A bioinformatics analysis and an experimental validation of the hypoxia-related prognostic model
Highlight box
Key findings
• We established a prognostic model based on 4 hypoxia-related genes (BHLHE40, ENO1, SDC4, and TGM2). The model can be used to predict the prognosis and assess the TME of PCA patients. Activation of the BHLHE40/TLR3 axis promoted invasion and migration of PCA cells.
What is known and what is new?
• Hypoxia in PCA is associated with tumor progression and poor prognosis. Hypoxia operates on immune cells to promote an immunosuppressive TME.
• We established a hypoxia-related prognostic model to predict the prognosis and assess the TME of PCA patients. The activation of BHLHE40/TLR3 axis promoted invasion and migration of PCA cells.
What is the implication, and what should change now?
• This study may provide new insights into how hypoxia affects the prognosis and TME of PCA patients and may benefit hypoxia-targeted therapies in the future.
Introduction
Pancreatic cancer (PCA) is a deadly malignant tumor. Its mortality is increasing year by year, and it is expected to become the second most common cause of cancer-related death by 2030 (1). The 5-year survival rate of PCA patients is less than 10% (2).
Hypoxia, as a major feature of the tumor microenvironment (TME), exists in most malignancies. PCA is characterized by numerous and severe hypoxic regions with a median oxygen pressure (pO2) of 0–5.3 mmHg (0–0.7%), whereas the pO2 of the adjacent normal pancreas is 24.3–92.7 mmHg (3.2–12.3%) (3). The presence of hypoxic regions within PCA is closely associated with tumor progression and poor prognosis (4,5). Moreover, hypoxia operates on various immune cells to promote an immunosuppressive TME, suggesting that it may be one of the critical factors leading to the failure of immune checkpoint inhibitor (ICI) treatment in PCA (6,7).
The hypoxic microenvironment promotes tumor metastasis, and HIF-1α plays an important role in this process (8). Under hypoxia, HIF-1α can activate the hepatocyte growth factor (HGF)/HGF receptor (c-MET) signaling pathways that loosen the adhesion between tumor cells and degrade extracellular matrix. More importantly, the ability of tumor cells to invade and metastasize is enhanced (9). Hypoxia can also induce epithelial-mesenchymal transition (EMT) in tumor cells, inhibiting the expression of the epithelial cell marker E-cadherin and greatly increasing the expression of mesenchymal cell markers such as N-cadherin, vimentin, and Snail. Therefore, tumor cells exhibit more mesenchymal-like features and are more likely to metastasize (10).
Research have shown that the carbohydrate antigen 19-9 (CA19-9) and the carcinoembryonic antigen (CEA) can be used as biomarkers to predict the prognosis of many cancers, including PCA (11,12). However, the specificity or sensitivity for use in PCA of these biomarkers are not sufficient. The construction of a prognostic model based on the expression of multiple genes in PCA is more meaningful for predicting the prognosis of PCA patients. Previous studies have explored the predictive role of hypoxia-related prognostic models in the prognosis of hepatocellular carcinoma (13), glioma (14), and breast cancer (15) patients. This study aimed to develop a hypoxia-related prognostic model to predict the prognosis of PCA patients and assess the TME. Moreover, we used data from the Gene Expression Omnibus (GEO) database to verify the prognostic model we established. The prognostic model can help researchers to better assess the relationship between hypoxia and prognosis in PCA. In addition, we explored the specific mechanisms by analyzing the biological functions of hypoxia-related genes (HRGs). We present this article in accordance with the TRIPOD reporting checklist (available at https://jgo.amegroups.com/article/view/10.21037/jgo-23-301/rc).
Methods
Datasets
Patient datasets
RNA sequencing (RNA-seq) data and corresponding clinicopathological information from 177 PCA and 4 adjacent normal tissue samples were obtained from The Cancer Genome Atlas (TCGA) database (http://cancergenome.nih.gov/). The University of California Santa Cruz (UCSC) Xena resource (http://xena.ucsc.edu/) was used to obtain data on 167 GTEx healthy pancreatic tissue samples. A training set was created by using TCGA pancreatic cancer data. Subsequently, data for 199 PCA patients from the GSE102238, GSE62452, and GSE85916 datasets were downloaded from the GEO database (https://www.ncbi.nlm.nih.gov/geo/). These 3 GEO datasets were combined for batch normalization, and the combined result was used as a validation set for further analysis. Patients with less than 30 days of survival were excluded from the Cox and Kaplan–Meier (KM) analyses. Detailed information about the training and validation sets is provided in Table 1. The study was conducted in accordance with the Declaration of Helsinki (as revised in 2013).
Table 1
Characteristics | Training set (TCGA), N=177 | Validation set (GEO), N=199 |
---|---|---|
Age category | ||
<65 years | 81 | 28 |
≥65 years | 96 | 22 |
NA | 0 | 149 |
Gender | ||
Male | 97 | 29 |
Female | 80 | 21 |
NA | 0 | 149 |
Status | ||
Alive | 89 | 57 |
Dead | 88 | 136 |
NA | 0 | 6 |
Grade | ||
G1 | 31 | 2 |
G2 | 94 | 35 |
G3 | 48 | 30 |
G4 | 2 | 1 |
NA | 2 | 131 |
Tumor stage | ||
I | 21 | 4 |
II | 146 | 46 |
III | 3 | 13 |
IV | 4 | 6 |
NA | 3 | 130 |
T stage | ||
T1 | 7 | 2 |
T2 | 24 | 9 |
T3 | 141 | 34 |
T4 | 3 | 5 |
NA | 2 | 149 |
M stage | ||
M0 | 79 | 47 |
M1 | 4 | 3 |
MX | 94 | 149 |
N stage | ||
N0 | 49 | 31 |
N1 | 123 | 19 |
NA | 5 | 149 |
NA, clinical data are unknown; PCA, pancreatic cancer; T, tumor; N, node; M, metastasis; TCGA, The Cancer Genome Atlas; GEO, Gene Expression Omnibus.
Other datasets
The GSE67549 dataset detailed the hypoxic gene expression profile in 9 human PCA cell lines. A total of 9 human PCA cells were cultured under normoxic or hypoxic conditions (1% oxygen) for 24 h. The GSE107300 dataset described the expression profile of MDA-MB-231 human breast cancer cells that had been stably transfected with short hairpin RNA (shRNA) to knockdown BHLHE40. The expression profiles of the control (sh-NC) and knockdown BHLHE40 (sh-BHLHE40) groups were analyzed. All the images of immunohistochemical (IHC) staining came from the Human Protein Atlas (HPA) database (http://www.proteinatlas.org/). The JASPAR database (http://www.jaspar.genereg.net/) was used to predict possible binding sites for BHLHE40 and the promoter of Toll-like receptor 3 (TLR3).
Consensus clustering
Consensus clustering analysis on samples was performed using the K-means algorithm in the “ConsensusClusterPlus” R package (16). The operation was repeated 1,000 times to ensure cluster consistency. The value of cluster number k was set to 2–9. The optimal number of clusters was determined through the cumulative distribution function (CDF) and the Delta plot of relative area change under the CDF curve, to ensure that the CDF distribution is smoother and the area improvement under the CDF curve is relatively greater.
Gene set enrichment analysis (GSEA)
GSEA was performed using GSEA 4.2.3 software (https://www.gsea-msigdb.org/gsea/index.jsp). The c2.cp.kegg.v7.5.1.symbols.gmt or c2.cp.reactome.v7.5.1.symbols.gmt in the Molecular Signature Database (MSigDB; https://www.gsea-msigdb.org/gsea/msigdb/) was used as the reference gene set. According to the gene set, 1,000 permutations were performed to determine P values. Pathways with nominal P value (NOM p-val) <0.05 and false discovery rate q-value (FDR q-val) <0.25 were considered significant. The most significantly enriched signaling pathways were selected based on the normalized enrichment score (NES).
Analysis of differentially expressed HRGs
The HRGs were obtained from the hallmark gene set collection of the MSigDB, which contains 200 genes that are commonly upregulated in human mammalian epithelial cells and a variety of cancers in response to low oxygen levels (17). In order to determine the differentially expressed HRGs between the tumor and normal pancreatic tissue in the training set, the R package “limma” was employed. The differentially expressed HRGs were defined as those with | log fold-change (FC) | >2.0 and FDR <0.05.
Construction of a prognostic model
In the training set, univariate Cox regression analysis was used to assess the relationship between the expression levels of differentially expressed HRGs and the overall survival (OS) of patients. The least absolute shrinkage and selection operator (LASSO) method was used to further screen the genes used to construct the prognostic model and obtain the corresponding regression coefficients. . “Exp” represents the expression level of the gene, and “Coe” represents the regression coefficient of each gene calculated by LASSO regression analysis. The risk score of each patient was calculated according to the prognostic model. Based on the median risk score, patients in TCGA cohort were divided into 2 groups.
Survival analysis
The OS between different groups of patients was compared by KM analysis using the survival and survminer packages in R. To determine whether risk score can be distinguished from other conventional clinical characteristics (age, gender, histological grade, and stage) as an independent prognostic factor in PCA patients, univariate and multivariate Cox regression analyses were performed. A P value <0.05 was considered statistically significant. A nomogram was constructed to better assess the 1-, 3-, and 5-year survival rates of PCA patients.
Analysis of the TME
Based on the RNA-seq data of PCA samples in TCGA, differences in TME between subgroups were assessed. The Estimation of STromal and Immune cells in MAlignant Tumor tissues using Expression data (ESTIMATE) algorithm was adopted to estimate the stromal and immune cells in PCA tissue, and the proportion of immune-stromal components in TME was calculated using the “estimate” R package, which generated ImmuneScore, StromalScore, and EstimateScore. The higher respective score indicated the greater proportion of the corresponding component in TME. Cell-type Identification by Estimating Relative Subsets of RNA Transcripts (CIBERSORT) is a common method for immune cell infiltration estimation and analysis, evaluating the ratio of diverse cell subtypes in mixed cell samples by RNA-seq expression profile (18). CIBERSORT and LM22 signature matrix were used to assess the proportions of 22 immune cell subtypes between different groups. The cancer-immunity cycle describes a cycle of processes involving the eradication of cancer by the immune system, including the following steps: step 1: cancer cell antigen release, step 2: cancer antigen presentation, step 3: priming and activation, step 4: trafficking of T cells to tumors, step 5: infiltration of T cells into tumors, step 6: recognition of cancer cells by T cells, and step 7: killing of cancer cells (19). Based on the single-sample gene set enrichment analysis (ssGSEA) algorithm, we profiled the enrichment scores of steps in the cancer-immunity cycle.
Cell culture and transfection
Three PCA cell lines MIA PaCa-2, SW1990, and PANC-1 cells were obtained from the American Type Culture Collection (ATCC; Manassas, VA, USA) and kept in Dulbecco’s modified Eagle medium (DMEM; Cat: SH30243, HyClone, Logan, UT, USA) supplemented with 10% fetal bovine serum (FBS; Cat: 10100147, Gibco, Billings, MT, USA) and 1% penicillin-streptomycin solution (Cat: C0222, Beyotime, Jiangsu, China). The cells were maintained at 37 ℃ in a humidified environment containing 5% CO2. The cells were passaged every 2–3 days. The small interfering RNAs (siRNAs) targeting BHLHE40 and TLR3 were synthesized by GENEWIZ (Suzhou, Jiangsu, China) and transfected with Lipofectamine 3000 reagent (Cat: 100022052, Invitrogen, Carlsbad, CA, USA) according to the manufacturer’s protocol. The sequences of siRNAs are summarized in Table S1. The knockdown efficiency was evaluated by quantitative real-time polymerase chain reaction (qRT-PCR) 48 h after transfection.
qRT-PCR
TRIzol reagent (Cat: 15596026, Thermo Fisher Scientific, Waltham, MA, USA) was applied to extract total RNA. Subsequently, reverse transcription was performed using the PrimeScript RT Reagent kit (Cat: RR037B, TaKaRa, Shiga, Japan). Aliquots of complementary DNA (cDNA) corresponding to equal amounts of RNA were used for quantification of messenger RNA (mRNA) by RT-PCR using the Light Cycler 96 Real-time Quantitative PCR Detection system (Roche, Indianapolis, IN, USA). The reaction system included the corresponding cDNA, forward primer, reverse primer, and SYBR Green PCR master mix (Cat: 04913914001, Roche). The primer sequences are summarized in Table S2.
Wound healing assay
Wound healing assays were performed to evaluate the migration ability of PANC-1 cells. PANC-1 cells were seeded into 6-well plates and then incubated for 48 h. A 200 µL pipette tip was used to draw a line perpendicular to the bottom of 6-well plate to produce a scratch. The cells then cultured in basal medium. After washing the cells with phosphate-buffered saline (PBS) to remove cellular fragments, each wound was imaged at 0-, 12-, and 24-h by inversion microscopy. Cell migration was quantified by measuring the relative wound areas using ImageJ (National Institutes of Health, Bethesda, MD, USA).
Transwell invasion assay
In order to assess the invasive capacity of the cells, the transwell invasion assay was performed. Matrigel (1:8 diluent of 50 mg/L) was coated on the upper surface of the bottom membrane of the transwell chamber and dried at 37 ℃. The PANC-1 cells with serum-free DMEM were seeded into the upper chamber. The complete medium was added to the lower chamber. After 48 h of incubation, the cells that moved via the Matrigel matrix membrane were fixed in 4% paraformaldehyde and then stained with 0.1% crystal violet. Finally, representative images were obtained under a microscope and the number of cells in the picture can be calculated through ImageJ.
Chromatin immunoprecipitation assay
PANC-1 cells were cross-linked with formaldehyde, lysed in sodium dodecyl sulfate (SDS) buffer, and sheared mechanically by sonication to fragment the DNA. Protein-DNA complexes were precipitated with anti-BHLHE40 antibody (ab70723, Abcam, Cambridge, MA, USA) or anti-rabbit IgG [Cat: 7074, Cell Signalling Technology (CST), Danvers, MA, USA] as a control. DNA eluted from the chromatin immunoprecipitation (ChIP) assay was amplified by qRT-PCR. All fold-enrichment values were normalized according to IgG values. ChIP primer sequences are presented in Table S3.
Statistical analysis
All data were analyzed using R version 4.1.2 (R Foundation for Statistical Computing, Vienna, Austria) or GraphPad Prism 8 (GraphPad Software Inc., San Diego, CA, USA), and all experiments were repeated at least 3 times. These results were presented as mean ± standard deviation (SD).
Research procedures
Based on the expression of HRGs, The PCA samples were clustered into different clusters by consensus clustering. Signaling pathways enriched in different clusters were analyzed using GSEA software. We identified the HRGs differentially expressed in PCA tumor tissue and normal pancreatic tissue. Then univariate Cox regression analysis was used to identify HRGs related to OS. The LASSO regression analysis was used to construct the prognostic model, and the corresponding regression coefficients of the genes involved in the model construction were calculated. The model was validated in the GEO datasets. Univariate and multivariate Cox regression analyses were performed to determine whether the risk score in the prognostic model could be distinguished from other conventional clinical characteristics as an independent prognostic factor. A nomogram was constructed to assess the 1-, 3-, and 5-year survival rates of PCA patients. Subsequently, the ESTIMATE algorithm was adopted to estimate the stromal and immune cells in PCA tissue. CIBERSORT and LM22 signature matrix were used to assess the proportions of 22 immune cell subtypes. Based on the ssGSEA algorithm, we then profiled the enrichment scores of steps in the cancer-immunity cycle. siRNA was transfected into PCA cells to interfere with the expression level of target genes, and the interference efficiency of siRNA was verified by qRT-PCR. A wound healing assay and transwell invasion assay were used to explore the biological functions of target genes in PCA. Finally, we predicted downstream genes of the target genes and explored the biological function.
Results
Subtype identification based on HRGs in PCA
By using the “ConsensusClusterPlus” R package, the PCA samples obtained from TCGA were clustered into different subtypes based on the expression levels of the 200 HRGs, which were downloaded from MSigDB. Figure 1A,1B show the area under the cumulative distribution function (CDF) of the consensus cluster and the relative change of the CDF from k=2 to 9. K=3 was proven to be the most suitable choice to divide the PCA samples into 3 clusters, namely, cluster 1 (n=78), cluster 2 (n=82), and cluster 3 (n=12). The matrix heatmap when k=3 is shown in Figure 1C. KM survival analysis was performed to verify the prognostic value of sample clustering in PCA patients. The results showed that the OS of patients in cluster 1 was significantly shorter than that in cluster 2 and cluster 3 (Figure 1D). Given the small sample size of cluster 3, only cluster 1 and cluster 2 were further analyzed for pathway enrichment using GSEA software. Transforming growth factor-β (TGF-β) receptor signaling in EMT was found to be significantly enriched in cluster 1 (Figure 1E), suggesting hypoxia condition could trigger the EMT and metastasis process in PCA.
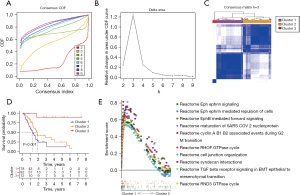
Screening of key HRGs in PCA
Next, we tried to screen key HRGs to construct a prognostic model for PCA. TCGA PCA data and Genotype-Tissue Expression GTEx normal pancreatic tissue data were combined as a training set. According to the cut-off criteria (|log FC| >2.0 and FDR <0.05), 18 HRGs that were differentially expressed in PCA samples and normal samples were identified. In PCA samples, 2 HRGs were lower expressed and 16 HRGs were higher expressed when compared to normal samples (Figure 2A,2B). To further screen out HRGs associated with OS in PCA, univariate Cox regression analysis was performed on these 18 differentially expressed HRGs. The results showed that 5 HRGs (BHLHE40, ENO1, PLAUR, SDC4, and TGM2) were significantly associated with OS in PCA (Figure 2C). These 5 genes were all considered as risk factors for survival of patients, and their high expressions may shorten the survival time of PCA patients [all P<0.05; hazard ratio (HR), 1.002–1.058]. The “corrplot” R package was used to plot the correlation of the expression levels of these 5 HRGs (Figure 2D). Then we performed LASSO regression analysis for further establishment of a hypoxia-related prognostic model of PCA based on gene expression levels (Figure 2E,2F). Finally, LASSO regression analysis established a prognostic model containing 4 HRGs: BHLHE40, ENO1, SDC4, and TGM2.
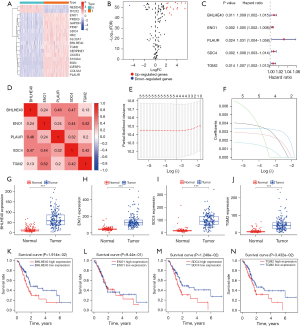
Figure 2G-2J show that the expression levels of BHLHE40, ENO1, SDC4, and TGM2 in PCA tissue samples were higher than those in normal pancreatic samples. Then, we explored their relationship with survival status in PCA. According to the median expression of each gene, PCA patients were divided into 2 groups: the high expression group and the low expression group, and the KM survival curves of the relationship between gene expression and the survival status of PCA patients were drawn respectively (Figure 2K-2N). It was found that patients with higher expressions of BHLHE40 (Figure 2K; P=0.01914), SDC4 (Figure 2M; P=0.01248), and TGM2 (Figure 2N; P=0.03432) had worse prognosis. However, there was no significant difference in prognosis between patients with high and low expression of ENO1 (Figure 2L).
Construction and validation of a hypoxia-related prognostic model
A hypoxia-related prognostic model was constructed to evaluate the prognosis of each patient as follows: Risk score = (0.0018× BHLHE40 expression) + (0.0020× ENO1 expression) + (0.0031× SDC4 expression) + (0.0002× TGM2 expression). The risk score of each patient was calculated according to the prognostic model. Based on the median risk score, 172 PCA patients in TCGA cohort were divided into 2 groups: the high-risk group (n=86) and the low-risk group (n=86). The risk score, survival time, and expression heatmap of the 4 HRGs for each patient are shown in Figure 3A-3C. The KM survival curve indicated that patients in the high-risk group showed markedly poorer OS than those in the low-risk group (Figure 3D). The data from the GSE102238, GSE62452, and GSE85916 datasets were combined as a validation set to validate the hypoxia-related prognostic model. Based on the same median risk score, we divided patients into a high-risk group (n=13) and a low-risk group (n=173). The risk score, survival time, and expression heatmap of 4 HRGs in the validation set are shown in Figure 3E-3G. Consistent with the results derived from the training set, the KM survival curve demonstrated that patients in the high-risk group had lower OS than those in the low-risk group in the validation set (Figure 3H).
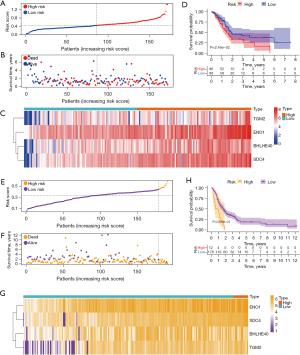
By using univariate Cox regression analysis, the risk score was found to be significantly related to OS (Figure 4A; P<0.05), suggesting that the risk score was an independent prognostic factor for PCA patients. After accounting for other clinical characteristics, multivariate Cox regression analysis revealed that the risk score remained an independent prognostic factor (Figure 4B; P<0.05). A nomogram was constructed to predict 1-, 3-, and 5-year OS rates in PCA patients based on age, gender, grade, stage, and risk score (Figure 4C). Furthermore, the calibration curves of the nomogram were in good agreement with the actual OS rate (Figure 4D-4F).
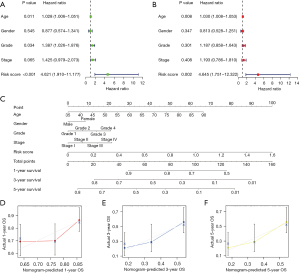
Analysis of the TME in the hypoxia-related prognostic model
Accumulating evidence suggests that tumor hypoxia may protect tumors from natural anti-tumor immune responses by inhibiting anti-tumor immune effector cells and facilitating immune escape (20,21). Here, we investigated the capability of the hypoxia-related prognostic model in evaluating the TME. The ESTIMATE algorithm was used to calculate the ImmuneScore, StromalScore, and EstimateScore for samples in the training set. We found that the ImmuneScore was significantly higher in the low-risk group than in the high-risk group (Figure 5A), indicating that the infiltration of immune cells in the high-risk group was decreased compared to that in the low-risk group. To further analyze the various immune cell subtypes, the CIBERSORT method was introduced to calculate the relative proportions of 22 immune cells in each sample (Figure 5B). Compared to the low-risk group, the high-risk group had higher infiltration levels of M0 macrophages yet lower infiltration levels of naïve B cells, plasma cells, CD8+ T cells, and activated CD4+ memory T cells (Figure 5C). The correlations between the risk score and the above 5 kinds of immune cells were calculated by Spearman correlation analysis (Figure 5D). Infiltration levels of M0 macrophages were positively correlated with the risk score, whereas infiltration levels of naïve B cells, plasma cells, CD8+ T cells, and activated CD4+ memory T cells were negatively correlated with the risk score (Figure 5D). Next, we analyzed the differences between the groups involved in the cancer-immunity cycle. We found that the high-risk group had a lower score in the following steps: CD4+ T cell recruiting, dendritic cell (DC) recruiting, macrophage recruiting, Th17 cell recruiting, and killing of cancer cells (Figure 5E), suggesting that hypoxia hindered these steps in the cancer-immunity cycle.
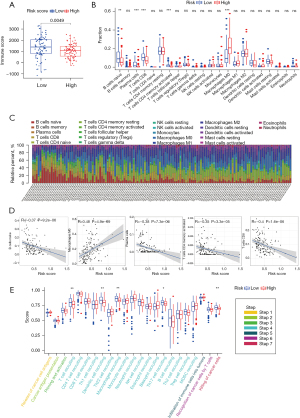
The clinical significance and biological function of BHLHE40 in PCA
In the GSE67549 dataset, 9 PCA cells were cultured under normoxic or hypoxic (1% oxygen) conditions for 24 h. Through a paired t-test, it was found that the expressions of BHLHE40, ENO1, SDC4, and TGM2 were up-regulated under hypoxic conditions, compared with those under normoxic conditions (Figure 6A). Since the FC of expression of BHLHE40 was the highest (Figure 6A), we chose BHLHE40 as a candidate target gene for further investigation. To determine the protein expression level of BHLHE40, IHC staining images of PCA tissue and normal pancreatic tissue were obtained from the Human Protein Atlas (HPA) database (http://www.proteinatlas.org/). The results showed that the protein expression of BHLHE40 in PCA tissue was higher than that in normal tissue (Figure 6B). Based on PCA data in TCGA cohort, we found that there were significant differences in the expression of BHLHE40 in patients with different grades, stages, tumor (T) stages, and metastasis (M) stages (Figure 6C-6F).
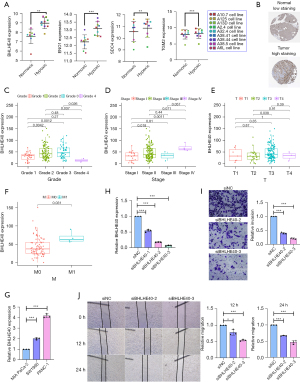
According to previous reports, tumor cells are more likely to invade and migrate under hypoxic conditions (9,10). To explore whether BHLHE40 could participate in the invasion and migration of PCA, we used siRNAs targeting BHLHE40 to investigate whether knockdown of BHLHE40 could affect the metastasis of PCA cells. Firstly, qRT-PCR was used to detect the expression of BHLHE40 in MIA PaCa-2, SW1990, and PANC-1 PCA cell lines. We found that PANC-1 cells expressed the highest level of BHLHE40 among these 3 cell lines (Figure 6G). Then, the PANC-1 cell line was applied in subsequent experiments. Then, 3 siRNAs targeting BHLHE40 were prepared, and their efficiency of knockdown was confirmed by qRT-PCR (Figure 6H). Among them, siBHLHE40-2 and siBHLHE40-3 presented better interference effects. Interfering of BHLHE40 significantly repressed the invasion of PANC-1 cells (Figure 6I). Consistent with the trend of invasion tests, the migration ability of PANC-1 cells was also significantly reduced by knockdown of BHLHE40 (Figure 6J). Therefore, we found that BHLHE40 participated in the metastasis of PCA cells.
TLR3 serves as a downstream target gene of BHLHE40
To explore the downstream target genes of BHLHE40, we performed differential analysis on the transcriptional data of the sh-NC group and the sh-BHLHE40 group in the GSE107300 dataset. Based on the criteria of |log2 FC| >2 and FDR <0.05, 77 differentially expressed genes (DEGs) in MDA-MB-231 cells were screened out: 29 genes were up-regulated and 48 genes were down-regulated upon knockdown of BHLHE40 (Figure 7A). Spearman correlation analysis was performed to further explore which of these DEGs were co-expressed with BHLHE40 in TCGA PCA cohort. The results showed 12 out of 48 down-regulated genes and 3 out of 29 up-regulated genes were positively and negatively correlated with BHLHE40, respectively, in TCGA PCA cohort (Figure S1). The KM survival analysis was then performed on the above 15 genes co-expressed with BHLHE40 to evaluate their relationship with prognosis (Figure S2). Finally, we identified 4 genes, CYP24A1, GCNT4, TLR3, and TMEM139, that were co-expressed with BHLHE40 (Figure 7B-7E) and related to prognosis (Figure 7F-7I). To explore the potential functions of these 4 genes, samples from TCGA PCA cohort were divided into high and low expression groups based on the median expression of each gene and the GSEA enrichment analysis was performed. No pathways were found to be significantly enriched in the TMEM139 high expression group. The significantly enriched pathways in the CYP24A1 highly expressed group were mainly pathways involved in cancer (Figure 7J). The pathways that were significantly enriched in the GCNT4 highly expressed group were mainly metabolism-related pathways (Figure 7K). Apoptosis, toll-like receptor, tight junction, and metabolism pathways were significantly enriched in the TLR3 highly expressed group (Figure 7L).
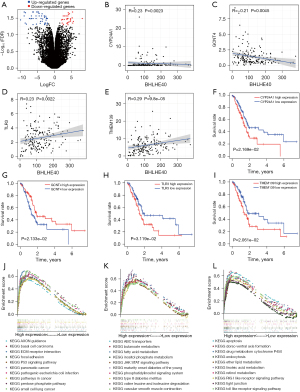
The BHLHE40/TLR3 axis promoted cell invasion and migration in PCA
Previous study has verified the capability of transcription regulating of BHLHE40 (22,23). In order to explore whether there were binding sites of BHLHE40 in the promoters of CYP24A1, GCNT4, TLR3, and TMEM139, the JASPAR database was used to analyze the sequences of these promoters (Figure S3). According to the criterion that the relative profile parameter threshold >80% and predicting score >5, no predicted binding sites were obtained in the promoter of CYP24A1 or TMEM139. Multiple predicted binding sites were identified in the promoter of GCNT4, and 2 binding sites were predicted in the promoter of TLR3, namely S1 (score =5.89 and 4.45) and S2 (score =4.99 and 6.76) (Figure 8A). Since pathways significantly enriched in the TLR3 highly expressed group participate in the metastasis of cancer (as shown in Figure 7L), we speculated that TLR3 might be responsible for BHLHE40-mediated invasion and migration. Interference against BHLHE40 down-regulated TLR3 mRNA expression (Figure 8B). To confirm the binding of BHLHE40 to the promoter of TLR3, we performed ChIP assay using PCR primers targeting S1 and S2 regions of the TLR3 promoter. As shown in Figure 8C, interfering with BHLHE40 could weaken the binding of BHLHE40 to the S1 and S2 sites of the TLR3 promoter.
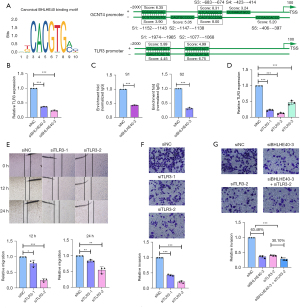
To further explore the biological function of TLR3, 3 siRNAs targeting TLR3 (siTLR3) were prepared and their efficiency was confirmed by qRT-PCR (Figure 8D). The siTLR3-1 and siTLR3-2 were chosen for subsequent experiments. TLR3 silencing by siRNAs suppressed the migration (Figure 8E) and invasion of PANC-1 cells (Figure 8F). Then, we co-transfected siBHLHE40 and siTLR3 into PANC-1 cells, and found that siTLR3 significantly reduced the inhibitory effect of siBHLHE40 on cell invasion (Figure 8G), suggesting that BHLHE40 promoted PCA metastasis through TLR3 dependent mechanism.
Discussion
PCA is one of the deadliest diseases worldwide due to its typically late diagnosis, early metastasis, and resistance to current treatment strategies, including chemotherapy and radiotherapy. PCA is highly hypoxic compared to other solid tumors (24,25). The formation of a hypoxic microenvironment in PCA is mainly due to its rich extracellular matrix, rapid proliferation of PCA cells, and poor vascularization, which leads to increased oxygen consumption and insufficient oxygen supply (26,27). Numerous studies have demonstrated that hypoxia plays an important role in the progression of cancer and the development of malignant phenotypes (28,29). Hypoxia can promote tumor cell metastasis, inhibit anti-tumor immune response, and promote dysfunctional angiogenesis (30-32). Although hypoxia is not associated with traditional clinical prognostic factors, such as tumor stage and tumor grade, it has been demonstrated as a significant unfavorable prognostic factor of tumor patients (33). In the present study, we found that hypoxia has a significant impact on the prognosis of PCA patients. Several methods have been developed to evaluate the degree of hypoxia in tumors, such as oxygen pressure measurement, blood flow velocity methods [such as blood oxygen level dependent magnetic resonance imaging (BLOD-MRI)], and the detection of hypoxia markers (such as HIF, nitroreductase) (34-36). We constructed and validated a prognostic model including 4 HRGs (BHLHE40, ENO1, SDC4, and TGM2) by LASSO regression analysis to evaluate the prognosis of PCA patients. This model has good prognostic predictive value. Furthermore, the nomogram we created could accurately predict the OS of individual PCA patients. These findings provide new insights into predicting the prognosis of PCA patients. In addition, by comparing the expression levels of 4 HRGs in the model under normoxic and hypoxic conditions, we found that they were all upregulated under hypoxic conditions. This means that changes in their expression levels to some extent can reflect tumor hypoxia. However, further confirmation is needed to determine whether these genes and the risk score of the model are related to the degree of tumor hypoxia.
Growing evidence suggests that hypoxia can drive tumor immunosuppression and immune escape (20,21). In a mouse model, HIF-1α knockout in the pancreas has been shown to significantly increase infiltration levels of B cells in the PCA TME, leading to increased levels of B cell attractant chemokines such as CXCL13 (37). This result shows that hypoxia leads to reduced B cell infiltration levels (37). Hypoxia-induced reactive oxygen species (ROS) preserves immunosuppressive and potentially fatal effects on T cells (38). Superoxide is created in the mitochondria by STAT3 and NADPH activation, which subsequently activates the caspase cascade and induces T cell death (39). Macrophages are a primary component of the innate immune response (40). M2 macrophages are located in more hypoxic areas of PCA, whereas M1 macrophages tend to be in normoxic areas farther away from cancer cells (41,42). In our present study, we found that the high-risk group had a lower ImmuneScore than the low-risk group in the hypoxia-related prognostic model. The infiltration levels of naïve B cells, plasma cells, CD8+ T cells, and activated CD4+ memory T cells in the high-risk group were lower than those in the low-risk group. Comparison of the cancer-immunity cycle score between groups revealed that the high-risk group had a lower score in the following steps, such as CD4+ T cell recruiting, DC recruiting, macrophage recruiting, Th17 cell recruiting, and killing of cancer cells. These results suggested that hypoxia in PCA affected the infiltration levels of immune cells in the TME, driving immune suppression and immune escape.
The 4 HRGs, BHLHE40, ENO1, SDC4, and TGM2, involved in the prognostic model, were all up-regulated under hypoxic conditions according to GSE67549 dataset, and the increased FC of BHLHE40 was the most significant among these 4 HRGs. BHLHE40, also known as DEC1/Stra13/Sharp2, is a basic helix-loop-helix (bHLH) transcription factor involved in circadian rhythm, cell proliferation, apoptosis, hypoxia response, various stresses, and EMT of tumor cells (22,23,43-45). Compared with adjacent normal tissue, BHLHE40 is highly expressed in many tumors, such as colon cancer (46), liver cancer (47), and brain tumors (48). By using bioinformatics analysis, we found that BHLHE40 was also highly expressed in PCA compared with normal pancreatic tissue. Furthermore, PCA patients with higher BHLHE40 expression level had a less favorable prognosis. To date, there have been few studies on BHLHE40 in tumor invasion or metastasis, and the results are inconsistent (49-51). According to previous studies, BHLHE40 mediates transcriptional repression of CLDN1 and promotes cell invasion by interacting with SP1 in breast cancer cells (49). BHLHE40 also promotes EMT in PCA cells by activating Smad3 phosphorylation (50). However, in endometrial adenocarcinoma, BHLHE40 inhibits the transcription of Twist by competing for the binding site with SP1, thereby inhibiting EMT (51). Our study verified the role of BHLHE40 in PCA cell invasion and migration in vitro through regulation on downstream TLR3. Besides BHLHE40, the other 3 HRGs, ENO1, SDC4, and TGM2, were found to be involved in the progression of cancers as well. Enolase 1 (ENO1), also known as pyruvate dehydrogenase 1, is a key regulatory enzyme of glycolysis (52). Consistent with the results of our bioinformatics analysis, ENO1 has been found to be highly expressed in PCA tissue compared to normal tissue, and is involved in PCA tumorigenesis, invasion, and metastasis (53-55). Studies have reported that high ENO1 expression predicted a poor prognosis in PCA (56,57). In our bioinformatics analysis, we found that ENO1 overexpression is an adverse factor affecting OS in PCA patients. It has been reported that ENO1 silencing impairs hypoxia-induced gemcitabine chemoresistance in PCA cells by modulation of redox homeostasis (57). FAM126A interacted with ENO1 mediates proliferation and metastasis in PCA via PI3K/AKT signaling pathway (58). Syndecan-4 (SDC4), as an important member of the SDC family, is a ubiquitously expressed transmembrane proteoglycan with heparan sulfate chains (59). SDC4 promotes tumor development and progression by affecting cell proliferation, invasion, and migration (60-63). Knockout of SDC4 in PCA cells significantly impairs macropinocytosis, colony formation, and xenograft tumor growth (64). Recent studies have shown that the expression level of SDC4 is significantly related to OS in patients with pancreatic cancer and affects the prognosis of patients, which is consistent with our results (65). Transglutaminase 2 (TGM2) is a member of the transglutaminase enzyme family (66). As a calcium-dependent cross-linking enzyme, TGM2 catalyzes protein modifications via transamidation. TGM2 is involved in multiple biological functions, including cell differentiation, extracellular matrix stabilization, and cell migration (67). TGM2 expression is negatively correlated with the prognosis of pancreatic cancer patients (68). Recent studies have confirmed that the promotion of EMT in hepatocellular carcinoma cells by activated hepatic stellate cells is mediated by pseudohypoxia induced via the TGM2/VHL/HIF-1a pathway (69). TGM2 also induces EMT in colon, breast, and gastric cancer cells, potentially leading to the acquisition of drug resistance (70-72).
In this study, we identified DEGs upon knockdown of BHLHE40 in the GSE107300 dataset. These genes were further screened in TCGA PCA cohort to identify those that were co-expressed with BHLHE40 and related to prognosis. Finally, we screened out CYP24A1, GCNT4, TLR3, and TMEM139 could be the candidate downstream targets of BHLHE40. Then, we predicted the potential functions of these 4 genes by using GSEA, and explored the interaction between BHLHE40 and the promoters of these 4 genes using the JASPAR database. Since the potential functions of TLR3 were involved in metastasis and 2 binding sites of BHLHE40 were identified in the promoter of TLR3, we proposed that TLR3 might function as a direct target of BHLHE40 mediating metastasis of PCA cells. TLR3 is an important member of the TLR family, a group of pattern recognition receptors that mediate immune responses (73). TLR3 is mostly found to be expressed in immune cells, such as macrophages, DC, and natural killer (NK) cells (74). It plays a role in activating innate immunity, resulting in the production of cytokines, chemokines, and adhesion molecules that cause inflammation (74). There is a growing body of evidence suggesting that TLR3 is also expressed in tumor cells and has dual roles in tumor progression. Several studies have shown that TLR3 promotes tumor cell apoptosis in breast (75), prostate (76), and liver (77) cancers. In addition, some studies have indicated that TLR3 can promote tumor progression (78-80). It has been reported that TLR3 is associated with more aggressive tumor behavior in head and neck cancer (81). TLR3 expressed in the host lung epithelial cell has been shown to promote lung pre-metastatic niche formation via tumor exosome-mediated neutrophil recruitment (82). In this study, we found that TLR3 is a downstream gene of BHLHE40, and that it participated in the invasion and migration of PCA cells.
This study has various limitations and needs further optimization. Although the prediction model we established has been verified with retrospective data in the public database, more prospective data are needed to verify its clinical applicability. Besides TLR3, the other 3 potential genes, including CYP24A1, GCNT4, and TMEM139, at the downstream of BHLHE40 could also be involved in the progression of PCA. Although the regulation of CYP24A1, GCNT4, or TMEM139 by BHLHE40 was not investigated in the present study, further study is still necessary to reveal the role of CYP24A1, GCNT4, and TMEM139 in the progression of PCA under hypoxic conditions. Moreover, the cell signaling transduction between BHLHE40 and downstream genes still needs further exploration.
Conclusions
In conclusion, the present study constructed and validated a prognostic model based on 4 HRGs, BHLHE40, ENO1, SDC4, and TGM2. The hypoxia-related prognostic model can also assess TME in PCA patients. Moreover, we found that the BHLHE40/TLR3 axis promoted invasion and migration of PCA cells, revealing a mechanism involved in the unfavorable prognosis of PCA. This study may provide new insights into how hypoxia affects the prognosis and TME of PCA and may benefit hypoxia-targeted therapies for this aggressive tumor in the future.
Acknowledgments
Funding: This work was supported by the National Natural Science Foundation of China (Nos. 81873587 and 81874454).
Footnote
Reporting Checklist: The authors have completed the TRIPOD reporting checklist. Available at https://jgo.amegroups.com/article/view/10.21037/jgo-23-301/rc
Data Sharing Statement: Available at https://jgo.amegroups.com/article/view/10.21037/jgo-23-301/dss
Peer Review File: Available at https://jgo.amegroups.com/article/view/10.21037/jgo-23-301/prf
Conflicts of Interest: All authors have completed the ICMJE uniform disclosure form (available at https://jgo.amegroups.com/article/view/10.21037/jgo-23-301/coif). The authors have no conflicts of interest to declare.
Ethical Statement: The authors are accountable for all aspects of the work in ensuring that questions related to the accuracy or integrity of any part of the work are appropriately investigated and resolved. The study was conducted in accordance with the Declaration of Helsinki (as revised in 2013).
Open Access Statement: This is an Open Access article distributed in accordance with the Creative Commons Attribution-NonCommercial-NoDerivs 4.0 International License (CC BY-NC-ND 4.0), which permits the non-commercial replication and distribution of the article with the strict proviso that no changes or edits are made and the original work is properly cited (including links to both the formal publication through the relevant DOI and the license). See: https://creativecommons.org/licenses/by-nc-nd/4.0/.
References
- Rahib L, Smith BD, Aizenberg R, et al. Projecting cancer incidence and deaths to 2030: the unexpected burden of thyroid, liver, and pancreas cancers in the United States. Cancer Res 2014;74:2913-21. [Crossref] [PubMed]
- Siegel RL, Miller KD, Fuchs HE, et al. Cancer Statistics, 2021. CA Cancer J Clin 2021;71:7-33. [Crossref] [PubMed]
- Koong AC, Mehta VK, Le QT, et al. Pancreatic tumors show high levels of hypoxia. Int J Radiat Oncol Biol Phys 2000;48:919-22. [Crossref] [PubMed]
- Zhou J, Schmid T, Schnitzer S, et al. Tumor hypoxia and cancer progression. Cancer Lett 2006;237:10-21. [Crossref] [PubMed]
- Taddei ML, Giannoni E, Comito G, et al. Microenvironment and tumor cell plasticity: an easy way out. Cancer Lett 2013;341:80-96. [Crossref] [PubMed]
- Lu Y, Hu J, Sun W, et al. Hypoxia-mediated immune evasion of pancreatic carcinoma cells. Mol Med Rep 2015;11:3666-72. [Crossref] [PubMed]
- Daniel SK, Sullivan KM, Labadie KP, et al. Hypoxia as a barrier to immunotherapy in pancreatic adenocarcinoma. Clin Transl Med 2019;8:10. [Crossref] [PubMed]
- Majmundar AJ, Wong WJ, Simon MC. Hypoxia-inducible factors and the response to hypoxic stress. Mol Cell 2010;40:294-309. [Crossref] [PubMed]
- Kitajima Y, Ide T, Ohtsuka T, et al. Induction of hepatocyte growth factor activator gene expression under hypoxia activates the hepatocyte growth factor/c-Met system via hypoxia inducible factor-1 in pancreatic cancer. Cancer Sci 2008;99:1341-7. [Crossref] [PubMed]
- Azab AK, Hu J, Quang P, et al. Hypoxia promotes dissemination of multiple myeloma through acquisition of epithelial to mesenchymal transition-like features. Blood 2012;119:5782-94. [Crossref] [PubMed]
- Zhou G, Liu X, Wang X, et al. Combination of preoperative CEA and CA19-9 improves prediction outcomes in patients with resectable pancreatic adenocarcinoma: results from a large follow-up cohort. Onco Targets Ther 2017;10:1199-206. [Crossref] [PubMed]
- Distler M, Pilarsky E, Kersting S, et al. Preoperative CEA and CA 19-9 are prognostic markers for survival after curative resection for ductal adenocarcinoma of the pancreas - a retrospective tumor marker prognostic study. Int J Surg 2013;11:1067-72. [Crossref] [PubMed]
- Cai C, Yang L, Zhou K. 8DEstablishment and validation of a hypoxia-related signature predicting prognosis in hepatocellular carcinoma. BMC Gastroenterol 2021;21:463. [Crossref] [PubMed]
- Lin W, Wu S, Chen X, et al. Characterization of Hypoxia Signature to Evaluate the Tumor Immune Microenvironment and Predict Prognosis in Glioma Groups. Front Oncol 2020;10:796. [Crossref] [PubMed]
- Yang X, Weng X, Yang Y, et al. A combined hypoxia and immune gene signature for predicting survival and risk stratification in triple-negative breast cancer. Aging (Albany NY) 2021;13:19486-509. [Crossref] [PubMed]
- Wilkerson MD, Hayes DN. ConsensusClusterPlus: a class discovery tool with confidence assessments and item tracking. Bioinformatics 2010;26:1572-3. [Crossref] [PubMed]
- Liberzon A, Birger C, Thorvaldsdottir H, et al. The Molecular Signatures Database (MSigDB) hallmark gene set collection. Cell Syst 2015;1:417-25. [Crossref] [PubMed]
- Newman AM, Liu CL, Green MR, et al. Robust enumeration of cell subsets from tissue expression profiles. Nat Methods 2015;12:453-7. [Crossref] [PubMed]
- Chen DS, Mellman I. Oncology meets immunology: the cancer-immunity cycle. Immunity 2013;39:1-10. [Crossref] [PubMed]
- Lee CT, Mace T, Repasky EA. Hypoxia-driven immunosuppression: a new reason to use thermal therapy in the treatment of cancer? Int J Hyperthermia 2010;26:232-46. [Crossref] [PubMed]
- Castillo-Rodriguez RA, Trejo-Solis C, Cabrera-Cano A, et al. Hypoxia as a Modulator of Inflammation and Immune Response in Cancer. Cancers (Basel) 2022;14:2291. [Crossref] [PubMed]
- Sato F, Bhawal UK, Yoshimura T, et al. DEC1 and DEC2 Crosstalk between Circadian Rhythm and Tumor Progression. J Cancer 2016;7:153-9. [Crossref] [PubMed]
- Kato Y, Kawamoto T, Fujimoto K, et al. DEC1/STRA13/SHARP2 and DEC2/SHARP1 coordinate physiological processes, including circadian rhythms in response to environmental stimuli. Curr Top Dev Biol 2014;110:339-72. [Crossref] [PubMed]
- Vaupel P, Hockel M, Mayer A. Detection and characterization of tumor hypoxia using pO2 histography. Antioxid Redox Signal 2007;9:1221-35. [Crossref] [PubMed]
- Tan Z, Xu J, Zhang B, et al. Hypoxia: a barricade to conquer the pancreatic cancer. Cell Mol Life Sci 2020;77:3077-83. [Crossref] [PubMed]
- Hu Q, Qin Y, Ji S, et al. UHRF1 promotes aerobic glycolysis and proliferation via suppression of SIRT4 in pancreatic cancer. Cancer Lett 2019;452:226-36. [Crossref] [PubMed]
- Erkan M, Hausmann S, Michalski CW, et al. The role of stroma in pancreatic cancer: diagnostic and therapeutic implications. Nat Rev Gastroenterol Hepatol 2012;9:454-67. [Crossref] [PubMed]
- Muz B, de la Puente P, Azab F, et al. The role of hypoxia in cancer progression, angiogenesis, metastasis, and resistance to therapy. Hypoxia (Auckl) 2015;3:83-92. [Crossref] [PubMed]
- Hamada S, Matsumoto R, Masamune A. HIF-1 and NRF2; Key Molecules for Malignant Phenotypes of Pancreatic Cancer. Cancers (Basel) 2022;14:411. [Crossref] [PubMed]
- Zhao X, Gao S, Ren H, et al. Hypoxia-inducible factor-1 promotes pancreatic ductal adenocarcinoma invasion and metastasis by activating transcription of the actin-bundling protein fascin. Cancer Res 2014;74:2455-64. [Crossref] [PubMed]
- Li Y, Patel SP, Roszik J, et al. Hypoxia-Driven Immunosuppressive Metabolites in the Tumor Microenvironment: New Approaches for Combinational Immunotherapy. Front Immunol 2018;9:1591. [Crossref] [PubMed]
- Ravi R, Mookerjee B, Bhujwalla ZM, et al. Regulation of tumor angiogenesis by p53-induced degradation of hypoxia-inducible factor 1alpha. Genes Dev 2000;14:34-44. [Crossref] [PubMed]
- Jubb AM, Buffa FM, Harris AL. Assessment of tumour hypoxia for prediction of response to therapy and cancer prognosis. J Cell Mol Med 2010;14:18-29. [Crossref] [PubMed]
- Brizel DM, Scully SP, Harrelson JM, et al. Tumor oxygenation predicts for the likelihood of distant metastases in human soft tissue sarcoma. Cancer Res 1996;56:941-3. [PubMed]
- Graeber TG, Osmanian C, Jacks T, et al. Hypoxia-mediated selection of cells with diminished apoptotic potential in solid tumours. Nature 1996;379:88-91. [Crossref] [PubMed]
- Li Y, Sun Y, Li J, et al. Ultrasensitive near-infrared fluorescence-enhanced probe for in vivo nitroreductase imaging. J Am Chem Soc 2015;137:6407-16. [Crossref] [PubMed]
- Lee KE, Spata M, Bayne LJ, et al. Hif1a Deletion Reveals Pro-Neoplastic Function of B Cells in Pancreatic Neoplasia. Cancer Discov 2016;6:256-69. [Crossref] [PubMed]
- Peng HY, Lucavs J, Ballard D, et al. Metabolic Reprogramming and Reactive Oxygen Species in T Cell Immunity. Front Immunol 2021;12:652687. [Crossref] [PubMed]
- Hildeman DA, Mitchell T, Teague TK, et al. Reactive oxygen species regulate activation-induced T cell apoptosis. Immunity 1999;10:735-44. [Crossref] [PubMed]
- Woo SR, Corrales L, Gajewski TF. Innate immune recognition of cancer. Annu Rev Immunol 2015;33:445-74. [Crossref] [PubMed]
- Ino Y, Yamazaki-Itoh R, Shimada K, et al. Immune cell infiltration as an indicator of the immune microenvironment of pancreatic cancer. Br J Cancer 2013;108:914-23. [Crossref] [PubMed]
- Henze AT, Mazzone M. The impact of hypoxia on tumor-associated macrophages. J Clin Invest 2016;126:3672-9. [Crossref] [PubMed]
- Jia Y, Liu Y, Zhu J, et al. DEC1 promotes progression of Helicobacter pylori-positive gastric cancer by regulating Akt/NF-kappaB pathway. J Cell Mol Med 2022;26:1943-54. [Crossref] [PubMed]
- Shan E, Huo Y, Wang H, et al. Differentiated embryonic chondrocyte expressed gene-1 (DEC1) enhances the development of colorectal cancer with an involvement of the STAT3 signaling. Neoplasia 2022;27:100783. [Crossref] [PubMed]
- Yang L, Zeng L, Wang Z, et al. Differentiated embryo chondrocyte 1, induced by hypoxia-inducible factor 1alpha, promotes cell migration in oral squamous cell carcinoma cell lines. Oral Surg Oral Med Oral Pathol Oral Radiol 2022;133:199-206. [Crossref] [PubMed]
- Li Y, Zhang H, Xie M, et al. Abundant expression of Dec1/stra13/sharp2 in colon carcinoma: its antagonizing role in serum deprivation-induced apoptosis and selective inhibition of procaspase activation. Biochem J 2002;367:413-22. [Crossref] [PubMed]
- Shi XH, Zheng Y, Sun Q, et al. DEC1 nuclear expression: a marker of differentiation grade in hepatocellular carcinoma. World J Gastroenterol 2011;17:2037-43. [Crossref] [PubMed]
- Preusser M, Birner P, Ambros IM, et al. DEC1 expression in 1p-aberrant oligodendroglial neoplasms. Histol Histopathol 2005;20:1173-7. [PubMed]
- Zheng Q, Wang C, Wang L, et al. Interaction with SP1, but not binding to the E-box motifs, is responsible for BHLHE40/DEC1-induced transcriptional suppression of CLDN1 and cell invasion in MCF-7 cells. Mol Carcinog 2018;57:1116-29. [Crossref] [PubMed]
- Wu Y, Sato F, Yamada T, et al. The BHLH transcription factor DEC1 plays an important role in the epithelial-mesenchymal transition of pancreatic cancer. Int J Oncol 2012;41:1337-46. [Crossref] [PubMed]
- Asanoma K, Liu G, Yamane T, et al. Regulation of the Mechanism of TWIST1 Transcription by BHLHE40 and BHLHE41 in Cancer Cells. Mol Cell Biol 2015;35:4096-109. [Crossref] [PubMed]
- Kang HJ, Jung SK, Kim SJ, et al. Structure of human alpha-enolase (hENO1), a multifunctional glycolytic enzyme. Acta Crystallogr D Biol Crystallogr 2008;64:651-7. [Crossref] [PubMed]
- Cappello P, Tomaino B, Chiarle R, et al. An integrated humoral and cellular response is elicited in pancreatic cancer by alpha-enolase, a novel pancreatic ductal adenocarcinoma-associated antigen. Int J Cancer 2009;125:639-48. [Crossref] [PubMed]
- Capello M, Ferri-Borgogno S, Cappello P, et al. alpha-Enolase: a promising therapeutic and diagnostic tumor target. FEBS J 2011;278:1064-74. [Crossref] [PubMed]
- Wang L, Liu HL, Li Y, et al. Proteomic analysis of pancreatic intraepithelial neoplasia and pancreatic carcinoma in rat models. World J Gastroenterol 2011;17:1434-41. [Crossref] [PubMed]
- Yin H, Wang L, Liu HL. ENO1 Overexpression in Pancreatic Cancer Patients and Its Clinical and Diagnostic Significance. Gastroenterol Res Pract 2018;2018:3842198. [Crossref] [PubMed]
- Wang L, Bi R, Yin H, et al. ENO1 silencing impaires hypoxia-induced gemcitabine chemoresistance associated with redox modulation in pancreatic cancer cells. Am J Transl Res 2019;11:4470-80. [PubMed]
- Li Y, Li Y, Luo J, et al. FAM126A interacted with ENO1 mediates proliferation and metastasis in pancreatic cancer via PI3K/AKT signaling pathway. Cell Death Discov 2022;8:248. [Crossref] [PubMed]
- Tumova S, Woods A, Couchman JR. Heparan sulfate chains from glypican and syndecans bind the Hep II domain of fibronectin similarly despite minor structural differences. J Biol Chem 2000;275:9410-7. [Crossref] [PubMed]
- Keller-Pinter A, Gyulai-Nagy S, Becsky D, et al. Syndecan-4 in Tumor Cell Motility. Cancers (Basel) 2021;13:3322. [Crossref] [PubMed]
- Jechorek D, Haeusler-Pliske I, Meyer F, et al. Diagnostic value of syndecan-4 protein expression in colorectal cancer. Pathol Res Pract 2021;222:153431. [Crossref] [PubMed]
- Onyeisi JOS, Lopes CC, Gotte M. Syndecan-4 as a Pathogenesis Factor and Therapeutic Target in Cancer. Biomolecules 2021;11:503. [Crossref] [PubMed]
- Yang H, Liu Y, Zhao MM, et al. Therapeutic potential of targeting membrane-spanning proteoglycan SDC4 in hepatocellular carcinoma. Cell Death Dis 2021;12:492. [Crossref] [PubMed]
- Cui C, Pan Y, Zhang C, et al. Eltrombopag binds SDC4 directly and enhances MAPK signaling and macropinocytosis in cancer cells. Am J Cancer Res 2022;12:2697-710. [PubMed]
- Zhu Y, Zheng D, Lei L, et al. High expression of syndecan-4 is related to clinicopathological features and poor prognosis of pancreatic adenocarcinoma. BMC Cancer 2022;22:1042. [Crossref] [PubMed]
- Lorand L, Graham RM. Transglutaminases: crosslinking enzymes with pleiotropic functions. Nat Rev Mol Cell Biol 2003;4:140-56. [Crossref] [PubMed]
- Eckert RL, Kaartinen MT, Nurminskaya M, et al. Transglutaminase regulation of cell function. Physiol Rev 2014;94:383-417. [Crossref] [PubMed]
- Wang F, Wang L, Qu C, et al. Kaempferol induces ROS-dependent apoptosis in pancreatic cancer cells via TGM2-mediated Akt/mTOR signaling. BMC Cancer 2021;21:396. [Crossref] [PubMed]
- Ma H, Xie L, Zhang L, et al. Activated hepatic stellate cells promote epithelial-to-mesenchymal transition in hepatocellular carcinoma through transglutaminase 2-induced pseudohypoxia. Commun Biol 2018;1:168. [Crossref] [PubMed]
- Ayinde O, Wang Z, Griffin M. Tissue transglutaminase induces Epithelial-Mesenchymal-Transition and the acquisition of stem cell like characteristics in colorectal cancer cells. Oncotarget 2017;8:20025-41. [Crossref] [PubMed]
- Agnihotri N, Kumar S, Mehta K. Tissue transglutaminase as a central mediator in inflammation-induced progression of breast cancer. Breast Cancer Res 2013;15:202. [Crossref] [PubMed]
- Wang X, Yu Z, Zhou Q, et al. Tissue transglutaminase-2 promotes gastric cancer progression via the ERK1/2 pathway. Oncotarget 2016;7:7066-79. [Crossref] [PubMed]
- Takeuchi O, Akira S. Pattern recognition receptors and inflammation. Cell 2010;140:805-20. [Crossref] [PubMed]
- Iwasaki A, Medzhitov R. Toll-like receptor control of the adaptive immune responses. Nat Immunol 2004;5:987-95. [Crossref] [PubMed]
- Salaun B, Coste I, Rissoan MC, et al. TLR3 can directly trigger apoptosis in human cancer cells. J Immunol 2006;176:4894-901. [Crossref] [PubMed]
- Paone A, Starace D, Galli R, et al. Toll-like receptor 3 triggers apoptosis of human prostate cancer cells through a PKC-alpha-dependent mechanism. Carcinogenesis 2008;29:1334-42. [Crossref] [PubMed]
- Guo Z, Chen L, Zhu Y, et al. Double-stranded RNA-induced TLR3 activation inhibits angiogenesis and triggers apoptosis of human hepatocellular carcinoma cells. Oncol Rep 2012;27:396-402. [PubMed]
- Liu HN, Shi HR, Zhao XL, et al. The TLR3, PI3K, survivin, FasL, and Fas genes as major risk factors of occurrence and development of cervical cancer disease. Gene 2014;550:27-32. [Crossref] [PubMed]
- Gonzalez-Reyes S, Marin L, Gonzalez L, et al. Study of TLR3, TLR4 and TLR9 in breast carcinomas and their association with metastasis. BMC Cancer 2010;10:665. [Crossref] [PubMed]
- Gonzalez-Reyes S, Fernandez JM, Gonzalez LO, et al. Study of TLR3, TLR4, and TLR9 in prostate carcinomas and their association with biochemical recurrence. Cancer Immunol Immunother 2011;60:217-26. [Crossref] [PubMed]
- Chuang HC, Huang CC, Chien CY, et al. Toll-like receptor 3-mediated tumor invasion in head and neck cancer. Oral Oncol 2012;48:226-32. [Crossref] [PubMed]
- Liu Y, Gu Y, Han Y, et al. Tumor Exosomal RNAs Promote Lung Pre-metastatic Niche Formation by Activating Alveolar Epithelial TLR3 to Recruit Neutrophils. Cancer Cell 2016;30:243-56. [Crossref] [PubMed]