Prognostic evaluation of pancreatic cancer based on the model of chemo-radiotherapy resistance-related genes
Highlight box
Key findings
• We established a prognostic signature for pancreatic cancer comprising SNAP25, GPR87, DLL1, LAD1, WASF3, ARHGAP29, ZBED2, GAD1, and JAG1, which can effectively predict the prognosis of patients. In vitro and in vivo experiments showed that JAG1 silencing can inhibit the chemoradiotherapy tolerance of pancreatic cancer cells, highlighting the potential role of JAG1 in anti-pancreatic cancer therapy.
What is known and what is new?
• Pancreatic cancer is an aggressive malignancy with a characteristic metastatic course and resistance to chemo-radiotherapy.
• We established a prognostic signature for chemo-radiotherapy resistance-related genes in patients with pancreatic cancer. We also explored the effect of candidate target genes in the malignant progression of pancreatic cancer.
What is the implication, and what should change now?
• Exploring the role of chemo-radiotherapy resistance-related genes as new prognostic biomarkers will help to develop novel strategies for the effective treatment of patients with pancreatic cancer.
Introduction
Pancreatic cancer is one of the deadliest solid organ tumors (1). Studies have shown that the incidence and mortality of pancreatic cancer are almost the same, with a 5-year survival rate of less than 10% (2). Given the specific anatomic location of the pancreas, patients with early-stage pancreatic cancer have mild or asymptomatic clinical symptoms, and most patients with pancreatic cancer are diagnosed at the advanced stages, after the onset of overt symptoms or at the time of physical examination, with little radical resection with little opportunity for radical resection (3). Even in operable patients, the 5-year survival rate improves to only about 20% (4). Due to the microenvironment around the tumor cells, the effects of chemotherapy and radiotherapy in patients with advanced pancreatic cancer are limited (5). The cancer microenvironment includes various factors such as hypoxia, immune cell infiltration, fibrosis, cytokine, oxidative stress, and acidosis. It has been shown that hypoxia-inducible factor 1α (HIF-1α) induced upregulation of retention in endoplasmic reticulum 1 (RER1) induces stemness and decreases chemosensitivity/radiosensitivity (6). Commonly used tumor markers related to the diagnosis of pancreatic cancer are carbohydrate antigen 19-9 (CA19-9), carcinoembryonic antigen (CEA), carbohydrate antigen 242 (CA242) and so on. However, CA19-9 may appear false positive in cases of biliary tract infection (cholangitis), inflammation or biliary obstruction, and it cannot indicate tumor or advanced lesions. CEA and CA242 also increased in other digestive system tumors, and were not specific (7,8). Therefore, there is an urgent need to explore new prognostic indicators to accurately predict the prognosis of pancreatic cancer patients and develop appropriate strategies to overcome chemoradiotherapy tolerance.
Effective chemotherapy drugs against pancreatic cancer include gemcitabine (GEM), paclitaxel, irinotecan, and 5-fluorouracil (5-FU). GEM is a nucleoside analogue and has been the most commonly used chemotherapeutic agent (9). The human albumin-associated paclitaxel (nab-paclitaxel) is also used for pancreatic cancer (10). Clinical trials have shown that nab-paclitaxel combined with GEM improves survival by 2 months in patients with advanced pancreatic cancer, without a significant increase in toxicity (11). Irinotecan, an inhibitor of topoisomerase I, has shown efficacy against cancer in several clinical trials (12), and its liposome encapsulation improves the treatment of refractory pancreatic cancer (13). 5-FU and its derivants, including capecitabine and S-1 (Tegafur Gimeracil Oteracil Potassium Capsule), are widely used for the treatment of gastrointestinal tumors owing to their ability to insert DNA and inhibit cell proliferation (14-16). In addition, radiotherapy is based on high-energy radiation that kills cancer cells and shrinks tumors, causing a series of physical and chemical reactions in which cells may lose their ability to divide and die (17). Currently, GEM-based monotherapy or in combination with chemo-radiotherapy remains the standard treatment option for pancreatic cancer (18). However, the poor prognosis of pancreatic cancer is mainly due to the fact that most patients receiving GEM chemotherapy eventually show resistance (19). Indeed, the fact that a small subset of cancer cells may be able to metabolize anti-cancer drugs during treatment, thereby developing resistance and allowing them to grow and become a dominant population, remains a major challenge for the treatment of pancreatic cancer (20). In addition, the failure of conventional chemo-radiotherapy to kill tumor-initiating cells or cancer stem cells (CSCs) is one of the major causes of tumor recurrence and drug resistance (21,22). During radiotherapy and chemotherapy, as long as tumor stem cells exist, they will continue to differentiate into tumor cells, even if the tumor cells are killed (23). Therefore, understanding the genes that influence resistance to therapy may help in the search for agents that reverse resistance to chemotherapy and radiotherapy.
With the rapid development of gene expression profiling technology represented by second-generation sequencing, the prognosis of patients can be predicted by gene expression profiling analysis and further screening of molecular markers with different characteristics. In this study, we established and validated a prognostic model based on chemo-radiotherapy resistant-related genes (CRRGs) to predict the prognosis of pancreatic cancer patients. We systematically investigated the prognostic value of CRRGs and their correlation with clinical features, revealing the potential role of CRRGs as potential prognostic biomarkers and novel therapeutic targets in pancreatic cancer patients. Furthermore, we confirmed the role of JAG1 in the maintenance of stemness in pancreatic cancer cells, which may be a promising target for the treatment of pancreatic cancer. We present this article in accordance with the TRIPOD reporting checklist (available at https://jgo.amegroups.com/article/view/10.21037/jgo-23-308/rc).
Methods
Data collection and preprocessing
By searching the Gene Expression Omnibus (GEO) database of the National Center for Biotechnology Information (NCBI) (http://www.ncbi.nlm.nih.gov/geo/), we obtained human microarray datasets of radiation-resistant pancreatic cancer cell lines (GSE193616) and gemcitabine-resistant pancreatic cancer cell lines (GSE80617). The gene expression data and clinical information of 177 pancreatic cancer patients were downloaded from The Cancer Genome Atlas (TCGA) database (https://portal.gdc.cancer.gov/). The GSE57495 and GSE78229 datasets were found in the Gene Expression Omnibus (GEO) database (http://www.ncbi.nlm.nih.gov/geo/) and were used for validation. Table 1 shows the clinical characteristics of patients from the three cohorts. To keep the gene expression levels of the training and testing sets at the same level, the GSE57495 and GSE78229 datasets from the TCGA and GEO cohorts were log2 (x+1) transformed and batch-normalized using the combat function in the “SVA” software package in R (version 4.1.2) (R Foundation for Statistical Computing, Vienna, Austria) to construct and validate a prognostic signature (24). All immunohistochemical (IHC) staining images were obtained from the Human Protein Atlas (HPA) database (http://www.proteinatlas.org/). The study was conducted in accordance with the Declaration of Helsinki (as revised in 2013).
Table 1
Characteristics | Training set (TCGA, N=177) |
Validation set (GEO, N=112) |
---|---|---|
Age (years) | ||
<65/≥65/NA | 81/96/0 | NA |
Gender | ||
Male/Female/NA | 97/80/0 | NA |
Status | ||
Alive/Dead/NA | 89/88/0 | 35/77/0 |
Grade | ||
G1/G2/G3/G4/NA | 31/94/48/2/2 | 2/24/21/1/64 |
Tumor stage | ||
I/II/III/IV/NA | 21/146/3/4/3 | 17/94/0/0/1 |
T stage | ||
T1/T2/T3/T4/NA | 7/24/141/3/2 | NA |
M stage | ||
M0/M1/MX | 79/4/94 | NA |
N stage | ||
N0/N1/NA | 49/123/5 | NA |
NA, clinical data are unknown; GEO, Gene Expression Omnibus; TCGA, The Cancer Genome Atlas.
Construction of a prognostic model
To investigate the relationship between the expression levels of CRRGs and overall survival (OS) in pancreatic cancer patients, we performed univariate Cox regression analysis using the survival R package with a significant screening criterion of P<0.05. The eligible CRRGs were applied to the next step of prognostic model construction. The least absolute shrinkage and selection operator (LASSO) Cox regression method was then used, run in the “GLMNET” software package, to avoid overfitting the prognostic features (25,26). Finally, a prognostic signature of nine CRRGs was constructed. We then calculated the risk scores based on the normalized messenger RNA (mRNA) expression data from the training set. For each patient, the risk score is the product of the prognostic marker gene expression level and the corresponding coefficient, i.e., the risk score = ∑ (coefficient × marker gene expression). The coefficient represented the weight of the corresponding CRRGs.
In addition, we classified patients with pancreatic cancer into high- and low-risk groups based on the median risk values. We performed log-rank to test Kaplan-Meier survival analyses to compare prognostic differences between the low- and high-risk groups. The receiver operating characteristic (ROC) curves were produced using the timeROC software package to evaluate the prediction efficiency of the model. The gene expression profiling interactive analysis (GEPIA) (http://GEPIA.cancer-pku.cn) method was used to analyze the relationship between the expression level of each gene and OS in the prognostic model of pancreatic cancer patients, using pancreatic cancer tumor data from TCGA and normal tissue matching data from TCGA and genotype-tissue expression (GTEX) (27).
Relationship between the risk score and clinicopathological features
To evaluate whether the risk score could be independently used to predict the prognosis of patients with pancreatic cancer, we performed univariate and multivariate Cox regression analyses of the risk score and other clinicopathological factors. Factors with hazard ratio (HR) >1 or <1 and P<0.05 in both analyses could be used as independent prognostic factors for predicting the prognosis of patients. Forest plots were plotted using the forestploter R package, showing the P value, HR, and 95% confidence interval (CI) for each variable. To further improve the accuracy of the risk score model in predicting the prognosis of patients and facilitate clinical application, we used the RMSR package to build a nomogram. Decision curve analysis (DCA) was used to compare the advantages of the different models.
Drug sensitivity analysis
Using the Genomics of Drug Sensitivity in Cancer (GDSC) (http://www.cancerrxgene.org/) database of the half-maximal inhibitory concentration (IC50) drug profile to analyze the relationship between gene expression and drug sensitivity (28), the Pearson correlation coefficient was applied to evaluate the correlation between gene expression and drug sensitivity. A positive correlation signified that the high gene expression is resistant to the drug, while the low gene expression is sensitive to the drug.
Gene set enrichment analysis (GSEA)
GSEA provided by MSIGDB was used to identify the genetic biological processes and signaling pathways (29). The GSEA software is available for download from the official website (https://www.broadinstitute.org/gsea/). The c2.cp.kegg.v7.5.1symbols.gmtgene set was selected as the reference gene set. The significance threshold was determined by a 1,000-permutations analysis, and False discovery rate (FDR) <25% and P<0.05 were considered statistically significant.
Cell culture
Human pancreatic cancer cell lines, PANC-1, SW1990, and MIA PaCa-2, were purchased from the American Type Culture Collection (ATCC, VA, USA). The cells were cultured in Dulbeccos minimum essential medium (DMEM, Cat: SH30243, HyClone, UT, USA). 10% fetal bovine serum (FBS, Cat: 10100147, Gibco, Carlsbad, CA, USA) and 1% penicillin-streptomycin solution (Cat: C0222, Beyotime, Jiangsu, China) were added to the medium. The cells were cultured in a humidified incubator with 5% CO2 at 37 ℃ and were propagated every 2–3 days to maintain growth.
Cell line construction
Radiation-resistant (RR) cancer cell lines have been established from nasopharyngeal, esophageal, breast, and lung cancers (30-34). In this study, we established an RR pancreatic cancer cell line according to these methods. PANC-1 was inoculated and cultured in a 10-cm culture dish. When the cells reached 50% confluency, they were irradiated with 2 Gy of radiation, incubated to 90% confluency, and then passaged. The irradiation process was repeated for each passage until a total radiation dose of at least 60 Gy was achieved. We generated GEM-resistant PANC-1 cells in the PANC-1 cell line by exposing them to increasing concentrations of GEM for about 3 months (35-38). Parental cell lines were first treated with 0.1 to 10 µM GEM for 48 hours, and cell viability was determined using methyl thiazolyl tetrazolium (MTT) colorimetry. The IC50 was then calculated. Then, PANC-1 cells were treated with GEM at a concentration less than the IC50. When the cells were acclimated to this concentration, the GEM concentration was increased to 1.5 µM. This procedure resulted in the establishment of a GEM-resistant (GR) cell line.
siRNA sequence
GENEWIZ/AZENTA (Suzhou, Jiangsu, China) synthesized the specific small-interfering RNAs (siRNAs) against JAG1 and its control. Cells were transfected using Lipofectamine 3000 reagent (Cat: 100022052, Invitrogen, Carlsbad, CA, USA) and siRNA according to the manufacturer’s instructions. The siRNA sequences were as follows:
si-NC: Sense: 5'- UUCUCCGAACGUGUCACGUdTdT-3'.
Antisense: 5'- ACGUGACACGUUCGGAGAAdTdT-3'.
Si-JAG1-1: Sense: 5'- CCAGUUAGAUGCAAAUGAATT-3'.
Antisense: 5'- UUCAUUUGCAUCUAACUGGTT-3'.
Si-JAG1-2: Sense: 5'- GGUCAGAAUUGUGACAUAATT-3'.
Antisense: 5'- UUAUGUCACAAUUCUGACCTT-3'.
Si-JAG1-3: Sense: 5'- GGACAAACAAACAGGACAATT-3'.
Antisense: 5'- UUGUCCUGUUUGUUUGUCCTT-3'
Quantitative real-time polymerase chain reaction (qRT-PCR)
Total RNA was isolated using Trizol reagent (Cat: 15596026, Thermo Fisher Scientific, Waltham, MA, USA) according to the manufacturer’s protocol. After spectrophotometric quantification, reverse transcription was performed using the Primescript RT kit (Perfect Real Time) Cat: RR037B, TaKaRa, Tokyo, Japan) with a final volume of 20 µL to obtain 1 µg of total RNA. A real-time PCR system (Roche, Indianapolis, IN, USA) based on Light Cycler 96 was used to detect the complementary DNA (cDNA) of the same amount of RNA. The reaction system (13 µL) contained reverse and forward primers, corresponding cDNA, and the SYBR Green PCR master mix (Roche, Cat: 04913914001). The data were analyzed using the 2-ΔΔCt method, with β-Actin gene expression serving as the internal standard. The following gene primers were used:
β-Actin, forward, 5'-TCATGAAGTGTGACGTGGACAT-3', reverse, 5'-CTCAGGAGGAGCAATGATCTTG-3'; JAG1, forward, 5'-ATTACCAGGATAACTGTGCGAA-3', reverse, 5'- CAAATGTGCTCCGTAGTAAGAC-3'; cluster of differentiation 24 (CD24), forward, 5'- CAGGGCAATGATGAATGAGAAT-3', reverse, 5'- CCTGGGCGACAAAGTGAGA-3’; CD44, forward, 5'-GTGATGGCACCCGCTATGTC-3', reverse, 5'-AACCTCCTGAAGTGCTGCTCC-3'; Epithelial cell adhesion molecule (EPCAM), forward, 5'-TAATCGTCAATGCCAGTGTACTTC-3', reverse, 5'-GCCATTCATTTCTGCCTTCAT-3'.
Colony formation assay
Cells were seeded in 12-well plates at a density of 500 cells per well and treated after 12 hours. The cell culture medium was replaced with fresh medium every 2–3 days. 12–14 days later, they were fixed with 4% paraformaldehyde (Beyotime, Cat: P0099) for 30 minutes at room temperature and stained with crystal violet solution (Beyotime Biotechnology Institute, Cat: C0121). The number of visible colonies was counted. Colony-forming efficiency = (average number of colonies/500) ×100%.
MTT assay
Cell growth was evaluated using the MTT [3-(4,5-dimethyl-2-yl)-2,5-diphenyl tetrazolium bromide] assay. The cells were seeded in 96-well plates at a rate of 3,000 cells per well and treated with GEM after 24 hours. MTT (methyl thiazolyl diphenyl tetrazolium bromide; Sigma # M5655) (Sigma-Aldrich, St. Louis, MO, USA) (5 mg/mL) was added to the medium at 0, 24, 48, and 72 h. After incubation at 37 ℃ for 4 hours, the medium was removed, and 200 mL of dimethyl sulfoxide (DMSO, Sigma, Cat: No D2650) was added to each well to dissolve. The absorbance of DMSO was measured at 490 nm using a microplate reader (Thermo Fisher Scientific, Waltham, MA, USA). The relative cell viability was calculated as follows: relative cell viability (%) = (absorbance of the experimental group/absorbance of the control group) × 100%.
Xenograft tumor model
Four-week-old female BALB/c thymic nude mice Hangzhou Ziyuan Experimental Animal Technology Co., Ltd. (Zhejiang, China) were nurtured in the animal laboratory under a specific pathogen-free environment. GR, RR, 5×106 PANC-1 cells transfected with siRNA-targeting JAG1 or a negative control were injected into the left axilla of the nude mice. Tumor-bearing nude mice were treated with chemotherapy or radiotherapy by intraperitoneal injection of GEM at a dose of 50 mg/kg, or were placed in a confined container and irradiated with tumors to receive a dose of 10 Gy once every 3 days. At the end of the experiment, the mice were anesthetized and the tumors were excised. The length (L) and width (W) of each subcutaneous tumor were measured using calipers. Tumor volume (TV) was calculated as TC = (L × W2)/2.
Statistical analysis
We used R software (V4.1.2) and GraphPad Prism9 (GraphPad Software Inc., San Diego, CA, USA) to analyze our data. Survival analysis was performed using Kaplan-Meier and Log-rank tests. The experimental data were analyzed by the Student’s t-test, one-way analysis of variance (ANONA), and two-way ANOVA. Each experiment was performed at least three times, and P<0.05 was considered statistically significant (*P<0.05, **P<0.01, ***P<0.001, #P<0.05, ##P<0.01, ###P<0.001, ####P<0.0001.).
Research procedures
We constructed the PANC-1RR and PANC-1GR cell lines and tested the tolerance of the two cells to chemotherapy and radiation by performing a clonal formation experiment and a nude mouse xenograft experiment. Subsequently, we performed difference analysis on the two datasets of GSE193616 and GSE80617, and performed Wayne analysis on the results to obtain CRRGs. A CRRGs-related prognostic model was constructed in the TCGA pancreatic cancer (TCGA-PAAD) cohort and we assessed the accuracy of the model using Kaplan-Meier survival analysis and ROC curve, which was validated in the GEO cohort. We then used univariate and multivariate independent prognostic analyses to explore whether risk scoring could become an independent factor in predicting the overall survival of patients with pancreatic cancer. The relationship between risk scores and clinical features was studied by correlation analysis. The performance of prognostic risk models was comprehensively evaluated by drawing clinical decision curves and nomograms. We analyzed the relationship between CRRGs expression and drug sensitivity using the GDSC database to identify genes for further study. Finally, the expression of JAG1 was inhibited by transfecting specific small interfering RNA into pancreatic cancer cell lines, and the biological function of JAG1 in pancreatic cancer was explored using qPCR, clone formation assay, MTT assay, and nude mice xenografts assay.
Results
GR and RR PANC-1 cell lines were cross-resistant to radiotherapy and chemotherapy
We treated GR, RR, and PANC-1 cells with GEM and X-ray, respectively. Both GR and RR PANC-1 cells were resistant to GEM and X-ray, suggesting a cross-resistance to chemotherapy and radiotherapy in GR and RR cells (Figure 1A,1B). In addition, in vivo experiments demonstrated cross-resistance of GR and RR cells to chemotherapy and radiotherapy (Figure 1C,1D). As shown in Figure 1E, we performed a differential analysis of the GSE193616 dataset [log2 fold-change (FC) >1] and obtained 1,779 genes that were highly expressed in RR pancreatic cancer cell lines. Similarly, a differential analysis of the GSE80617 dataset using the limma package (P<0.05, log2FC >1) yielded 864 genes that were highly expressed in GR pancreatic cancer cell lines (Figure 1F). We performed a Venn analysis on the genes that differed between the two datasets, which revealed 25 CRRGs (Figure 1G).
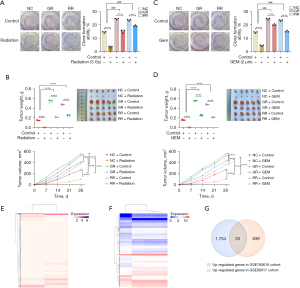
Screening of critical CRRGs to establish and validate a prognostic model for pancreatic cancer
Figure 2A,2B display the expressions of the 25 CRRGs in the GSE193616 and GSE80617 datasets, respectively. To better understand the prognostic role of CRRGs in pancreatic cancer, we performed univariate Cox regression analysis on the gene expression levels in TCGA-PAAD dataset and obtained 12 genes associated with the prognosis of pancreatic cancer patients (Figure 2C). CRRGs that may be highly correlated with other CRRGs were then removed by LASSO Cox regression analysis to avoid overfitting, which could confound the predictions. Finally, prognostic characteristics were determined for nine CRRGs (SNAP25, GPR87, DLL1, LAD1, WASF3, ARHGAP29, ZBED2, GAD1, and JAG1) based on the optimal value of λ (Figure 2D).
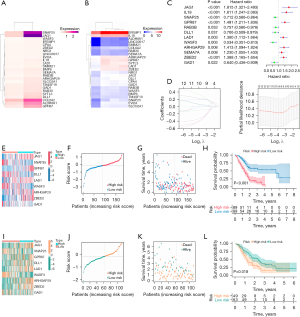
The nine gene-based prognostic features were used to calculate the risk score for each sample according to the following formula: Risk Score = (0.2188 × expression of JAG1) + (−0.0946 × expression of SNAP25) + (0.1991 × expression of GPR87) + (−0.3228 × expression of DLL1) + (0.0525 × expression of LAD1) + (−0.3787 × expression of WASF3) + (0.3205 × expression of ARHGAP29) + (0.0620 × expression of ZBED2) + (−0.4118 × expression of GAD1).
The difference in CRRGs expressions between the low- and high-risk groups in the TCGA-PAAD dataset is shown in the heatmap (Figure 2E). To further explore the significance of the risk scores, patients were divided into high-risk (n=89) and low-risk (n=89) groups based on the median cut-offs. The distribution of risk scores and survival status were then investigated (Figure 2F,2G), and the results showed that the higher the risk score, the denser the distribution of death status, indicating that the nine-CRRGs score is accurate and reliable for predicting the prognosis and survival of pancreatic cancer patients. In addition, Kaplan-Meier survival curves consistently showed that OS was significantly lower in the high-risk group than in the low-risk group (Figure 2H, P<0.001).
To further validate the predictive value of the predictive models, we used a cohort from the GEO database (GSE57495 and GSE78229) for external validation. The difference in CRRGs expressions between the low- and high-risk groups in the validation set is shown in the heatmap (Figure 2I). The prognostic risk scores were calculated for patients in the validation cohort based on the expression values of the nine predictive CRRGs. Each patient in the validation cohort was flagged as a high- or low-risk case by comparing the patient’s risk score with the cutoff from the training cohort (Figure 2J,2K). Consistent with the results generated by the training cohort, the Kaplan-Meier survival curves showed that individuals from the high-risk group had worse outcomes than those in the low-risk group (Figure 2L, P=0.018).
Suitability of the nine-CRRGs signature as an independent prognostic indicator
To explore whether the prognostic model is independent of traditional clinical factors, we used univariate Cox regression to analyze pancreatic cancer patients from TCGA cohort. The results showed that age (P=0.015), histological grade (P=0.028), and risk score (P<0.001), all of which were factors of high-risk pancreatic cancer, were significantly related to the patient’s OS (Figure 3A). We further conducted multivariate Cox regression analysis, and the results verified that risk score (P<0.001) was a significant independent factor (Figure 3B).
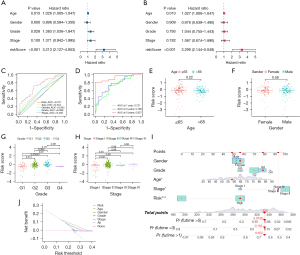
We also analyzed the predictive power of prognostic models associated with CRRGs using multivariate ROC curves (Figure 3C) and time-dependent ROC curves (Figure 3D), and the results showed that nine-CRRGs score was more accurate and reliable in predicting the prognosis of patients with pancreatic cancer than traditional clinical factors. Next, we conducted a stratified analysis to explore the relationship between risk score and clinicopathological characteristics according to age (≤65, >65 years), gender (male and female), grade (G1, G2, G3, G4), and stage (I, II, III, IV). The results showed that the risk scores of different grades and stages were significantly different (Figure 3E-3H).
We then built a predictive nomograph (Figure 3I) based on risk score and gender, age, grade, and stage in TCGA cohort to further improve the predictive ability of CRRGs prognostic characteristics. At the same time, we performed a DCA to evaluate the predictive value of the nomogram in the clinical decision of TCGA cohort (Figure 3J). The DCA curve showed that the risk-scoring model is superior to other clinical features in predicting the benefits of clinical maps.
Identification of candidate target genes among the nine-CRRGs
We used the GEPIA online site to map whether the expression of each gene in the prognostic model differed between the pancreatic cancer tumor tissues and normal tissues. As shown in Figure 4A-4I, JAG1, GPR87, LAD1, ZBED2, and GAD1 genes were highly expressed in tumor tissues (P<0.05). Furthermore, we used Kaplan-Meier plots to test whether the expression of each gene in the prognostic model was associated with the prognosis of pancreatic cancer (Figure 4J-4R). The results showed that patients with higher SNAP25 or WASF3 expressions had a better prognosis, whereas those with higher JAG1, GPR87, or ZBED2 expressions had a worse prognosis.
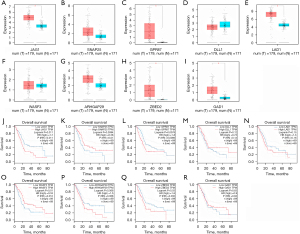
According to the IC50 drug data in GDSC, we found that the expressions of JAG1, GPR87, LAD1, ARHGAP29, ZBED2, and GAD1, were positively correlated with the IC50 of 5-FU, GEM, Irinotecan, Oxaliplatin, and/or Paclitaxel, showing resistance to these drugs. Meanwhile, the expression of SNAP25 was negatively correlated with the IC50 of Irinotecan, and the expression of DLL1 was negatively correlated with the IC50 of irinotecan, oxaliplatin, and paclitaxel (Figure 5A-5E).
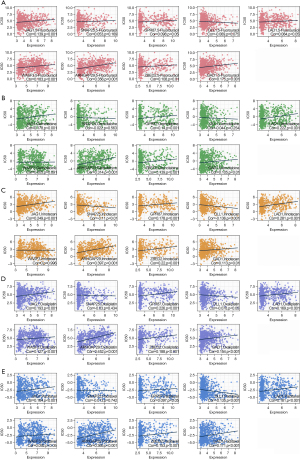
Among the nine CRRGs, JAG1 and ZBED2 were highly expressed in tumor tissues, and negatively correlated with prognosis. Moreover, a higher expression of JAG1 or ZBED2 indicated resistance against all effective chemotherapeutics in pancreatic cancer.
JAG1 enhanced stemness and promoted the growth of pancreatic cancer cells
In the training set, we ran GSEA on the high and low JAG1 expression groups. The enrichment pathways of the high JAG1 expression group included apoptosis, axonal guidance, extracellular matrix (ECM)receptor interaction, the wingless/integrated (WNT) signaling pathway, the Notch signaling pathway, the Hedgehog signaling pathway, the P53 signaling pathway, tight junctions, and the transforming growth factor-β (TGF-β) signaling pathway (Figure 6A). Among these pathways, the WNT, Notch, Hedgehog, and TGF-β pathways were involved in stemness maintenance (39). In our previous study, we proved that the existence of pancreatic CSCs induced resistance to chemotherapy (40). Considering that a previous study demonstrated that JAG1 affects tumor stem cell-like properties in breast cancer (41), we speculated that JAG1 could trigger tolerance to chemoradiotherapy by maintaining the stemness of pancreatic cancer cells.
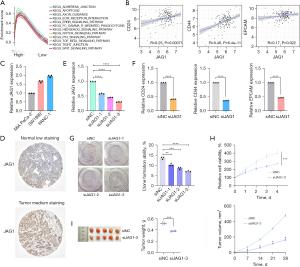
We further analyzed the relationship between JAG1 and the surface markers (CD24, CD44, and EPCAM) of pancreatic CSCs in the TCGA-PAAD cohort. JAG1 expression was positively correlated with these three markers, according to the Pearson correlation coefficient (Figure 6B). Next, we detected the expression of JAG1 in three human pancreatic cancer cells, MIA Paca-2, SW1990, and Panc-1, and identified that PANC-1 expressed the highest level of JAG1 in these three cell lines (Figure 6C). By using siRNAs, JAG1 could be successfully knocked down in PANC-1 cells (Figure 6D). After JAG1 silencing in PANC-1 cells, the expressions of CD24, CD44, and EPCAM in the pancreatic cells were also down-regulated (Figure 6E).
To determine the protein expression level of JAG1, IHC staining images of pancreatic cancer tissues and normal pancreatic tissues were obtained from the HPA database (http://www.proteinatlas.org/). The results showed that JAG1 protein expression in pancreatic cancer was higher than that in normal tissues (Figure 6F). In vitro assays proved that the knock-down of JAG1 repressed the colony-forming ability and viability of PANC-1 cells (Figure 6G,6H). The in vivo studies further confirmed that the knock-down of JAG1 inhibited the growth of xenografts (Figure 6I).
Knockdown of JAG1 overcame the chemo-radiotherapy resistance of GR and RR pancreatic cancer cells
The in vitro assays confirmed that the knock-down of JAG1 sensitized PANC-1 cells to GEM treatment (Figure 7A), and the in vivo studies further confirmed that the knock-down of JAG1 increased GEM sensitivity (Figure 7B). We then detected the expression of JAG1 in GR and RR PANC-1 cells. As shown in Figure 7C, JAG1 was highly expressed in GR and RR cells compared with the control group. Knock-down of JAG1 not only repressed the colony formation ability of GR and RR cells (Figure 7D) but also recovered the sensitivity to GEM in GR cells (Figure 7E) and the sensitivity to X-ray both in RR cells (Figure 7F). Moreover, the cross-resistance to radiotherapy in GR cells and the cross-resistance to chemotherapy in RR cells could also be attenuated by silencing JAG1 (Figure 7G,7H), suggesting that cross-resistance was mediated by JAG1.
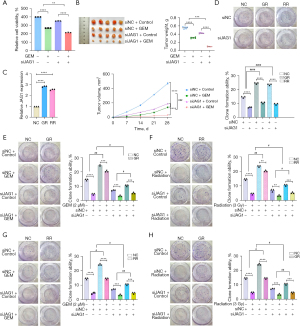
Discussion
Pancreatic cancer has high rates of morbidity and mortality as well as a considerable socio-economic burden (42). Moreover, the tumors have a high degree of heterogeneity, which presents significant challenges for prognostic prediction and individualized treatment. The accurate prediction of OS in pancreatic cancer will contribute to the development of individualized treatment regimens. Therefore, there is an urgent need to find novel markers to provide new ideas for the classification and treatment of pancreatic cancer and improve the prognosis of patients.
A high correlation between response to cisplatin and subsequent radiation response has been reported in patients who received radiotherapy after receiving a cisplatin-based regimen (43,44). In addition, a high correlation between resistance to cisplatin and radiation therapy was also observed in these studies. In the present study, we found that the GR cells showed resistance to radiotherapy, and RR cells were also tolerant to chemotherapy, suggesting that some universal mechanisms could be shared by chemotherapy resistance and radiation resistance. Bioinformatics and statistical tools were applied to systematically analyze the predictive accuracy of CRRGs in pancreatic cancer. We first performed univariate Cox and LASSO Cox regression analyses of CRRGs in a sample of 178 pancreatic cancer patients obtained from the TCGA database, and nine CRRGs that were significantly associated with OS were identified (SNAP25, GPR87, DLL1, LAD1, WASF3, ARHGAP29, ZBED2, GAD1, and JAG1).
SNAP25 is a presynaptic membrane-binding protein that is anchored to the cell membrane surface by palmitoyl side chains located in the central region of the molecule. It is associated with maturation and synaptogenesis during neuronal development (45) and also affects the expression of receptors such as N-methyl-D-aspartic acid receptors (NMDARs) in the plasma membrane (46,47). Previous studies have shown that SNAP25 regulates proliferation and chemotherapy resistance in gastric neuroendocrine carcinoma cells by controlling the stability of the protein kinase B (PKB) (48). The expression of G protein-coupled receptor 87 (GPR87) is up-regulated in pancreatic cancer and clinical tissues, and its overexpression promotes the proliferation, metastasis, angiogenesis, and resistance to chemotherapy-induced apoptosis in pancreatic cancer (49). DLL1 is a canonical receptor in the Notch signaling pathway (50), and elevated DLL1 levels in the tumor microenvironment decrease tumor vessel density, increase vascular perfusion, and reduce hypoxia in tumor tissue. Furthermore, it is suggested that DLL1 induces tumor vessel normalization (51). Study has also revealed that DLL1 passes nuclear factor kappa-B (NF-κB) pathway increases the resistance to DNA damage and cell death, and plays a key role in promoting the progression, metastasis, and chemoresistance of invasive breast intraluminal tumors (52).
LAD1 is a collagen-anchored filament protein in the basement membrane of mammalian epidermal cells (53,54). A physical interaction between LAD1 and Stratifin (14-3-3σ) has been reported to promote breast tumor invasion by regulating actin filament turnover (55). LAD1 is highly expressed in docetaxel-resistant prostate cancer cells but its expression is significantly suppressed in tumor samples after docetaxel treatment, suggesting that the upregulation of LAD1 may contribute to the development of docetaxel resistance in prostate cancer (56). WASF3 is a member of the Wiskott-Aldrich syndrome protein family and plays an important role in the regulation of actin cytoskeletal dynamics as well as in cancer cell invasion and metastasis (57). The inhibition of WASF3 expression enhances the sensitivity of MGC-803 cells to Oxaliplatin by reducing ATG12 (autophagy related 12)-mediated autophagy, promoting apoptosis, and inhibiting cell proliferation (58). ARHGAP29 is a RhoGTPase-activating protein that is involved in RhoA (Ras homolog gene family, member A) regulation. It is expressed in several tissues, including the heart, skeletal muscle, and placenta (59). The levels of ARHGAP29 in circulating tumor cells and renal carcinoma cells are positively correlated with metastatic potential and may play a role in cancer by modulating actin kinetics (60,61).
The ZBED gene family encodes nine zinc finger-containing transcription factors (TFs) in humans and is derived from a domesticated DNA transposase gene from the histone acetyltransferases (hAT) transposable element (62). A genome-wide association study identified ZBED2 as a candidate locus affecting the risk of smoking-induced pancreatic cancer (63). The expression of ZBED2 is most correlated with the expression of immune-related signaling pathways, including interferon alpha (IFNα), IFNγ, and tumor necrosis factor alpha (TNFα) in breast cancer cells, and exerts tumor inhibitory effects by enhancing IFN signaling (64). Glutamate decarboxylase 1 (GAD1) is involved in the regulation of glutamate (65), and its altered expression has been associated with psychiatric diagnoses, including schizophrenia, bipolar disorder, and autism (66). Study has shown that GAD1 expression is associated with pleural invasion, vascular invasion, and advanced stages of non-small cell lung cancer (NSCLC) (67).
JAG1 is also one of the most important model genes in our research. Similar to DLL1, JAG1 is also a receptor in the Notch signaling pathway (50) and plays an important role in numerous human diseases. It has been reported that JAG1 is targeted by microRNA (miR-26b-5p) to regulate cell proliferation and apoptosis in multiple myeloma (68). The miR-30d/JAG1 axis regulates pulmonary fibrosis through Notch signaling (69). In HER2 (human epidermal growth factor receptor-2)-positive gastric carcinoma (GC) cells, the autocrine effect of the interleukin-6/signal transducer and activator of transcription 3 (IL-6/STAT3) signaling pathway can activate JAG1/Notch signaling and induce trastuzumab resistance (70). EGFRVIII is a variant of the epidermal growth factor receptor (EGFR), which can drive abnormal mitogen-activated protein kinase (MAPK) signal transduction, such as MEK/ERK (mitogen-activated extracellular signal-regulated kinase/extracellular regulated protein kinases) signal transduction. Its expression level is positively correlated with the expression level of JAG1 in glioblastoma patients. JAG1/Notch signaling stimulated by the EGFRVIII/MEK/ERK axis maintains the stem-like properties of glioma-initiating cells and induces their resistance to radiation therapy (71).
Considering that tumor evolution is a complex process, the multi-gene-based prognostic risk model applied in the present study can predict the prognosis of cancer patients more accurately than single-gene prediction. Since there is still the possibility of overtraining or false positives, we used the GSE57495 and GSE78229 datasets from the GEO database to further validate the prognostic value of the CRRGs signature. The findings showed that CRRG signaling is reproducible and robust in the prognosis of pancreatic cancer patients. To play a complementary role between molecular characteristics and clinical characteristics, the CRRGs prognostic model and clinical characteristics were combined to construct a nomogram, and the prognostic estimation level of pancreatic was improved. In conclusion, CRRGs’ prognostic markers can accurately predict the survival outcomes of pancreatic cancer patients, demonstrating great clinical application potential for individualized prognostic evaluation and treatment.
In recent years, a small subset within tumors, also known as tumor stem cells, has received research attention for its ability to self-renew and generate heterogeneous tumor cell lineages (72). CSCs recognize other cancer cells by expressing stem cell surface markers such as CD24, CD44, and EPCAM. These cells are characterized by high therapeutic resistance and proliferative capacity (73). Local recurrence of tumors after surgical resection, chemotherapy, and/or radiotherapy is associated with drug resistance and the presence of CSCs, which are characterized by high treatment resistance and proliferation capacity (73). Targeting molecules and signaling pathways associated with CSCs is a potential strategy to overcome these problems. In addition to its involvement in angiogenesis through the Notch1 signaling pathway, JAG1 is also involved in a variety of tumor-related functions, including CSC development, tumor cell growth, epithelial-mesenchymal transition (EMT), drug resistance, and migration (74-76). In this study, we observed increased expression of JAG1 in pancreatic cancer tissue compared with normal pancreatic tissue. In addition, the expression of JAG1 was positively correlated with the expressions of pancreatic cancer tumor stem cell surface markers, CD24, CD44, and EPCAM. We also found that JAG1 silencing induces anti-pancreatic cancer effects in vitro. JAG1 silencing significantly inhibited the proliferation, viability, as well as chemotherapy and radiotherapy resistance of human pancreatic cancer cells. Therefore, we suggest that JAG1 may be a promising therapeutic target for pancreatic cancer. Chemotherapy is still the main treatment for pancreatic cancer. Although a large number of studies on targeted drugs or immunotherapy have failed to prove that it is superior to the standard chemotherapy regimen, some drugs, whether used alone or in combination with other drugs, have achieved promising results. Approval of olaparib maintenance treatment for BRCA-mutant PC represents an encouraging achievement in individualized treatment of this intractable disease, and opens the door for studying various drugs with potential synergistic effects with PARPi, including immunotherapy and tyrosine kinase inhibitors (77). There are new drugs, such as NTRK inhibitor for NTRK fusion positive tumor or Pamuzumab for MSI-H tumor, which makes it reasonable for people to find new treatment schemes in different subgroups of PC patients through genetic testing (78).
However, this study has various limitations and needs further optimization. First, although we confirmed our prediction model with retrospective data from a public database, more prospective real-world data are required to confirm its clinical applicability. Secondly, our prognostic model is based on the mRNA expression level to predict the prognosis of patients; however, the gene protein level may be more consistent with the actual clinical situation of patients. Due to the lack of clinical samples and corresponding survival information, it is impossible to verify the relationship between the protein expression level of characteristic genes and the prognosis of patients. Therefore, this very critical component will need to be refined in future clinical work. Third, the specific mechanism of JAG1-mediated chemoradiotherapy tolerance of pancreatic cancer needs to be further studied, and the biological functions of several other CRRGs in pancreatic cancer also need to be further verified through a series of experiments.
Conclusions
In conclusion, this study established a pancreatic cancer prognostic model based on the SNAP25, GPR87, DLL1, LAD1, WASF3, ARHGAP29, ZBED2, GAD1, and JAG1 genes, which can effectively predict the prognosis of pancreatic cancer patients. Based on our results, it seems likely that these genes could be used as biomarkers to generally predict the survival of individuals with pancreatic cancer. In vitro and in vivo experiments showed that silencing JAG1 inhibits the chemoradiotherapy tolerance of pancreatic cancer cells, thereby playing a role in anti-pancreatic cancer therapy.
Acknowledgments
Funding: This work was supported by the National Natural Science Foundation of China (Nos. 81873587 and 81874454).
Footnote
Reporting Checklist: The authors have completed the TRIPOD reporting checklist. Available at https://jgo.amegroups.com/article/view/10.21037/jgo-23-308/rc
Data Sharing Statement: Available at https://jgo.amegroups.com/article/view/10.21037/jgo-23-308/dss
Peer Review File: Available at https://jgo.amegroups.com/article/view/10.21037/jgo-23-308/prf
Conflicts of Interest: All authors have completed the ICMJE uniform disclosure form (available at https://jgo.amegroups.com/article/view/10.21037/jgo-23-308/coif). The authors have no conflicts of interest to declare.
Ethical Statement: The authors are accountable for all aspects of the work in ensuring that questions related to the accuracy or integrity of any part of the work are appropriately investigated and resolved. The study was conducted in accordance with the Declaration of Helsinki (as revised in 2013).
Open Access Statement: This is an Open Access article distributed in accordance with the Creative Commons Attribution-NonCommercial-NoDerivs 4.0 International License (CC BY-NC-ND 4.0), which permits the non-commercial replication and distribution of the article with the strict proviso that no changes or edits are made and the original work is properly cited (including links to both the formal publication through the relevant DOI and the license). See: https://creativecommons.org/licenses/by-nc-nd/4.0/.
References
- Rawla P, Sunkara T, Gaduputi V. Epidemiology of Pancreatic Cancer: Global Trends, Etiology and Risk Factors. World J Oncol 2019;10:10-27. [Crossref] [PubMed]
- Moravec R, Divi R, Verma M. Detecting circulating tumor material and digital pathology imaging during pancreatic cancer progression. World J Gastrointest Oncol 2017;9:235-50. [Crossref] [PubMed]
- Mizrahi JD, Surana R, Valle JW, et al. Pancreatic cancer. Lancet 2020;395:2008-20. [Crossref] [PubMed]
- Zhang Q, Zeng L, Chen Y, et al. Pancreatic Cancer Epidemiology, Detection, and Management. Gastroenterol Res Pract 2016;2016:8962321. [Crossref] [PubMed]
- Yamasaki A, Yanai K, Onishi H. Hypoxia and pancreatic ductal adenocarcinoma. Cancer Lett 2020;484:9-15. [Crossref] [PubMed]
- Chen S, Zhang J, Chen J, et al. RER1 enhances carcinogenesis and stemness of pancreatic cancer under hypoxic environment. J Exp Clin Cancer Res 2019;38:15. [Crossref] [PubMed]
- Stojkovic Lalosevic M, Stankovic S, Stojkovic M, et al. Can preoperative CEA and CA19-9 serum concentrations suggest metastatic disease in colorectal cancer patients? Hell J Nucl Med 2017;20:41-5. [PubMed]
- Zhu L, Xue HD, Liu W, et al. Enhancing pancreatic mass with normal serum CA19-9: key MDCT features to characterize pancreatic neuroendocrine tumours from its mimics. Radiol Med 2017;122:337-44. [Crossref] [PubMed]
- Adamska A, Domenichini A, Falasca M. Pancreatic Ductal Adenocarcinoma: Current and Evolving Therapies. Int J Mol Sci 2017;18:1338. [Crossref] [PubMed]
- Mohammed S, Van Buren G 2nd, Fisher WE. Pancreatic cancer: advances in treatment. World J Gastroenterol 2014;20:9354-60. [PubMed]
- Goldstein D, El-Maraghi RH, Hammel P, et al. nab-Paclitaxel plus gemcitabine for metastatic pancreatic cancer: long-term survival from a phase III trial. J Natl Cancer Inst 2015;107:dju413. [Crossref] [PubMed]
- Yagublu V, Caliskan N, Lewis AL, et al. Treatment of experimental pancreatic cancer by doxorubicin-, mitoxantrone-, and irinotecan-drug eluting beads. Pancreatology 2013;13:79-87. [Crossref] [PubMed]
- Kipps E, Young K, Starling N. Liposomal irinotecan in gemcitabine-refractory metastatic pancreatic cancer: efficacy, safety and place in therapy. Ther Adv Med Oncol 2017;9:159-70. [Crossref] [PubMed]
- Burris HA 3rd, Moore MJ, Andersen J, et al. Improvements in survival and clinical benefit with gemcitabine as first-line therapy for patients with advanced pancreas cancer: a randomized trial. J Clin Oncol 1997;15:2403-13. [Crossref] [PubMed]
- Ueno H, Ioka T, Ikeda M, et al. Randomized phase III study of gemcitabine plus S-1, S-1 alone, or gemcitabine alone in patients with locally advanced and metastatic pancreatic cancer in Japan and Taiwan: GEST study. J Clin Oncol 2013;31:1640-8. [Crossref] [PubMed]
- Uesaka K, Boku N, Fukutomi A, et al. Adjuvant chemotherapy of S-1 versus gemcitabine for resected pancreatic cancer: a phase 3, open-label, randomised, non-inferiority trial (JASPAC 01). Lancet 2016;388:248-57. [Crossref] [PubMed]
- Chen GZ, Zhu HC, Dai WS, et al. The mechanisms of radioresistance in esophageal squamous cell carcinoma and current strategies in radiosensitivity. J Thorac Dis 2017;9:849-59. [Crossref] [PubMed]
- Liu K, Geng Y, Wang L, et al. Systematic exploration of the underlying mechanism of gemcitabine resistance in pancreatic adenocarcinoma. Mol Oncol 2022;16:3034-51. [Crossref] [PubMed]
- Karasic TB, O'Hara MH, Loaiza-Bonilla A, et al. Effect of Gemcitabine and nab-Paclitaxel With or Without Hydroxychloroquine on Patients With Advanced Pancreatic Cancer: A Phase 2 Randomized Clinical Trial. JAMA Oncol 2019;5:993-8. [Crossref] [PubMed]
- Zeng S, Pöttler M, Lan B, et al. Chemoresistance in Pancreatic Cancer. Int J Mol Sci 2019;20:4504. [Crossref] [PubMed]
- Gangemi R, Paleari L, Orengo AM, et al. Cancer stem cells: a new paradigm for understanding tumor growth and progression and drug resistance. Curr Med Chem 2009;16:1688-703. [Crossref] [PubMed]
- Bao S, Wu Q, McLendon RE, et al. Glioma stem cells promote radioresistance by preferential activation of the DNA damage response. Nature 2006;444:756-60. [Crossref] [PubMed]
- Ma Y, Xue H, Wang W, et al. The miR-567/RPL15/TGF-β/Smad axis inhibits the stem-like properties and chemo-resistance of gastric cancer cells. Transl Cancer Res 2020;9:3539-49. [Crossref] [PubMed]
- Wu M, Li X, Zhang T, et al. Identification of a Nine-Gene Signature and Establishment of a Prognostic Nomogram Predicting Overall Survival of Pancreatic Cancer. Front Oncol 2019;9:996. [Crossref] [PubMed]
- McEligot AJ, Poynor V, Sharma R, et al. Logistic LASSO Regression for Dietary Intakes and Breast Cancer. Nutrients 2020;12:2652. [Crossref] [PubMed]
- Tibshirani R. The lasso method for variable selection in the Cox model. Stat Med 1997;16:385-95. [Crossref] [PubMed]
- Tang Z, Li C, Kang B, et al. GEPIA: a web server for cancer and normal gene expression profiling and interactive analyses. Nucleic Acids Res 2017;45:W98-W102. [Crossref] [PubMed]
- Ding C, Shan Z, Li M, et al. Characterization of the fatty acid metabolism in colorectal cancer to guide clinical therapy. Mol Ther Oncolytics 2021;20:532-44. [Crossref] [PubMed]
- Wu T, Hu E, Xu S, et al. clusterProfiler 4.0: A universal enrichment tool for interpreting omics data. Innovation (Camb) 2021;2:100141. [Crossref] [PubMed]
- Chang JT, Chan SH, Lin CY, et al. Differentially expressed genes in radioresistant nasopharyngeal cancer cells: gp96 and GDF15. Mol Cancer Ther 2007;6:2271-9. [Crossref] [PubMed]
- Matsuyama A, Inoue H, Shibuta K, et al. Hepatoma-derived growth factor is associated with reduced sensitivity to irradiation in esophageal cancer. Cancer Res 2001;61:5714-7. [PubMed]
- Fukuda K, Sakakura C, Miyagawa K, et al. Differential gene expression profiles of radioresistant oesophageal cancer cell lines established by continuous fractionated irradiation. Br J Cancer 2004;91:1543-50. [Crossref] [PubMed]
- Wang T, Tamae D, LeBon T, et al. The role of peroxiredoxin II in radiation-resistant MCF-7 breast cancer cells. Cancer Res 2005;65:10338-46. [Crossref] [PubMed]
- Xu QY, Gao Y, Liu Y, et al. Identification of differential gene expression profiles of radioresistant lung cancer cell line established by fractionated ionizing radiation in vitro. Chin Med J (Engl) 2008;121:1830-7. [Crossref] [PubMed]
- Nakahira S, Nakamori S, Tsujie M, et al. Involvement of ribonucleotide reductase M1 subunit overexpression in gemcitabine resistance of human pancreatic cancer. Int J Cancer 2007;120:1355-63. [Crossref] [PubMed]
- Lai IL, Chou CC, Lai PT, et al. Targeting the Warburg effect with a novel glucose transporter inhibitor to overcome gemcitabine resistance in pancreatic cancer cells. Carcinogenesis 2014;35:2203-13. [Crossref] [PubMed]
- Zalacain M, Cundliffe E. Cloning of tlrD, a fourth resistance gene, from the tylosin producer, Streptomyces fradiae. Gene 1991;97:137-42. [Crossref] [PubMed]
- Tan Y, Li X, Tian Z, et al. TIMP1 down-regulation enhances gemcitabine sensitivity and reverses chemoresistance in pancreatic cancer. Biochem Pharmacol 2021;189:114085. [Crossref] [PubMed]
- Pattabiraman DR, Weinberg RA. Tackling the cancer stem cells - what challenges do they pose? Nat Rev Drug Discov 2014;13:497-512. [Crossref] [PubMed]
- Wang WJ, Wu MY, Shen M, et al. Cantharidin and norcantharidin impair stemness of pancreatic cancer cells by repressing the β-catenin pathway and strengthen the cytotoxicity of gemcitabine and erlotinib. Int J Oncol 2015;47:1912-22. [Crossref] [PubMed]
- Bocci F, Gearhart-Serna L, Boareto M, et al. Toward understanding cancer stem cell heterogeneity in the tumor microenvironment. Proc Natl Acad Sci U S A 2019;116:148-57. [Crossref] [PubMed]
- Siegel RL, Miller KD, Jemal A. Cancer statistics, 2019. CA Cancer J Clin 2019;69:7-34. [Crossref] [PubMed]
- Ervin TJ, Clark JR, Weichselbaum RR, et al. An analysis of induction and adjuvant chemotherapy in the multidisciplinary treatment of squamous-cell carcinoma of the head and neck. J Clin Oncol 1987;5:10-20. [Crossref] [PubMed]
- Ensley JF, Jacobs JR, Weaver A, et al. Correlation between response to cisplatinum-combination chemotherapy and subsequent radiotherapy in previously untreated patients with advanced squamous cell cancers of the head and neck. Cancer 1984;54:811-4. [Crossref] [PubMed]
- Catsicas S, Larhammar D, Blomqvist A, et al. Expression of a conserved cell-type-specific protein in nerve terminals coincides with synaptogenesis. Proc Natl Acad Sci U S A 1991;88:785-9. [Crossref] [PubMed]
- Selak S, Paternain AV, Aller MI, et al. A role for SNAP25 in internalization of kainate receptors and synaptic plasticity. Neuron 2009;63:357-71. [Crossref] [PubMed]
- Lau CG, Takayasu Y, Rodenas-Ruano A, et al. SNAP-25 is a target of protein kinase C phosphorylation critical to NMDA receptor trafficking. J Neurosci 2010;30:242-54. [Crossref] [PubMed]
- Chen P, Wang W, Wong SW, et al. RUNDC3A regulates SNAP25-mediated chemotherapy resistance by binding AKT in gastric neuroendocrine carcinoma (GNEC). Cell Death Discov 2022;8:296. [Crossref] [PubMed]
- Wang L, Zhou W, Zhong Y, et al. Overexpression of G protein-coupled receptor GPR87 promotes pancreatic cancer aggressiveness and activates NF-κB signaling pathway. Mol Cancer 2017;16:61. [Crossref] [PubMed]
- Ridgway J, Zhang G, Wu Y, et al. Inhibition of Dll4 signalling inhibits tumour growth by deregulating angiogenesis. Nature 2006;444:1083-7. [Crossref] [PubMed]
- Tchekneva EE, Goruganthu MUL, Uzhachenko RV, et al. Determinant roles of dendritic cell-expressed Notch Delta-like and Jagged ligands on anti-tumor T cell immunity. J Immunother Cancer 2019;7:95. [Crossref] [PubMed]
- Kumar S, Nandi A, Singh S, et al. Dll1+ quiescent tumor stem cells drive chemoresistance in breast cancer through NF-κB survival pathway. Nat Commun 2021;12:432. [Crossref] [PubMed]
- Motoki K, Megahed M, LaForgia S, et al. Cloning and chromosomal mapping of mouse ladinin, a novel basement membrane zone component. Genomics 1997;39:323-30. [Crossref] [PubMed]
- Teixeira JC, de Filippo C, Weihmann A, et al. Long-Term Balancing Selection in LAD1 Maintains a Missense Trans-Species Polymorphism in Humans, Chimpanzees, and Bonobos. Mol Biol Evol 2015;32:1186-96. [Crossref] [PubMed]
- Boudreau A, Tanner K, Wang D, et al. 14-3-3σ stabilizes a complex of soluble actin and intermediate filament to enable breast tumor invasion. Proc Natl Acad Sci U S A 2013;110:E3937-44. [Crossref] [PubMed]
- Li J, Wang Z, Tie C. High expression of ladinin-1 (LAD1) predicts adverse outcomes: a new candidate docetaxel resistance gene for prostatic cancer (PCa). Bioengineered 2021;12:5749-59. [Crossref] [PubMed]
- Loveless R, Teng Y. Targeting WASF3 Signaling in Metastatic Cancer. Int J Mol Sci 2021;22:836. [Crossref] [PubMed]
- Nie Y, Liang X, Liu S, et al. WASF3 Knockdown Sensitizes Gastric Cancer Cells to Oxaliplatin by Inhibiting ATG12-Mediated Autophagy. Am J Med Sci 2020;359:287-95. [Crossref] [PubMed]
- Saras J, Franzén P, Aspenström P, et al. A novel GTPase-activating protein for Rho interacts with a PDZ domain of the protein-tyrosine phosphatase PTPL1. J Biol Chem 1997;272:24333-8. [Crossref] [PubMed]
- Miyazaki J, Ito K, Fujita T, et al. Progression of Human Renal Cell Carcinoma via Inhibition of RhoA-ROCK Axis by PARG1. Transl Oncol 2017;10:142-52. [Crossref] [PubMed]
- Qiao Y, Chen J, Lim YB, et al. YAP Regulates Actin Dynamics through ARHGAP29 and Promotes Metastasis. Cell Rep 2017;19:1495-502. [Crossref] [PubMed]
- Hayward A, Ghazal A, Andersson G, et al. ZBED evolution: repeated utilization of DNA transposons as regulators of diverse host functions. PLoS One 2013;8:e59940. [Crossref] [PubMed]
- Tang H, Wei P, Duell EJ, et al. Axonal guidance signaling pathway interacting with smoking in modifying the risk of pancreatic cancer: a gene- and pathway-based interaction analysis of GWAS data. Carcinogenesis 2014;35:1039-45. [Crossref] [PubMed]
- Liu D, Hao Q, Li J, et al. ZBED2 expression enhances interferon signaling and predicts better survival of estrogen receptor-negative breast cancer patients. Cancer Commun (Lond) 2022;42:663-7. [Crossref] [PubMed]
- Chessler SD, Lernmark A. Alternative splicing of GAD67 results in the synthesis of a third form of glutamic-acid decarboxylase in human islets and other non-neural tissues. J Biol Chem 2000;275:5188-92. [Crossref] [PubMed]
- Akbarian S, Huang HS. Molecular and cellular mechanisms of altered GAD1/GAD67 expression in schizophrenia and related disorders. Brain Res Rev 2006;52:293-304. [Crossref] [PubMed]
- Tsuboi M, Kondo K, Masuda K, et al. Prognostic significance of GAD1 overexpression in patients with resected lung adenocarcinoma. Cancer Med 2019;8:4189-99. [Crossref] [PubMed]
- Jia CM, Tian YY, Quan LN, et al. miR-26b-5p suppresses proliferation and promotes apoptosis in multiple myeloma cells by targeting JAG1. Pathol Res Pract 2018;214:1388-94. [Crossref] [PubMed]
- Zhao S, Xiao X, Sun S, et al. MicroRNA-30d/JAG1 axis modulates pulmonary fibrosis through Notch signaling pathway. Pathol Res Pract 2018;214:1315-23. [Crossref] [PubMed]
- Yang Z, Guo L, Liu D, et al. Acquisition of resistance to trastuzumab in gastric cancer cells is associated with activation of IL-6/STAT3/Jagged-1/Notch positive feedback loop. Oncotarget 2015;6:5072-87. [Crossref] [PubMed]
- Kim EJ, Kim SO, Jin X, et al. Epidermal growth factor receptor variant III renders glioma cancer cells less differentiated by JAGGED1. Tumour Biol 2015;36:2921-8. [Crossref] [PubMed]
- Lytle NK, Barber AG, Reya T. Stem cell fate in cancer growth, progression and therapy resistance. Nat Rev Cancer 2018;18:669-80. [Crossref] [PubMed]
- Nassar D, Blanpain C. Cancer Stem Cells: Basic Concepts and Therapeutic Implications. Annu Rev Pathol 2016;11:47-76. [Crossref] [PubMed]
- Sethi N, Dai X, Winter CG, et al. Tumor-derived JAGGED1 promotes osteolytic bone metastasis of breast cancer by engaging notch signaling in bone cells. Cancer Cell 2011;19:192-205. [Crossref] [PubMed]
- Choi JH, Park JT, Davidson B, et al. Jagged-1 and Notch3 juxtacrine loop regulates ovarian tumor growth and adhesion. Cancer Res 2008;68:5716-23. [Crossref] [PubMed]
- Xing F, Kobayashi A, Okuda H, et al. Reactive astrocytes promote the metastatic growth of breast cancer stem-like cells by activating Notch signalling in brain. EMBO Mol Med 2013;5:384-96. [Crossref] [PubMed]
- Kaufman B, Shapira-Frommer R, Schmutzler RK, et al. Olaparib monotherapy in patients with advanced cancer and a germline BRCA1/2 mutation. J Clin Oncol 2015;33:244-50. [Crossref] [PubMed]
- Di Federico A, Tateo V, Parisi C, et al. Hacking Pancreatic Cancer: Present and Future of Personalized Medicine. Pharmaceuticals (Basel) 2021;14:677. [Crossref] [PubMed]