Elevated NDC1 expression predicts poor prognosis and correlates with immunity in hepatocellular carcinoma
Highlight box
Key findings
• We first predicted the prognostic and immunological value of NDC1 in HCC.
What is known and what is new?
• NDC1 played an important role in tumor progression, including reported cases of non-small cell lung cancer and cervical cancer.
• We investigated the relationship between NDC1 and HCC for the first time, which was completely new.
What is the implication, and what should change now?
• This provides new biomarkers and possible potential therapeutic targets for better prediction and treatment of HCC. On the basis of bioinformatics analysis and previous experiments, we need to further explore the biological role of NDC1 in vivo, which requires deeper demonstration in the future.
Introduction
Hepatocellular carcinoma (HCC) is one of the most common abdominal malignancies, accounting for 91.5% of primary liver cancers, and ranks sixth in terms of incidence and third in terms of mortality among all malignant tumors worldwide (1,2). Due to its insidious onset and the lack of typical symptoms in the early stage, most HCC patients have already developed to the middle or even advanced stage at the initial diagnosis. For these patients, there is no opportunity for surgical intervention and the treatment prospects are slim (3). Therefore, finding reliable biomarkers for the early diagnosis of HCC is crucial, so that a series of treatment measures such as surgery or chemotherapy can be employed in time to prolong the survival time of patients (4,5).
NDC1, also known as TMEM48, is the most conserved membrane nucleoporin localized to the nuclear pore complex (NPC), playing a crucial role in NPC and nuclear envelope (NE) assembly (6). The integrity of NE and correct nucleocytoplasmic transport are important for many cellular processes, including genome stability, DNA replication, DNA damage, DNA repair, and cell death (7). Notably, abnormal expression of nucleoporin has been reported to exert a critical impact on the progression of various cancers (8-11). Nonetheless, the expression pattern and underlining mechanisms of NDC1 in HCC have been rarely known.
Therefore, this study was conducted to explore the potential functions of NDC1 in HCC using proper bioinformatics and experiments. Firstly, we elucidated a remarkably elevated NDC1 expression in HCC and also identified a closer relationship with poor prognosis in the up-regulated NDC1 group. Moreover, NDC1 was confirmed as an independent prognostic factor for HCC via univariate and multivariate Cox regression analyses, and NDC1-related signaling pathways were simultaneously determined through gene set enrichment analysis (GSEA) analysis. Furthermore, we evaluated the immune correlations of NDC1 expression in HCC and validated the relationship between NDC1 and immunotherapy for the first time. Finally, we conducted in vitro experiments through overexpression and small interfering RNA (siRNA) interference, which consistently determined the crucial roles of NDC1 in cell proliferation, migration, and invasion of HCC cells. Collectively, these facts strongly suggested that NDC1 might serve as an essential role in predicting HCC prognosis and immunotherapy. We can say that NDC1 plays a carcinogenic role in HCC. We present the following article in accordance with the MDAR reporting checklist (available at https://jgo.amegroups.com/article/view/10.21037/jgo-22-1166/rc).
Methods
Data gathering and processing in The Cancer Genome Atlas (TCGA) database
We utilized the TCGA (https://tcga-data.nci.nih.gov/tcga/) database to obtain the RNA sequencing results and relevant clinical data of 374 cases of HCC and 50 cases of adjacent normal tissues. All data were standardized using R software (https://www.r-project.org/). In addition, differentially expressed genes (DEGs) were determined by using the “Limma” R package, with the cutoff criteria of adjusted P values (false discovery rate; FDR) <0.05 and |log2 fold change (FC)| ≥1 (12-14).
Patients and tissue samples
We collected 8 pairs of HCC tissues and adjacent non-cancerous tissues from 2021 inpatients in the Department of Hepatobiliary Surgery, The Second Affiliated Hospital of Nantong University. None of the patients had received radiotherapy, chemotherapy, or other adjuvant therapy before surgery. The study was conducted in accordance with the Declaration of Helsinki (as revised in 2013). The study was approved by Ethics Committee of The Second Affiliated Hospital of Nantong University (No. 2022KT244) and informed consent was taken from all the patients. Once isolated, the tissue samples were quickly frozen in liquid nitrogen and stored at −80 ℃ for later use.
Cell lines and cell culture
We purchased human HCC cell lines (SMMC-7721, Hep3B, SK-Hep1, HepG2) and a human normal hepatocyte cell line (QSG-7701) to perform the experiments. We used DMEM (Dulbecco’s modified Eagle’s medium) which was supplemented with 10% fetal bovine serum (FBS), 100 µg/mL streptomycin, and 100 units/mL penicillin to grow the cells and all cell lines were incubated in a 37 ℃ and 5% CO2 incubator.
Quantitative real-time polymerase chain reaction (qRT-PCR)
Trizol RNA isolation reagent (Ambion, Austin, TX, USA) was used to extract total RNA from the HCC tissues and cell lines. Then we took advantage of the first-strand cDNA synthesis kit (Vazyme, Nanjing, China) to reverse transcribe the RNA into cDNA. QRT-PCR was performed using the resulting cDNAs (1 µL) on the ABI QuantStudio5 Real-Time PCR System (Thermo Fisher Scientific, USA) with the measurement of Fast SYBR® Green Master Mix (Servicebio, China) and calculated by the 2-ΔΔCt method. The following primers were used (synthesized by Sangon, based in Shanghai, China): NDC1 (F: 5'-GCTGCTGTCAGTGGTAATAA -3'; R: 5'- GCAGGGAACCACAAGAAC -3') and GAPDH (glyceraldehyde-3-phosphate dehydrogenase) (F: 5'- CAGGAGGCATTGCTGATGAT -3'; R: 5'- GAAGGCTGGGGCTCATTT -3').
The Human Protein Atlas (HPA) and Clinical Proteomic Tumor Analysis Consortium (CPTAC) databases
NDC1 protein expression in HCC via immunohistochemical staining was determined by searching the online HPA (http://www.proteinatlas.org/) database. Furthermore, the statistical differences in NDC1 expression in HCC tissues and non-cancerous normal tissues were determined using the CPTAC database, and the relationship between NDC1 and gender in HCC was also explored.
Univariate and multivariate Cox regression analyses
Univariate and multivariate Cox regression analyses were applied to further investigate whether NDC1 and other clinical parameters (tumor grade, pathological stage, age, gender, race, and T, N, and M stages) were independent factors related to the overall survival (OS) of HCC patients.
GSEA analysis of NDC1
To better understand the possible biological functions of NDC1 in HCC, we employed GSEA to probe the crucial signaling pathways of NDC1 using normalized RNA-sequencing data obtained from TCGA, with the threshold of the normalized enrichment score (NSE) >1.5 and a nominal P value <0.05 (15).
Protein-protein interaction (PPI) network and Sangerbox website tools
A PPI network was constructed using the STRING (https://string-db.org/) database to identify the potential NDC1-related genes (16). Meanwhile, the Sangerbox website tools (http://www.sangerbox.com/tool), which is a free online platform, were applied to study the relationships between NDC1 and tumor neoantigen burden (TNB), tumor mutational burden (TMB), microsatellite instability (MSI), immune cell infiltration, tumor microenvironment, immune checkpoint molecules, and immune cell pathways via the Spearman or Pearson methods. This was subsequently visualized using the R package, and P<0.01 was set as the cutoff threshold (17-20).
Alteration analysis of NDC1
We used the cBioPortal database for cancer genomics (http://cbioportal.org) to verify the frequency and mutation site of NDC1 in HCC (21).
Prediction of NDC1 expression in response to immunotherapy
The response of NDC1 to immunotherapy was predicted by utilizing the The Cancer Imaging Archive (TCIA) databases. Data processing and graphing were performed using the “ggpubr”, “limma”, and “impute” R/Bioconductor packages.
Western blotting
Total protein, extracted from tissues or cells, was lysed in RIPA lysis buffer (Radio Immunoprecipitation Assay), which was composed of 50 mM NaCl, 0.1% Tween-20, 150 mM Tris-HCl, 1% NP-40 and a freshly added protease inhibitor cocktail (Sigma, USA). Before gel electrophoresis, the total protein concentration was determined with a Bio-Rad protein assay (BioRad, Hercules, CA, USA). An equal amount of protein was separated by 10% SDS-PAGE (Sodium Dodecyl Sulfate PolyAcrylamide Gel Electrophoresis) and transferred onto polyvinylidene fluoride (PVDF) membranes (Immobilon, Millipore). After blocking for 2 h in 5% dried skim milk in Tris Buffer Solution Tween (TBST) at room temperature, the membranes were then incubated overnight with primary antibodies against NDC1 (1:1,000, Thermo Fisher Scientific, USA), Proliferating Cell Nuclear Antigen (PCNA) (1:1,000, Proteintech, Wuhan, China), E-cadherin (1:1,000, Cell Signaling, USA), vimentin (1:1,000, Cell Signaling), and GAPDH (1:5,000, Proteintech, Wuhan, China). After incubating with an anti-rabbit/mouse immunoglobulin G (IgG) (Thermo Fisher Scientific) and a horseradish peroxidase-conjugated secondary antibody, the protein was visualized by enhanced chemiluminescence (ECL) (Thermo Fisher Scientific).
EDU (5-Ethynyl-2’-deoxyuridine) incorporation assay
The EDU cell proliferation detection kit was purchased from RiboBio Biotechnology Co., LTD (Guangzhou, China) and the experiment was carried out according to the manufacturer’s instructions. Briefly, the HepG2 cells were incubated with 50 µM EDU for 2 h. Before the cells were stained with EDU and the cell nuclei were counterstained with Hoechst 33342, they needed to be fixed in 4% paraformaldehyde and permeated in 0.5% Triton X-100. EDU-positive nuclei were detected by the fluorescence microscope (Leica, Germany). We calculated the cell proliferation rate according to the proportion of nucleated cells incorporating EDU in five high-power fields per well.
Cell-Counting-Kit-8 (CCK-8) assay
Cell viability was measured using the CCK-8 kit obtained from Vazyme. In brief, HepG2 cells were seeded on 96-well plates at a concentration of 2×104/well and grew overnight. Before detection, 10 µL aliquot of CCK-8 was added into each well under different treatments and the cells were incubated at 37 ℃ for 2 h. The absorbance was measured at 450 nm by using the automated microplate reader (Bio-Rad).
Cell cycle analysis
In order to analyze the cell cycle distribution, we performed the flow cytometrical analysis. The transfected cells were collected and washed with phosphate buffered saline (PBS) for three times before being fixed in 70% methanol overnight at 4 ℃. The fixed cells were centrifuged, washed again with PBS, and incubated with 1 mg/mL RNase (Ribonuclease) A at 37 ℃ away from light for 30 min. Next, the cells were stained with propidium iodide (PI) (50 µg/mL, Becton-Dickinson, USA) in PBS and 1% Triton-X 100. Samples were measured using a BD FACSCAN flow cytometer (Becton-Dickinson) and the acquired data was analyzed through the Cell Quest Acquisition and analysis programs (Becton-Dickinson).
Plasmid, siRNA, and transfection
The NDC1 overexpression plasmid was obtained from Genechem Biotechnologies Co., Ltd (Shanghai, China), and NDC1 siRNA oligos were synthesized by GenePharma (Shanghai, China) and inserted into a pLKO.1 plasmid. All of the transfection assays were performed using the Lipofectamine 2000 transfection reagent (Invitrogen, Carlsbad, CA, USA) according to the manufacturer’s protocol.
Wound healing assays
HepG2 cells after transfection for 48 hours were spread into 6-well plates with 5×105 cells per well. We utilized a sterile pipette tip to form straight-line scratch wounds until they were completely confluent. The cells were then washed with PBS to remove the non-adherent cells, and the serum-free medium was replaced for further culture. Every 24 h, the scratched cells were photographed using an inverted Leica phase-contrast microscope (Leica DFC 300 FX).
Migration and invasion assays
As for the migration and matrigel invasion assays, a 24-well transwell (8-µm pore size; Millipore) was used. We prepared the matrigel-coated chamber in advance according to the instructions and added 500 µL DMEM with 10% FBS into the bottom chamber. For the migration assay, the concentration of cells was adjusted to 1.0×105/mL 24 h after transfection by DMEM FBS-free medium, and a 100 µL cell suspension was loaded into the non-matrigel-coated top chamber. While in the invasion assay, the concentration of cells was adjusted to 2.0×105/mL, and a 100 µL cell suspension was instead added into the upper chamber coating matrigel. The cells were then fixed with paraformaldehyde, stained with crystal violet, and counted immediately after staining.
Statistical analysis
All statistical data analysis and figures were performed using SPSS 24.0 (IBM, Chicago, USA), GraphPad Prism 9.0 (San Diego, USA), and R 4.1.1 (https://www.r-project.org/). All data shown represent the results of at least three independent experiments, and P<0.05 was considered statistically significant.
Results
NDC1 mRNA (messenger RNA) expression levels in HCC from TCGA database
As shown in Figure 1A, TCGA database showed 17 different kinds of tumors with high NDC1 expression, including HCC (P<0.01). We then selected HCC for further research. Both the boxplot (N=50, T=374, Figure 1B) and also the pairwise boxplot (N=50, T=50, Figure 1C) demonstrated higher NDC1 mRNA levels in cancer tissues versus non-cancerous normal tissues in TCGA (both P<0.01). QRT-PCR experiments were further carried out to verify the mRNA expression of NDC1 in HCC and normal hepatocyte cells, and the outcome was consistent with those mentioned above (Figure 1D).
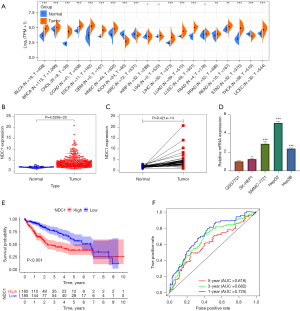
Next, the HCC patients were divided into high and low expression groups according to the median expression of NDC1 and the subsequent Kaplan-Meier (K-M) survival analysis displayed an expected result; that is, the high NDC1 expression group had a significantly worse prognosis than the low NDC1 expression group (P<0.001, Figure 1E). To comprehensively assess the diagnostic value of NDC1, we constructed receiver operating characteristic (ROC) curves, which revealed that the 1-, 3-, and 5-year area under the curve (AUC) values were 0.725, 0.682, and 0.616, respectively (Figure 1F). In summary, NDC1 was significantly related to HCC and could predict the prognosis of HCC patients to a certain extent. Thus, NDC1 might be a promising new gene for HCC exploration.
Protein expression of NDC1 in HCC
Next, we discussed the expression of NDC1 at the protein level from two aspects. On the one hand, immunohistochemical staining from the HPA database exhibited a low NDC1 expression state in HCC tissues, which could not be detected in the adjacent normal tissues (Figure 2A,2B). From the CPTAC database, we observed that NDC1 expression in HCC tissues was markedly elevated compared to that in the normal samples, and differences between males and females were also found (Figure 2C,2D, both P<0.001). Also, the western blot analyses of the NDC1 overexpression pattern in HCC tissues and cells showed similar results (Figure 2E, all P<0.01).
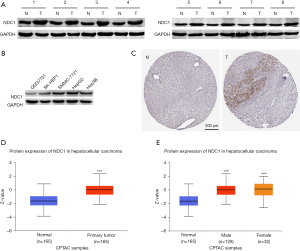
Associations between NDC1 expression and clinicopathological parameters
We observed the mRNA and protein expressions of NDC1 in HCC tissues and then sought to determine whether there were any correlations between NDC1 and clinicopathological parameters. As shown in Figure 3, we found that the NDC1 expression was significantly related to the tumor grade, pathological stage, and T stage (all P<0.01) but not age, gender, race, or M and N stage. In other words, these results reflected that HCC patients with high NDC1 expression might have a worse clinical stage and poorer prognosis, and might also encounter more complex and difficult clinical treatment options.
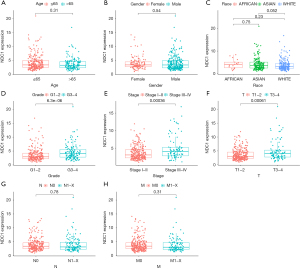
NDC1 could independently predict OS in HCC
Subsequently, univariate and multivariate Cox regression analyses were conducted to estimate which of the nine clinical parameters, including NDC1, were independent prognostic factors associated with OS in HCC (the other eight clinical parameters were as follows: age, gender, race, tumor grade, pathological stage, and T, N, and M stage). Univariate Cox regression analysis showed that NDC1, stage, and T stage were significantly correlated with OS in HCC, while the multivariate Cox regression analysis indicated that NDC1 and M stage could predict OS in HCC (all P<0.01, Figure 4 and Table 1). In summary, NDC1 was identified as an independent prognostic indicator in HCC.
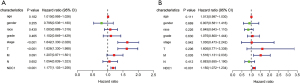
Table 1
Characteristics | Univariate analysis | Multivariate analysis | |||||||
---|---|---|---|---|---|---|---|---|---|
HR | 95% CI low limit | 95% CI high limit | P value | HR | 95% CI low limit | 95% CI high limit | P value | ||
Age | 1.010346 | 0.995177 | 1.025747 | 0.182343 | 1.013476 | 0.996941 | 1.030285 | 0.110734 | |
Gender | 0.795041 | 0.535683 | 1.17997 | 0.254908 | 0.907348 | 0.581349 | 1.416154 | 0.668599 | |
Race | 1.082731 | 0.88876 | 1.319036 | 0.430024 | 0.844642 | 0.642698 | 1.11004 | 0.225849 | |
Grade | 1.101676 | 0.849479 | 1.428746 | 0.465355 | 1.075294 | 0.800488 | 1.444441 | 0.629733 | |
Stage | 1.642365 | 1.329845 | 2.028328 | 4.09E-06 | 1.029542 | 0.472782 | 2.241955 | 0.94155 | |
T | 1.62586 | 1.329955 | 1.9876 | 2.12E-06 | 1.604844 | 0.771265 | 3.339351 | 0.20578 | |
M | 1.207148 | 0.970571 | 1.501391 | 0.090729 | 1.516158 | 1.130575 | 2.033244 | 0.005442 | |
N | 1.053726 | 0.839219 | 1.323062 | 0.652257 | 0.882648 | 0.654918 | 1.189564 | 0.412293 | |
NDC1 | 1.177126 | 1.102923 | 1.256322 | 9.16E-07 | 1.149912 | 1.071934 | 1.233563 | 9.67E-05 |
HR, hazard ratio; HCC, hepatocellular carcinoma; TCGA, The Cancer Genome Atlas.
NDC1-related signaling pathways in HCC
Since up-regulated NDC1 was significantly related to the occurrence and development of HCC, we sought to determine the signaling pathways through which NDC1 promoted HCC progression. So, we utilized GSEA analysis to determine the mechanism and signaling pathways of NDC1 regulation in HCC, which revealed seven enrichment pathways, including the cell cycle, the ERBB signaling pathway, the INSULIN signaling pathway, the MTOR signaling pathway, the NOTCH signaling pathway, the P53 signaling pathway, and the WNT signaling pathway (Figure 5).
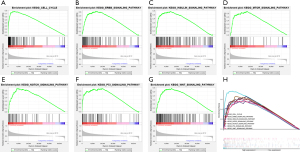
Associations between NDC1 and the PPI network, MSI, TNB, and TMB, and NDC1 alteration analysis in HCC
We utilized the STRING database to analyze the PPI in HCC and detected 10 genes that were potentially associated with NDC1: CCNB1, CCNB2, CDK1, NUP93, NUP21, NUP35, NUP205, NUP155, CDCA8, and SEH1L (Figure 6A). Next, we further analyzed the relationship between NDC1 and MSI, TNB, and TMB. The outcomes revealed that NDC1 had a certain correlation with MSI (P=0.043) but no links to TNB (P=0.49) and TMB (P=0.93) (Figure 6B-6D). Using the cBioPortal database (TCGA, Pan-cancer Atlas), we also studied the mutational characteristics of NDC1 in HCC. The NDC1 mutation frequency results in pan-cancer and the mutation site in HCC were illustrated in Figure 6E,6F.
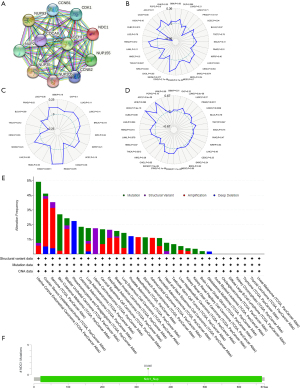
Relationships between NDC1 and immune cell infiltration, tumor microenvironment, immune checkpoint molecules, and immune cell pathways
Using the Spearman method, we concluded that NDC1 mRNA expression was significantly linked to six types of immune cell infiltration (B cells, neutrophils, CD4+ T cells, CD8+ T cells, dendritic cells, and macrophages; all P<0.01, Figure 7A). Nevertheless, the mRNA expression of NDC1 exhibited no obvious associations with the ESTIMATEScore (P=0.284), StromalScore (P=0.602), or ImmuneScore (P=0.127), as shown in Figure 7B. As for the immune checkpoint molecules and immune cell pathways, we confirmed that the NDC1 mRNA level was probably related to 35 kinds of immune checkpoint molecules using the Pearson method, including VTCN1, VSIR, TNFSF9, TNFSF4, TNFSF18, TNFSF15, TNFSF8, TIGIT, NRP1, LAIR1, KIR3DL1, ICOSLG, ICOS, PDCD1, PDCD1LG2 and so on (all P<0.05, Figure 7C). Similarly, based on the immune cell pathways, the NDC1 mRNA level was strongly correlated to Type 2 T-helper cells, neutrophils, natural killer T-cells, monocytes, immature dendritic cells, eosinophils, effector memory CD4 T-cells, central memory CD4 T-cells, CD56 bright natural killer cells, activated CD4 T-cells, activated CD8 T-cell, CD56 dim natural killer cells, memory B cell, Type 1 T-helper cells and Type 17 T-helper cells (all P<0.05, Figure 7D).
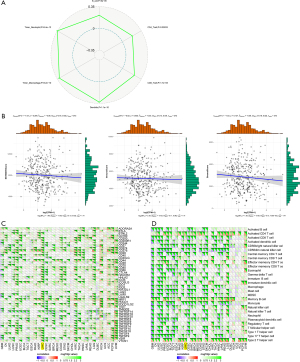
Prediction of NDC1 expression in response to immunotherapy
Ultimately, we attempted to probe the possible responses of high and low NDC1 expressions to anti-cytotoxic T lymphocyte antigen 4 (CTLA-4) or anti-programmed cell death protein 1 (anti-PD-1) using the TCIA database. Our outcomes indicated that the low-NDC1 expression group tended to be much more sensitive to CTLA-4 or PD-1 immunotherapy alone (both P<0.001), as opposed to a combination of both (P=0.0021, Figure 8A-8C).
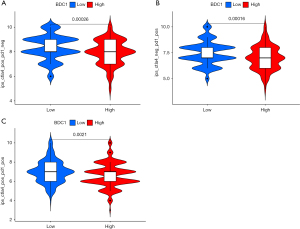
NDC1 promoted the proliferation of HCC cells
NDC1 has been reported to play an important role in cell proliferation in cervical cancer and non-small cell lung carcinoma (22-24). We speculated that NDC1 had a potential effect on HCC cell proliferation. To verify this hypothesis, we constructed NDC1 overexpression and small interference models using synchronous HCC cells. In this study, we selected the HepG2 cell line for further study since previous experiments had shown higher NDC1 mRNA and protein expression in HepG2. Following the transfection of HepG2 cells with the NDC1-overexpressing plasmid or siRNA, we observed a dramatic rise or decrease in the expressions of NDC1 and PCNA compared to the controls, which was associated with cell proliferation (Figure 9A,9B).
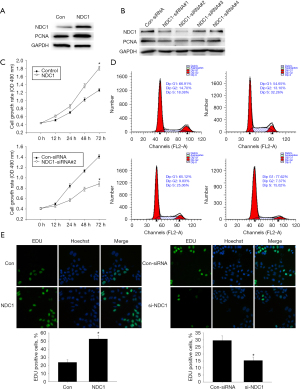
Meanwhile, we defined NDC1-siRNA-2 as the most interfering site, and used it in the subsequent assays. First of all, we performed CCK-8 assays to detect cell viability, so as to comprehensively confirm the effects of NDC1 on cell proliferation in HCC. We found that cell viability was obviously increased in HepG2 cells with NDC1 overexpression. Meanwhile, the opposite effects on cell viability were also observed with NDC1 knockdown (Figure 9C). In addition, the percentage of cells in the S phase increased markedly in the presence of NDC1 overexpression but quickly decreased after NDC1 expression defects (Figure 9D). The EDU incorporation assays also showed similar outcomes (Figure 9E). All of the data indicated that NDC1 facilitated the G1-S transition of HCC cells. Together, these results demonstrated that NDC1 participated in cell cycle regulation and eventually resulted in the impaired proliferation of HCC cells.
NDC1 regulated HCC cell migration and invasion
Based on the finding that NDC1 was associated with the migration and invasion ability of cervical cancer cells (24), we speculated that NDC1 might exert a crucial function in the regulation of cell migration and invasion in HCC progression. Wound healing and transwell assays (without matrigel) showed that NDC1 overexpression could promote cell migration ability in HepG2 cells compared to the control group, while depletion of NDC1 suppressed this ability. Moreover, in the transwell assay (with matrigel), an enhanced invasive ability was observed in NDC1-overexpressing HepG2 cells, which declined with NDC1 knockdown (Figure 10A-10D). Furthermore, we detected the expression of E-cadherin and Vimentin by western blot analysis. NDC1-overexpressing HepG2 cells had a notable decrease in the level of E-cadherin and an increase in the level of Vimentin. However, knockdown of NDC1 not only decreased the expression of Vimentin, but also obviously enhanced the expression of E-cadherin (Figure 10E). These results suggested that NDC1 might regulate the migration and invasion of HCC cells.
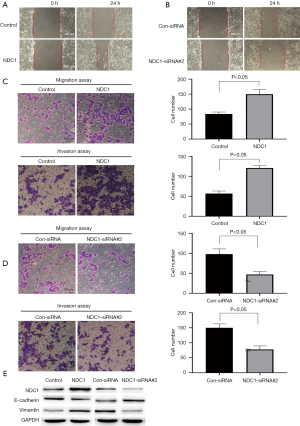
Discussion
HCC is a common malignant tumor with high rates of morbidity and mortality both in China and around the world (25). Although advances in cancer screening, diagnosis, and management have improved the prognosis of HCC, the current 5-year survival rate for HCC is still unsatisfactory due to its high rates of metastasis and recurrence after surgical resection (26,27). Fortunately, an increasing number of molecules have been shown to play vital roles in the occurrence and development of HCC, which is greatly beneficial for enhancing the survival of HCC patients (28-31). NDC1, the most conserved component of NPCs, is a transmembrane nucleoporin that plays vital roles in NPC assembly and nucleocytoplasmic transport. A previous study has reported on the requirement of SEPT12 (Septin12)-NDC1 complexes for mammalian spermiogenesis (32). Yamazumi et al. suggested that the NDC1-mediated localization of ALADIN (ALacrima Achalasia aDrenal Insufficiency Neurologic disorder) to NPCs was essential for selective nuclear protein import, and that abrogation of the interaction between ALADIN and NDC1 might induce triple-A syndrome (33), both of which indicated the indispensable function of NDC1 in biological processes. In recent years, studies on NDC1 in malignant tumors have gradually increased, mainly in non-small cell lung cancer and cervical cancer (22-24). However, little is currently known about the function of NDC1 in HCC. Therefore, we conducted this research to explore the potential prognostic and immunological value of NDC1 in HCC.
Firstly, significant upregulation of NDC1 expression was established in HCC tissues compared to non-tumor normal tissues in TCGA database and was found to be correlated with poor OS. Similar results were also obtained through qRT-PCR, immunohistochemistry, western blotting, and the CPTAC database. In addition, NDC1 expression was significantly linked to tumor grade, pathological stage, and T stage. More interestingly, NDC1 was identified as an independent prognostic factor for HCC via univariate and multivariate Cox regression analyses, which was of great significance for the clinical application of NDC1.
To comprehensively investigate the mechanisms through which NDC1 was involved in HCC progression, we searched the GSEA database and found that NDC1 was preferably enriched in the cell cycle, ERBB, INSULIN, MTOR, NOTCH, P53, and WNT-related pathways. Large early studies had confirmed that an aberrant cell cycle could induce the malignant proliferation of various tumor cells (34-37). Similarly, ERBB receptors are commonly overexpressed or overactivated in numerous cancers, especially in breast, ovarian, and non-small cell lung cancers, which are strongly correlated with poor prognosis, drug resistance, cancer metastasis, and a lower survival rate (38-40). Moreover, a growing body of evidence indicates that insulin and insulin-like growth factors can regulate cell proliferation, differentiation, apoptosis, glucose transport, and energy metabolism, and thus, play a critical role in tumorigenesis (41). Also, mTOR is frequently activated in cancer and could efficiently control cell growth and metabolism (42). Emerging data has validated that Notch signaling could contribute to increased aggressive properties such as invasion, tumor heterogeneity, angiogenesis, or tumor cell dormancy within solid cancer tissues, especially in epithelial cancers (43). P53, which is a key tumor suppressor, is frequently a prerequisite for cancer development owing to its function loss (44). As one of the most important signaling pathways, Wnt signaling is most active during embryogenesis, facilitating new organism formation by cell differentiation, polarization, and migration, and is commonly activated during the development of several tumors (45). Taken together, these findings highlight that NDC1-related signaling pathways are definitely involved in the key processes of oncogenesis, which lays a good foundation for further studies of NDC1 in HCC.
Considering the widespread participation of immune cells and related secretory factors in the regulation of malignant tumor growth and invasion, we further probed the relationship between NDC1 and immunity. Previous studies had reported that TMB, TNB, and MSI could be used as biomarkers related to pan-cancer immunotherapy efficacy and survival prognosis (46-48). Hence, we utilized the Pearson method and observed a subtle correlation between NDC1 expression and MSI, but not TNB or TMB. In recent decades, studies on immune cell infiltration, the tumor microenvironment, immune checkpoint molecules, and immune cell pathways have become increasingly important for tumorigenesis and development, and a large amount of data have verified remarkable links between the above elements and tumor prognosis and immunotherapy response (49-51). In the present study, we shed light on the dramatic crosstalk between NDC1 and immune cell infiltration using the Spearman method and fortunately determined apparent associations between NDC1 and B cells, neutrophils, CD4+ T cells, CD8+ T cells, dendritic cells, and macrophages. However, we did not observe any relationships between NDC1 and the ESTIMATEScore, StromalScore, or ImmuneScore. As for the immune checkpoint molecules and immune cell pathways, our results demonstrated that NDC1 was significantly associated with up to 35 immune checkpoint molecules and 15 immune cell pathways. At present, cancer cells are known to possess the ability to activate different immune checkpoint pathways that harbor immunosuppressive functions. Monoclonal antibodies that target immune checkpoint molecules have provided an important breakthrough in cancer therapeutics (52,53). Many studies have shown that there may be a correlation between immune cells and HCC prognosis, among which M1 macrophages (54), CD4+ T cells (55), CD8+ T cells (56) and B cells (57) are associated with a good prognosis of HCC, while M2 macrophages (58), regulatory T cells (Tregs) (59) and regulatory B cells (Bregs) (60) may be associated with poor prognosis of HCC. In addition, it is well known that PD-1 is a molecule that can block immune function. Its high expression can mediate tumor immune escape and allow tumor growth, proliferation and spread. PD-1 and its ligand inhibitors have become a promising therapeutic method (61). Interestingly, our prediction not only found that NDC1 was closely associated with these immune cell infiltrations, but also with PD-1(PCDC1) and its ligand PDCDLG2, so could NDC1 regulate immune cells and ultimately promote HCC progression by influencing the expression of PD-1 or its ligand? This is an important question for us to pay attention to and further study. Therefore, we have more reasons to believe that NDC1 is highly likely to become the next biomarker for tumor therapy.
Finally, we determined the potential roles of NDC1 in the cell proliferation, migration, and invasion of HCC. Through CCK-8, EDU, and flow cytometry analysis, we discovered that NDC1 overexpression could significantly enhance cell proliferation and depletion of NDC1-suppressed cell proliferation. EMT is involved in the invasion and metastasis of various tumor cells, and plays an important role in tumor progression, including HCC. We detected the expression of EMT-related proteins, such as E-cadherin and Vimentin, in HepG2 cells (62). The silencing of NDC1 enhanced E-cadherin protein expression but reduced that of Vimentin. NDC1-overexpressing HepG2 cells displayed the opposite results. Furthermore, through wound healing and transwell assays, we found that the overexpression of NDC1 remarkably enhanced the HCC cell migration and invasion abilities.
The key finding of our paper was that it elucidated the expression pattern and potential functions of NDC1 in HCC for the first time, and also provided new ideas for the prognosis and immunotherapy of HCC. However, there were still some limitations that should be noted. Firstly, the precise mechanism and other possible oncogenic roles of NDC1 in HCC progression still need to be investigated. In future research, we will aim to study the detailed mechanism of NDC1 in HCC through a series of in vitro and in vivo experiments, including in cells, tissues, and nude mice. We expect that in the near future, NDC1 will become a new target for the clinical treatment of HCC and present novel approaches for combating HCC.
Conclusions
In summary, our article demonstrated elevated NDC1 expression in HCC tissues versus corresponding normal tissues at both RNA and protein levels. Furthermore, NDC1 was speculated to play an irreplaceable role in HCC prognosis prediction and immunotherapy. As well, we constructed NDC1 overexpression and small interference models to verify its functions in HCC cell proliferation, migration, and invasion. Overall, NDC1 served as a key tumor promoter during HCC progression.
Acknowledgments
Funding: None.
Footnote
Reporting Checklist: The authors have completed the MDAR reporting checklist. Available at https://jgo.amegroups.com/article/view/10.21037/jgo-22-1166/rc
Data Sharing Statement: Available at https://jgo.amegroups.com/article/view/10.21037/jgo-22-1166/dss
Peer Review File: Available at https://jgo.amegroups.com/article/view/10.21037/jgo-22-1166/prf
Conflicts of Interest: All authors have completed the ICMJE uniform disclosure form (available at https://jgo.amegroups.com/article/view/10.21037/jgo-22-1166/coif). The authors have no conflicts of interest to declare.
Ethical Statement: The authors are accountable for all aspects of the work in ensuring that questions related to the accuracy or integrity of any part of the work are appropriately investigated and resolved. This study was approved by the Ethics Committee of the Second Affiliated Hospital of Nantong University (No.2022KT244). The study was conducted in accordance with the Declaration of Helsinki (as revised in 2013). Informed consent was provided by all participants.
Open Access Statement: This is an Open Access article distributed in accordance with the Creative Commons Attribution-NonCommercial-NoDerivs 4.0 International License (CC BY-NC-ND 4.0), which permits the non-commercial replication and distribution of the article with the strict proviso that no changes or edits are made and the original work is properly cited (including links to both the formal publication through the relevant DOI and the license). See: https://creativecommons.org/licenses/by-nc-nd/4.0/.
References
- Wang L, Wan YY. The role of gut microbiota in liver disease development and treatment. Liver Res 2019;3:3-18. [Crossref] [PubMed]
- Zepeda-Bastida A, Ocampo-López J, Alarcón-Sánchez BR, et al. Aqueous extracts from Tenebrio molitor larval and pupal stages inhibit early hepatocarcinogenesis in vivo. J Zhejiang Univ Sci B 2021;22:1045-52. [Crossref] [PubMed]
- Ding XX, Zhu QG, Zhang SM, et al. Precision medicine for hepatocellular carcinoma: driver mutations and targeted therapy. Oncotarget 2017;8:55715-30. [Crossref] [PubMed]
- Khattab M, Fouad M, Ahmed E. Role of biomarkers in the prediction and diagnosis of hepatocellular carcinoma. World J Hepatol 2015;7:2474-81. [Crossref] [PubMed]
- Beyoğlu D, Idle JR. Metabolomic and Lipidomic Biomarkers for Premalignant Liver Disease Diagnosis and Therapy. Metabolites 2020;10:50. [Crossref] [PubMed]
- Mansfeld J, Güttinger S, Hawryluk-Gara LA, et al. The conserved transmembrane nucleoporin NDC1 is required for nuclear pore complex assembly in vertebrate cells. Mol Cell 2006;22:93-103. [Crossref] [PubMed]
- D'Angelo MA, Hetzer MW. Structure, dynamics and function of nuclear pore complexes. Trends Cell Biol 2008;18:456-66. [Crossref] [PubMed]
- Hong SH, Son KH, Ha SY, et al. Nucleoporin 210 Serves a Key Scaffold for SMARCB1 in Liver Cancer. Cancer Res 2021;81:356-70. [Crossref] [PubMed]
- Zhang J, Lv W, Liu Y, et al. Nucleoporin 37 promotes the cell proliferation, migration, and invasion of gastric cancer through activating the PI3K/AKT/mTOR signaling pathway. In Vitro Cell Dev Biol Anim 2021;57:987-97. [Crossref] [PubMed]
- Chen M, Long Q, Borrie MS, et al. Nucleoporin TPR promotes tRNA nuclear export and protein synthesis in lung cancer cells. PLoS Genet 2021;17:e1009899. [Crossref] [PubMed]
- Bersini S, Lytle NK, Schulte R, et al. Nup93 regulates breast tumor growth by modulating cell proliferation and actin cytoskeleton remodeling. Life Sci Alliance 2020;3:e201900623. [Crossref] [PubMed]
- Xu WX, Zhang J, Hua YT, et al. An Integrative Pan-Cancer Analysis Revealing LCN2 as an Oncogenic Immune Protein in Tumor Microenvironment. Front Oncol 2020;10:605097. [Crossref] [PubMed]
- Zhou RS, Zhang EX, Sun QF, et al. Integrated analysis of lncRNA-miRNA-mRNA ceRNA network in squamous cell carcinoma of tongue. BMC Cancer 2019;19:779. [Crossref] [PubMed]
- Guo S, Wu J, Zhou W, et al. Identification and analysis of key genes associated with acute myocardial infarction by integrated bioinformatics methods. Medicine (Baltimore) 2021;100:e25553. [Crossref] [PubMed]
- Liu Z, Li H, Pan S. Discovery and Validation of Key Biomarkers Based on Immune Infiltrates in Alzheimer’s Disease. Front Genet 2021;12:658323. [Crossref] [PubMed]
- Li L, Lei Q, Zhang S, et al. Screening and identification of key biomarkers in hepatocellular carcinoma: Evidence from bioinformatic analysis. Oncol Rep 2017;38:2607-18. [Crossref] [PubMed]
- Liu J, Wang Y, Yin J, et al. Pan-Cancer Analysis Revealed SRSF9 as a New Biomarker for Prognosis and Immunotherapy. J Oncol 2022;2022:3477148. [Crossref] [PubMed]
- Liu Y, Fu S, Zhang Z, et al. GRAMD1A Is a Biomarker of Kidney Renal Clear Cell Carcinoma and Is Associated with Immune Infiltration in the Tumour Microenvironment. Dis Markers 2022;2022:5939021. [Crossref] [PubMed]
- Wei C, Wang B, Peng D, et al. Pan-Cancer Analysis Shows That ALKBH5 Is a Potential Prognostic and Immunotherapeutic Biomarker for Multiple Cancer Types Including Gliomas. Front Immunol 2022;13:849592. [Crossref] [PubMed]
- Yoshihara K, Shahmoradgoli M, Martínez E, et al. Inferring tumour purity and stromal and immune cell admixture from expression data. Nat Commun 2013;4:2612. [Crossref] [PubMed]
- Gao J, Aksoy BA, Dogrusoz U, et al. Integrative analysis of complex cancer genomics and clinical profiles using the cBioPortal. Sci Signal 2013;6:pl1. [Crossref] [PubMed]
- Qiao W, Han Y, Jin W, et al. Overexpression and biological function of TMEM48 in non-small cell lung carcinoma. Tumour Biol 2016;37:2575-86. [Crossref] [PubMed]
- Akkafa F, Koyuncu İ, Temiz E, et al. miRNA-mediated apoptosis activation through TMEM 48 inhibition in A549 cell line. Biochem Biophys Res Commun 2018;503:323-9. [Crossref] [PubMed]
- Jiang XY, Wang L, Liu ZY, et al. TMEM48 promotes cell proliferation and invasion in cervical cancer via activation of the Wnt/β-catenin pathway. J Recept Signal Transduct Res 2021;41:371-7. [Crossref] [PubMed]
- Liu D, Song T. Changes in and challenges regarding the surgical treatment of hepatocellular carcinoma in China. Biosci Trends 2021;15:142-7. [Crossref] [PubMed]
- Pezzuto F, Buonaguro L, Buonaguro FM, et al. The Role of Circulating Free DNA and MicroRNA in Non-Invasive Diagnosis of HBV- and HCV-Related Hepatocellular Carcinoma. Int J Mol Sci 2018;19:1007. [Crossref] [PubMed]
- Chen W, Chen L, Cai Z, et al. Overexpression of annexin A4 indicates poor prognosis and promotes tumor metastasis of hepatocellular carcinoma. Tumour Biol 2016;37:9343-55. [Crossref] [PubMed]
- Zhou F, Shang W, Yu X, et al. Glypican-3: A promising biomarker for hepatocellular carcinoma diagnosis and treatment. Med Res Rev 2018;38:741-67. [Crossref] [PubMed]
- Zhao H, Yan G, Zheng L, et al. STIM1 is a metabolic checkpoint regulating the invasion and metastasis of hepatocellular carcinoma. Theranostics 2020;10:6483-99. [Crossref] [PubMed]
- Zhou Y, Huan L, Wu Y, et al. LncRNA ID2-AS1 suppresses tumor metastasis by activating the HDAC8/ID2 pathway in hepatocellular carcinoma. Cancer Lett 2020;469:399-409. [Crossref] [PubMed]
- Zheng Y, Huang C, Lu L, et al. STOML2 potentiates metastasis of hepatocellular carcinoma by promoting PINK1-mediated mitophagy and regulates sensitivity to lenvatinib. J Hematol Oncol 2021;14:16. [Crossref] [PubMed]
- Lai TH, Wu YY, Wang YY, et al. SEPT12-NDC1 Complexes Are Required for Mammalian Spermiogenesis. Int J Mol Sci 2016;17:1911. [Crossref] [PubMed]
- Yamazumi Y, Kamiya A, Nishida A, et al. The transmembrane nucleoporin NDC1 is required for targeting of ALADIN to nuclear pore complexes. Biochem Biophys Res Commun 2009;389:100-4. [Crossref] [PubMed]
- Kar S. Unraveling Cell-Cycle Dynamics in Cancer. Cell Syst 2016;2:8-10. [Crossref] [PubMed]
- Cui XH, Peng QJ, Li RZ, et al. Cell division cycle associated 8: A novel diagnostic and prognostic biomarker for hepatocellular carcinoma. J Cell Mol Med 2021;25:11097-112. [Crossref] [PubMed]
- Zhang L, Kang W, Lu X, et al. LncRNA CASC11 promoted gastric cancer cell proliferation, migration and invasion in vitro by regulating cell cycle pathway. Cell Cycle 2018;17:1886-900. [Crossref] [PubMed]
- Lee J, Kim K, Ryu TY, et al. EHMT1 knockdown induces apoptosis and cell cycle arrest in lung cancer cells by increasing CDKN1A expression. Mol Oncol 2021;15:2989-3002. [Crossref] [PubMed]
- Lupu R, Cardillo M, Harris L, et al. Interaction between erbB-receptors and heregulin in breast cancer tumor progression and drug resistance. Semin Cancer Biol 1995;6:135-45. [Crossref] [PubMed]
- de Graeff P, Crijns AP, Ten Hoor KA, et al. The ErbB signalling pathway: protein expression and prognostic value in epithelial ovarian cancer. Br J Cancer 2008;99:341-9. [Crossref] [PubMed]
- Karlsen EA, Kahler S, Tefay J, et al. Epidermal Growth Factor Receptor Expression and Resistance Patterns to Targeted Therapy in Non-Small Cell Lung Cancer: A Review. Cells 2021;10:1206. [Crossref] [PubMed]
- Hopkins BD, Goncalves MD, Cantley LC. Insulin-PI3K signalling: an evolutionarily insulated metabolic driver of cancer. Nat Rev Endocrinol 2020;16:276-83. [Crossref] [PubMed]
- Mossmann D, Park S, Hall MN. mTOR signalling and cellular metabolism are mutual determinants in cancer. Nat Rev Cancer 2018;18:744-57. [Crossref] [PubMed]
- Misiorek JO, Przybyszewska-Podstawka A, Kałafut J, et al. Context Matters: NOTCH Signatures and Pathway in Cancer Progression and Metastasis. Cells 2021;10:94. [Crossref] [PubMed]
- Zhang C, Liu J, Xu D, et al. Gain-of-function mutant p53 in cancer progression and therapy. J Mol Cell Biol 2020;12:674-87. [Crossref] [PubMed]
- Taciak B, Pruszynska I, Kiraga L, et al. Wnt signaling pathway in development and cancer. J Physiol Pharmacol 2018;
- Cortes-Ciriano I, Lee S, Park WY, et al. A molecular portrait of microsatellite instability across multiple cancers. Nat Commun 2017;8:15180. [Crossref] [PubMed]
- Addeo A, Friedlaender A, Banna GL, et al. TMB or not TMB as a biomarker: That is the question. Crit Rev Oncol Hematol 2021;163:103374. [Crossref] [PubMed]
- Wang P, Chen Y, Wang C. Beyond Tumor Mutation Burden: Tumor Neoantigen Burden as a Biomarker for Immunotherapy and Other Types of Therapy. Front Oncol 2021;11:672677. [Crossref] [PubMed]
- Mao X, Xu J, Wang W, et al. Crosstalk between cancer-associated fibroblasts and immune cells in the tumor microenvironment: new findings and future perspectives. Mol Cancer 2021;20:131. [Crossref] [PubMed]
- Liu X, Wu S, Yang Y, et al. The prognostic landscape of tumor-infiltrating immune cell and immunomodulators in lung cancer. Biomed Pharmacother 2017;95:55-61. [Crossref] [PubMed]
- He X, Xu C. Immune checkpoint signaling and cancer immunotherapy. Cell Res 2020;30:660-9. [Crossref] [PubMed]
- Chakrabarti R, Kapse B, Mukherjee G. Soluble immune checkpoint molecules: Serum markers for cancer diagnosis and prognosis. Cancer Rep (Hoboken) 2019;2:e1160. [Crossref] [PubMed]
- Darvin P, Toor SM, Sasidharan Nair V, et al. Immune checkpoint inhibitors: recent progress and potential biomarkers. Exp Mol Med 2018;50:1-11. [Crossref] [PubMed]
- Zhang YL, Li Q, Yang XM, et al. SPON2 Promotes M1-like Macrophage Recruitment and Inhibits Hepatocellular Carcinoma Metastasis by Distinct Integrin-Rho GTPase-Hippo Pathways. Cancer Res 2018;78:2305-17. [Crossref] [PubMed]
- Jin Z, Lei L, Lin D, et al. IL-33 Released in the Liver Inhibits Tumor Growth via Promotion of CD4(+) and CD8(+) T Cell Responses in Hepatocellular Carcinoma. J Immunol 2018;201:3770-9. [Crossref] [PubMed]
- Xu X, Tan Y, Qian Y, et al. Clinicopathologic and prognostic significance of tumor-infiltrating CD8+ T cells in patients with hepatocellular carcinoma: A meta-analysis. Medicine (Baltimore) 2019;98:e13923. [Crossref] [PubMed]
- Brunner SM, Itzel T, Rubner C, et al. Tumor-infiltrating B cells producing antitumor active immunoglobulins in resected HCC prolong patient survival. Oncotarget 2017;8:71002-11. [Crossref] [PubMed]
- Chen Y, Wen H, Zhou C, et al. TNF-α derived from M2 tumor-associated macrophages promotes epithelial-mesenchymal transition and cancer stemness through the Wnt/β-catenin pathway in SMMC-7721 hepatocellular carcinoma cells. Exp Cell Res 2019;378:41-50. [Crossref] [PubMed]
- Park H, Jung JH, Jung MK, et al. Effects of transarterial chemoembolization on regulatory T cell and its subpopulations in patients with hepatocellular carcinoma. Hepatol Int 2020;14:249-58. [Crossref] [PubMed]
- Shao Y, Lo CM, Ling CC, et al. Regulatory B cells accelerate hepatocellular carcinoma progression via CD40/CD154 signaling pathway. Cancer Lett 2014;355:264-72. [Crossref] [PubMed]
- Macek Jilkova Z, Aspord C, Decaens T. Predictive Factors for Response to PD-1/PD-L1 Checkpoint Inhibition in the Field of Hepatocellular Carcinoma: Current Status and Challenges. Cancers (Basel) 2019;11:1554. [Crossref] [PubMed]
- Acloque H, Adams MS, Fishwick K, et al. Epithelial-mesenchymal transitions: the importance of changing cell state in development and disease. J Clin Invest 2009;119:1438-49. [Crossref] [PubMed]
(English Language Editor: A. Kassem)