A novel senescence-associated LncRNA signature predicts the prognosis and tumor microenvironment of patients with colorectal cancer: a bioinformatics analysis
Introduction
While the five-year survival rate for early colorectal cancer (CRC) ranges from 65 to 92 percent depending on stage, it drops to 13 percent after cancer metastasis (1). Frontline treatment for CRC, such as chemotherapy and targeted therapy, is often associated with dose-limiting toxicities in patients and resistance in cancer cells (2). Immunotherapy for CRC has had limited success, as it is only effective in cases with microsatellite instability (3,4). As a result, new effective diagnostic markers, treatment targets, and prognostic factors must be identified to improve patient outcomes and quality of life.
The known traditional biomarker of CRC, such as carcinoembryonic antigen (CEA), is the only tumor-specific marker that is widely recommended for the clinical treatment of CRC (5). Even though CEA can sometimes detect CRC patients, its false positive rate is too high to be acceptable, and the same context, it is difficult to offer prognostic information, much less therapeutic advice (6). Long non-coding RNAs (lncRNAs) are currently thought to have a significant role in the malignant nature of CRC due to aberrant expression. For example, the small protein SRSP (splicing regulatory small protein) encoded by lncRNA LOC90024 promotes “oncogenic” RNA splicing and CRC tumorigenesis (7). High expression of LncRNA HOTAIR in CRC patients was associated with venous invasion, advanced tumor invasion and distant metastasis, and patients also had significantly shorter overall survival (OS) and disease-free survival (DFS) (8). Although several lncRNA prognostic models for CRC have been published (9-11), the majority do not predict the immune microenvironment or immunotherapeutic effects of CRC. Cellular senescence is an irreversible type of proliferative arrest that evolved as a protective mechanism for tissue homeostasis, ostensibly as a supplement to programmed cell death. It serves to inactivate and eventually destroy sick, defective, or otherwise superfluous cells (12). There are numerous examples of senescent preventive effects in cancer progression that have been well-documented (13). However, a growing body of research suggests that senescent cells may, in some cases, promote tumor formation and progression (14). Furthermore, the ability of senescent cells to promote cancer is not limited to cancer cells; other components of the tumor microenvironment (TME), such as fibroblasts and immune cells, appear to be particularly susceptible to the effects of senescence (15). Cancer-associated fibroblasts and other abundant stromal cells in tumors have been shown to undergo senescence, passing on cancer hallmark capabilities to cancer cells in the TME (16,17). In addition, senescent fibroblasts in normal tissues have been suspected of reshaping tissue microenvironments via their senescence-associated secretory phenotype (SASP) in order to provide paracrine support for local invasion and distant metastasis (14). In short, cellular senescence is a critical factor in the development of various cancers, including CRC, and has recently been systemically summarized as a novel cancer hallmark (12). Nevertheless, the potential role and mechanism of genes involved in senescence regulation in CRC TME remain unknown.
LncRNAs with more than 200 nucleotides are involved in various biological processes such as cell proliferation, differentiation, development, apoptosis, and metastasis (18). Evidence suggests that lncRNAs play an important role in the development and progression of a wide range of human cancers, including CRC (19). A recent transcription sequence study revealed that the majority of RNAs altered during senescence were protein-encoding transcriptions, but lncRNAs also appear to have undergone significant transcriptional changes in cancer and senescence progression (20). For example, the lncRNA PURPL was one of the most abundant and consistent transcriptions in senescent cells (20). The transcription factor p53, which is usually elevated in senescent cells, has been shown to transcriptionally control PURPL production (21). On the other hand, PURPL helps to maintain low basal p53 concentrations in colon cancer cells by interacting with MYBBP1A and preventing the formation of the stable p53–MYBBP1A complex (21). Because PURPL promotes tumorigenicity in colon cancer cells by acting as a pro-survival factor, PURPL may also contribute to the pro-survival phenotype of senescent cells (21). Given this, identifying lncRNA transcriptional changes can help define a senescent cancer signature.
Given the potentially harmful role of cell senescence in promoting various aspects of tumorigenesis, measuring senescent bursting as a toxicity biomarker warrants further investigation. Herein, we created a senescence-associated lncRNA signal and systematically examined the relationship between senescence-associated lncRNAs and CRC patients’ prognosis and clinicopathological characteristics. To predict survival in these patients, a nomogram was created that included a senescence-associated LncRNA signature (SenALSig) and clinical factors. Immune status and responses to chemotherapy and targeted therapy were compared in high- and low-risk groups. Our findings shed light on the regulatory mechanism underlying senescence-induced CRC and have the potential to improve the efficacy of individualized treatment and prognostic assessment. We present the following article in accordance with the TRIPOD reporting checklist (available at https://jgo.amegroups.com/article/view/10.21037/jgo-22-721/rc).
Methods
Data acquisition and differentially expressed senescence-related gene (DEG) identification
Figure 1 depicts the flow chart of the present study. The original transcriptome sequencing dataset and clinical characteristics of CRC [colon adenocarcinoma (COAD) and rectum adenocarcinoma (READ)] were obtained from The Cancer Genome Atlas (TCGA) database (https://portal.gdc.cancer.gov/). The study included a total of 631 patients with CRC. Following the exclusion of patients who lacked complete follow-up information, had a survival time of less than 30 days, or lacked complete clinicopathological data, a total of 573 patients were recruited for subsequent analyses. The prognosis outcomes data of OS and DFS from TCGA were extracted for the following analysis.
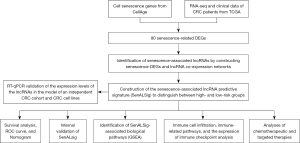
CellAge (http://genomics.senescence.info/cells), a manually curated database of 279 human genes, was used to obtain the genes associated with cell senescence (22). To identify cell senescence-related DEGs between tumor and normal tissues, we used a false discovery rate (FDR) of <0.05 and absolute log2 fold change values greater than 1.
Identification of a predictive signature of senescence-associated lncRNAs for CRC
Senescence-associated lncRNAs were identified by building a senescence-associated mRNA-lncRNA co-expression network with absolute Pearson correlation coefficient values of >0.3 and P<0.001 as the thresholds. Cytoscape 3.7.2 was used to visualize the lncRNA-mRNA co-expression networks. The R package “ggalluvial” was used to obtain the co-expression Sankey map. By using univariate Cox regression analyses with a P<0.01 cutoff, significant prognostic senescence-associated lncRNAs were identified and incorporated into a multivariate Cox regression analysis to establish the risk scores. A risk formula was used to calculate the risk score for each patient, where risk score = explncRNA1 × coef lncRNA1 + explncRNA2 × coef lncRNA2 +...+ explncRNAi × coef lncRNAi. The relationship between survival and the different risk groups was examined using linear regression analysis. The distribution of survival statuses based on the risk score levels was investigated. Clinicopathological data were included in the univariate and multivariate Cox regression analyses to determine whether the risk score was an independent prognostic indicator. The accuracy of the risk model was evaluated using receiver operating characteristic (ROC) curves. The R package “pheatmap” was used to construct and visualize the clinicopathological variables in the high- and low-risk groups. Principal component analysis (PCA) was also used to assess and illustrate the distribution of high-risk and low-risk patients, using the R package “scatterplot3d”.
Construction of the nomogram
Risk scores and other demographic parameters, such as gender, age, N stage, and total stage, were combined to develop a nomogram that could predict survival in CRC patients. We used a calibration curve to see if the predicted survival rate matched the actual survival rate. The R package “rms” was used to develop the nomogram.
Functional enrichment analysis of the senescence-associated lncRNAs predictive signature
According to the prognostic model, gene set enrichment analysis (GSEA) was carried out for the high- and low-risk groups. Significant biological processes and pathways were enriched when the nominal P value (NOM p) was <0.05 and FDR q<0.25. The c5.go.v7.4, symbols.gmt, and c2.cp.kegg.v7.4.symbols.gmt were used as reference files from the Molecular Signatures Database (MSigDB, http://software.broadinstitute.org/gsea/msigdb/index.jsp). The results were visualized using the R package “ggplot2.” A single-sample gene set enrichment analysis (ssGSEA) was used to calculate the infiltration scores of 16 immune cells and the activities of 13 immune-related pathways using the “GSVA” package in R (23).
Assessment of the predictive signature’s ability to predict drug treatment response
First, the expression differences of 40 immunological checkpoints between the high- and low-risk groups were analyzed. We also compared the half-maximal inhibitory concentration (IC50) of the drugs used to treat CRC between the high- and low-risk groups in order to assess the signature’s potential to predict treatment response. The Wilcoxon signed-rank test was used to compare the IC50 values.
Patients and tissue specimens of the independent validation CRC cohort
Patients were eligible for inclusion if they had histologically confirmed CRC with resectable tumors, regardless of microsatellite status or mismatched repair protein expression. The eighth edition of the American Joint Committee on Cancer (AJCC) was used to determine all staging (24). The presence of metastatic disease (stage IV), unresectable or recurrent CRC, CRC with active bleeding, perforation, or complete obstruction necessitating emergency surgery, and any prior anticancer therapy for CRC, such as chemotherapy, targeted therapy, or immunotherapy are important exclusion criteria. The baseline demographic and clinical characteristics of the quantitative reverse transcription polymerase chain reaction (RT-qPCR) independent validation CRC group are shown in Table 1.
Table 1
Clinical characteristics | RT-qPCR validation group (n=30) |
---|---|
Age (years) | |
Median | 60.6 |
Range | 45–72 |
Gender, n (%) | |
Female | 9 (30.00) |
Male | 21 (70.00) |
Tumor location, n (%) | |
Ascending colon | 3 (10.00) |
Transverse colon | 4 (13.33) |
Descending colon | 4 (13.33) |
Sigmoid colon | 11 (36.67) |
Rectum | 4 (13.33) |
Disease stage, n (%) | |
I | 6 (20.00) |
II | 6 (20.00) |
III | 18 (60.00) |
T stage, n (%) | |
T1 | 2 (6.67) |
T2 | 11 (36.67) |
T3 | 14 (46.67) |
T4 | 3 (10.00) |
N stage, n (%) | |
N0 | 12 (40.00) |
N1 | 5 (16.67) |
N2 | 13 (43.33) |
Histological differentiation, n (%) | |
Well | 12 (40.00) |
Moderately | 11 (36.67) |
Poorly | 7 (23.33) |
Loss of expression of mismatch repair proteins | 0 |
High microsatellite instability (testing by PCR) | 0 |
T, extension of the primary tumor; N, lymph node invasion; RT-qPCR, real-time quantitative reverse transcription polymerase chain reaction; CRC, colorectal cancer.
Tissue samples were obtained from CRC patients who underwent surgical resection at the Sixth Affiliated Hospital of Sun Yat-sen University in 2021. Written informed consent was obtained from all participants. The study was conducted in accordance with the Declaration of Helsinki (as revised in 2013) and approved by the Research Ethics Committee of the Sixth Affiliated Hospital of Sun Yat-sen University (No. 2021ZSLYEC-064). We excised tumor tissue and adjacent tumor-free tissue and preserved them in RNAfixer Reagent (Biotek, Beijing, China).
Cell culture
Three human GC cell lines (HCT116, SW480, and HCT8) and one normal human colon mucosal epithelial cell line, NCM460, were obtained from The Cell Bank of Type Culture Collection of the Chinese Academy of Sciences Committee (Shanghai, China). All the cell lines were cultured in RPMI 1640 medium. All media were supplemented with 1% penicillin-streptomycin (Invitrogen, Carlsbad, CA, USA) and 10% fetal bovine serum (Invitrogen). The cells were cultured at 37 °C in a humidified atmosphere containing 5% CO2.
Real-time RT-qPCR
A TRIzol reagent (Invitrogen, Carlsbad, USA) and an RNA-Quick Purification Kit (ES-RN001; Yishan Biotechnology, Shanghai, China) were used to extract the total RNA from tissues or cell lines according to the manufacturer’s instructions. The RT-qPCR analysis was performed as previously described (25). GAPDH was used as the normalization control. The 2–ΔCt or 2–ΔΔCt method was utilized for quantification.
Statistical analysis
R software (Version 4. 1.2) was used for all statistical analyses. The expression levels of senescence DEGs in cancerous and healthy tissues were compared using the Wilcoxon test. Patients in different groups were compared for differences in OS using the log-rank test. The Kruskal-Wallis test was used to compare the differences in the area under the ROC (AUC) between groups. When the AUC value is higher than 0.7, the model is regarded as performing well. For clinical data analysis, the chi-squared test or Fisher’s exact test was used. Demographic information was incorporated into the univariate and multivariate Cox regression analysis to test the hypothesis that the SenALSig is an independent prognostic predictor. Using Spearman or Pearson correlation coefficients, we investigated the relationships among lncRNA expression, immune checkpoint gene expression, and immunological infiltration. Statistical significance was defined as a corrected P value 0.05.
Results
Identification of senescence-related DEGs in CRC
We first looked into the expression of 279 genes to check if there was any aberrant expression of these senescence-related transcripts (https://cdn.amegroups.cn/static/public/jgo-22-721-1.docx) in normal and tumor tissues. A subset of 80 senescence-related DEGs was obtained, including 53 upregulated genes and 27 downregulated genes (Figure 2A, Table S1).
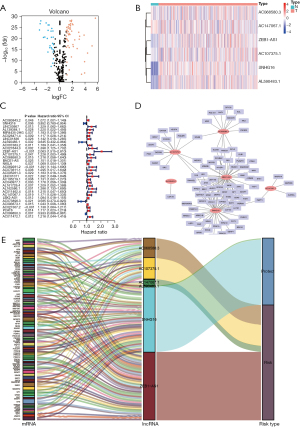
Construction of the senescence-associated lncRNA predictive signature—SenALSig
By using Pearson’s correlation analysis, a total of 1161 senescence-associated lncRNAs were identified under the absolute values of Pearson’s correlation coefficient >0.3 and P<0.001 as thresholds with correlation to senescence-related DEGs (https://cdn.amegroups.cn/static/public/jgo-22-721-2.xlsx). Thirty-two lncRNAs were identified as being related to the prognosis of CRC patients by a univariate Cox regression analysis (Figure 2B). Using multivariate Cox regression analysis, six senescence-associated lncRNAs (SNHG16, AL590483.1, ZEB1-AS1, AC107375.1, AC068580.3, and AC147067.1) were identified to form a predictive signature termed “SenALSig.” A heatmap of the six SenALSig lncRNAs’ expression levels in CRC patients is shown in Figure 2C. The co-expression network consisted of 120 pairs of senescence-associated lncRNA-mRNAs and was further visualized using Cytoscape software (Figure 2D; |R2| >0.3 and P<0.001). Figure 2E depicts a Sankey diagram demonstrating that SNHG16 and AL590483.1 were protective factors, whereas ZEB1-AS1, AC107375.1, AC068580.3, and AC147067.1 were risk factors.
Correlation between the SenALSig and the prognosis of CRC patients
The risk score of SenALSig was calculated as follows: risk score = (–0.8994×SNHG16 expression) + (–0.8211×AL590483.1 expression) + (0.9144×ZEB1-AS1 expression) + (0.6663×AC107375.1 expression) + (0.7681×AC068580.3 expression) + (0.5576×AC147067.1 expression). The formula was used to get the risk score for each patient, and the patients were then categorized into high- and low-risk groups on the basis on the median risk score. Finally, 278 patients were assigned to the high-risk group, while 295 patients were assigned to the low-risk group (Figure 3A). According to the Kaplan-Meier analysis, the OS time (Figure 3A) and progression-free survival time (PFS, Figure S1) of the high-risk group were significantly shorter than that of the low-risk group. Figure 3B,3C show the risk scores and survival statistics for individual patients. As risk scores rose, so did the number of patients who died. In the univariate and multivariate Cox regression analyses, the risk score was an independent predictor of OS in CRC patients (Figure 3D,3E). The risk score’s AUC was 0.714, which was better than most clinicopathological variables in predicting CRC patients’ prognosis (Figure 3F). The AUCs of the 1-, 3-, and 5-year ROC curves were 0.688, 0.718, and 0.733, respectively, indicating SenALSig’s robust prognostic predictive performance (Figure 3G).
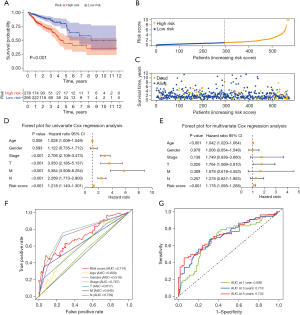
Figure 4A illustrates the risk group-specific expression of the six lncRNAs from the SenALSig model and the clinicopathological factors. Then, using our SenALSig model, patients with varying risks could be differentiated clearly, as shown in Figure 4B-4E, proving the model’s accuracy.
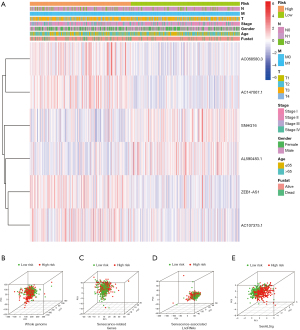
Furthermore, when assessing the relationship between the SenALSig risk score and demographic parameters, as shown in Figure 5A-5Q, we found significant relationships between the risk score and age (Figure 5A,5B), gender (female and male, Figure 5C,5D), distant metastasis (M0 and M1, Figure 5E,5F), lymph node metastasis (N1 and N2, Figure 5H,5I), T4 stage (Figure 5M), stage III and IV (Figure 5P,5Q). Using a stratification analysis, we again demonstrated that patients with a high-risk score had a significantly poorer prognosis. Taken together, these results showed that the SenALSig-based risk score was a significant independent prognostic risk factor for patients with CRC.
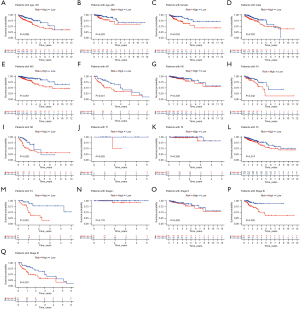
Construction and verification of a nomogram
To determine the likelihood that CRC patients would survive, we created a therapeutically adaptable nomogram employing the SenALSig risk score in conjunction with additional clinicopathological factors (Figure 6A). The nomogram’s calibration plots for 1-, 3-, and 5-year survival (Figure 6B-6D) demonstrated that the estimated mortality was reasonably similar to the actual mortality.
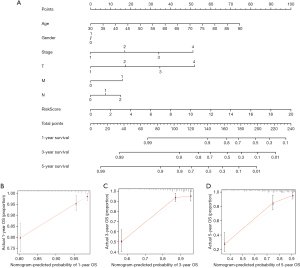
Internal validation of the SenALSig
To investigate the applicability of the SenALSig for OS based on the complete TCGA dataset, the dataset of 573 CRC patients was randomly split into two cohorts (n=288 in the first cohort and n=285 in the second cohort). Table 2 shows the demographic characteristics of the patients in the two cohorts. According to the findings derived from the complete TCGA cohort, the OS rate of patients in the high-risk group was lower than that of the low-risk group in both internal cohorts (Figure 7A,7B). Furthermore, the time-dependent ROC curves of the two cohorts performed well in terms of prediction. AUCs for 1-, 3-, and 5-year survival were 0.771, 0.788, and 0.758 in the first internal cohort (Figure 7C), and 0.625, 0.644, and 0.713 in the second internal cohort, respectively (Figure 7D). By internal verification from these two cohorts, we confirmed the robust predictive role of SenALSig in the prognosis of CRC.
Table 2
Clinical characteristics | Entire TCGA CRC samples (n=573) | First internal validation cohort (n=288) | Second internal validation cohort (n=285) | P-overall |
---|---|---|---|---|
Age, mean (SD) | 65.5 (12.4) | 64.6 (12.2) | 66.5 (12.5) | 0.168 |
Gender (%) | 0.814 | |||
Female | 259 (45.2) | 134 (46.5) | 125 (43.9) | |
Male | 314 (54.8) | 154 (53.5) | 160 (56.1) | |
Stage (%) | 0.153 | |||
Stage I + II | 303 (52.9) | 137 (47.6) | 166 (58.2) | |
Stage III + IV | 250 (43.6) | 139 (48.3) | 111 (38.9) | |
Unknown | 20 (3.5) | 12 (4.2) | 8 (2.8) | |
T (%) | 0.779 | |||
T1 + 2 | 118 (20.6) | 64 (22.2) | 54 (18.9) | |
T3 + 4 | 453 (79.1) | 222 (77.1) | 231 (81.1) | |
Tis | 1 (0.2) | 1 (0.3) | 0 | |
Unknown | 1 (0.2) | 1 (0.3) | 0 | |
M (%) | 0.302 | |||
M0 | 426 (74.3) | 208 (72.2) | 218 (76.5) | |
M1 | 82 (14.3) | 39 (13.5) | 43 (15.1) | |
MX_unknown | 65 (11.3) | 41 (14.2) | 24 (8.4) | |
N (%) | 0.052 | |||
N0 | 322 (56.2) | 146 (50.7) | 176 (61.8) | |
N1 + 2 | 248 (43.3) | 139 (48.3) | 109 (38.2) | |
NX_unknown | 3 (0.5) | 3 (1.0) | 0 |
T, tumor; M, metastasis; N, lymph node; P-overall, the P value is indicated for the chi-square test and Kruskal-Wallis test among the three groups, significant threshold <0.05; CRC, colorectal cancer.
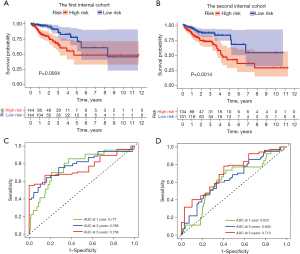
Identification of SenALSig-associated biological pathways
The gene set functional annotation in SenALSig-identified high- and low-risk CRC patient groups was performed using GSEA software. The Gene ontology (GO) terms positive regulation of GTPase activity [normalized enrichment score (NES) =2.634, NOM P<0.001, FDR q<0.001], regulation of GTPase activity (NES =2.617, NOM P<0.001, FDR q<0.001), positive regulation of MAPK cascade (NES =2.589, NOM P<0.001, FDR q<0.001), negative regulation of cytokine production (NES =2.579, NOM P<0.001, FDR q<0.001), and positive regulation of cell projection organization pathway (NES =2.567, NOM P<0.001, FDR q<0.001) were enriched in CRC patients with high-risk scores (Figure 8A and https://cdn.amegroups.cn/static/public/jgo-22-721-3.xlsx). In contrast, pathways related to mitochondrial function, such as mitochondrial gene expression (NES =−2.422, NOM P<0.001, FDR q<0.001), cytoplasmic translation (NES =−2.401, NOM P<0.001, FDR q<0.001), mitochondrial translation (NES =−2.345, NOM P<0.001, FDR q=0.002), ribosome assembly (NES =−2.343, NOM P<0.001, FDR q=0.002), and mitochondrial protein containing complex (NES =−2.342, NOM P<0.001, FDR q=0.002) were enriched in CRC samples with low-risk scores, (Figure 8A and https://cdn.amegroups.cn/static/public/jgo-22-721-4.xlsx). In addition, 178 enriched Kyoto Encyclopedia of Genes and Genomes (KEGG) pathways were identified. JAK/STAT signaling pathway (NES =2.454, NOM P<0.001, FDR q<0.001), cytokine-cytokine receptor interaction (NES =2.438, NOM P<0.001, FDR q<0.001), chemokine signaling pathway (NES =2.395, NOM P<0.001, FDR q<0.001), calcium signaling pathway (NES =2.370, NOM P<0.001, FDR q<0.001), and focal adhesion signaling pathways (NES =2.367, NOM P<0.001, FDR q<0.001) were enriched in the high-risk group (Figure 8B and https://cdn.amegroups.cn/static/public/jgo-22-721-5.xlsx). Citrate—tricarboxylic acid (TCA) cycle (NES =−2.181, NOM P<0.001, FDR q=0.014), Parkinson’s disease (NES =−2.126, NOM P=0.006, FDR q=0.015), oxidative phosphorylation (NES =−2.125, NOM P=0.004, FDR q=0.010), ribosome (NES =−2.089, NOM P<0.001, FDR q=0.010), and aminoacyl tRNA biosynthesis (NES =−2.067, NOM P=0.002, FDR q=0.010), were enriched in the low-risk group (Figure 8B and https://cdn.amegroups.cn/static/public/jgo-22-721-6.xlsx). These findings, particularly the enrichment pathway identified from the KEGG analysis of high-risk patients, suggested that SenALSig may be involved in the tumor immune microenvironment (TIME). Thus, we subsequently compared immune cell infiltration, immune pathway activation and immune checkpoint expression between the high- and low-risk groups.
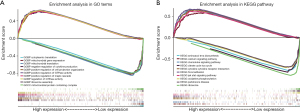
Immune cell infiltration, immune-related functions, and the expression of immune checkpoints in different risk groups
As shown in Figure 9A, we found that antitumor cell types, such as T cell CD4 memory resting, T cell CD4 memory activated, and dendritic cell resting, had strongly infiltrated the low-risk group. Treg cells with an immunosuppressive function had a significantly higher degree of infiltration in the high-risk group, but CD8+ T cells did not differ significantly between the two groups. In addition, the immune function scores of antigen-presenting cell (APC) co-stimulation, chemokine receptor (CCR), T cell co-stimulation, type I and II IFN response were higher in the high-risk group than in the low-risk group (Figure 9B). The expression of the majority of 47 immune checkpoint genes differed significantly between the high- and low-risk groups (Figure 9C). The high-risk group had much higher levels of clinically applied immunotherapeutic markers, such as CD274, PDCD-1 and CTLA4. These findings imply that the SenALSig-based risk score grouping could be used as a potential biomarker for immune checkpoint blockade therapy.
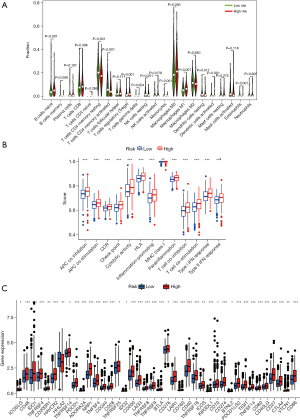
Correlation between the SenALSig and CRC chemotherapy and targeted therapy
Chemotherapy is an aggressive, non-specific treatment regimen that targets rapidly growing cells. Due to their non-proliferative nature, senescent fibroblasts, other stromal components, and SASP cancer cells are already highly resistant to chemotherapy (26). To address this issue, we investigated the relationships between the risk score and the efficacy of CRC-targeted therapeutics and chemotherapeutics (Figure 10A-10H). Interestingly, patients with high-risk scores were more sensitive to the targeted therapeutics vorinostat (P=3.6e-12, Figure 10A) and pyrimethamine (P=0.01, Figure 10B). Vorinostat treatment significantly reduced suppressor immune cell populations (27), which improved anti-tumor immunity in CRC patients. Furthermore, pyrimethamine inhibited cell growth in CRC cells by inducing S-phase arrest followed by cellular senescence, increased CD8+ T-cell–mediated cytotoxicity, and exerted antitumor activity in vivo (28). In addition, some routinely used clinical drugs, such as erlotinib (P=0.00016, Figure 10C) and gemcitabine (P=7.8e-06, Figure 10D), have also shown significant sensitivity effects in high-risk groups. Correspondingly, patients in the low-risk group showed more drug susceptibility: mTOR inhibitors, such as temsirolimus (P=0.022, Figure 10E), rapamycin (P=0.02, Figure 10F), and AZD8055 (P=0.00042, Figure 10G) appear to be particularly sensitive in this group of patients. The IC50 value of cisplatin (P=0.045, Figure 10H) was also significantly higher in patients with low-risk scores. Taken together, our drug sensitivity assays are useful for investigating individualized treatment plans for patients in both high- and low-risk groups.
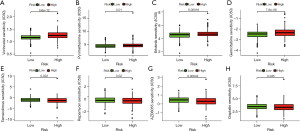
Independent validation of the six lncRNAs’ expression in the SenALSig model using CRC samples and cell lines
An independent validation cohort of 30 CRC tumor and non-tumor tissues was recruited to further validate the SenALSig model’s expression levels of SNHG16, AL590483.1, ZEB1-AS1, AC107375.1, AC068580.3, and AC147067.1. The RT-qPCR primer sequences are listed in Table S2. According to the RT-qPCR results, the expression levels of these lncRNAs differed significantly between tumor and normal tissues (Figure 11A-11F). AC147067.1 (Figure 11C) had a significantly lower expression in tumor tissues than the other five lncRNAs, which had significantly higher expressions. In addition, as shown in Figure 11G-11L), the expression of these six lncRNAs in at least one CRC cell line differed significantly from those of normal CRC epithelial cells. Furthermore, the online tool GEPIA2 (gepia2.cancer-pku.cn) was used to analyze the different expressions of the six lncRNAs in the CRC data set of TCGA (Figure S2A-S2F). Importantly, the expression pattern has been cross-validated with TCGA, our independent CRC cohorts, and CRC cell lines.
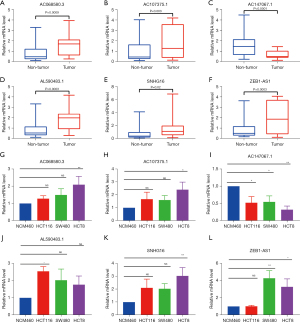
Discussion
More than 1.8 million new CRC cases and 881,000 CRC-related deaths occur yearly, ranking it third (10.2 %) and second (9.2%) among all cancer types in incidence and mortality rates, respectively (29,30). At present, the curative effect for CRC remains unsatisfactory, which is partly due to the lack of biomarkers for diagnosis, treatment, and prognosis. Combining multiple clinical genetic markers into a model could indeed improve predictive accuracy and contribute to the improvement of personalized treatment plans when compared to single clinical biomarkers (31). Cellular senescence, an essential component of aging and cancer, occurs due to various triggers, including telomere attrition, macromolecular damage, and signaling from activated oncogenes (20). Senescent cells (of any cellular origin) have recently been identified as functionally important cell types in the TME (12). The presence of senescent cells in the TME is associated with a lower DFS rate. Patients had a shorter OS when hepatocellular carcinoma tissues expressed more senescence-related genes (32). When senescent cells expressing the cell-cycle inhibitor p16INK4a+ in aging mice were pharmacologically ablated, there was a lower incidence of spontaneous tumorigenesis and cancer-associated deaths, as well as a delay in a number of age-related symptoms (33). Some lncRNAs are thought to regulate cellular senescence (34-36). For instance, the lncRNA PURPL has been shown to be a more robust senescent biomarker than p16 mRNA and p21 mRNA in the colon cancer cell line HCT116. It may promote tumorigenicity by acting as a survival factor and contributing to the survival phenotype of senescent cells (21).
Currently, senescent cells are identified by a combination of multiple traits, including expression and secretion of senescence-related proteins, DNA damage, and β-galactosidase activity; however, these traits are neither exclusively nor universally present in senescent cells (20,37-40). In addition, although several lncRNA signatures associated with CRC have been reported (9-11,41-44), only a few studies have focused on senescent-related lncRNA predictive signatures in CRC, prompting us to construct a SenALSig signature for simultaneous characterization of senescence and CRC. Based on the ROC curve, the SenALSig demonstrated a moderate predictive performance for CRC OS by using a six lncRNA senescence-associated signature (SNHG16, AL590483.1, ZEB1-AS1, AC107375.1, AC068580.3, and AC147067.1). Additionally, we believe that our newly established nomogram will help with the development of treatment strategies and enhance clinical decision-making. In the lncRNA model, both SNHG16 and ZEB1-AS1 have previously been identified as oncogenes in a number of cancers (45-48), including colon cancer. SNHG16 has been shown to promote colon cancer cell proliferation, migration, and epithelial-mesenchymal transition via miR-124-3p/MCP-1 (49), and ZEB1-AS1 promotes PAK2 expression by sponging miR-455-3p, facilitating colon cancer cell growth and metastasis (50). Furthermore, AL590483.1 and AC068580.3 have been linked to a colorectal prognosis signature component in several lncRNA risk models (51-55). However, studies on AC107375.1 and AC147067.1 in cancer are lacking. The impact of these lncRNAs in CRC and senescence must therefore be further studied.
The most valuable contribution of the present study is that it highlights the significant relationship between SenALSig and CRC prognosis. To date, the effect of the aging microenvironment on tumor treatment response is often overlooked in preclinical studies. For example, most animal models studied are 4-8 week old mice, rather than older mice with better mimicry (15). This may partly explain the fact that many preclinical treatments that were very effective have failed when they entered clinical trials. Furthermore, when using AUC as a cross-sectional comparison, our SenALSig model is comparable to most studies utilizing TCGA to establish lncRNA biomarker signatures (Table 3). This suggests that senescent cells have an impact on the prognosis of CRC and are at least as valuable as other biomarkers.
Table 3
Studies | No. of lncRNAs | AUC of time-dependent ROC | ||
---|---|---|---|---|
1-year | 3-year | 5-year | ||
Zhang et al. (9) | 4 | 0.622 | 0.635 | 0.675 |
Zhao et al. (10) | 3 | 0.679 | 0.663 | 0.699 |
Wang et al. (11) | 5 | NA | NA | 0.740 |
Cheng et al. (44) | 6 | NA | 0.797 | 0.771 |
Sun et al. (52) | 10 | NA | 0.725 | 0.803 |
Wang et al. (53) | 15 | NA | NA | 0.708 |
Chen et al. (54)* | 15 | 0.796 | 0.828 | 0.866 |
Zhou et al. (55)* | 10 | 0.796 | 0.790 | 0.723 |
The present study | 6 | 0.688 | 0.718 | 0.733 |
*, these studies only assessed colon cancer samples in the TCGA database. AUC, area under the ROC curve; ROC, receiver operating characteristic curve; NA, not available.
Additionally, we demonstrated a significant relationship between SenALSig and the TIME. SenALSig stratification revealed that immune cell infiltration, immune pathway activation, and immune checkpoint expression were all higher in the high-risk group than in the low-risk group. Notably, different states of the immune microenvironment, named immune-hot or -cold, have significant effects on therapeutic efficacy and the OS of CRC patients (56). Pre-existing anti-tumor immune responses within the TME are necessary for immune checkpoint blockade to be effective (57). Since our findings link cellular senescence markers to immune infiltration in CRC, targeting these senescence-associated lncRNAs in combination with immune checkpoint inhibitors may be a promising new direction. Interestingly, Immunotherapy markers such as CD274, PDCD-1, and CTLA4 were found to be significantly higher in the high-risk group, implying that immunotherapy may benefit these patients more. These results provide new insights into tumor immunotherapy. Taken together, the current study emphasizes the implication of cellular senescence on TIME and the future value of treating cellular senescence as a complement to enhance the efficacy of immunotherapy.
The transitional senescent state is another aspect of senescent cancer cells’ impact on cancer phenotype. In this case, senescent cancer cells can escape the proliferative state to avoid detection by the immune system and chemotherapeutic agents, but under the right conditions, they can resume cell proliferation and exhibit oncogenic cellular capacity (12,58). For example, in breast cancer models, treatment-induced senescent endothelial cells can increase the development of cancer cells (59,60). This situation suggests that clinicians should pay sufficient attention to strategies that target senescent cells during oncology treatment. Because our SenALSig signature fully considers senescence in patient risk stratification, it may have potential benefits for clinical drug selection.
The current study had several limitations. First, the study only used the TCGA dataset due to the current lack of external CRC databases. To decide if the SenALSig model will be able to accurately fit the dataset in the future, more data that is currently accessible should be taken into account. Second, some factors that can clearly influence prognosis were not included in the nomogram because they were not available. Third, functional and mechanistic research should be carried out to better understand the role of senescence-associated lncRNAs.
To summarize, we constructed a SenALSig lncRNA signature for CRC that can be used to predict prognosis. SenALSig was included in our effective nomogram. By using 30 pair-matched tumor and non-tumor tissues, we validated the expression levels of the identified lncRNAs independently. Importantly, SenALSig is associated with immune infiltration of the TME as well as immunotherapeutic efficacy.
Acknowledgments
Funding: The work was funded by the National Key Clinical Discipline, the Basic and Applied Basic Research Fund Project of Guangdong Province (No. 2021A1515410004, to Zhou TC), the National Natural Science Foundation of China (No. 81973858, to Zeng B and No. 82172790, to Li YR), the Basic and Applied Basic Research Fund Project of Guangdong Province (No. 2019A1515011200, to Zeng B), the Science and Technology Plan Project of Qingyuan City (No. 2019A028, to Zeng B).
Footnote
Reporting Checklist: The authors have completed the TRIPOD reporting checklist. Available at https://jgo.amegroups.com/article/view/10.21037/jgo-22-721/rc
Data Sharing Statement: Available at https://jgo.amegroups.com/article/view/10.21037/jgo-22-721/dss
Conflicts of Interest: All authors have completed the ICMJE uniform disclosure form (available at https://jgo.amegroups.com/article/view/10.21037/jgo-22-721/coif). TZ reports that the work was funded by the National Key Clinical Discipline, the Basic and Applied Basic Research Fund Project of Guangdong Province (No. 2021A1515410004). BZ reports that the work was funded by the National Natural Science Foundation of China (No. 81973858), the Basic and Applied Basic Research Fund Project of Guangdong Province (No. 2019A1515011200), and the Science and Technology Plan Project of Qingyuan City (No. 2019A028). YL reports that the work was funded by the National Natural Science Foundation of China (No. 82172790). The other authors have no conflicts of interest to declare.
Ethical Statement: The authors are accountable for all aspects of the work in ensuring that questions related to the accuracy or integrity of any part of the work are appropriately investigated and resolved. The study was conducted in accordance with the Declaration of Helsinki (as revised in 2013). The study was approved by the Research Ethics Committee of the Sixth Affiliated Hospital of Sun Yat-sen University (No. 2021ZSLYEC-064) and written informed consent was obtained from all participants.
Open Access Statement: This is an Open Access article distributed in accordance with the Creative Commons Attribution-NonCommercial-NoDerivs 4.0 International License (CC BY-NC-ND 4.0), which permits the non-commercial replication and distribution of the article with the strict proviso that no changes or edits are made and the original work is properly cited (including links to both the formal publication through the relevant DOI and the license). See: https://creativecommons.org/licenses/by-nc-nd/4.0/.
References
- Carlsen L, Huntington KE, El-Deiry WS. Immunotherapy for Colorectal Cancer: Mechanisms and Predictive Biomarkers. Cancers (Basel) 2022;14:1028. [Crossref] [PubMed]
- Xie YH, Chen YX, Fang JY. Comprehensive review of targeted therapy for colorectal cancer. Signal Transduct Target Ther 2020;5:22. [Crossref] [PubMed]
- André T, Shiu KK, Kim TW, et al. Pembrolizumab in Microsatellite-Instability-High Advanced Colorectal Cancer. N Engl J Med 2020;383:2207-18. [Crossref] [PubMed]
- Huyghe N, Baldin P, Van den Eynde M. Immunotherapy with immune checkpoint inhibitors in colorectal cancer: what is the future beyond deficient mismatch-repair tumours? Gastroenterol Rep (Oxf) 2020;8:11-24. [Crossref] [PubMed]
- Duffy MJ, van Dalen A, Haglund C, et al. Tumour markers in colorectal cancer: European Group on Tumour Markers (EGTM) guidelines for clinical use. Eur J Cancer 2007;43:1348-60. [Crossref] [PubMed]
- Primrose JN, Perera R, Gray A, et al. Effect of 3 to 5 years of scheduled CEA and CT follow-up to detect recurrence of colorectal cancer: the FACS randomized clinical trial. JAMA 2014;311:263-70. [Crossref] [PubMed]
- Meng N, Chen M, Chen D, et al. Small Protein Hidden in lncRNA LOC90024 Promotes “Cancerous” RNA Splicing and Tumorigenesis. Adv Sci (Weinh) 2020;7:1903233. [Crossref] [PubMed]
- Chen S, Zhang C, Feng M. Prognostic Value of LncRNA HOTAIR in Colorectal Cancer: A Meta-analysis. Open Med (Wars) 2020;15:76-83. [Crossref] [PubMed]
- Zhang W, Fang D, Li S, et al. Construction and Validation of a Novel Ferroptosis-Related lncRNA Signature to Predict Prognosis in Colorectal Cancer Patients. Front Genet 2021;12:709329. [Crossref] [PubMed]
- Zhao Z, Yang YB, Li XY, et al. Comprehensive Analysis of N6-Methyladenosine-Related lncRNA Signature for Predicting Prognosis and Immune Cell Infiltration in Patients with Colorectal Cancer. Dis Markers 2021;2021:8686307. [Crossref] [PubMed]
- Wang XC, Liu Y, Long FW, et al. Identification of a lncRNA prognostic signature-related to stem cell index and its significance in colorectal cancer. Future Oncol 2021;17:3087-100. [Crossref] [PubMed]
- Hanahan D. Hallmarks of Cancer: New Dimensions. Cancer Discov 2022;12:31-46. [Crossref] [PubMed]
- Kowald A, Passos JF, Kirkwood TBL. On the evolution of cellular senescence. Aging Cell 2020;19:e13270. [Crossref] [PubMed]
- Wang B, Kohli J, Demaria M. Senescent Cells in Cancer Therapy: Friends or Foes? Trends Cancer 2020;6:838-57. [Crossref] [PubMed]
- Fane M, Weeraratna AT. How the ageing microenvironment influences tumour progression. Nat Rev Cancer 2020;20:89-106. [Crossref] [PubMed]
- Faget DV, Ren Q, Stewart SA. Unmasking senescence: context-dependent effects of SASP in cancer. Nat Rev Cancer 2019;19:439-53. [Crossref] [PubMed]
- Birch J, Gil J. Senescence and the SASP: many therapeutic avenues. Genes Dev 2020;34:1565-76. [Crossref] [PubMed]
- Yao RW, Wang Y, Chen LL. Cellular functions of long noncoding RNAs. Nat Cell Biol 2019;21:542-51. [Crossref] [PubMed]
- Chen S, Shen X. Long noncoding RNAs: functions and mechanisms in colon cancer. Mol Cancer 2020;19:167. [Crossref] [PubMed]
- Casella G, Munk R, Kim KM, et al. Transcriptome signature of cellular senescence. Nucleic Acids Res 2019;47:7294-305. [Crossref] [PubMed]
- Li XL, Subramanian M, Jones MF, et al. Long Noncoding RNA PURPL Suppresses Basal p53 Levels and Promotes Tumorigenicity in Colorectal Cancer. Cell Rep 2017;20:2408-23. [Crossref] [PubMed]
- Avelar RA, Ortega JG, Tacutu R, et al. A multidimensional systems biology analysis of cellular senescence in aging and disease. Genome Biol 2020;21:91. [Crossref] [PubMed]
- Rooney MS, Shukla SA, Wu CJ, et al. Molecular and genetic properties of tumors associated with local immune cytolytic activity. Cell 2015;160:48-61. [Crossref] [PubMed]
- Weiser MR. AJCC 8th Edition: Colorectal Cancer. Ann Surg Oncol 2018;25:1454-5.
- Huang E, Fu J, Yu Q, et al. CircRNA hsa_circ_0004771 promotes esophageal squamous cell cancer progression via miR-339-5p/CDC25A axis. Epigenomics 2020;12:587-603. [Crossref] [PubMed]
- Fairfield H, Dudakovic A, Khatib CM, et al. Myeloma-Modified Adipocytes Exhibit Metabolic Dysfunction and a Senescence-Associated Secretory Phenotype. Cancer Res 2021;81:634-47. [Crossref] [PubMed]
- Patel S, Hurez V, Nawrocki ST, et al. Vorinostat and hydroxychloroquine improve immunity and inhibit autophagy in metastatic colorectal cancer. Oncotarget 2016;7:59087-97. [Crossref] [PubMed]
- Dong H, Hu L, Li W, et al. Pyrimethamine inhibits cell growth by inducing cell senescence and boosting CD8+ T-cell mediated cytotoxicity in colorectal cancer. Mol Biol Rep 2022;49:4281-92. [Crossref] [PubMed]
- Yang Y, Han Z, Li X, et al. Epidemiology and risk factors of colorectal cancer in China. Chin J Cancer Res 2020;32:729-41. [PubMed]
- Bray F, Ferlay J, Soerjomataram I, et al. Global cancer statistics 2018: GLOBOCAN estimates of incidence and mortality worldwide for 36 cancers in 185 countries. CA Cancer J Clin 2018;68:394-424. [Crossref] [PubMed]
- Guo Y, Qu Z, Li D, et al. Identification of a prognostic ferroptosis-related lncRNA signature in the tumor microenvironment of lung adenocarcinoma. Cell Death Discov 2021;7:190. [Crossref] [PubMed]
- Eggert T, Wolter K, Ji J, et al. Distinct Functions of Senescence-Associated Immune Responses in Liver Tumor Surveillance and Tumor Progression. Cancer Cell 2016;30:533-47. [Crossref] [PubMed]
- Baker DJ, Childs BG, Durik M, et al. Naturally occurring p16(Ink4a)-positive cells shorten healthy lifespan. Nature 2016;530:184-9. [Crossref] [PubMed]
- Nojima T, Tellier M, Foxwell J, et al. Deregulated Expression of Mammalian lncRNA through Loss of SPT6 Induces R-Loop Formation, Replication Stress, and Cellular Senescence. Mol Cell 2018;72:970-984.e7. [Crossref] [PubMed]
- Haemmig S, Yang D, Sun X, et al. Long noncoding RNA SNHG12 integrates a DNA-PK-mediated DNA damage response and vascular senescence. Sci Transl Med 2020;12:eaaw1868.
- Zhuang L, Xia W, Chen D, et al. Exosomal LncRNA-NEAT1 derived from MIF-treated mesenchymal stem cells protected against doxorubicin-induced cardiac senescence through sponging miR-221-3p. J Nanobiotechnology 2020;18:157. [Crossref] [PubMed]
- van Deursen JM. The role of senescent cells in ageing. Nature 2014;509:439-46. [Crossref] [PubMed]
- Kastenhuber ER, Lowe SW. Putting p53 in Context. Cell 2017;170:1062-78. [Crossref] [PubMed]
- Georgakilas AG, Martin OA, Bonner WM. p21: A Two-Faced Genome Guardian. Trends Mol Med 2017;23:310-9. [Crossref] [PubMed]
- Lee BY, Han JA, Im JS, et al. Senescence-associated beta-galactosidase is lysosomal beta-galactosidase. Aging Cell 2006;5:187-95. [Crossref] [PubMed]
- Yang Y, Feng M, Bai L, et al. Comprehensive analysis of EMT-related genes and lncRNAs in the prognosis, immunity, and drug treatment of colorectal cancer. J Transl Med 2021;19:391. [Crossref] [PubMed]
- Lu Y, Wang W, Liu Z, et al. Long non-coding RNA profile study identifies a metabolism-related signature for colorectal cancer. Mol Med 2021;27:83. [Crossref] [PubMed]
- Qin F, Xu H, Wei G, et al. A Prognostic Model Based on the Immune-Related lncRNAs in Colorectal Cancer. Front Genet 2021;12:658736. [Crossref] [PubMed]
- Cheng L, Han T, Zhang Z, et al. Identification and Validation of Six Autophagy-related Long Non-coding RNAs as Prognostic Signature in Colorectal Cancer. Int J Med Sci 2021;18:88-98. [Crossref] [PubMed]
- Zhang Y, Zhu B, He M, et al. N6-Methylandenosine-Related lncRNAs Predict Prognosis and Immunotherapy Response in Bladder Cancer. Front Oncol 2021;11:710767. [Crossref] [PubMed]
- Chen L, Qiu CH, Chen Y, et al. LncRNA SNHG16 drives proliferation, migration, and invasion of lung cancer cell through modulation of miR-520/VEGF axis. Eur Rev Med Pharmacol Sci 2020;24:9522-31. [PubMed]
- Du SM. The SNHG16/miR-30a axis promotes breast cancer cell proliferation and invasion by regulating RRM2. Neoplasma 2020;67:567-75. [Crossref] [PubMed]
- Chen C, Feng Y, Wang X. LncRNA ZEB1-AS1 expression in cancer prognosis: Review and meta-analysis. Clin Chim Acta 2018;484:265-71. [Crossref] [PubMed]
- Chen ZY, Wang XY, Yang YM, et al. LncRNA SNHG16 promotes colorectal cancer cell proliferation, migration, and epithelial-mesenchymal transition through miR-124-3p/MCP-1. Gene Ther 2022;29:193-205. [Crossref] [PubMed]
- Ni X, Ding Y, Yuan H, et al. Long non-coding RNA ZEB1-AS1 promotes colon adenocarcinoma malignant progression via miR-455-3p/PAK2 axis. Cell Prolif 2020;53:e12723. [Crossref] [PubMed]
- Wang WJ, Li HT, Yu JP, et al. A Competing Endogenous RNA Network Reveals Novel Potential lncRNA, miRNA, and mRNA Biomarkers in the Prognosis of Human Colon Adenocarcinoma. J Surg Res 2019;235:22-33. [Crossref] [PubMed]
- Sun Y, Peng P, He L, et al. Identification of lnc RNAs Related to Prognosis of Patients With Colorectal Cancer. Technol Cancer Res Treat 2020;19:1533033820962120. [Crossref] [PubMed]
- Wang X, Zhou J, Xu M, et al. A 15-lncRNA signature predicts survival and functions as a ceRNA in patients with colorectal cancer. Cancer Manag Res 2018;10:5799-806. [Crossref] [PubMed]
- Chen W, Chen Y, Liu L, et al. Comprehensive Analysis of Immune Infiltrates of Ferroptosis-Related Long Noncoding RNA and Prediction of Colon Cancer Patient Prognoses. J Immunol Res 2022;2022:9480628. [Crossref] [PubMed]
- Zhou W, Zhang S, Li HB, et al. Development of Prognostic Indicator Based on Autophagy-Related lncRNA Analysis in Colon Adenocarcinoma. Biomed Res Int 2020;2020:9807918. [Crossref] [PubMed]
- Tang T, Huang X, Zhang G, et al. Advantages of targeting the tumor immune microenvironment over blocking immune checkpoint in cancer immunotherapy. Signal Transduct Target Ther 2021;6:72. [Crossref] [PubMed]
- Mlecnik B, Bindea G, Angell HK, et al. Integrative Analyses of Colorectal Cancer Show Immunoscore Is a Stronger Predictor of Patient Survival Than Microsatellite Instability. Immunity 2016;44:698-711. [Crossref] [PubMed]
- De Blander H, Morel AP, Senaratne AP, et al. Cellular Plasticity: A Route to Senescence Exit and Tumorigenesis. Cancers (Basel) 2021;13:4561. [Crossref] [PubMed]
- Hwang HJ, Lee YR, Kang D, et al. Endothelial cells under therapy-induced senescence secrete CXCL11, which increases aggressiveness of breast cancer cells. Cancer Lett 2020;490:100-10. [Crossref] [PubMed]
- Wang D, Xiao F, Feng Z, et al. Sunitinib facilitates metastatic breast cancer spreading by inducing endothelial cell senescence. Breast Cancer Res 2020;22:103. [Crossref] [PubMed]