Construction and validation of a prognostic nomogram for locally recurrent rectal cancer: a population-based study
Highlight box
Key findings
• The first nomogram for survival of locally recurrent rectal cancer was derived using commonly available clinicopathological factors.
What is known and what is new?
• It was difficult to predict the prognosis of LRRC, and some clinicopathologic factors may be associated with the survival of LRRC.
• We combine the specific clinicopathologic factors to create the first prediction model to evaluate the survival of LRRC patients.
What is the implication, and what should change now?
• This nomogram might help clinicians provide personalized treatment and clinical decisions in LRRC. Actually, we need more patients with locally recurrent rectal cancer to validate the model’s capabilities.
Introduction
Colorectal cancer is currently one of the most common tumors worldwide, with an estimated 151,030 new cases in America in 2022, with rectal cancer accounting for approximately 30% (1). With the introduction of total mesorectal excision, the application of perioperative chemoradiotherapy, and the progression of multidisciplinary treatment strategies, the incidence of locally recurrent rectal cancer (LRRC) has decreased to less than 5% in recent randomized control trials (2,3). However, the postoperative recurrence of rectal cancer remains a thorny problem.
Treatment of LRRC is challenging. Radical resection is considered to be the preferred treatment for LRRC. Radiotherapy, especially neoadjuvant radiotherapy for LRRC has been gradually valued by specialists (4,5). Chemotherapy, immunotherapy, and hyperthermia are commonly used in patients with nonsurgical treatment. However, the impact of these non-surgical treatments is inconclusive due to the limited evidence of prospective studies. There is still no “gold standard” therapeutic strategy for LRRC.
It is difficult to estimate the survival of LRRC after treatment. Although it has been reported in studies that types of recurrence (6), surgery (7,8), neoadjuvant therapy (9,10), age (11,12), carcinoembryonic antigen (CEA) (13), systemic inflammatory-related parameters (14), and other indicators are associated with survival rate, their predictive power has not been widely recognized. Therefore, there is an urgent need for a simple and sensitive assessment model to allow the prediction of therapy outcomes and to individualize therapeutic protocols.
As a prognostic tool, the nomogram incorporates important clinicopathological factors to predict individual survival outcomes by quantifying the impact of each variable and presenting the results graphically. In this current study, the clinicopathological factors of LRRC from the National Cancer Institute’s Surveillance, Epidemiology, and End Results (SEER) database were analyzed, and the predictive ability of these factors was evaluated. A novel nomogram was built to assess survival prognosis in LRRC and validate its predictive power. We present this article in accordance with the TRIPOD reporting checklist (available at https://jgo.amegroups.com/article/view/10.21037/jgo-22-995/rc).
Methods
Patient cohort
This study population was assembled from the SEER database. SEER*Stat version 8.4.0 (http://www.seer.cancer.gov/seerstat). was applied to retrospectively search patients with LRRC from the SEER database. LRRC was defined as the presence of a tumor with high biological similarity to the primary tumor in the surgical field after radical surgical treatment of rectal cancer, with or without distant metastasis. A total of 1,120 patients, according to the 3rd Edition of the International Classification of Diseases for Oncology (ICD-O-3) of the rectum (C19.9, C20.9) who had a local recurrence between 2004 and 2019, were identified. The variable for local recurrence was identified by creating a cohort of patients who had a primary tumor site meeting the inclusion criteria and who had the same tumor at the same site during follow-up. The inclusion criteria were (I) pathologically diagnosed rectal cancer (ICD-O3: C19.9, C20.9). (II) Complete follow-up and survival data. (III) The histology was adenocarcinoma, mucinous adenocarcinoma, or signet-ring cell carcinoma. The exclusion criteria: (I) the M stage of primary tumor was M1/unknown. (II) Concomitant history of other tumors. (III) Multiple tumor recurrence (≥2). The final analytic sample comprised 744 individuals. The study was conducted in accordance with the Declaration of Helsinki (as revised in 2013).
The following clinicopathological variables were identified from the dataset: age, gender (male or female), race (white, black, or others), marital status (married, unmarried, or unknown), histological type of primary tumor or recurrent tumor (adenocarcinoma, mucinous adenocarcinoma or signet ring cell carcinoma), grade of the primary tumor or recurrent tumor (I/II, III/IV or unknown), M stage of recurrent tumor (MLRRC) (M0, M1 or unknown), stage of primary tumor (0/I, II/III or unknown), chemotherapy of the primary tumor or recurrent tumor (no/unknown or yes), surgery of the primary tumor or recurrent tumor (no surgery, local tumor excision, wedge or segmental resection, resection with other organs or unknown), radiotherapy of the primary tumor (no radiotherapy, radiotherapy before surgery, radiotherapy after surgery or other types), radiotherapy of recurrent tumor (no radiotherapy, radiotherapy before surgery, radiotherapy after surgery or other types), systemic therapy of recurrent tumor (no systemic therapy, systemic therapy before surgery, systemic therapy after surgery or other types), treatment gap (the interval from the detection of relapse to the initiation of treatment), regional nodes examined of primary or recurrent tumor (n<12 or n≥12), perineural invasion of primary or recurrent tumor (negative, positive or unknown), time of recurrence, tumor size (<3 cm, ≥3 cm or unknown), CEA {positive [>5 ng/mL (for smokers) and >2.5 ng/mL (for nonsmokers)], negative or unknown}, tumor deposits (TD) (negative, positive or unknown), survival information. The primary endpoint outcome of the study was 3-, 5-year overall survival (OS). OS was defined as the time from diagnosis until death or last follow-up.
Based on the advantage of a relatively larger sample size of SEER database, the cohort was randomly split into a 70% training sample (n=503) for model development and a 30% test sample (n=241) for model evaluation in R. Multiple imputations by chained equations (MICE) were used to replace missing value and maintain the stability of the model. Imputed variables were marital status, grade of the primary tumor or recurrent tumor, MLRRC stage, stage of primary tumor, surgery of the primary tumor or recurrent tumor, systemic therapy of recurrent tumor, treatment gap, regional nodes examined of primary or recurrent tumor, time of recurrence, tumor size, perineural invasion of primary or recurrent tumor. After imputation, univariate and multivariate analyses were performed to find correlations between the clinicopathological variables and OS in training set.
Establishing the nomogram
The least absolute shrinkage and selection operator (LASSO) regression is a type of linear least-squares regression that includes a regularization penalty for non-zero coefficients. As the LASSO regression was used to select the most valuable predict factors associated with OS by effectively avoiding redundancy or over-fitting. Before further construction of the Cox proportional hazards model, all the included variables must satisfy the proportional hazards assumption. Nomogram was used to predict 3- and 5-year survival in LRRC. The effectiveness of the nomogram was tested by determining its discriminatory ability by Harrel’s concordance index (C-index), calibration curve, and decision curve analysis. The closer C-index was to 1, the more accurate nomogram predictions would be. The calibration curve, which was based on a random resampling procedure with 1,000 resamples, intuitively displayed the consistency between the predicted survival rate and the actual survival data, the calibration plots resembled a 45-degree line in a well-calibrated model. Decision curve analysis was used to evaluate the clinical utility of the nomogram, the curve with the high benefit was the great option, which meant that it was of great clinical utility. Then the nomogram was used to calculate the risk scores of all patients, and according to the above scores, the patients were divided into three groups: high-risk, medium-risk, and low-risk by the X-tile software (15).
Statistical analysis
Count and percentage were used to describe categorical measurements, while mean and range were applied to present continuous measurements. The Chi-squared test was used to compare the categorical measurements, while the t-test or Mann-Whitney U-test was used to compare continuous variables. Survival analysis was performed by the Kaplan-Meier (KM) analysis and log-rank test. All analyses were double-sided, and a P value lower than 0.05 was deemed statistically significant. All analyses were performed using the statistical software R version 4.1.2 (https://www.r-project.org/) (for Windows). The “mice”, “survival”, “glmnet”, “rms”, “pec”, “ggDCA”, “nomogramFormula”, and “survminer” packages in R were used to perform the analyses above.
Results
Patient demographics
A total of 744 patients, diagnosed with LRRC from 2004 to 2019, were included in the study (Figure 1). Data on age, gender, marital status, tumor size, surgery, radiotherapy, and other clinicopathological factors were obtained from the SEER database. These populations were randomized into the training set (503 patients) and the validation set (241 patients). The 1-, 3-, 5-year survival rates of all patients were 75%, 37.9%, and 20.8%. The 1-, 3-, 5-year survival rates in the training set were 73.3%, 38.6%, 21.4%; the 1-, 3-, 5-year survival rates in the testing set were 78.6%, 36.3%, 19.7%. No significant difference was observed between the two sets and the overall data. The demographic characteristics are summarized in Table S1.
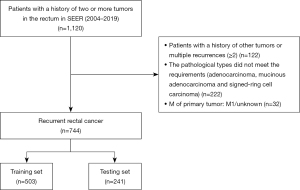
Independent predictors for OS of LRRC
In the training set, all the clinicopathological factors were extracted to construct a Cox regression model (Table 1, detailed information is provided in Table S2). The univariate analysis demonstrated that age, marital status, histological type of primary tumor and recurrent tumor, MLRRC stage, surgery, stage of primary tumor, radiotherapy of primary tumor and recurrent tumor, systemic therapy, regional nodes examined, tumor size, CEA, and TD were correlated with OS (all P<0.05). The variables were further used for the multivariate analysis, which demonstrated that age [hazard ratio (HR) =1.0189; 95% CI: 1.0089–1.029; P<0.001], marital status (HR =1.3454; 95% CI: 1.0443–1.7334; P=0.0217), stage of primary tumor (HR =1.6906; 95% CI: 1.2712–2.2473; P<0.001), MLRRC stage (HR =1.9461; 95% CI: 1.4349–1.2.6394; P<0.001), surgery (HR =0.4789; 95% CI: 0.3317–0.6914; P<0.001), tumor size (HR =1.4434; 95% CI: 1.102–1.8905; P=0.0077), CEA (HR =1.8954; 95% CI: 1.4491–2.479; P<0.001) were independent prognostic factors of OS.
Table 1
Variable | Univariate Cox regression | Multivariate Cox regression | |||
---|---|---|---|---|---|
HR (95% CI) | P value | HR (95% CI) | P value | ||
Age | 1.025 (1.015–1.035) | <0.001 | 1.0189 (1.0089–1.029) | <0.001 | |
Marital status | |||||
Married | 1 | 1 | |||
Unmarried | 1.497 (1.183–1.895) | <0.001 | 1.3454 (1.0443–1.7334) | 0.0217 | |
Histology type | |||||
Adenocarcinoma | 1 | 1 | |||
Mucinous adenocarcinoma and signet ring cell carcinoma | 1.518 (1.027–2.243) | 0.0364 | 1.3143 (0.8707–1.984) | 0.1933 | |
P. Histology type | |||||
Adenocarcinoma | 1 | 1 | |||
Mucinous adenocarcinoma and signet ring cell carcinoma | 2.653 (1.681–4.189) | <0.001 | 1.5389 (0.9299–2.5466) | 0.0934 | |
P. Stage | |||||
0, I | 1 | 1 | |||
II, III | 1.602 (1.245–2.06) | <0.001 | 1.6906 (1.2712–2.2473) | <0.001 | |
MLRRC stage | |||||
M0 | 1 | 1 | |||
M1 | 2.889 (2.206–3.783) | <0.001 | 1.9461 (1.4349–2.6394) | <0.001 | |
Surgery | |||||
No surgery | 1 | 1 | |||
Local tumor excision | 0.4249 (0.2824–0.6395) | <0.001 | 0.7774 (0.4815–1.2553) | 0.3031 | |
Wedge or segmental resection | 0.2644 (0.2040–0.3426) | <0.001 | 0.4789 (0.3317–0.6914) | <0.001 | |
Resection with other organs | 0.4146 (0.1688–1.0184) | 0.0548 | 0.8412 (0.3208–2.2057) | 0.7251 | |
Radiotherapy | |||||
No radiotherapy | 1 | 1 | |||
Radiotherapy before surgery | 0.374 (0.2435–0.5745) | <0.001 | 0.6695 (0.3168–1.4147) | 0.2932 | |
Radiotherapy after surgery | 0.5821 (0.3949–0.8580) | 0.00626 | 0.9466 (0.5708–1.5699) | 0.8317 | |
Other types | 0.3311 (0.106–1.0347) | 0.05727 | 0.4837 (0.1246–1.877) | 0.2938 | |
P. Radiotherapy | |||||
No radiotherapy | 1 | 1 | |||
Radiotherapy before surgery | 1.093 (0.7956–1.502) | 0.5825 | 1.1391 (0.7899–1.6426) | 0.4858 | |
Radiotherapy after surgery | 0.847 (0.5795–1.238) | 0.3911 | 0.848 (0.566–1.2703) | 0.4239 | |
Other types | 3.644 (1.155–11.496) | 0.0274 | 2.6543 (0.8181–8.6115) | 0.104 | |
Systemic therapy | |||||
No systemic therapy | 1 | 1 | |||
Systemic therapy before surgery | 0.3786 (0.2393–0.599) | <0.001 | 0.866 (0.4021–1.8652) | 0.7133 | |
Systemic therapy after surgery | 0.5608 (0.4008–0.7848) | <0.001 | 0.8176 (0.5204–1.2844) | 0.3821 | |
Other types | 0.3932 (0.2085–0.7416) | 0.0039 | 1.095 (0.4316–2.7559) | 0.8546 | |
Regional nodes examined | |||||
<12 | 1 | 1 | |||
≥12 | 0.4538 (0.3247–0.6342) | <0.001 | 0.6816 (0.462–1.006) | 0.0533 | |
Tumor size (cm) | |||||
<3 | 1 | 1 | |||
≥3 | 1.958 (1.528–2.508) | <0.001 | 1.4434 (1.1020–1.8905) | 0.0077 | |
CEA | |||||
Negative | 1 | 1 | |||
Positive | 2.126 (1.678–2.695) | <0.001 | 1.8954 (1.4491–2.479) | <0.001 | |
Tumor deposits | |||||
Negative | 1 | 1 | |||
Positive | 1.719 (1.034–2.86) | 0.0369 | 1.0867 (0.6359–1.8572) | 0.761 |
HR, hazard ratio; CI, confidence interval; P., the indicators are related to the primary tumor; MLRRC, M stage of recurrent tumor; LRRC, locally recurrent rectal cancer; CEA, carcinoembryonic antigen.
Construction and validation of the nomogram
Based on the result of LASSO regression (Figure 2A,2B), ten factors (age, marital status, MLRRC stage, stage of primary tumor, surgery, radiotherapy, systemic therapy, CEA, regional nodes examined, tumor size) were finally incorporated into the development of a survival nomogram of the training set. The complex Cox regression formulas were transformed into intuitive visual graphics (Figure 3). The C-index was used to judge the predictive ability of the prediction model. The C-index of the nomogram of 3-, 5-year OS in the training set was 0.756 and 0.747, respectively, and it was 0.719 and 0.726 in the testing set (Figure 4). As shown in Figure 5, good concordance between the predicted probabilities and actual outcomes was showed by the calibration curves of the survival nomogram. The decision curves for models are provided in Figure 6, which could verify the effectiveness of the survival nomogram. These results implied that the nomograms were practical and reliable.
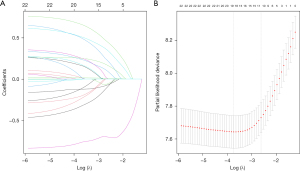
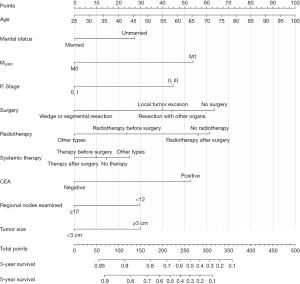
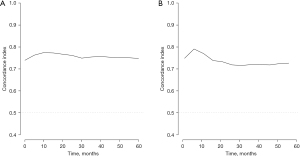
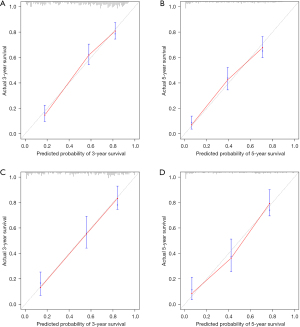
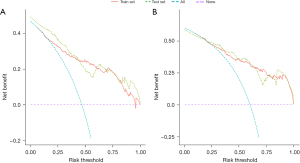
Development of risk stratification system
Based on the established prediction model, the risk scores of all patients were calculated, X-tile was used to calculate two cutoff values, and all patients were divided into three groups (Figure 7), a low-risk group (score ≤261, n=433), a medium-risk group (score 261–320.3, n=138), and a high-risk group (score ≥320.3, n=163). The 3-, 5-year survival rates in the low-risk group were 53.3%, 32.3%, while these rates were 28.4%, 10.1% in the medium-risk group, and 5.5%, 0% in the high-risk group. The difference was statistically significant (P<0.001). Likewise, we divided the training set and testing sets into low-risk (n=298 in training set and n=135 in testing set), medium-risk (n=98 in training set and n=50 in testing set), and high-risk groups (n=114 in training set and n=49 in testing set), respectively (Figure 8). In the training set, the 3-, 5-year survival rates in the low-risk group were 56%, 33.6%, the 3-, 5-year survival rates were 24.5%, 9.2% in the medium-risk group, and 5.3%, 0% in the high-risk group. The difference was statistically significant (P<0.001); in the testing set, the 3-, 5-year survival rates in the low-risk group were 47.4%, 29.6%, 36%, 12% in the medium-risk group, and 6.1%, 0% in the high-risk group. The difference was statistically significant (P<0.001).

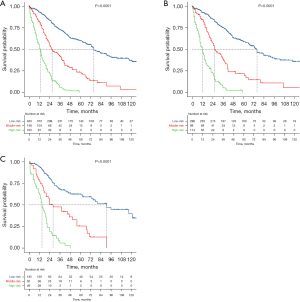
Discussion
Despite the progression of surgical techniques and improvement of multidisciplinary treatment, LRRC is still a major concern for colorectal specialists due to the poor prognosis and massive damage to quality of life. In recent years, the type of recurrent tumor and R0 resection has been often used to evaluate the survival prognosis of patients. R0 resection is recognized as an independent predictor of postoperative survival of LRRC (7,16). Nevertheless, due to the surgical history of the primary tumor, radiation, and large tumor size, the plane of the operation might be distorted, which might raise the risk of R+ resection. Coupled with the inconsistency of treatment strategies among current medical centers, clinical efficacy is always mixed. Considering that R0 resection is the most important prognostic factor for survival, it is reasonable to combine neoadjuvant therapy to improve the R0 resection rate. A retrospective study of 152 patients with LRRC at the National Cancer Institute of Milan (NCIM) found that patients who received neoadjuvant therapy combined with surgery achieved better OS (1-, 3-, 5-year OS: 90.7%, 56.4%, 46.3% in neoadjuvant chemoradiotherapy plus surgery vs. 80.4%, 48.1%, 21.9% in upfront surgery, P=0.048) (9). In Nordkamp’s (10) study, patients were divided into three groups (full-course chemoradiotherapy group, re-radiotherapy group, and radiotherapy solely for primary tumor group) for survival analysis, and found that patients who received full-course chemoradiotherapy had the highest OS (full-course chemoradiotherapy vs. reirradiation, P=0.005; full-course chemoradiotherapy vs. radiotherapy solely for primary tumor, P=0.003). The European Society for Medical Oncology (4) and the Beyond TME Collaborative group (5) recommended the use of neoadjuvant therapy in combination with other therapies for LRRC patients as much as possible. However, the gold standard treatment for LRRC remains undefined, and the specific treatment still needs to be explored, which is not conclusive enough for doctors and patients to judge the prognosis of survival. Several phase III trials are searching for the optimal perioperative treatment (17), and the result are being anticipated.
The single predictive factor is sometimes discriminative but usually not a good predictor. Tanaka et al. (18) found that a high level of CEA was associated with worse progression-free survival (PFS) (HR =1.82; 95% CI: 1.29–2.58; P=0.01) in their study on the prognosis of LRRC patients undergoing radical surgery, and CEA was an independent predictor of OS (HR =1.86; 95% CI: 1.21–2.84; P=0.004). Sakamoto et al. (13) proposed the CEA doubling time (CEA-DT) for LRRC prognosis, and the 3-year recurrence-free survival (RFS) was significantly better in the long CEA-DT group than in the short CEA-DT group (58.8% vs. 25.0%, P=0.0063). Similarly, in the current study, univariate results revealed CEA was related to OS (HR =1.8954; 95% CI: 1.4491–2.479; P<0.001). Synchronous distant metastasis was one of the major problems in LRRC, with a distant metastasis rate as high as 48% (19). Due to the poor therapeutic effect of LRRC with distant metastasis, it was considered an absolute contraindication for surgical treatment in the past, but the results varied by Cyr’s research, there was a significant difference in median disease-specific survival (DSS) between M0 and M1 after surgical treatment (60 vs. 21 months, P=0.002) (7). However, in Voogt’s study, although patients with synchronous metastasis tended to have worse OS than patients without synchronous metastasis (HR =1.43; 95% CI: 0.98–2.11), the history of metastasis did not affect OS (20). Both studies were based on large referral centers and excluded unresectable M1 patients, whereas patients were based on broader inclusion criteria. We found that there was a significant difference in survival between M0 and M1 in multivariate analysis (HR =1.9461; 95% CI: 1.4349–2.6394; P<0.001). In general, not all M1 patients were equal. But few studies have focused on the survival difference of LRRC with different metastasis. Because most specific metastasis of LRRC in SEER database is in missing value, we did not further explore the effect of different metastatic site. We looked forward to future studies to improve this point. Surgical treatment was still the preferred treatment for resectable LRRC. R0 resection could improve OS significantly. Hagemans et al. (8) reported 447 LRRC patients in their center from 2000 to 2015. The 5-year OS of patients with R0/R1 resection was significantly better than that of non-surgical patients (R0: 51% vs. 4%, P<0.001; R1: 34% vs. 4%, P<0.001). Similar results were seen in another ultra-long-term study (7), in which patients after surgery had 41%, 33%, and 31% DSS at 5, 10, and 15 years. And if the patient achieved R0 resection, the DSS at 5, 10, and 15 years was as high as 58%, 47%, and 44%. In this study, the survival advantage of surgery was validated again (HR =0.4789; 95% CI: 0.3317–0.6914; P<0.001). Radical surgery should still be the first consideration for most patients. However, the wide range of procedures would also increase the risk of complications, such as “empty basin syndrome” that result from pelvic resection. In our multivariate analysis, there was no significant difference in survival prognosis between patients who underwent resection with other organs and those who did not undergo surgery (HR =0.8412; 95% CI: 0.3208–2.2057; P=0.7251). This might be related to the serious complications caused by the combined resection of organs.
Anatomic classification systems and novel clinical markers had also been used to predict the survival of LRRC. Surgeons often used the classification system of LRRC to predict the probability of R0 resection, thereby indirectly predicting patient survival prognosis. A variety of LRRC classification models were proposed by scholars from different countries in different eras. In 2021, Sorrentino et al. (6) evaluated nine different classification systems for LRRC, by analyzing 152 LRRC from their center. Four of the NCIM patterns (S1a: P=0.04, S1b: P=0.002, S2b: P=0.004, S3: P=0.01) were significantly correlated with the resection status, and the NCIM was considered the most predictive classification for R0 surgery. However, the defect of this study was that patients in that research were screened according to the NCIM system proposed by their center. This might partly explain the better predictive effect of the NCIM classification system for R0 resection. It was recognized that the anatomical classification of preoperative imaging was correlated with survival prognosis. Unfortunately, these classification data, which might increase the predictive power of the model, were lacking in the database. Systemic inflammatory-related parameters as potential prognostic factors were also used to predict the prognosis of LRRC, neutrophil-to-lymphocyte ratio >3.9 (HR =3.96; P=0.049) and platelet-to-lymphocyte ratio >275 (HR =5.39; P=0.002) were considered to be associated with poorer OS (14). However, these predictors could only provide rough estimates of patient survival prognosis, not survival rates. It is necessary to establish a prediction model based on preoperative clinical data to determine whether OS is beneficial. Our study used a large sample database to screen for factors associated with the OS of LRRC, and then a nomogram was constructed to assess the patient’s survival rate to provide accurate and personalized treatment options for LRRC.
A growing body of research reported the value of nomogram that has been widely used in clinical oncology, with intuitive graphs simplifying the use of prediction models (21,22). But it was absent in the nomogram for LRRC. In our study, based on LASSO regression, we identified age, marital status, MLRRC stage, stage of primary tumor, surgery, radiotherapy, systemic therapy, CEA, regional nodes examined, and tumor size as informative factors, and incorporated them in the establishment of the prediction model. Both in the training set and the testing set, the C-index of the new model was greater than 0.7 each time, indicating that the model had excellent discrimination ability. Calibration diagrams and decision curve analysis also demonstrated better accuracy and clinical applicability. Then the nomogram was used to score all the patients and divided them into three groups: low-risk, medium-risk, and high-risk group. From the survival analysis, we could find that there were significant differences in survival prognosis among the three groups (P<0.001), indicating that our risk stratification was reasonably valid. The nomogram was valuable for patient counseling, helping clinicians predict the likelihood of improvement in the clinical outcomes of each patient. In addition, all the variables contained in models can be easily obtained, which facilitated the application of nomograms in clinical practice. Although we intended to consider all the predictors of survival, some important predictors were missing in the database, like preoperative imaging classification, specific application of neoadjuvant therapy, etc. In the future, LRRC prognosis-related genes and tumor immune microenvironment could also be considered for model establishment. Guinney (23) summarized four consensus molecular subtypes (CMS) with distinguishing features: CMS1: hypermutated, microsatellite unstable, strong immune activation; CMS2: epithelial, chromosomally unstable, marked WNT and MYC signaling activation; CMS3: epithelial, evident metabolic dysregulation; and CMS4: prominent transforming growth factor β activation, stromal invasion, and angiogenesis. And CMS2 had a higher survival rate after tumor recurrence, and CMS1 had a poor survival rate.
This study had several limitations. First, this study was a retrospective study, and some patients were not included in this study due to a lack of data, which might be biased. Second, although MICE was a good imputation technique, since the data might not be missing at random, and this might introduce some bias. Third, due to limitations of the SEER database, some interesting indicators, such as the detailed treatment protocol and specific metastasis, were lacking. We looked forward to building models based on these indicators. Currently, no prognostic survival nomogram exists that could incorporate the above clinical-pathological factors. Developing such a nomogram is important because it can help to stratify the risk of patients and provide individualized guidance for clinical management of LRRC.
Conclusions
In conclusion, a nomogram for predicting OS in patients of LRRC was first being developed and validated in this study. This nomogram showed sufficient predictive power, discrimination, and good clinical application value and might help clinicians provide personalized treatment and clinical decisions in LRRC.
Acknowledgments
Funding: None.
Footnote
Reporting Checklist: The authors have completed the TRIPOD reporting checklist. Available at https://jgo.amegroups.com/article/view/10.21037/jgo-22-995/rc
Peer Review File: Available at https://jgo.amegroups.com/article/view/10.21037/jgo-22-995/prf
Conflicts of Interest: All authors have completed the ICMJE uniform disclosure form (available at https://jgo.amegroups.com/article/view/10.21037/jgo-22-995/coif). The authors have no conflicts of interest to declare.
Ethical Statement: The authors are accountable for all aspects of the work in ensuring that questions related to the accuracy or integrity of any part of the work are appropriately investigated and resolved. The study was conducted in accordance with the Declaration of Helsinki (as revised in 2013).
Open Access Statement: This is an Open Access article distributed in accordance with the Creative Commons Attribution-NonCommercial-NoDerivs 4.0 International License (CC BY-NC-ND 4.0), which permits the non-commercial replication and distribution of the article with the strict proviso that no changes or edits are made and the original work is properly cited (including links to both the formal publication through the relevant DOI and the license). See: https://creativecommons.org/licenses/by-nc-nd/4.0/.
References
- Siegel RL, Miller KD, Fuchs HE, et al. Cancer statistics, 2022. CA Cancer J Clin 2022;72:7-33. [Crossref] [PubMed]
- Fleshman J, Branda ME, Sargent DJ, et al. Disease-free Survival and Local Recurrence for Laparoscopic Resection Compared With Open Resection of Stage II to III Rectal Cancer: Follow-up Results of the ACOSOG Z6051 Randomized Controlled Trial. Ann Surg 2019;269:589-95. [Crossref] [PubMed]
- Bonjer HJ, Deijen CL, Abis GA, et al. A randomized trial of laparoscopic versus open surgery for rectal cancer. N Engl J Med 2015;372:1324-32. [Crossref] [PubMed]
- Glynne-Jones R, Wyrwicz L, Tiret E, et al. Rectal cancer: ESMO Clinical Practice Guidelines for diagnosis, treatment and follow-up. Ann Oncol 2018;29:iv263. [Crossref] [PubMed]
- Consensus statement on the multidisciplinary management of patients with recurrent and primary rectal cancer beyond total mesorectal excision planes. Br J Surg 2013;100:E1-33. [Crossref] [PubMed]
- Sorrentino L, Belli F, Guaglio M, et al. Prediction of R0/R+ surgery by different classifications for locally recurrent rectal cancer. Updates Surg 2021;73:539-45. [Crossref] [PubMed]
- Cyr DP, Zih FS, Wells BJ, et al. Long-term outcomes following salvage surgery for locally recurrent rectal cancer: A 15-year follow-up study. Eur J Surg Oncol 2020;46:1131-7. [Crossref] [PubMed]
- Hagemans JAW, van Rees JM, Alberda WJ, et al. Locally recurrent rectal cancer; long-term outcome of curative surgical and non-surgical treatment of 447 consecutive patients in a tertiary referral centre. Eur J Surg Oncol 2020;46:448-54. [Crossref] [PubMed]
- Sorrentino L, Belli F, Valvo F, et al. Neoadjuvant (re)chemoradiation for locally recurrent rectal cancer: Impact of anatomical site of pelvic recurrence on long-term results. Surg Oncol 2020;35:89-96. [Crossref] [PubMed]
- Nordkamp S, Voogt ELK, van Zoggel DMGI, et al. Locally recurrent rectal cancer: oncological outcomes with different treatment strategies in two tertiary referral units. Br J Surg 2022;109:623-31. [Crossref] [PubMed]
- Rydbeck D, Asplund D, Bock D, et al. Younger age at onset of colorectal cancer is associated with increased patient's delay. Eur J Cancer 2021;154:269-76. [Crossref] [PubMed]
- Ketelaers SHJ, Voogt ELK, Simkens GA, et al. Age-related differences in morbidity and mortality after surgery for primary clinical T4 and locally recurrent rectal cancer. Colorectal Dis 2021;23:1141-52. [Crossref] [PubMed]
- Sakamoto J, Ozawa H, Nakanishi H, et al. Usefulness of Carcinoembryonic Antigen Doubling Time in Prognosis Prediction after Curative Resection of Locally Recurrent Rectal Cancer: A Retrospective Study. Dig Surg 2022;39:17-23. [Crossref] [PubMed]
- Daveri E, Sorrentino L, Lalli L, et al. Low baseline neutrophil-to-lymphocyte and platelet-to-lymphocyte ratios predict increased overall survival in locally recurrent rectal cancer despite R1 margins. Dig Liver Dis 2022;54:864-70. [Crossref] [PubMed]
- Camp RL, Dolled-Filhart M, Rimm DL. X-tile: a new bio-informatics tool for biomarker assessment and outcome-based cut-point optimization. Clin Cancer Res 2004;10:7252-9. [Crossref] [PubMed]
- Lau YC, Jongerius K, Wakeman C, et al. Influence of the level of sacrectomy on survival in patients with locally advanced and recurrent rectal cancer. Br J Surg 2019;106:484-90. [Crossref] [PubMed]
- Induction chemotherapy followed by chemoradiotherapy versus chemoradiotherapy alone as neoadjuvant treatment for locally recurrent rectal cancer: study protocol of a multicentre, open-label, parallel-arms, randomized controlled study (PelvEx II). BJS Open 2021;5:zrab029. [Crossref] [PubMed]
- Tanaka M, Kanemitsu Y, Shida D, et al. Prognostic Impact of Intra-abdominal/Pelvic Inflammation After Radical Surgery for Locally Recurrent Rectal Cancer. Dis Colon Rectum 2017;60:827-36. [Crossref] [PubMed]
- van den Brink M, Stiggelbout AM, van den Hout WB, et al. Clinical nature and prognosis of locally recurrent rectal cancer after total mesorectal excision with or without preoperative radiotherapy. J Clin Oncol 2004;22:3958-64. [Crossref] [PubMed]
- Voogt ELK, van Zoggel DMGI, Kusters M, et al. Impact of a history of metastases or synchronous metastases on survival in patients with locally recurrent rectal cancer. Colorectal Dis 2021;23:1120-31. [Crossref] [PubMed]
- Liu X, Zheng S, Peng Y, et al. Construction of the Prediction Model for Locally Advanced Rectal Cancer Following Neoadjuvant Chemoradiotherapy Based on Pretreatment Tumor-Infiltrating Macrophage-Associated Biomarkers. Onco Targets Ther 2021;14:2599-610. [Crossref] [PubMed]
- Li Y, Liu Z, Zhao X, et al. Nomogram for predicting survival of renal cell carcinoma with tumor thrombus based on perioperative clinicopathological factors from a Chinese high-volume center. Int J Urol 2022;29:984-93. [Crossref] [PubMed]
- Guinney J, Dienstmann R, Wang X, et al. The consensus molecular subtypes of colorectal cancer. Nat Med 2015;21:1350-6. [Crossref] [PubMed]