A risk model constructed using 14 N6-methyladenosine-related lncRNAs as a new prognostic marker that correlates with the immunomodulatory effect and drug sensitivity in colorectal cancer
Highlight box
Key findings
• We developed a risk model constructed by 14 m6A-related lncRNAs serves as a new prognostic marker and correlates with immunomodulatory effect and drug sensitivity in CRC.
What is known and what is new?
• Although some models of m6A-related lncRNA had been reported in CRC, most had only been validated for prognostic purposes, which may be a limitation.
• In the ensuing analyses, we added the verification of its grouping ability. Further, we investigated the potential regulatory mechanisms of the model while uncovering its relevance to immune function and drug sensitivity.
What is the implication, and what should change now?
• Our study developed a valuable model and provided a comprehensive account of the implications of the constructed model. More biological research is needed in the future to deeply understand the mechanisms through m6A-related lncRNA affect prognosis and drug sensitivity in patients with CRC.
Introduction
In the latest global cancer statistics, colorectal cancer (CRC) ranks firmly in third in incidence among 36 cancers. It remains the most common gastrointestinal malignancy in both males and females worldwide. Conventional treatments such as surgery, chemotherapy, and radiotherapy, can be applied to treat CRC. Nonetheless, its mortality rate remains high, ranking second (1), and recurrence and metastasis contribute to a cumulative 5-year survival rate of only 60.8% for patients with CRC (2). The emerging targeted therapies and immunotherapy, while valuable, are only highly effective in select patients (3). In addition, the frequency of mutations in tumors (4,5) and tumor drug resistance (6) are common factors affecting patients’ prognoses. Therefore, in clinical practice, there is a need to identify new factors that may affect the prognosis of those with CRC and develop effective treatment strategies. In particular, the genomic characteristics of CRC can be exploited in developing effective treatments and predicting the risk of recurrence and survival.
N6-methyladenosine (m6A) methylation modification is present in various types of RNA. Since its discovery in the 1920s, m6A has been considered to be a common modification in messenger RNA (mRNA) and noncoding RNA. It affects the splicing, translation, stability, and epigenetic features of some noncoding RNAs (7,8). The formation of m6A is a reversible and dynamic RNA epigenetic process in mammalian cells. The process is regulated by the reader (m6A binding protein), writer (methyltransferase), and eraser (demethylase) proteins responsible for m6A function, methylation, and demethylation, respectively (9). An increasing amount of evidence suggests that the abnormal expression and regulation of m6A are related to the progression of CRC. YTHDC2 (an m6A binding protein) was found to be upregulated in CRC, and it promoted metastasis by inducing the expression of metastasis-related genes (10). Additionally, knockdown of the methyladenosine reader IGF2BP3 was reported to result in the inhibition of DNA replication, cell proliferation, and angiogenesis in CRC (11). Modifications induced by the m6A writer m6A methyltransferase-like 3 (METTL3) maintain the stability of chromo box protein homolog 8 (CBX8), thereby increasing the stemness of CRC and reducing its chemical sensitivity (12). Besides, m6A modification could increase the expression of PD-L1, thus playing an important role in tumor immune escape. Deletion of methyltransferases METTL3 and METTL14 inhibited this modification and enhanced the response to anti PD-1 treatment (13).
Numerous studies have shown that long noncoding RNAs (lncRNAs) are non-protein-coding genes with complex structures and a length of over 200 bp, and these have been proven to play a critical regulatory role in epigenetics (14). lncRNAs participates in various biological processes such as cell growth and differentiation, as well as the occurrence and development of tumors. Abnormal lncRNA expression is closely related to CRC. Huang et al. (15) found that SNHG15 could independently affect the prognosis of patients with CRC and act as a cancer-promoting factor. Furthermore, overexpression of RP11-708H21.4 was found to cause CRC cell cycle arrest in the G0 and G1 phase; inhibit its proliferation, migration, and invasion; and, additionally, improve the therapeutic efficacy of fluorouracil (5-FU) (16). Finally, the lncRNA CCAT2 was reported to promote the development of CRC by inducing chromosome instability (17).
The role of m6A in modifying lncRNAs and thereby regulating their expression to influence tumor development (18), immune regulation (19), and drug resistance (20) has been confirmed. m6A modification led to LINC00958 upregulation, which aggravated HCC malignant phenotypes in vitro and in vivo (21). The m6A dependent lnc-Dpf3 feedback-controlled DC migration and inflammatory response (22). In esophageal cancer, m6A modification exacerbated the enrichment of lncRNA CASC8, thereby promoting cancer invasiveness and chemical resistance (23). In addition, m6A-related lncRNAs could help determine the prognosis to a certain extent (24). However, the significance of m6A-related lncRNAs in CRC has not been comprehensively examined. Therefore, in this study, data from 446 patients with CRC were analyzed using bioinformatics and statistical tools to construct an optimal risk model consisting of 14 m6A-related lncRNAs. Through a series of analyses, we validated the constructed risk model’s predictive accuracy, reliability, clinical applicability, and grouping ability. Additionally, we investigated its correlation with tumor mutation burden (TMB), immune function, and drug sensitivity and performed Gene Ontology (GO) enrichment analysis to explore the potential regulatory mechanisms. Finally, to improve clinical practice, we built a nomogram to help physicians predict the overall survival (OS) of patients with CRC. We present this article in accordance with the TRIPOD reporting checklist (available at https://jgo.amegroups.com/article/view/10.21037/jgo-23-412/rc).
Methods
Data acquisition and preprocessing
The transcriptomic and mutation data of patients with CRC were retrieved from The Cancer Genome Atlas (TCGA) database (25). All gene IDs in the TCGA-CRC datasets were matched with the Genome Reference Consortium Human genome build 38 (GRCh38) from GENCODE to distinguish mRNAs and lncRNAs. The corresponding clinical information was also downloaded from this database. Patients with no survival information in their clinical data were excluded from further evaluation to reduce the statistical deviation. Ultimately, TCGA datasets of 446 patients with CRC were obtained for in-depth analysis in this study. The study was conducted in accordance with the Declaration of Helsinki (as revised in 2013).
Identification of m6A-related lncRNAs in CRC
From previous studies, 23 regulatory factors acting as switches in m6A methylation were identified for subsequent analysis (26,27). The genes were classified into 3 categories according to their roles in the methylation process, including 13 readers (YTHDC1, YTHDC2, YTHDF1, YTHDF2, YTHDF3, HNRNPC, FMR1, LRPPRC, HNRNPA2B1, IGFBP1, IGFBP2, IGFBP3, RBMX), 8 writers (METTL3, METTL14, METTL16, WTAP, VIRMA, ZC3H13, RBM15, RBM15B), and 2 erasers (FTO and ALKBH5). We implemented gene coexpression analysis (|Pearson R| >0.5 and P<0.001), through which the lncRNAs associated with the m6A methylation regulation genes in TCGA-CRC datasets were mined. These lncRNAs were defined as m6A-related lncRNAs (available online: https://cdn.amegroups.cn/static/public/jgo-23-412-1.xlsx).
Construction and verification of the risk model based on m6A-related lncRNAs in CRC
We randomly assigned 446 patients to the training and test groups at a 1:1 ratio, and further compared the information on patients included in both groups (Table 1), which is sufficient to demonstrate comparability. We constructed the risk model using documentation from patients in the training group and validated it in the test group and the whole group. Afterward, univariate Cox regression analysis was carried out to determine the m6A-related lncRNAs with prognostic values in combination with the training patient’ clinical data. Hazard ratios (HRs) were used classify these prognostic lncRNAs into risk (HR >1) or protective (HR <1) indicators. We subsequently conducted least absolute shrinkage and selection operator (LASSO) Cox regression analysis to further identify 14 appropriate lncRNAs to build the optimal risk model. Based on the obtained risk coefficients of these lncRNAs (Table S1), the risk score of each patient was calculated as follows:
Table 1
Variables | Training group | Test group | Total | P value |
---|---|---|---|---|
Age | 0.7130 | |||
<65 | 90 | 93 | 183 | |
≥65 | 134 | 129 | 263 | |
Gender | 0.2991 | |||
Female | 101 | 111 | 212 | |
Male | 123 | 111 | 234 | |
Stage | 0.2974 | |||
Stage I–II | 120 | 130 | 250 | |
Stage III–IV | 100 | 85 | 185 | |
Unknown | 4 | 7 | 11 | |
T | 0.5986 | |||
T1–2 | 41 | 45 | 86 | |
T3–4 | 183 | 177 | 360 | |
N | 0.2399 | |||
N0 | 127 | 138 | 265 | |
N1 | 97 | 84 | 181 | |
M | 0.6021 | |||
M0 | 161 | 168 | 329 | |
M1 | 34 | 27 | 61 | |
Unknown | 29 | 27 | 56 |
T, tumor; N, node; M, metastasis.
where Coefi represents the coefficients, and Xi is the fragments per kilobase of exon model per million reads mapped (FPKM) value of each modeled lncRNA.
We thus calculated the median risk score value of the patients in the training group to define whether the patients belonged to the high- or low-risk group (available online: https://cdn.amegroups.cn/static/public/jgo-23-412-2.xlsx). With additional use of patients’ clinical prognostic data, we analyzed the survival differences between patients in the high- and low-risk groups. Subsequently, we plotted the risk curve, survival status map, and risk heat map. All the above analyses were accomplished synchronously in the test group and the whole group.
Independent prognostic and precision verification analysis of the risk model
To determine whether the constructed model could be used as a prognostic factor independently of other clinical traits, we conducted univariate and multivariate Cox regression analyses based on the information of those patients with CRC in TCGA database. Next, we plotted the risk model’s receiver operating characteristic (ROC) curves for predicting patient survival at 1, 3, and 5 years. Moreover, we compared the ROC curves and concordance indexes (C-indexes) of the risk score with other clinical variables (age, gender, stage) to verify the reliability and precision of the risk model.
Construction and verification of the nomogram
The risk model was subsequently combined with additional independent prognostic factors (age and stage) to construct a nomogram using the “rms” package in R software (The R Foundation of Statistical Computing). Meanwhile, the nomogram’s calibration curves for predicting the 1-, 3- and 5-year OS in patients with CRC were plotted to assess the consistency between the real outcomes and the survival predicted by the nomogram.
Clinical applicability and grouping verification of the risk model
The clinical applicability of the model was evaluated. We distinguished patients in different clinical subgroups (female and male, age <65 years and age ≥65 years, stage T1–2 and stage T3–4, stage N0 and stage N1–2, and stage M0 and stage M1) and then performed a differential survival analysis in each subgroup between the high-risk and low-risk groups to ascertain whether the constructed risk model could be applied to patients in different clinical subgroups. Additionally, using principal component analysis, we verified the ability of the modeled lncRNAs to distinguish between the high- and low-risk groups of patients.
GO enrichment analysis and immuno-reactivity of the risk model
Using the “limma” package in R software, we obtained the differentially expressed genes (DEGs) (Table S2) between patients in the high and low-risk groups. GO gene enrichment analysis related to biological process (BP), molecular function (MF), and cellular component (CC) was conducted us to clarify the potential functions of the risk model. We collated the gene files associated with 13 immune functions and obtained the immune scoring for each sample using single-sample gene set enrichment analysis (ssGSEA). Then, we merged the risk files and immune scoring files, cycled each immune-associated function, and performed statistical analysis to observe which immune functions differed between the high- and low-risk groups. Finally, we calculated the tumor immune dysfunction and exclusion (TIDE) score for patients in the high- and low-risk groups, and based on the scores, we could observe which group of patients had better outcomes from immunotherapy.
Tumor mutation burden analysis for the risk model
We calculated each patient with CRC’s tumor mutation burden (TMB) (available online: https://cdn.amegroups.cn/static/public/jgo-23-412-3.xlsx) in TCGA database via Perl script. The top 15 genes with the most mutations in the high- and low-risk groups and the total mutation frequency were visualized separately with waterfall plots. With the assistance of the “limma” package in R software, we performed a differential analysis of the TMB between the high- and low-risk groups. The result was visualized with a violin plot drawn by the “ggpubr” package in R. Based on the TMB of patients obtained from previous calculations, we divided patients with CRC into high- and low-TMB groups. The Kaplan-Meier method was used to calculate the survival differences between the 2 groups and evaluate the association of the risk model combined with TMB on patient survival.
Drug sensitivity analysis for the risk model
To identify potential therapeutic agents using the risk model, we collected information on drug treatments from the Cancer Genome Project (CGP). We used the “pRRophetic” package in the R software to perform a round-robin comparison of drug half maximal inhibitory concentration (IC50) in order to determine which drugs had differing sensitivities in the high-risk versus low-risk groups. The difference results were visualized with box plots drawn by the “ggplot2” package in R (P<0.05).
Statistical analysis
Univariate and LASSO regression analyses were implemented to identify factors affecting the prognosis of patients with CRC and to develop the optimal risk model. Pearson correlation coefficient analysis was used to assess the correlation between m6A-regulated genes and 14 modeled lncRNAs. Kaplan-Meier curves and log-rank tests were used to calculate the differences in OS between different subgroups, while the chi-squared test was used to determine the distribution of the 14 modeled lncRNAs in the high- and low-risk groups. The results of the above statistical analyses were completed using R software (version 4.0.4; https://cran.r-project.org/). The statistical values in this study were 2-sided, and a P value <0.05 was considered to be statistically significant.
Results
Identification of m6A-related lncRNAs in CRC
The flowchart in Figure 1A displays the construction and verification process of the risk model based on the m6A-related lncRNAs and subsequent analyses. We downloaded transcriptomic data from TCGA-GDC (https://portal.gdc.cancer.gov/) for CRC. Expression files for 14,086 lncRNAs and 23 m6A-regulated genes were used for the analysis work in this study. Based on the gene co-expression analysis network, we identified those lncRNAs that were significantly associated with the expression of m6A-regulated genes as m6A-related lncRNAs (|Pearson R| >0.5 and P<0.001) and visualized the results in a Sankey diagram (Figure 1B).
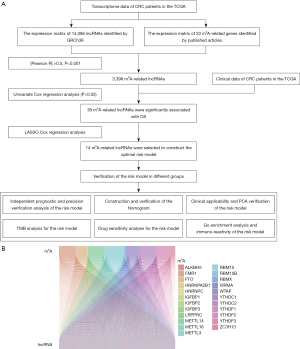
Construction of a valuable risk model based on m6A-related lncRNAs in CRC
With reference to the clinical information of the patients (data from TCGA-GDC), we performed a univariate Cox regression analysis of previously obtained m6A-related lncRNAs in the training group, of which 38 were significantly associated with OS in CRC (Figure 2A). We then proceeded to perform LASSO Cox regression analysis and further selected 14 m6A-related lncRNAs (Figure 2B,2C) to construct the optimal risk model. In addition, we visualized the expression correlation of 14 modeled m6A-related lncRNAs with 23 m6A-regulated genes (Figure 2D).
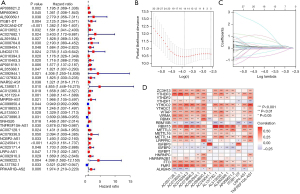
Verification of the risk model in the different groups
We calculated patient’ risk scores based on the resulting risk coefficients of the 14 lncRNAs. They were then classified into a high-risk and low-risk group according to the median risk score of patients in the training group. The Kaplan-Meier survival analysis showed that in the training group, patients in the high-risk group had a significantly worse prognosis than did those in the low-risk group (Figure 3A1). Risk curves, survival status diagrams, and risk distribution heat maps were subsequently drawn. The results demonstrated that the frequency of deaths increased significantly with the increase in patient’ risk scores (Figure 3A2,3A3). Moreover, in the heat maps, we observed the expression of AP006621.2, AL590369.1, ITGB1-DT, AC013652.1, AC016394.3, AC092944.1, AL513550.1, AC007128.1, AC245041.1, and LRP4-AS1 to be more abundant in patients with high risk, suggesting that they may act as risk factors in CRC. In contrast, AC099850.4, AC073896.3, TNFRSF10A-AS1, and AL137782 were more concentrated in patients with low risk, indicating their high expression may have a beneficial effect (Figure 3A4). We performed the same analyses as in the training group to verify the risk model in both the test group (Figure 3B) and the whole group (Figure 3C). The final results revealed that the trends were consistent with those of the training group.
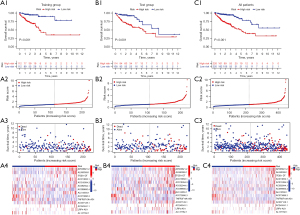
Independent prognostic and precision verification analysis of the risk model
Using univariate Cox regression analysis, we probed the relationship between the clinical traits, risk scores, and OS of patients with CRC (Figure 4A). Multivariate Cox regression analysis was then performed to verify whether the risk model developed in this study could be an independent predictor of prognosis for patients with CRC (Figure 4B). The results showed that the P value of the risk score (univariate Cox regression analysis: HR 1.297, 95% CI: 1.192−1.41; multivariate Cox regression analysis: HR 1.189, 95% CI: 1.090−1.298) was less than 0.001, which demonstrated that the risk model developed in our study could predict the prognosis of patients with CRC independently of other clinical traits. In addition, our results simultaneously identified age and stage as independent predictors of prognosis in CRC (P<0.001). Next, we applied the area under the ROC curve (AUC) of the risk score and the C-index to verify the precision of the risk model in predicting the prognosis of patients with CRC. The AUCs of our risk model were 0.715, 0.763, and 0.749 for survival at 1, 3, and 5 years, respectively, indicating the high accuracy of our model (Figure 4C). Furthermore, the AUC of our risk model was the most significant compared to the other independent prognostic factors (Figure 4D). Similar findings were evident when we plotted the C-index curve (Figure 4E), demonstrating that our model had the best precision in predicting the prognosis of patients with CRC.
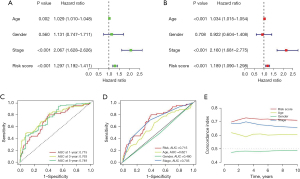
Construction and verification of the nomogram
From the above analysis, age, stage, and risk score were identified as independent prognostic factors for CRC, which we used to construct the nomogram, a visual, quantitative tool to predict patient’ OS. The nomogram showed the predicted outcome of the patient with ID TCGA-CM-6164, a low-risk patient aged 46 years with stage II disease (Figure 5A). By matching the information, the final expected survival rates for this patient were 0.988, 0.971, and 0.952 at 1, 3, and 5 years, respectively. According to the clinical information collected, the patient was still alive at the end of the data count, with a tracked survival time of 883 days, which is consistent with our predicted outcome. In parallel, we plotted the calibration curves for the nomogram to predict OS in patients with CRC (Figure 5B). The results showed that the predicted calibration curves at 1, 3, and 5 years were in good agreement with the standard curve, verifying that the nomogram had good prediction accuracy.
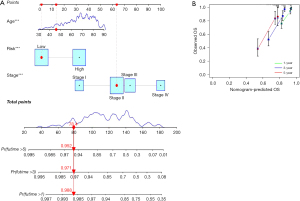
Clinical applicability and grouping verification of the risk model
We then classified patients by age, gender, and tumor- node-metastasis (TNM) stages to determine the population to which the risk model could be applied. Kaplan-Meier survival analysis showed that patients identified as high-risk in all subgroups had a significantly worse prognosis than did low-risk patients (P<0.001) (Figure 6A-6E), which demonstrated the solid clinical applicability of our risk model. Moreover, we used principal component analysis (PCA) to verify whether the 14 lncRNAs involved in the risk model could better classify patients as high and low risk. As shown in Figure 6F-6I, the 14 lncRNAs in the risk model distinguished more clearly between high- and low-risk patients than did the all genes, the m6A-related genes, and the m6A-related lncRNAs.
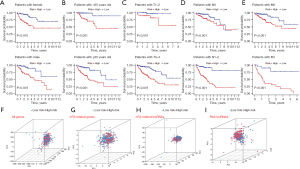
GO enrichment analysis and immuno-reactivity of the risk model
In the following step in the study, we further investigated the impact of the potential biological function of the risk model. We first identified 18 genes significantly differentially expressed between patients the high- and low-risk groups (|log fold change| >1 and false-discovery rate <0.05) and then used GO enrichment analysis to determine which biological functions they were enriched in, thus clarifying the underlying physical processes involved in the risk model. According to the analysis, the model was significantly correlated with humoral immune response, aging, response to chemokine, and positive regulation of neutrophil migration in the enrichment of BPs. Among the CCs, we observed keratin filament. The MF enrichment further suggested that the risk model was associated with signaling receptor activator activity, peptidoglycan binding, structural constituent of cytoskeleton, and cyclin-dependent protein serine/threonine kinase inhibitor activity (Figure 7A).
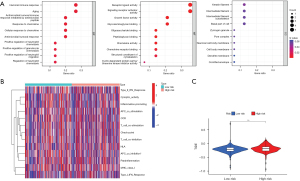
In the abovementioned results, there were correlations between the risk model and immunity. Therefore, to investigate whether the risk model had promising applications in immunotherapy, we analyzed the differences in immune function between patients in the high- and low-risk groups. As demonstrated in the heatmap in Figure 7B, there was a statistical difference in the distribution of antigen presenting cell (APC) co-inhibition among the 13 immune-related functions between the high- and low-risk groups. There was also a significant difference between the 2 groups in the TIDE scores, evincing the efficacy of immunotherapy (Figure 7C). Patients in the high-risk group had higher TIDE scores, indicating that in patients in the high-risk group, there is a greater potential for immune escape and lower efficacy of immunotherapy than in patients in the low-risk group. The results thus indicated that the risk model may be particularly valuable in relation to immunotherapy.
TMB analysis of the risk model
In addition, the waterfall plots (Figure 8A,8B) were created to clarify whether there was a difference in TMB between patients in the high-risk and low-risk groups and show the mutation frequencies and mutation information for the 15 genes with the highest mutation frequencies in the 2 groups, respectively. The results showed that patients in the high-risk group had a slightly higher frequency of mutations than did those in the low-risk group; however, the violin plot showed that this difference was not statistically significant (Figure 8C). However, further survival analysis suggested that patients in the high TMB group had a statistically significant shorter OS than did those in the low TMB group (P=0.021) (Figure 8D). Furthermore, when we considered the bivariate effect on patient prognosis, patients with both low risk and low TMB had the best prognosis (P<0.001) (Figure 8E). These results suggest that our risk model correlated with TMB and could consistently predict the prognosis of patients with CRC.
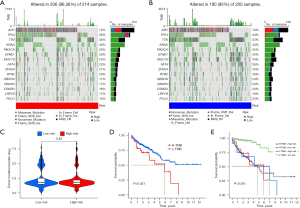
Drug sensitivity analysis for the risk model
Finally, we performed a drug sensitivity analysis based on a risk model that provided a theoretical basis for treatment recommendations. We used the “pRRophetic” package in R, which can detect the responsiveness of the expression matrix to drugs by combining information on drug sensitivity and risk expression to determine which drugs have different sensitivities between the high- and low-risk groups. The results showed that A-443654, AS605240, bortezomib, erlotinib, HG-6-64-1, LAQ824, phenformin, salubrinal, sorafenib, WH-4-023, WZ3105, and YM155 had lower IC50 values in patients in the high-risk group, indicating that patients in the high-risk group were more sensitive to these drugs (Figure 9A). In contrast, BAY 61-3606, BMS345541, FMK, FR-180204, FTI-277, gemcitabine, Genentech Cpd 10, ispinesib mesylate, JW-7-52-1, LY317615, NPK76-l1-72-1, NSC-87877, NSC-207895, QL-Xll-47, rapamycin, ruxolitinib, STF-62247, VX-11e, XL-184, XMD14-99, and Z-LLNle-CHO had lower IC50 values in patients in the low-risk group, suggesting that these drugs would have better therapeutic effects in patients in the low-risk group (Figure 9B).
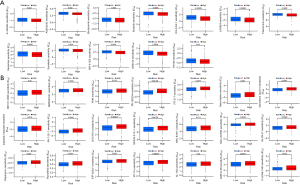
Discussion
CRC is a common malignant tumor for which there are many treatment options, such as surgery, chemotherapy, radiotherapy, targeted therapy, immunotherapy, and others. However, there is a lack of personalized treatment during actual diagnosis and treatment, and the overall mortality rate of patients with CRC remains high due to recurrence and metastasis (2). Finding novel targeted therapeutic molecules or obtaining accurate prognoses of patients with CRC may be helpful for individual-centric clinical decision-making, thus increasing the clinical benefits to patients.
lncRNA has a specific and complex secondary spatial structure, which can provide ample binding sites for various molecules (28). It has been reported that lncRNA is involved in chromatin modification (29), transcriptional activation (30), transcriptional interference (31), and regulation of cancer (32). In addition, m6A is considered the most common epigenetic modification of RNA, which also plays a critical regulatory role in cancer biology. A few recent, studies found that some m6A regulators can control tumor occurrence and development by modifying specific lncRNA. For example, Xue et al. indicated that METTL3 induced a high expression of ABHD11-AS1 in non-small cell lung cancer, thereby promoting proliferation and the Warburg effect (33). METTL3 regulator also upregulated LINC00958 by promoting the stability of its RNA transcript, which fosters the animation of breast cancer cells (34). As a cofactor of IGF2BP3, the lncRNA DMDRMR stabilizes the target gene in an m6A-dependent manner, thus playing an essential carcinogenic role in clear cell renal cell carcinoma (ccRCC) (35). However, the role of m6A-related lncRNAs in CRC progression has not been adequately studied.
We therefore aimed to discover whether m6A-related lncRNAs play a unique regulatory role in CRC to identify potential therapeutic targets or prognostic indicators. We screened 14 suitable m6A-related lncRNAs to construct the optimal risk model and validated their value and reliability. The correlation between the constructed risk model and immune regulation, TMB, and drug sensitivity was further investigated, and finally, the potential biological processes involved in this model were identified. Through this study, we hope to provide an effective prognostic prediction tool for patients with CRC and provide a theoretical basis for clinicians to evaluate the disease status and select appropriate treatment methods.
Our study involved 446 patients with CRC identified from TCGA database. Through univariate Cox regression analysis, we first determined 38 m6A-related lncRNAs with prognostic values. Then, 14 prognostic m6A-related lncRNAs (AP006621.2, AL590369.1, ITGB1-DT, AC013652.1, AC016394.3, AC092944.1, AC099850.4, AL513550.1, AC073896.3, TNFRSF10A-AS1, AC007128.1, AC245041.1, LRP4-AS1, and AL137782.1) were further screened to establish the optimal risk model using LASSO Cox regression analysis for predicting the prognosis of patients with CRC. Some lncRNAs found in our study have also been reported in previous studies. For instance, AP006621.2 was recognized to independently predict the prognosis of ccRCC (36). ITGB1-DT can act as a carcinogenic lncRNA by activating the ITGB1-DT/ITGB1/Wnt/β-catenin/MYC pathway (37), which is related to prognosis in lung adenocarcinoma (LUAD) and could be used as an independent survival predictor (38). The targeted competition between AC099850.4 and miR-664b-3p is important in advanced serous ovarian cancer (39). AC007128.1 is a key factor affecting the survival of patients with esophageal cancer (40). In addition, AL513550.1 is considered to be an immune-related lncRNA and may predict the prognosis of patients with colon cancer (41). Interestingly, the autophagy-related lncRNAs, AC073896.3 and TNFRSF10A-AS1, were shown to be protective factors in CRC, which is consistent with our results. The expression of these 2 kinds of lncRNAs was more common in the low-risk group and positively correlated with a good prognosis, indicating that our prediction was reliable. Nevertheless, of these 14 lncRNAs, AL590369.1, AC016394.3, AC092944.1, and LRP4-AS1 have never been reported, even for model building. Our study is the first to mine these molecules, serving as a foundation for future research. Consequently, more studies need to demonstrate their role and mechanism in tumors.
Subsequently, we validated the predictive ability of the risk model constructed based on the screened 14 m6A-related lncRNAs for patients with CRC. First, patients included in the study were randomized equally into the training group and test group, and all analyses were matched in the test group and the whole group for synchronization verification. As defined by the median risk score of patients in the training group, patients with CRC were distinguished into high- and low-risk groups. Fortunately, the results of all groups were consistent. High-risk patients had significantly worse OS than did low-risk patients. Then, through combination with the clinical data, the risk score was confirmed to be an independent prognostic factor in CRC. The ROC and C-index analysis further indicated that the risk model was the best predictor. The characteristics of advanced age and late tumor stage were also strongly relevant to poor prognosis, consistent with previous research (42). Following this, we integrated the risk score, age, and stage to build a nomogram. Calibration curves showed that the nomogram-predicted 1-, 3-, and 5-year survival rates of patients with CRC were close to those of the actual clinical situation. Overall, the 14 m6A-related lncRNAs identified in this study present strong prognostic ability and have potential value for further exploration of the mechanism underlying the occurrence and development of CRC. The nomogram also shows an excellent capability to predict the survival rate of patients. For clinicians, it is a good practical tool for clinical application and can provide a reference for analyzing patients’ conditions and formulating appropriate treatment strategies. The survival differences between the high- and low-risk groups in the different subgroups and the principal component analysis further validated our constructed risk model's broad clinical applicability and clear grouping ability.
To further explore the potential biological functions of the risk model, we screened the differentially expressed genes from the high- and low-risk patients for GO enrichment analysis. These differential genes were enriched in the functions related to tumorigeneses, such as response to chemokine, signaling receptor activator activity, structural constituent of cytoskeleton, and cyclin-dependent protein serine/threonine kinase inhibitor activity. In addition, they were also mainly enriched in immune-related functions such as peptidoglycan binding, humoral immune response, and positive regulation of neutrophil migration. Peptidoglycan is an immune enhancer of the human immune system, stimulating immune cells to release immunomodulatory substances such as tumor necrosis factor α (TNF-α), interleukins (ILs; IL-1, IL-6, IL-8, IL-12), and interferon α. With this in mind, we went on to investigate the relevance of this model for immunotherapy.
Immunotherapy is efficacious for certain refractory patients in a variety of cancers (43,44), but there is no clear delineation of the population for which it is indicated. In our study, patients in the high- and low-risk groups differed significantly in immune function APC coinhibition, which is an important factor in suppressing immune function. Moreover, the difference in TIDE scores in the 2 groups further validated their different sensitivity to immune checkpoint inhibitors (ICIs). Patients in the high-risk group had a greater probability of immune escape, and their immunotherapy outcomes were worse. Therefore, the risk model we constructed could provide a reference basis for determining whether patients with CRC should receive immunotherapy or not.
TMB is an emerging biomarker for predicting response to ICI therapy (45). Tumor cells in patients with high TMB produce a large number of a variety of antigens that provide the immune system with the opportunity to recognize them and, therefore, a greater the probability of killing these tumor cells after the application of ICIs (46). Unfortunately, TMB did not show a difference between patients in the high and low-risk groups in this study. We further analyzed whether OS differences existed between patients with high and low TMB. The results showed that patients with high TMB had a significantly worse prognosis than did those with low TMB and that patients with both low TMB and low risk had the best prognosis. Therefore, TMB could be used as a prognostic indicator for the survival of patients with CRC together with our risk model.
Finally, we investigated the relationship between the constructed model and the sensitivity of other anticancer therapeutic agents, including chemotherapeutic and targeted therapeutic agents. The results showed that the 12 drugs had lower IC50 values in the high-risk group, suggesting that these 12 drugs, including A-443654 and sorafenib, are more suitable for patients in the high-risk group. In comparison, 21 drugs, including gemcitabine and rapamycin, had lower IC50 values in the low-risk group, suggesting that the patients in the low-risk group were more sensitive to these 21 drugs and experienced better antitumor effects with these drugs, which may also be one of the reasons for the worse prognosis of patients in the high-risk group. In short, our model may also help to select treatment options for patients with CRC.
Compared to other models, our model has a more comprehensive set of assessment metrics. We conducted a multivariate comparison and demonstrated that the model we developed had the greatest predictive power relative to other prognostic factors. Aside from the test group, we also added the entire population of patients to validate the model and used PCA to verify that the model is capable of discriminating among the population as a whole. With the intention of examining the constructed model in relation to immune function, we have also added an assessment of the TMB (which has been considered a marker of immunotherapy in recent years) and found that it can be used as a helpful prognostic predictor in conjunction with our model. As a result of our study, we were able to discover all the drugs that the model could distinguish, including chemotherapies, targeted therapies, etc., and not only immunotherapy, thus providing a reference for the clinical treatment of colorectal cancer and for future research. It is important to note that the therapeutic drugs they recommend vary considerably as a result of the different molecules involved in constructing the model. Thus, in summary, the model we have constructed is more comprehensive than other models and has proven to be a superior predictor of prognosis for colorectal cancer patients compared to other factors.
The important role of m6A-related lncRNAs in different cancers is now gradually being explored (47,48). In this study, we developed a prognostic risk model and validated its accuracy and reliability from various aspects. In addition, we investigated the potential biological significance of this risk model and its relationship with immunotherapy, chemotherapy, targeted therapies, and TMB, providing a comprehensive account of the implications of the constructed risk model. Nevertheless, there are still some limitations to this study. Although the training and test groups were different populations, the model was only validated in TCGA database. More external data are needed in the future to assess its accuracy and utility. In addition, more biological studies are needed regarding the specific mechanisms of how the 14 m6A-related lncRNAs affect the prognosis and differences in drug sensitivity in patients with CRC.
Conclusions
In any case, our study identified a risk model based on 14 m6A-related lncRNAs that could be the most appropriate biomarker independently of other clinical traits for predicting the prognosis of patients with CRC. Moreover, this study may serve as the basis for further studies on regulating CRC using m6A-related lncRNAs.
Acknowledgments
Funding: The present study was supported by the Natural Science Foundation of Jiangxi Province (No. 20202BABL206075), and Natural Science Foundation of Health Department of Jiangxi Province (No. 202130151).
Footnote
Reporting Checklist: The authors have completed the TRIPOD reporting checklist. Available at https://jgo.amegroups.com/article/view/10.21037/jgo-23-412/rc
Peer Review File: Available at https://jgo.amegroups.com/article/view/10.21037/jgo-23-412/prf
Conflicts of Interest: All authors have completed the ICMJE uniform disclosure form (available at https://jgo.amegroups.com/article/view/10.21037/jgo-23-412/coif). The authors have no conflicts of interest to declare.
Ethical Statement: The authors are accountable for all aspects of the work in ensuring that questions related to the accuracy or integrity of any part of the work are appropriately investigated and resolved. The study was conducted in accordance with the Declaration of Helsinki (as revised in 2013).
Open Access Statement: This is an Open Access article distributed in accordance with the Creative Commons Attribution-NonCommercial-NoDerivs 4.0 International License (CC BY-NC-ND 4.0), which permits the non-commercial replication and distribution of the article with the strict proviso that no changes or edits are made and the original work is properly cited (including links to both the formal publication through the relevant DOI and the license). See: https://creativecommons.org/licenses/by-nc-nd/4.0/.
References
- Sung H, Ferlay J, Siegel RL, et al. Global Cancer Statistics 2020: GLOBOCAN Estimates of Incidence and Mortality Worldwide for 36 Cancers in 185 Countries. CA Cancer J Clin 2021;71:209-49. [Crossref] [PubMed]
- Kalcan S, Sisik A, Basak F, et al. Evaluating factors affecting survival in colon and rectum cancer: A prospective cohort study with 161 patients. J Cancer Res Ther 2018;14:416-20. [Crossref] [PubMed]
- Picco G, Cattaneo CM, van Vliet EJ, et al. Werner Helicase Is a Synthetic-Lethal Vulnerability in Mismatch Repair-Deficient Colorectal Cancer Refractory to Targeted Therapies, Chemotherapy, and Immunotherapy. Cancer Discov 2021;11:1923-37. [Crossref] [PubMed]
- Weren RD, Ligtenberg MJ, Kets CM, et al. A germline homozygous mutation in the base-excision repair gene NTHL1 causes adenomatous polyposis and colorectal cancer. Nat Genet 2015;47:668-71. [Crossref] [PubMed]
- Chida K, Kotani D, Masuishi T, et al. The Prognostic Impact of KRAS G12C Mutation in Patients with Metastatic Colorectal Cancer: A Multicenter Retrospective Observational Study. Oncologist 2021;26:845-53. [Crossref] [PubMed]
- Wang Q, Shen X, Chen G, et al. Drug Resistance in Colorectal Cancer: From Mechanism to Clinic. Cancers (Basel) 2022;14:2928. [Crossref] [PubMed]
- Wang X, Lu Z, Gomez A, et al. N6-methyladenosine-dependent regulation of messenger RNA stability. Nature 2014;505:117-20. [Crossref] [PubMed]
- Deng X, Su R, Weng H, et al. RNA N(6)-methyladenosine modification in cancers: current status and perspectives. Cell Res 2018;28:507-17. [Crossref] [PubMed]
- Yang Y, Hsu PJ, Chen YS, et al. Dynamic transcriptomic m(6)A decoration: writers, erasers, readers and functions in RNA metabolism. Cell Res 2018;28:616-24. [Crossref] [PubMed]
- Tanabe A, Tanikawa K, Tsunetomi M, et al. RNA helicase YTHDC2 promotes cancer metastasis via the enhancement of the efficiency by which HIF-1α mRNA is translated. Cancer Lett 2016;376:34-42. [Crossref] [PubMed]
- Yang Z, Wang T, Wu D, et al. RNA N6-methyladenosine reader IGF2BP3 regulates cell cycle and angiogenesis in colon cancer. J Exp Clin Cancer Res 2020;39:203. [Crossref] [PubMed]
- Zhang Y, Kang M, Zhang B, et al. m(6)A modification-mediated CBX8 induction regulates stemness and chemosensitivity of colon cancer via upregulation of LGR5. Mol Cancer 2019;18:185. [Crossref] [PubMed]
- Wang L, Hui H, Agrawal K, et al. m A RNA methyltransferases METTL3/14 regulate immune responses to anti-PD-1 therapy. The EMBO journal 2020;39:e104514. [Crossref] [PubMed]
- de Goede OM, Nachun DC, Ferraro NM, et al. Population-scale tissue transcriptomics maps long non-coding RNAs to complex disease. Cell 2021;184:2633-2648.e19. [Crossref] [PubMed]
- Huang L, Lin H, Kang L, et al. Aberrant expression of long noncoding RNA SNHG15 correlates with liver metastasis and poor survival in colorectal cancer. J Cell Physiol 2019;234:7032-9. [Crossref] [PubMed]
- Sun L, Jiang C, Xu C, et al. Down-regulation of long non-coding RNA RP11-708H21.4 is associated with poor prognosis for colorectal cancer and promotes tumorigenesis through regulating AKT/mTOR pathway. Oncotarget 2017;8:27929-42. [Crossref] [PubMed]
- Chen B, Dragomir MP, Fabris L, et al. The Long Noncoding RNA CCAT2 Induces Chromosomal Instability Through BOP1-AURKB Signaling. Gastroenterology 2020;159:2146-62.e33. [Crossref] [PubMed]
- Li ZX, Zheng ZQ, Yang PY, et al. WTAP-mediated m(6)A modification of lncRNA DIAPH1-AS1 enhances its stability to facilitate nasopharyngeal carcinoma growth and metastasis. Cell Death Differ 2022;29:1137-51. [Crossref] [PubMed]
- Peng L, Pan B, Zhang X, et al. Lipopolysaccharide facilitates immune escape of hepatocellular carcinoma cells via m6A modification of lncRNA MIR155HG to upregulate PD-L1 expression. Cell Biol Toxicol 2022;38:1159-73. [Crossref] [PubMed]
- Zhang H, Wang SQ, Wang L, et al. m6A methyltransferase METTL3-induced lncRNA SNHG17 promotes lung adenocarcinoma gefitinib resistance by epigenetically repressing LATS2 expression. Cell Death Dis 2022;13:657. [Crossref] [PubMed]
- Zuo X, Chen Z, Gao W, et al. M6A-mediated upregulation of LINC00958 increases lipogenesis and acts as a nanotherapeutic target in hepatocellular carcinoma. J Hematol Oncol 2020;13:5. [Crossref] [PubMed]
- Liu J, Zhang X, Chen K, et al. CCR7 Chemokine Receptor-Inducible lnc-Dpf3 Restrains Dendritic Cell Migration by Inhibiting HIF-1α-Mediated Glycolysis. Immunity 2019;50:600-15.e15. [Crossref] [PubMed]
- Wu Q, Zhang H, Yang D, et al. The m6A-induced lncRNA CASC8 promotes proliferation and chemoresistance via upregulation of hnRNPL in esophageal squamous cell carcinoma. Int J Biol Sci 2022;18:4824-36. [Crossref] [PubMed]
- Huang H, Wu W, Lu Y, et al. The development and validation of a m6A-lncRNAs based prognostic model for overall survival in lung squamous cell carcinoma. J Thorac Dis 2022;14:4055-72. [Crossref] [PubMed]
- Cancer Genome Atlas Research Network. The Cancer Genome Atlas Pan-Cancer analysis project. Nat Genet 2013;45:1113-20. [Crossref] [PubMed]
- Chen XY, Zhang J, Zhu JS. The role of m(6)A RNA methylation in human cancer. Mol Cancer 2019;18:103. [Crossref] [PubMed]
- Huang J, Chen Z, Chen X, et al. The role of RNA N (6)-methyladenosine methyltransferase in cancers. Mol Ther Nucleic Acids 2021;23:887-96. [Crossref] [PubMed]
- Isoda T, Moore AJ, He Z, et al. Non-coding Transcription Instructs Chromatin Folding and Compartmentalization to Dictate Enhancer-Promoter Communication and T Cell Fate. Cell 2017;171:103-19.e18. [Crossref] [PubMed]
- Mumbach MR, Granja JM, Flynn RA, et al. HiChIRP reveals RNA-associated chromosome conformation. Nat Methods 2019;16:489-92. [Crossref] [PubMed]
- Gil N, Ulitsky I. Production of Spliced Long Noncoding RNAs Specifies Regions with Increased Enhancer Activity. Cell Syst 2018;7:537-47.e3. [Crossref] [PubMed]
- Latos PA, Pauler FM, Koerner MV, et al. Airn transcriptional overlap, but not its lncRNA products, induces imprinted Igf2r silencing. Science 2012;338:1469-72. [Crossref] [PubMed]
- Xiang JF, Yin QF, Chen T, et al. Human colorectal cancer-specific CCAT1-L lncRNA regulates long-range chromatin interactions at the MYC locus. Cell Res 2014;24:513-31. [Crossref] [PubMed]
- Xue L, Li J, Lin Y, et al. m(6) A transferase METTL3-induced lncRNA ABHD11-AS1 promotes the Warburg effect of non-small-cell lung cancer. J Cell Physiol 2021;236:2649-58. [Crossref] [PubMed]
- Rong D, Dong Q, Qu H, et al. m(6)A-induced LINC00958 promotes breast cancer tumorigenesis via the miR-378a-3p/YY1 axis. Cell Death Discov 2021;7:27. [Crossref] [PubMed]
- Gu Y, Niu S, Wang Y, et al. DMDRMR-Mediated Regulation of m(6)A-Modified CDK4 by m(6)A Reader IGF2BP3 Drives ccRCC Progression. Cancer Res 2021;81:923-34. [Crossref] [PubMed]
- Qi-Dong X, Yang X, Lu JL, et al. Development and Validation of a Nine-Redox-Related Long Noncoding RNA Signature in Renal Clear Cell Carcinoma. Oxid Med Cell Longev 2020;2020:6634247. [Crossref] [PubMed]
- Chang R, Xiao X, Fu Y, et al. ITGB1-DT Facilitates Lung Adenocarcinoma Progression via Forming a Positive Feedback Loop With ITGB1/Wnt/β-Catenin/MYC. Front Cell Dev Biol 2021;9:631259. [Crossref] [PubMed]
- Zeng L, Wang W, Chen Y, et al. A five-long non-coding RNA signature with the ability to predict overall survival of patients with lung adenocarcinoma. Exp Ther Med 2019;18:4852-64. [Crossref] [PubMed]
- Zhao J, Song X, Xu T, et al. Identification of Potential Prognostic Competing Triplets in High-Grade Serous Ovarian Cancer. Front Genet 2020;11:607722. [Crossref] [PubMed]
- Liu H, Zhang Q, Lou Q, et al. Differential Analysis of lncRNA, miRNA and mRNA Expression Profiles and the Prognostic Value of lncRNA in Esophageal Cancer. Pathol Oncol Res 2020;26:1029-39. [Crossref] [PubMed]
- Mu M, Tang Y, Yang Z, et al. Effect of Different Expression of Immune-Related lncRNA on Colon Adenocarcinoma and Its Relation to Prognosis. Biomed Res Int 2020;2020:6942740. [Crossref] [PubMed]
- Ponz de Leon M, Sant M, Micheli A, et al. Clinical and pathologic prognostic indicators in colorectal cancer. A population-based study. Cancer 1992;69:626-35. [Crossref] [PubMed]
- Dummer R, Flaherty KT, Robert C, et al. COLUMBUS 5-Year Update: A Randomized, Open-Label, Phase III Trial of Encorafenib Plus Binimetinib Versus Vemurafenib or Encorafenib in Patients With BRAF V600-Mutant Melanoma. J Clin Oncol 2022;40:4178-88. [Crossref] [PubMed]
- Sehgal A, Hoda D, Riedell PA, et al. Lisocabtagene maraleucel as second-line therapy in adults with relapsed or refractory large B-cell lymphoma who were not intended for haematopoietic stem cell transplantation (PILOT): an open-label, phase 2 study. Lancet Oncol 2022;23:1066-77. [Crossref] [PubMed]
- Robert C, Gautheret D. Multi-omics prediction in melanoma immunotherapy: A new brick in the wall. Cancer Cell 2022;40:14-6. [Crossref] [PubMed]
- Ribas A, Lawrence D, Atkinson V, et al. Combined BRAF and MEK inhibition with PD-1 blockade immunotherapy in BRAF-mutant melanoma. Nat Med 2019;25:936-40. [Crossref] [PubMed]
- Liu Y, Shi M, He X, et al. LncRNA-PACERR induces pro-tumour macrophages via interacting with miR-671-3p and m6A-reader IGF2BP2 in pancreatic ductal adenocarcinoma. J Hematol Oncol 2022;15:52. [Crossref] [PubMed]
- Liu HT, Zou YX, Zhu WJ, et al. lncRNA THAP7-AS1, transcriptionally activated by SP1 and post-transcriptionally stabilized by METTL3-mediated m6A modification, exerts oncogenic properties by improving CUL4B entry into the nucleus. Cell Death Differ 2022;29:627-41. [Crossref] [PubMed]