STAD (Figure 3D). We also observed that the high level of methylation of NPRL2 in STAD was correlated with the low expression of the gene, as shown in Figure S1C, and that most methylation of probes was negatively correlated with the expression of NPRL2. These results suggested that the abnormal methylation of the NPRL2 gene might be contributory to the low expression of NPRL2 in STAD.
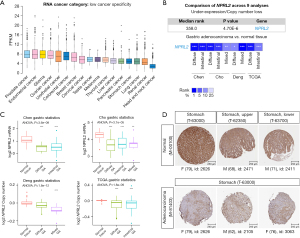
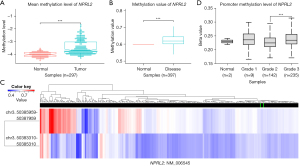
NPRL2 expression in STAD was positively associated with prognosis
In pan-cancer from TCGA, NPRL2 expression was found to be negatively correlated with grade and stage in STAD patients (Figure 4A,4B), and ANOVA analysis also showed that NPRL2 was differentially expressed in different cancer stages in the GEPIA database (21) (Figure 4C, P=0.0334). To determine the association between the expression of NPRL2 and the prognosis of STAD patients, survival analysis was conducted using the gene expression data and clinical information of STAD patients from TCGA. Patients with high expression of NPRL2 had significantly longer overall survival (OS, P=0.0225), progression-free interval (PFI, P=0.004), and disease-specific survival (DSS, P=0.005) than those with lower expression of NPRL2, though no difference was found between the two groups in the disease-free interval (DFI, P=0.113) (Figure 4D). A similar positive association was also found between NPRL2 expression and prognosis based on OS data from the Human Protein Atlas database (Figure 4E, P=0.046). In addition, using data accessed from the KM plotter (19), we found that STAD patients with low expression of NPRL2 had poorer OS (P=0.021) and first progression (FP, P=0.021) than patients high NPRL2 expression with (Figure 4F). Furthermore, the meta-analysis of survival data in gastric cancer cases based on the expression level of the NPRL2 suggested that NPRL2 was an important protective factor for gastric cancer (Figure S2, HR =0.78, 95% CI: 0.69–0.87, P<0.01). Next, clinical information of patients with different pathological characteristics accessed from the KM plotter was used for further survival analysis (Table S1), NPRL2 was found to be potentially beneficial for patients of different tumour-node-metastasis (TNM) stages and sexes, while for Lauren classification, NPRL2 could affect the prognosis of patients with diffuse STAD. Interestingly, NPRL2 might have a beneficial effect on patients treated with surgery alone and adjuvant chemotherapy other than 5-fluorouracil-based therapies. Notably, NPRL2 was a protective factor in patients with HER2 negative but had no significant effect on HER2-positive patients. The evidence above suggested that NPRL2 might be an inhibitory factor of STAD, and the high expression of NPRL2 was associated with a better prognosis in STAD patients.
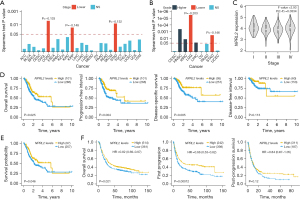
NPRL2 regulated the epithelial-mesenchymal transition (EMT) and stemness of STAD
Considering the effect of NPRL2 on the prognosis of STAD, we next investigated the relationship between NPRL2 and the malignant behaviour of cancer. In STAD, NPRL2 was positively correlated with the expression of epithelial marker CDH1, while negatively correlated with the expression of mesenchymal markers VIM and EMT regulators, ZEB1 and SNAI2 (Figure 5A,5B, Figure S3A), which were hallmarks for distal tumour metastasis (35-37). We found a negative correlation between NPRL2 and CD44 expression (Figure 5B), a marker for gastric cancer stem cells (38), implicating the potential role of NPRL2 in the regulation of EMT and stemness of STAD cells.
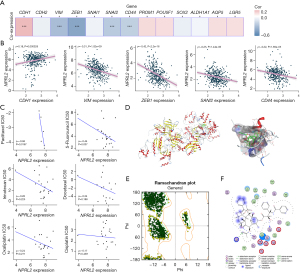
To further clarify the mechanism underlying the role of NPRL2 in the malignant behaviour of cancer, the PPI network of NPRL2 was constructed on STRING (27) to identify proteins that interacted with NPRL2. The PPI network of NPRL2 found 48 proteins that had been experimentally confirmed to interact with NPRL2 (Figure S3B). Additionally, the top 100 genes co-expressed with NPRL2 in STAD were also identified using GEPIA 2 (21) (P<0.001) (Figure S3C, Table S2). These genes represented a subset of genes whose function and regulation were closely related to NPRL2. We explored this gene set with gene enrichment analysis for KEGG terms and GO terms. Gene enrichment analysis found that NPRL2 and NPRL2-related genes were mainly involved in the mTOR signalling pathways, autophagy, cellular response to amino acid starvation, and RNA transport (Figure S3D,S3E). At the molecular level, these genes were mainly located in the vacuolar membrane, lysosome membrane, lytic vacuole membrane, and GATOR2 complex, performing the molecular function of basic amino acid transmembrane transporter activity, and protein serine/threonine kinase activity (Figure S3E). The mTOR signalling pathway was a key pathway that regulated EMT and cancer stem cells (39,40). These findings suggested that NPRL2 might influence EMT, and stemness of STAD cells by regulating mTOR signalling.
NPRL2 regulated the sensitivity of STAD cells to chemotherapy drugs
Chemotherapy resistance is still the main cause of poor prognosis in STAD patients (41). Previous studies have shown that NPRL2 can overcome chemotherapy resistance to prostate, and colon cancer to a certain extent (10,42). To explore whether NPRL2 had a similar effect in STAD, the correlation between the expression of NPRL2 and chemotherapeutic sensitivity was analysed in STAD cell lines. Based on pharmacogenomic data from the GDSC database (43), mRNA expression of NPRL2 was found significantly negatively correlated with IC50 values for paclitaxel (ρ=−0.94, P=0.0167) and moderately negatively correlated with 5-fluorouracil (ρ=−0.44, P=0.037) (Figure 5C), indicating that STAD cells with a high expression of NPRL2 were more sensitive to paclitaxel and 5-fluorouracil. We hypothesized that paclitaxel could interact with NPRL2 to enhance chemotherapeutic sensitivity. In silico validation using a MOE-constructed drug-protein docking model suggested that paclitaxel was able to steadily bind to the GATOR1 protein complex, which is formed by NPRL2, NPRL3, and DEPDC5, at Arg202, Ser417, and Glu419 residues of NPRL3 (S=−8.3761, RMSD =1.92Å) (Figure 5D,5F). Therefore, GATOR1 might act to reverse drug resistance through the direct binding to paclitaxel.
NPRL2 affected immune infiltration and immunotherapy in STAD
The tumour microenvironment (TME) is composed of matrix cells, fibroblasts, endothelial cells and immune cells and is very important to the occurrence and development of tumours. The interaction between tumour cells and TME affects tumour growth, progression, invasion and metastasis (44). The mutual regulation and dynamic balance of tumour cells and immune cells are closely related to the prognosis of patients (45). To explore the correlation between NPRL2 and non-tumour cells, the tumour purity and proportion of immune and stromal cells of the STAD samples were estimated using the ESTIMATE package (29). The expression of NPRL2 was negatively correlated with the ImmuneScore, StromalScore and ESTIMATEScore of STAD, indicating a high proportion of immune cells and stromal cells in STAD tissue with low expression of NPRL2 (Figure 6A). As Figure S4 showed, NPRL2 was negatively correlated with the infiltration levels of most types of TICS (Table S3). We then estimated the immune infiltration scores of the STAD sample using ImmuCellAI (30) and found that NPRL2 showed a significant negative correlation with the immune infiltration score (Figure 6B, ρ=−0.27, P=1.59e-07), and patients with higher immune infiltration had a poorer prognosis (Figure 6C, P=0.011). In addition, the immunotherapy-responsive group (n=122) had a much lower infiltration score (Figure 6D, P<0.001) but a higher NPRL2 expression (Figure 6E, P<0.001) and a better survival probability (Figure 6F, P=0.038) than the non-responsive group (n=253), suggesting a potential link between NPRL2, immune infiltration and responsiveness to immunotherapy.
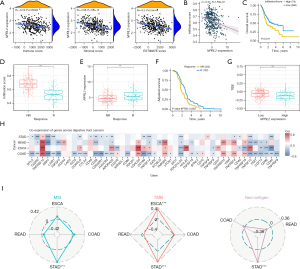
One of the most important reasons for the deficiency of anti-tumour capacity in tumour-infiltrating immune cells is that the immune checkpoints signalling pathway, represented by PD-1 and CTLA-4, can suppress anti-tumour immunity and create a microenvironment conducive to tumour growth (46). Immune checkpoint inhibitor (ICI) targeting immune checkpoint is currently one of the predominant immunotherapy methods for multiple cancers and the role of ICIs in gastric cancer has been tentatively confirmed (2,47). ICIs could improve the survival of patients with chemo-refractory gastric cancer and were more effective when used in combination with chemotherapy than chemotherapy alone (3). TIDE prediction found that patients with lower expression of NPRL2 had higher TIDE scores (Figure 6G, high: 105, low: 270, P<0.001), indicating an unfavourable response to ICIs in these patients (31). Co-expression analysis found that NPRL2 had a negative relationship with the expression of co-inhibitory immune checkpoints in gastrointestinal tumours, such as PDCD1LG2, LAIR1, and BTLA. Moreover, NPRL2 was also positively correlated with co-stimulatory immune checkpoints TNFSF9 and TNFRSF14 (48), suggesting a potential association between NPRL2 and immune signalling pathways (Figure 6H). Notably, NPRL2 was significantly positively correlated with immunotherapy prognostic markers, such as MSI, tumour mutation burden (TMB), and neo-antigen in STAD, but not in other gastrointestinal tumours (Figure 6I). These results suggested that NPRL2 might affect the infiltration levels of immune cells and be a potential immunotherapy marker for STAD.
The construction of the NPRL2-related gene prognostic risk model
To further evaluate the prognostic value of NPRL2-related genes, cox regression of the 148 NPRL2-related genes obtained from the PPI network and GEPIA 2 was conducted to obtain genes associated with STAD prognosis (Figure S5A), 9 of which were screened out with the LASSO algorithm (Figure 7A). In the risk model constructed with Cox regression, STAD patients were divided into high- and low-risk groups based on risk scores. SLC7A1, RTN3, TMEM115, C1orf109, SHQ1, and RPP14 were enriched as beneficial genes in the low-risk group of patients with better clinical outcomes, while SLC7A3, NPR3, and LRCH2 were enriched in the high-risk group as unfavourable factors (Figure 7B). Univariate Cox regression and survival analysis suggested that the risk score was strongly associated with the worse prognosis of STAD patients (HR =4.545, 95% CI: 2.589–7.981, P<0.001), and multivariate Cox risk regression analysis showed that the risk score was an independent prognostic factor for STAD patients (HR =4.855, 95% CI: 2.683–8.785, P<0.001) (Figure 7C-7E). To validate the applicability of the risk model, we constructed a Nomogram based on the patients’ clinical information and risk scores to predict patients’ survival probability (Figure 7F). In the risk model, the risk scores had an AUC value of 0.641 (cut value =−0.263), which was better than other clinical characteristics (Figure 7G). In addition, calibration curves demonstrated that the prognostic model could effectively predict the probability of patient survival at 3 and 5 years (Figure 7H,7I). Additional cohort using data of patients with STAD in the GSE84437 dataset validated the constructed prognostic model (Figure S5B), where the high risk score was a risk factor for patients’ prognosis, with an AUC value of 0.605 for the Nomogram (cut value =−0.317), and predicted survival at 3 and 5 years of patients was highly consistent with actual results (Figure S5C-S5I). Given these findings, NPRL2-associated genes would be useful in prognosis prediction for STAD.
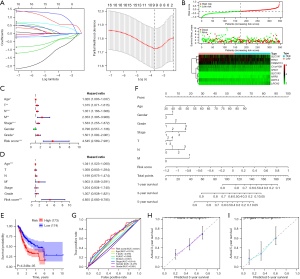
Discussion
The present study highlighted the candidate tumour suppressor gene NPRL2 was a potential prognostic predictive molecule in gastric adenocarcinoma and might serve as a new immunotherapeutic biomarker. Specifically, NPRL2 expression was lower in STAD than in normal tissue, and NPRL2 expression was associated with better overall survival, progression-free interval, and disease-specific survival in patients. NPRL2 negatively correlated with the expression of the cancer invasion, stemness, immune cell infiltration, and sensitivity to chemotherapeutic agents in gastric cancer cells, while positively correlated with immunotherapy biomarkers.
In previous studies, NPRL2 was found to be under-expressed in a variety of cancers and acts as a tumour suppressor gene, including lung, kidney, breast, colorectal cancer and glioma, which was consistent with our study, but the opposite in prostate cancer (8-11,49,50). In comparison, our study provided a more comprehensive analysis of the differential expression and prognostic value of NPRL2 in STAD based on a large number of samples from multiple databases and multiple survival outcome indicators.
EMT and gastric cancer stem cells underlie the development of metastasis in gastric cancer and are important causes of refractory and recurrent gastric cancer (37,51). Our study revealed that NPRL2 might regulate the malignant biological behaviour of gastric cancer by affecting the EMT regulators and stemness of cells, which was consistent with Ji et al.’s study in lung cancer (8), where NPRL2 was able to inhibit the growth and metastasis of cancer cells. Functional enrichment analysis indicated that NPRL2-related genes were associated with mTOR signalling, which was considered a potential target to inhibit the invasive metastasis of many cancer cells (39). The mTOR signalling pathway was also involved in cancer cell proliferation and EMT, promoted cancer stem cell survival, stemness maintenance and tumorigenic capacity, and was associated with the metabolism of cancer stem cells (40). Bar-Peled et al. (6) have revealed that NPRL2 was an inhibitor of mTOR signalling and that cells with NPRL2 mutation were sensitive to rapamycin treatment. Based on the evidence above, we cautiously proposed that NPRL2 might inhibit EMT and stemness in gastric adenocarcinoma through the inhibition of the mTOR signalling pathway.
In addition, our study found that in STAD cells, NPRL2 expression was positively associated with the drug sensitivity of 5-fluorouracil and Paclitaxel, chemotherapy drugs commonly used in STAD, consistent with Liu et al.’s study where NPRL2 was able to enhance colon cancer cells sensitivity to 5-fluorouracil (10). Kurata et al. (52) also found that the high expression of NPRL2 indirectly enhanced the sensitivity of cancer cells to anti-cancer drugs, including cisplatin, doxorubicin, paclitaxel, VP-16, and 17-AAG. Unfortunately, our study did not yield positive results in docetaxel, irinotecan, oxaliplatin, and cisplatin due to the small sample size. Previous studies have reported some mechanisms by which NPRL2 affected drug sensitivity (10,12,52-53). The overexpression of NPRL2 activated the DNA damage checkpoint pathway to resensitise drug-resistant cells to cisplatin treatment (12). NPRL2 inhibited PDK1 activation through binding to PDK1 and affected downstream molecules to enhance the sensitivity of cancer cells to anticancer drugs (52). NPRL2 also affected the PI3K/Akt/mTOR signalling and inhibited the activation of the multidrug resistance transporter proteins P-gp and MRP1 to reverse 5-fluorouracil resistance in colorectal cancer (10,53). In our study, KEGG analysis also suggested that genes co-expressed with NPRL2 were significantly enriched for genes regulating autophagy, which were essential for the regulation of gastric cancer chemotherapy resistance (41). Autophagy cut both ways in disease according to the different types and stages. For instance, Chen et al. (54) found that abnormally upregulated NPRL2 in castration-resistant prostate cancer inhibited the mTOR signalling pathway, induced autophagy, and ultimately led to the development of chemotherapy drug resistance. On the contrary, in anaplastic thyroid carcinoma, mTOR inhibitors promoted autophagy to sensitize cancer cells to paclitaxel (55). We also found that NPRL2 might have an indirect interaction with paclitaxel via NPRL3 and the effect of the interaction on the sensitivity of cancer cells to drugs needed to be confirmed by experiments. Whether NPRL2 regulated the sensitivity of chemotherapeutic agents for gastric adenocarcinoma through autophagy and mTOR signalling pathways or other mechanisms reported warrants further investigation.
Immunotherapy is a promising treatment for chemotherapy-resistant cancers and clinical trials by Kang et al. (56) have confirmed the survival benefits of ICIs for patients with refractory gastric cancer. Immune cells in the TME are the basis of immunotherapy, and the infiltration abundance of tumour immune cells may be a predictor of prognosis and response to immunotherapy in cancer patients (57). In our study, we found that NPRL2 was negatively correlated with infiltration score and patients with high infiltration scores had a worse prognosis using the ESTIMATE algorithm and ImmuCellAI and Li et al. (58) also proved a high immune score predicted a poorer outcome in gastric cancer based on the CIBERSORT algorithm and the Cox model. Furthermore, the predictive value of MSI, TMB and neo-antigen for immunotherapy has been demonstrated in a variety of cancers (59-61). In the present study, these immunomarkers were positively correlated with the expression of NPRL2 in STAD, and TIDE score prediction also indicated that patients with high expression of NPRL2 were more responsive to immunotherapy. Altogether, NPRL2 is expected to become a new immunotherapy marker for STAD. Furthermore, considering individual differences and high heterogeneity of tumours, future large-scale immunotherapy cohort data may help to elucidate the predictive value of NPRL2 in the immunotherapy of gastric adenocarcinoma.
Acknowledgments
We would like to thank PhD candidate Zongze Li from Cardiff University (Cardiff, UK) for his invaluable assistance in proofreading and providing invaluable advice on scientific writing.
Funding: The study was funded by “Effect and Mechanism of NK cells combined with PD1 Inhibitors on Immunotherapy of Gastric Cancer” from Tian Qing Stem Cell.
Footnote
Reporting Checklist: The authors have completed the TRIPOD reporting checklist. Available at https://jgo.amegroups.com/article/view/10.21037/jgo-22-115/rc
Conflicts of Interest: All authors have completed the ICMJE uniform disclosure form (available at https://jgo.amegroups.com/article/view/10.21037/jgo-22-115/coif). The authors have no conflicts of interest to declare.
Ethical Statement: The authors are accountable for all aspects of the work in ensuring that questions related to the accuracy or integrity of any part of the work are appropriately investigated and resolved. This study was conducted in accordance with the Declaration of Helsinki (as revised in 2013).
Open Access Statement: This is an Open Access article distributed in accordance with the Creative Commons Attribution-NonCommercial-NoDerivs 4.0 International License (CC BY-NC-ND 4.0), which permits the non-commercial replication and distribution of the article with the strict proviso that no changes or edits are made and the original work is properly cited (including links to both the formal publication through the relevant DOI and the license). See: https://creativecommons.org/licenses/by-nc-nd/4.0/.
References
- Sung H, Ferlay J, Siegel RL, et al. Global Cancer Statistics 2020: GLOBOCAN Estimates of Incidence and Mortality Worldwide for 36 Cancers in 185 Countries. CA Cancer J Clin 2021;71:209-49. [Crossref] [PubMed]
- Siebenhüner AR, De Dosso S, Helbling D, et al. Advanced Gastric Cancer: Current Treatment Landscape and a Future Outlook for Sequential and Personalized Guide: Swiss Expert Statement Article. Oncol Res Treat 2021;44:485-94. [Crossref] [PubMed]
- Smyth EC, Nilsson M, Grabsch HI, et al. Gastric cancer. Lancet 2020;396:635-48. [Crossref] [PubMed]
- Machlowska J, Baj J, Sitarz M, et al. Gastric Cancer: Epidemiology, Risk Factors, Classification, Genomic Characteristics and Treatment Strategies. Int J Mol Sci 2020;21:4012. [Crossref] [PubMed]
-
. Available online: https://www.ncbi.nlm.nih.gov/homologene/?term=NPRL2.HomoloGene - Bar-Peled L, Chantranupong L, Cherniack AD, et al. A Tumor suppressor complex with GAP activity for the Rag GTPases that signal amino acid sufficiency to mTORC1. Science 2013;340:1100-6. [Crossref] [PubMed]
- Zhao X, Jiang L, Hu D, et al. NPRL2 reduces the niraparib sensitivity of castration-resistant prostate cancer via interacting with UBE2M and enhancing neddylation. Exp Cell Res 2021;403:112614. [Crossref] [PubMed]
- Ji L, Nishizaki M, Gao B, et al. Expression of several genes in the human chromosome 3p21.3 homozygous deletion region by an adenovirus vector results in tumor suppressor activities in vitro and in vivo. Cancer Res 2002;62:2715-20. [PubMed]
- Tang Y, Jiang L, Tang W. Decreased expression of NPRL2 in renal cancer cells is associated with unfavourable pathological, proliferation and apoptotic features. Pathol Oncol Res 2014;20:829-37. [Crossref] [PubMed]
- Liu MN, Liu AY, Pei FH, et al. Functional mechanism of the enhancement of 5-fluorouracil sensitivity by TUSC4 in colon cancer cells. Oncol Lett 2015;10:3682-8. [Crossref] [PubMed]
- Peng Y, Dai H, Wang E, et al. TUSC4 functions as a tumor suppressor by regulating BRCA1 stability. Cancer Res 2015;75:378-86. [Crossref] [PubMed]
- Jayachandran G, Ueda K, Wang B, et al. NPRL2 sensitizes human non-small cell lung cancer (NSCLC) cells to cisplatin treatment by regulating key components in the DNA repair pathway. PLoS One 2010;5:e11994. [Crossref] [PubMed]
- Otani S, Takeda S, Yamada S, et al. The tumor suppressor NPRL2 in hepatocellular carcinoma plays an important role in progression and can be served as an independent prognostic factor. J Surg Oncol 2009;100:358-63. [Crossref] [PubMed]
- Liu AY, Liu DG, Du YJ, et al. Relationship between tumor and peripheral blood NPRL2 mRNA levels in patients with colorectal adenoma and colorectal cancer. Cancer Biol Ther 2014;15:489-95. [Crossref] [PubMed]
- Karlsson M, Zhang C, Méar L, et al. A single-cell type transcriptomics map of human tissues. Sci Adv 2021;7:eabh2169. [Crossref] [PubMed]
- Oncomine™. Available online: https://www.oncomine.org/resource/main.html.
- Yoon SJ, Park J, Shin Y, et al. Deconvolution of diffuse gastric cancer and the suppression of CD34 on the BALB/c nude mice model. BMC Cancer 2020;20:314. [Crossref] [PubMed]
- Kassambara A, Kosinski M, Biecek P. survminer: Drawing Survival Curves using ‘ggplot2’. 2020. Available online: https://rpkgs.datanovia.com/survminer/
- Lánczky A, Győrffy B. Web-Based Survival Analysis Tool Tailored for Medical Research (KMplot): Development and Implementation. J Med Internet Res 2021;23:e27633. [Crossref] [PubMed]
- Ru B, Wong CN, Tong Y, et al. TISIDB: an integrated repository portal for tumor-immune system interactions. Bioinformatics 2019;35:4200-2. [Crossref] [PubMed]
- Tang Z, Kang B, Li C, et al. GEPIA2: an enhanced web server for large-scale expression profiling and interactive analysis. Nucleic Acids Res 2019;47:W556-60. [Crossref] [PubMed]
- Zouridis H, Deng N, Ivanova T, et al. Methylation subtypes and large-scale epigenetic alterations in gastric cancer. Sci Transl Med 2012;4:156ra140. [Crossref] [PubMed]
- Aryee MJ, Jaffe AE, Corrada-Bravo H, et al. Minfi: a flexible and comprehensive Bioconductor package for the analysis of Infinium DNA methylation microarrays. Bioinformatics 2014;30:1363-9. [Crossref] [PubMed]
- Chandrashekar DS, Karthikeyan SK, Korla PK, et al. UALCAN: An update to the integrated cancer data analysis platform. Neoplasia 2022;25:18-27. [Crossref] [PubMed]
- Xiong Y, Wei Y, Gu Y, et al. DiseaseMeth version 2.0: a major expansion and update of the human disease methylation database. Nucleic Acids Res 2017;45:D888-95. [Crossref] [PubMed]
- Koch A, Jeschke J, Van Criekinge W, et al. MEXPRESS update 2019. Nucleic Acids Res 2019;47:W561-5. [Crossref] [PubMed]
- Szklarczyk D, Gable AL, Nastou KC, et al. The STRING database in 2021: customizable protein-protein networks, and functional characterization of user-uploaded gene/measurement sets. Nucleic Acids Res 2021;49:D605-12. [Crossref] [PubMed]
- Yu G, Wang LG, Han Y, et al. clusterProfiler: an R package for comparing biological themes among gene clusters. OMICS 2012;16:284-7. [Crossref] [PubMed]
- Yoshihara K, Shahmoradgoli M, Martínez E, et al. Inferring tumour purity and stromal and immune cell admixture from expression data. Nat Commun 2013;4:2612. [Crossref] [PubMed]
- Miao YR, Zhang Q, Lei Q, et al. ImmuCellAI: A Unique Method for Comprehensive T-Cell Subsets Abundance Prediction and its Application in Cancer Immunotherapy. Adv Sci (Weinh) 2020;7:1902880. [Crossref] [PubMed]
- Fu J, Li K, Zhang W, et al. Large-scale public data reuse to model immunotherapy response and resistance. Genome Med 2020;12:21. [Crossref] [PubMed]
- Austin PC, Schuster T. The performance of different propensity score methods for estimating absolute effects of treatments on survival outcomes: A simulation study. Stat Methods Med Res 2016;25:2214-37. [Crossref] [PubMed]
- Pezzi A, Cavo M, Biggeri A, et al. Inverse probability weighting to estimate causal effect of a singular phase in a multiphase randomized clinical trial for multiple myeloma. BMC Med Res Methodol 2016;16:150. [Crossref] [PubMed]
- Usui G, Matsusaka K, Mano Y, et al. DNA Methylation and Genetic Aberrations in Gastric Cancer. Digestion 2021;102:25-32. [Crossref] [PubMed]
- Cho ES, Kang HE, Kim NH, et al. Therapeutic implications of cancer epithelial-mesenchymal transition (EMT). Arch Pharm Res 2019;42:14-24. [Crossref] [PubMed]
- Paolillo M, Schinelli S. Extracellular Matrix Alterations in Metastatic Processes. Int J Mol Sci 2019;20:4947. [Crossref] [PubMed]
- Hashimoto I, Oshima T. Claudins and Gastric Cancer: An Overview. Cancers (Basel) 2022;14:290. [Crossref] [PubMed]
- Xiao S, Zhou L. Gastric Stem Cells: Physiological and Pathological Perspectives. Front Cell Dev Biol 2020;8:571536. [Crossref] [PubMed]
- Karimi Roshan M, Soltani A, Soleimani A, et al. Role of AKT and mTOR signaling pathways in the induction of epithelial-mesenchymal transition (EMT) process. Biochimie 2019;165:229-34. [Crossref] [PubMed]
- Yang L, Shi P, Zhao G, et al. Targeting cancer stem cell pathways for cancer therapy. Signal Transduct Target Ther 2020;5:8. [Crossref] [PubMed]
- Ruan T, Liu W, Tao K, et al. A Review of Research Progress in Multidrug-Resistance Mechanisms in Gastric Cancer. Onco Targets Ther 2020;13:1797-807. [Crossref] [PubMed]
- Chen X, Chen Z, Zheng B, et al. Targeting NPRL2 to enhance the efficacy of Olaparib in castration-resistant prostate cancer. Biochem Biophys Res Commun 2019;508:620-5. [Crossref] [PubMed]
- Yang W, Soares J, Greninger P, et al. Genomics of Drug Sensitivity in Cancer (GDSC): a resource for therapeutic biomarker discovery in cancer cells. Nucleic Acids Res 2013;41:D955-61. [Crossref] [PubMed]
- Hinshaw DC, Shevde LA. The Tumor Microenvironment Innately Modulates Cancer Progression. Cancer Res 2019;79:4557-66. [Crossref] [PubMed]
- Maiorino L, Daßler-Plenker J, Sun L, et al. Innate Immunity and Cancer Pathophysiology. Annu Rev Pathol 2022;17:425-57. [Crossref] [PubMed]
- Qin S, Xu L, Yi M, et al. Novel immune checkpoint targets: moving beyond PD-1 and CTLA-4. Mol Cancer 2019;18:155. [Crossref] [PubMed]
- Kruger S, Ilmer M, Kobold S, et al. Advances in cancer immunotherapy 2019 - latest trends. J Exp Clin Cancer Res 2019;38:268. [Crossref] [PubMed]
- Chen L, Flies DB. Molecular mechanisms of T cell co-stimulation and co-inhibition. Nat Rev Immunol 2013;13:227-42. [Crossref] [PubMed]
- Huang N, Cheng S, Mi X, et al. Downregulation of nitrogen permease regulator like-2 activates PDK1-AKT1 and contributes to the malignant growth of glioma cells. Mol Carcinog 2016;55:1613-26. [Crossref] [PubMed]
- Chen Z, Luo S, Chen Y, et al. High expression of NPRL2 is linked to poor prognosis in patients with prostate cancer. Hum Pathol 2018;76:141-8. [Crossref] [PubMed]
- Walcher L, Kistenmacher AK, Suo H, et al. Cancer Stem Cells-Origins and Biomarkers: Perspectives for Targeted Personalized Therapies. Front Immunol 2020;11:1280. [Crossref] [PubMed]
- Kurata A, Katayama R, Watanabe T, et al. TUSC4/NPRL2, a novel PDK1-interacting protein, inhibits PDK1 tyrosine phosphorylation and its downstream signaling. Cancer Sci 2008;99:1827-34. [Crossref] [PubMed]
- Liu A, Qiao J, He L, et al. Nitrogen Permease Regulator-Like-2 Exhibited Anti-Tumor Effects And Enhanced The Sensitivity Of Colorectal Cancer Cells To Oxaliplatin And 5-Fluorouracil. Onco Targets Ther 2019;12:8637-44. [Crossref] [PubMed]
- Chen Z, Jiang Q, Zhu P, et al. NPRL2 enhances autophagy and the resistance to Everolimus in castration-resistant prostate cancer. Prostate 2019;79:44-53. [Crossref] [PubMed]
- Milošević Z, Banković J, Dinić J, et al. Potential of the dual mTOR kinase inhibitor AZD2014 to overcome paclitaxel resistance in anaplastic thyroid carcinoma. Cell Oncol (Dordr) 2018;41:409-26. [Crossref] [PubMed]
- Kang YK, Boku N, Satoh T, et al. Nivolumab in patients with advanced gastric or gastro-oesophageal junction cancer refractory to, or intolerant of, at least two previous chemotherapy regimens (ONO-4538-12, ATTRACTION-2): a randomised, double-blind, placebo-controlled, phase 3 trial. Lancet 2017;390:2461-71. [Crossref] [PubMed]
- Ren X, Zhang L, Zhang Y, et al. Insights Gained from Single-Cell Analysis of Immune Cells in the Tumor Microenvironment. Annu Rev Immunol 2021;39:583-609. [Crossref] [PubMed]
- Li L, Ouyang Y, Wang W, et al. The landscape and prognostic value of tumor-infiltrating immune cells in gastric cancer. PeerJ 2019;7:e7993. [Crossref] [PubMed]
- Dermime S, Merhi M, Merghoub T. Editorial: Dynamic Biomarkers of Response to Anti-Immune Checkpoint Inhibitors in Cancer. Front Immunol 2021;12:781872. [Crossref] [PubMed]
- Jardim DL, Goodman A, de Melo Gagliato D, et al. The Challenges of Tumor Mutational Burden as an Immunotherapy Biomarker. Cancer Cell 2021;39:154-73. [Crossref] [PubMed]
- Nakamura Y, Kawazoe A, Lordick F, et al. Biomarker-targeted therapies for advanced-stage gastric and gastro-oesophageal junction cancers: an emerging paradigm. Nat Rev Clin Oncol 2021;18:473-87. [Crossref] [PubMed]