Effect of urokinase-type plasminogen activator combined with clinical stage and Barcelona Clinic Liver Cancer stage on the prognosis of patients with hepatocellular carcinoma
Highlight box
Key findings
• The decreased expression of plasminogen activator urokinase (PLAU) can prolong the disease-specific survival (DSS), overall survival (OS), and progression free interval (PFI) in liver hepatocellular carcinoma (LIHC) patients, and can be utilized as a novel predictive index.
What is known and what is new?
• As one of the major proteolytic enzymes involved in extracellular matrix degradation, PLAU has been shown to play a key role in biological events such as tissue remodeling and migration during development and tumorigenesis.
• The study evaluated the association and potential mechanism between PLAU and the prognosis of LIHC patients and analyzed the clinical value of PLAU combined with clinicopathological features in the early screening and prognosis of LIHC.
What is the implication, and what should change now?
• This study provides multilayered evidence for the importance of PLAU in the development of LIHC and its potential as a biomarker of LIHC disease progression.
Introduction
Liver cancer is a common malignancy and the 4th major cause of death by cancer globally. Liver cancer accounts for fifth place in global cancer cases, accounting for about 8.1%, and is the 2nd most common cause of mortality in men (1), accounting for 90% of primary liver cancers, and is the most common histological type. While current clinical treatments for liver hepatocellular carcinoma (LIHC) include surgery, ablation, embolization, and targeted therapy (2), the onset of the disease is insidious, and most patients are already in the middle and late stages once diagnosed. The above-mentioned treatment methods have little effect on clinically advanced patients, and most have only 6-8 months of expected average median survival time (3). Therefore, exploring the mechanism of LIHC occurrence and finding suitable biomarkers can effectively improve the ability of diagnosis, treatment, prognosis, and prevention of LIHC. There have been many previous research reports related to biomarkers of LIHC, but their sensitivity and specificity are low, such as alpha fetoprotein, alpha fetoprotein lens agglutinin 3, and deproteinization-γ-Carboxyprothrombin, etc. (4). However, both sensitivity and specificity are low. With the development of detection technology, various biomarker combinations and many new types of LIHC biomarkers have been discovered and gradually applied in clinical practice, improving the early diagnosis rate and prognosis level of LIHC, such as GALAD score, AKR1B10, MicroRNAs, and circular RNA (5-7).
Plasminogen activator, urokinase (PLAU), also called urokinase-type plasminogen activator (uPA), is a serine protease produced by epithelial cells, monocytes, fibroblasts, neutrophils, and tumor cells. Contradictory to other urokinases, PLAU lacks kinase function, is a serine peptidase of the PA family S1, and functions as a protease. PLAU is involved in the conversion of plasminogen to plasmin, the proteolysis of proteins involved in the remodeling of extracellular matrix (ECM), and in the activation of growth factors (8). PLAU stimulates the JAK_STAT signaling pathway, MAPK, and the focal adhesion kinase system by directly interacting with uPAR (uPA receptor) or indirectly activating plasmin to release growth factors from the ECM (9). As one of the main proteolytic enzymes associated with ECM degradation, PLAU has been revealed to be involved in biological activities such as tissue remodeling and migration during development and tumorigenesis (10,11). For example, network pharmacological studies based on Tripterygium wilfordii have found that triptolide suppresses the growth and migration of pancreatic cancer cells by down-regulating PLAU (12), and that long non-coding RNA TRPM2-AS modulates microRNA miR-138-5p and PLAU to stimulate gastric adenocarcinoma progression (13). Further, when PLAU mRNA is stabilized in an m6A-dependent manner it assists metastasis of colorectal cancer (14), and PSMC2 overexpression aids in the incidence and progression of human breast cancer by modulating PLAU (15). However, there is currently no research on the relevant mechanism of PLAU in LIHC. Immune cells in the tumor microenvironment play an important role in the occurrence, development and prognosis of LIHC (16). For example, tumor-infiltrating lymphocytes establish a complex network of cell-cell interactions, which can regulate the tumor microenvironment, promote tumor immune escape, and then promote tumor progression. At present, the mechanism of PLAU affecting the occurrence, development and prognosis of LIHC is still unclear. Therefore, we analyzed the correlation between PLAU and LIHC tumor non-invasion to infer its role in LIHC.
In this study, we preliminarily evaluated the expression of PLAU in multiple databases such as The Cancer Genome Atlas (TCGA), and performed bioinformatics and validation analysis to investigate the impact of PLAU on the cellular behavior of tumors and the associated mechanism. In addition, combined with the clinical data of LIHC patients in our hospital, we explored the clinical factors that can evaluate the treatment effect and predict prognosis, in order to provide a new strategy for the clinical diagnosis and treatment of LIHC. We present this article in accordance with the TRIPOD reporting checklist (available at https://jgo.amegroups.com/article/view/10.21037/jgo-23-311/rc).
Methods
Data assessment via TCGA database
We investigated the expression of PLAU in different tumors in the Tumor Immune Estimation Resource (TIMER) and TCGA databases, and also analyzed the expression of PLAU in LIHC cancer and adjacent tissues.
Association between PLAU and clinical manifestations of LIHC patients in TCGA database
Clinical manifestations were acquired from the TCGA database, then compared and assessed for the correlation of PLAU.
Nomogram construction and analysis
To personalize the predicted survival probabilities for 1, 3, and 5 years, we constructed a column chart based on the results of multivariate analysis. The RMS R package is used to generate column charts, which include clinical features related to PLAU and calibration charts. Calibration and discrimination are the most commonly used methods for evaluating model performance. In this study, the calibration curve was graphically evaluated by mapping the predicted probability of the column plot to the observed ratio, with a 45-degree line representing the best predicted value. The consistency index (C-index) is used to determine the discriminative power of the column chart, which is calculated using a bootstrap method with 1000 resamples. In addition, the C-index was used to compare the predictive accuracy of column charts and individual prognostic factors.
Analysis of genes interacting with PLAU in GeneMANIA database and string database
The GeneMANIA (http://www.genemania.org) and string (https://string-db.org/) databases were used for the genetic interaction and that of protein with DNA or protein, the associated pathways, physiological and biochemical activities, and genes from protein expression perspectives. Protein domain and phenotypic screening and the related genes interacting with PLAU were analyzed, and a protein-protein interaction (PPI) network was established.
Correlation between PLAU and immune cells in TIMER and TCGA databases
We constructed stick figures via the TIMER (https://cistrome.shinyapps.io/timer/) database to indicate the correlation of PLAU levels with various immune cells in LIHC. To further analyze the possible role of PLAU in LIHC tumor immune microenvironment.
Screening of drugs for treating colon cancer in Comparative Toxicogenomics Database (CTD) database
We queried the compounds related to PLAU for the treatment of colon cancer with the CTD database (https://ctdbase.org/), and screened according to the condition of interactions >10. The 2D or 3D structures of these associated compounds were then explored in the PubChem database (https://pubchem.ncbi.nlm.nih.gov/).
Gene Set Enrichment Analysis (GSEA) enrichment analysis
For functional analysis, Metascape online was used, and differential genes were also added.
Clinical data of LIHC patients
In this retrospective investigation, the clinical data of LIHC patients (n=100) admitted to the Shanghai Eastern Hepatobiliary Surgery Hospital between January 2018 to August 2019 were analyzed. Inclusion criteria were: (I) patients initially diagnosed with hepatic cancer and who underwent surgery; (II) postoperative pathology showed LIHC; (III) complete pathological, clinical, and follow-up data; (IV) good cognitive function and normal communication; (V) good compliance, with the patient able to cooperate and complete relevant inspections. Exclusion criteria were: (I) patients with serious underlying diseases; (II) patients who received neoadjuvant chemoradiotherapy before surgery or postoperatively received adjuvant chemoradiotherapy; (III) patients with incomplete clinical data; (IV) patients with liver metastases from other tumors. Finally, 100 patients were selected for this research, including 84 and 16 males and females aged between 38–73 years (mean age 51.2±9.5 years). The study was conducted in accordance with the Declaration of Helsinki (as revised in 2013). The study was approved by committee of the Shanghai Eastern Hepatobiliary Surgery Hospital (No. EHBHKY2022-H063-P001). Individual consent for this retrospective analysis was waived.
Follow-up
Follow-up was conducted by outpatient reexamination, medical records re-admission, and via telephone. After three months of surgery, liver ultrasound, chest X-ray, and serum alpha fetoprotein (AFP) were examined, whereas CT contrast examination was performed every six months (additional examinations were added based on the patient’s state). Tumor recurrence was confirmed based on radiological, clinical, and/or pathological examination. Early recurrence was considered when the tumor reappeared within 2 years after radical resection of liver cancer, and non-early recurrence after 2 years. Progression free survival (PFS) was considered as progression of the disease from the beginning of treatment to follow-up of any type. The details of those who were still alive and those who could not be followed-up were statistically analyzed at the end of the follow-up and were entered as the final cut-off time.
Statistical analysis
For statistical evaluation and visualization, R software (version 3.6.3) was used. Gene set enrichment analysis was performed to identify the possible cellular mechanism of PLAU, and the measurement results are depicted by mean ± standard deviation (SD). For comparison analysis t-test was performed, while % depicts the count data, and the χ2 test or Fisher’s exact probability test was conducted for comparison. To evaluate patient survival, the Kaplan-Meier method was utilized, and for determining the significance, the log-rank statistical method was applied. Statistical analyzes in Yu’s study were evaluated automatically from the aforementioned online database. A P value <0.05 or a log-rank P value <0.05 was deemed statistically important.
Results
PLAU is upregulated in LIHC
Using the Timer and TCGA databases, through pan-cancer evaluation we found that PLAU expression differed in tumors including BLCA, BRCA, COAD, ESCA, and GBM, while it was reduced in tumor tissues such as KICH, KIRC, KIRP, and PRAD, and the variability was statistically significant (P<0.05) (Figure 1A,1B). At the same time, in unpaired and paired LIHC tumor tissues, PLAU expression was greater than that in paracancerous tissues (Figure 1C,1D), and the ROC curve showed the AUC was 0.848, indicating PLAU has good predictive research value (Figure 1E).
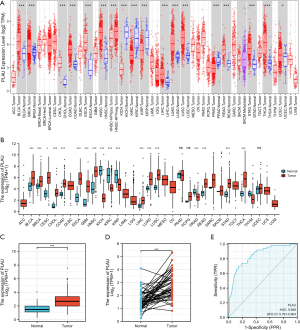
Correlation between PLAU in TCGA database and the prognosis of LIHC patients
Analysis of the TGCA database showed LIHC individuals with low PLAU expression had better OS, DSS, and PFI. The difference was statistically significant (P<0.05). When analyzing the relationship between PLAU expression and patient PFI, although the difference was not statistically significant, we can still see the trend, and the results also suggest that LIHC patients with low PLAU expression have relatively better PFI (Figure 2).
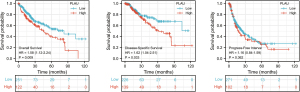
Association between PLAU expression in the TCGA database and the clinical details of LIHC individuals
In comparing the clinical data of LIHC individuals, those with increased PLAU expression had higher T, N, M, and pathological stages, along with alpha fetoprotein (AFP) level, pathological grade, and worse nutritional status level. This is also consistent with the above results that PLAU plays the role of a “oncogenic gene” in LIHC (Figure 3).
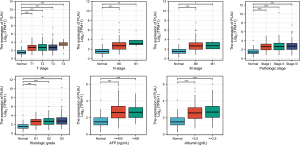
Establishing the nomogram
To individualize the prediction of the 1-, 3-, and 5-year of mortality rates of LIHC patients, a nomogram was constructed via the TCGA database depending on the multivariate assessment data, and the C-index of the nomogram was 0.643 (0.618–0.701) (Figure 4A). This prediction model has a certain degree of prediction accuracy. The deviation correction line in the calibration chart is close to the ideal curve (i.e., 45 degree line), indicating good consistency between the predicted and observed values (Figure 4B).
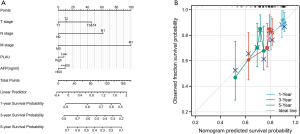
Determining the PLAU-interacting genes and proteins
A PLAU gene-gene interaction network was generated, and the neighboring genes were altered via GeneMania (Figure 5A). The PPI network of PLAU was established with the help of the STRING database (Figure 5B).
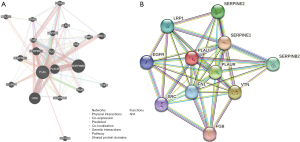
Association of PLAU with immune cells in TIMER and TCGA databases
We demonstrated the correlation of PLAU expression with tumor purity and various immune cells in LIHC through the TIMER database. This showed PLAU expression was positively associated with six types of immune infiltrating cells, comprising macrophages, CD4+ T, B, neutrophils, CD8+ T, and dendritic cells (Figure 6A). To additionally determine the impact of PLAU on the tumor microenvironment (TME), we analyzed the PLAU correlation with certain immune cells, and the data indicated it was only negatively associated with Th17 cells but was positively correlated with other immune cells including iDC, Th1 cells, TFH, and Tem (Figure 6B-6D). Further analysis revealed the PLAU levels were directly associated with the immune checkpoint-related molecules CTLA4, PDCD1, and CD274, and the difference was statistically significant (Figure 6E). On the basis of these results, it can be speculated that PLAU expression might be linked with the tumor immune infiltration of LIHC, and that PLAU may play a crucial function in tumor cell immune escape in the TME of LIHC, which provides a novel basis for future research.
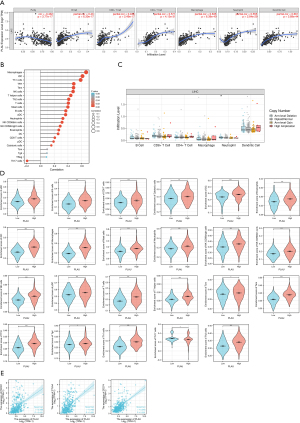
Correlation of PLAU with molecular targeted therapy drug target molecules in TCGA database
At present, whether surgery, interventional therapy, or chemotherapy are used, the therapeutic effect on LIHC is not significant, and its 5-year survival rate is still low. Current research guidelines also recommend corresponding targeted therapy, and the targeted therapy of liver cancer has been limited to anti-angiogenesis, principally comprising epidermal growth factor receptor (EGFR) inhibitory drugs, vascular endothelial growth factor receptor (VEGFR) antagonists, multikinase inhibitors, PI3K/Akt/mTOR signaling pathway, hepatocyte growth factor receptor (Met) inhibitors, and TGFβ receptor inhibitors. The analysis showed the PLAU expression was positively correlated with EGFR, VEGFC, VEGFA, VEGFB, AKT3, AKT1, AKT2, MTOR, TGFB2, TGFB1, TGFB3, FGFR3, FGFR1, and FGFR2, and the variability was statistically important (P<0.05) (Figure 7).
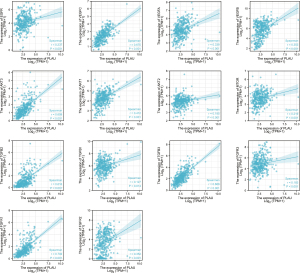
Screening of therapeutic LIHC drugs in CTD database
We searched the compounds related to PLAU for the treatment of colon cancer through the CTD database (https://ctdbase.org/), and screening according to the condition of interactions >10 showed six compounds related to PLAU for the treatment of LIHC, as shown in Table 1. We then explored the 2D or 3D structures of these related compounds in the PubChem database (https://pubchem.ncbi.nlm.nih.gov/), as represented in Figure 8.
Table 1
Interacting chemical | Interactions | Organisms |
---|---|---|
Resveratrol | 18 | 2 |
Carbon tetrachloride | 17 | 2 |
Plant extracts | 13 | 3 |
Arsenic trioxide | 12 | 1 |
Tetrachlorodibenzodioxin | 12 | 3 |
Amiloride | 11 | 2 |
LIHC, liver hepatocellular carcinoma; CTD, Comparative Toxicogenomics Database.
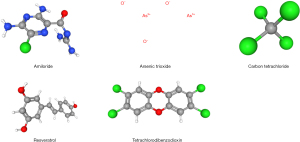
GSEA-based signaling pathways prediction
We performed an online GSEA functional analysis via Metascape and discovered that PLAU may impact the physiological activities of LIHC by participating in VEGFAVEGFR2, MAPK, JAK_STAT, B_CELL_RECEPTOR, and NOTCH signaling pathways along with platelet calcium homeostasis, angiogenesis, and P53, which resulted in the different prognosis of LIHC (Figure 9).
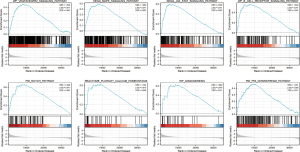
Relationship between PLAU and clinicopathological data of LIHC patients
We finally included the clinical details of 100 LIHC individuals and used immunohistochemistry to evaluate and determine their high and low PLAU expression. For immunohistochemical evaluation, immunohistochemical results were scored by the quantity and cellular staining intensity of positive cells. Scores were based on the quantity of positively stained cells: 0 (negative), 1 (≤25%), 2 (25–50%), 3 (51–75%), and 4 (>75%), and staining intensity: 0 (negative or no staining), 1 (weak positive), 2 (moderate positive), and 3 (strong positive). The final score of each specimen was obtained by multiplying the two scores. After determining the arithmetic mean of the final scores, those with a score <7 were considered to have low PLAU expression. Statistically important differences were obtained between the two groups in T stage and Edmondson grade (P<0.05), whereas gender, age, AFP, microvascular invasion, early recurrence, albumin, tumor diameter, HBsAg, CTP grade, Barcelona Clinic Liver Cancer (BCLC) stage, CS stage, and pathological types showed no significant difference (P>0.05) (Table 2).
Table 2
Variables | Total, n | PLAU low expression, n | PLAU high expression, n | P value |
---|---|---|---|---|
Gender | >0.9999 | |||
Male | 84 | 42 | 42 | |
Female | 16 | 8 | 8 | |
Age (years) | 0.8405 | |||
≤60 | 56 | 29 | 27 | |
>60 | 44 | 21 | 23 | |
AFP (ng/mL) | 0.2184 | |||
≤20 | 39 | 23 | 16 | |
>20 | 61 | 27 | 34 | |
HBsAg | 0.4695 | |||
Positive | 78 | 37 | 41 | |
Negative | 22 | 13 | 9 | |
Albumin (g/L) | 0.8369 | |||
≤40 | 62 | 32 | 30 | |
>40 | 38 | 18 | 20 | |
T stage | 0.0035 | |||
I–II | 63 | 39 | 24 | |
III–IV | 37 | 11 | 26 | |
Microvascular invasion | 0.4238 | |||
Yes | 49 | 27 | 22 | |
No | 51 | 23 | 28 | |
Early recurrence | 0.6353 | |||
Yes | 77 | 37 | 40 | |
No | 23 | 13 | 10 | |
Tumor diameter (cm) | 0.6820 | |||
≤5 | 61 | 32 | 29 | |
>5 | 39 | 18 | 21 | |
CTP grade | 0.4193 | |||
A/B | 57 | 31 | 26 | |
C/D | 43 | 19 | 24 | |
CS stage | 0.2975 | |||
1–2 | 36 | 21 | 15 | |
3–4 | 64 | 29 | 35 | |
BCLC stage | 0.0969 | |||
A–B | 37 | 14 | 23 | |
C–D | 63 | 36 | 27 | |
Pathological type | 0.7596 | |||
Hepatocellular carcinoma | 88 | 45 | 43 | |
Cholangiocarcinoma + mixed liver cancer | 12 | 5 | 7 | |
Edmondson grade | 0.0327 | |||
I–II | 67 | 39 | 28 | |
III–IV | 33 | 11 | 22 |
P<0.05, the variability is statistically important. PLAU, plasminogen activator urokinase; AFP, alpha fetoprotein; HBsAg, hepatitis B surface antigen; CTP, Child-Turcotte-Pugh; CS, clinical stage; BCLC, Barcelona Clinic Liver Cancer.
Comparison of OS and PFS between the two groups of patients
All patients were followed up for 3 years. A total of 57 patients survived, with a survival rate of 57%, and 43 died, with a mortality rate of 43%. The tumor progression rates of patients in the low PLAU group and high PLAU group were 88% (44/50) and 92% (46/50), respectively, and the early recurrence rates were 60% (30/50) and 72% (36/50), and the median PFS were 29.5 months and 23 months, respectively (Figure 10).
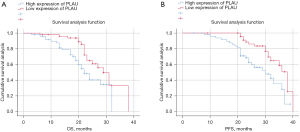
Univariate and multivariate analysis
Univariate and multivariate analysis revealed PLAU expression, CS stage, and BCLC stage were independent prognostic factors affecting tumor progression in LIHC patients (Tables 3,4).
Table 3
Variable | HR | 95% CI | P value |
---|---|---|---|
Gender | 1.084 | 0.455–2.586 | 0.631 |
Age (years) | 0.529 | 0.274–1.019 | 0.771 |
AFP (ng/mL) | 2.301 | 1.210–5.602 | 0.332 |
HBsAg | 2.157 | 0.927–5.384 | 0.340 |
Albumin (g/L) | 0.740 | 0.383–1.429 | 0.370 |
T stage | 5.472 | 1.954–8.517 | 0.035 |
Microvascular invasion | 2.719 | 1.306–5.782 | 0.291 |
Early recurrence | 6.252 | 3.048–9.815 | 0.027 |
Tumor diameter (cm) | 3.680 | 1.852–6.313 | 0.200 |
CTP grade | 4.613 | 1.951–7.059 | 0.062 |
CS stage | 9.356 | 4.242–19.637 | 0.000 |
BCLC stage | 8.295 | 3.943–19.011 | 0.000 |
Pathological types | 0.920 | 0.103–1.810 | 0.642 |
Edmondson grade | 4.508 | 1.893–6.892 | 0.073 |
PLAU expression | 7.768 | 2.583–16.136 | 0.013 |
OS, overall survival; HR, hazard ratio; CI, confidence interval; PLAU, plasminogen activator urokinase; AFP, alpha fetoprotein; HBsAg, hepatitis B surface antigen; CTP, Child-Turcotte-Pugh; CS, clinical stage; BCLC, Barcelona Clinic Liver Cancer.
Table 4
Variable | HR | 95% CI | P value |
---|---|---|---|
T stage | 2.784 | 0.985-5.428 | 0.287 |
Early recurrence | 3.521 | 1.759-6.058 | 0.211 |
CS stage | 5.904 | 2.021–8.689 | 0.029 |
BCLC stage | 5.374 | 1.987–8.397 | 0.038 |
PLAU expression | 4.992 | 1.648–7.028 | 0.044 |
OS, overall survival; HR, hazard ratio; CI, confidence interval; CS, clinical stage; BCLC, Barcelona Clinic Liver Cancer; PLAU, plasminogen activator urokinase.
Discussion
Hepatocellular carcinoma is responsible for 70–90% of primary hepatic cancer and is one of the most malignant tumors. To enhance the recovery rate of liver cancer, medical institutions in various countries have been actively exploring more effective treatment methods (17,18). Statistics show that globally there are 854,000 new cases of liver cancer annually, of which 466,000 cases are found in China, accounting for about 55% (19). Therefore, the research and development of liver cancer-specific target drugs is important in China. Current studies have shown that immunohistochemical methods combined with patient clinical case parameters can more accurately determine the malignancy and prognosis of tumors from the perspective of histology.
This study obtained the differentially expressed gene PLAU in LIHC from multiple databases such as TCGA and explored its levels and role in predicting the mortality rate in patients with the disease. We also conducted bioinformatics analysis and verification to elucidate the effect of PLAU on the behavior of tumor cells, and its potential mechanism. In the TCGA database, we found that PLAU expression in LIHC tumor tissues was enhanced compared with paracancerous tissues, and LIHC patients with low PLAU levels had better OS, DSS, and PFI than those with high levels. Moreover, individuals with increased PLAU expression had increased T stage, N stage, M stage, pathological stage, pathological grade, and AFP levels and a worse nutritional status level. In the Timer database, we discovered that PLAU expression was positively correlated with six types of infiltrating immune cells, including B, macrophages, CD4+ T, neutrophils, CD8+ T, and dendritic cells, and had good correlation with other different immune cells. Moreover, PLAU also had certain positive correlations with the immune checkpoint-associated molecules CTLA4, PDCD1, and CD274. Previous studies have also shown that immune cells play an important role in the biological events of LIHC, such as miR-155 regulating M2 polarization of tumor associated macrophages infected with hepatitis B virus, thereby regulating the malignant progression of hepatocellular carcinoma (20). CD8+ CD226high T cells in liver metastasis determine the prognosis of colorectal cancer patients receiving chemotherapy and radical surgery treatment (21). Attenuated WNV poly (A) exerts broad-spectrum oncolytic effects through selective viral replication and CD8+ T cell dependent immune response (22). The various behavioral activities of various immune cells from birth to death are almost without exception accompanied by the basic attribute of migration. In other words, immunity is dynamic. The essence of dynamics is the change of time and space. One study showed that the upregulation of PLAU was not only related to the invasive phenotype related to the growth and metastasis of PDAC, such as epithelial mesenchymal transformation (EMT), dryness, hypoxia, and immunosuppression (TME) in the tumor microenvironment. Elevated PLAU is associated with increased invasive phenotype, matrix score, and immunosuppression in PDAC (23). According to the obtained data, it can be speculated that PLAU expression might be associated with the tumor immune infiltration of LIHC. We then analyzed the correlation of PLAU with molecular targeted therapy drug target molecules and the results indicated its expression was positively correlated with EGFR, VEGFC, VEGFA, VEGFB, AKT3, AKT1, AKT2, MTOR, TGFB2, TGFB1, TGFB3, FGFR3, FGFR1, and FGFR2. Based on these two results, we found that PLAU is not only associated with the immune infiltration of LIHC, but may also be related to the selection and efficacy of targeted drugs in LIHC.
At present, the clinical treatment of LIHC involves individualization, multi-program combination, and combined immune and targeted therapy. There are also related clinical trials to verify and explore the clinical value of treatment, such as the COSMIC-312 trial, which evaluated the efficacy of cabozantinib combined with atezolizumab and sorafenib as first-line systemic therapy for advanced LIHC and found the mPFS advantage of the former treatment regimen was obvious (24). In addition, the AK105-203 trial proposed pembrolizumab and anlotinib as first-line treatment of unresectable LIHC and showed promising antitumor activity and good safety (25). These studies also reflect the clinical value and scientific research significance of our research molecules.
Through GSEA analysis, we found that PLAU may affect the biological events of LIHC by participating in the VEGFAVEGFR2 signaling pathway, MAPK signaling pathway, JAK_STAT signaling pathway, B_CELL_RECEPTOR signaling pathway, NOTCH signaling pathway, and platelet calcium homeostasis, angiogenesis, and P53, resulting in different prognoses of LIHC. Past studies on related mechanisms have shown Circ_0001178 regulates the miR-382/VEGFA axis to promote the progression of LIHC (26), that down-regulation of AnnexinA7 may inhibit the proliferation and metastasis of human liver cancer cells through the MAPK/ERK pathway (27), and that TMIGD2 in cancer cells binds to activate the JAK/STAT signaling pathway (28). Further, the Nogo-B receptor was seen to promote the growth of human LIHC cells through the Akt signaling pathway (29), while inhibiting RFX6 inhibited the invasion ability of tumor cells through the Notch pathway and affected tumor immunity in LIHC (30). Finally, aerobic glycolysis and tumor progression in LIHC was seen to be mediated by P53 K48-linked ubiquitin regulated by TRIM37 (31). These research results are good references for our further in vivo and in vitro experiments of PLAU in LIHC.
Then we further analyzed the clinical data of our hospital, and ultimately included the clinical data of 100 patients with LIHC. KM survival analysis showed that patients with low expression of PLAU in LIHC have better prognosis. COX regression analysis found that PLAU expression, CS stage, and BCLC stage were independent prognostic factors affecting tumor progression in patients with LIHC. Compared to other staging methods, the biggest characteristic of BCLC staging method is its guiding effect on treatment and its identification effect on early patients, with strong clinical practicality (32). The CS staging method is more based on patients with advanced liver cancer, and lacks the ability to distinguish between patients who may benefit from “radical treatment” at an earlier stage (33). Therefore, combining the two methods to assess the status of patients with LIHC has better clinical practical value.
Conclusions
In conclusion, this study provides multilayered evidence for the importance of PLAU in the development of LIHC and its potential as a biomarker of LIHC disease progression. At the same time, we also discovered the clinical value of PLAU combined with CS staging and BCLC staging in the early screening and prognosis of LIHC. Multi-center clinical trials are needed to verify the accuracy and practicability of our research results.
Acknowledgments
Funding: None.
Footnote
Reporting Checklist: The authors have completed the TRIPOD reporting checklist. Available at https://jgo.amegroups.com/article/view/10.21037/jgo-23-311/rc
Data Sharing Statement: Available at https://jgo.amegroups.com/article/view/10.21037/jgo-23-311/dss
Peer Review File: Available at https://jgo.amegroups.com/article/view/10.21037/jgo-23-311/prf
Conflicts of Interest: All authors have completed the ICMJE uniform disclosure form (available at https://jgo.amegroups.com/article/view/10.21037/jgo-23-311/coif). The authors have no conflicts of interest to declare.
Ethical Statement: The authors are accountable for all aspects of the work in ensuring that questions related to the accuracy or integrity of any part of the work are appropriately investigated and resolved. The study was conducted in accordance with the Declaration of Helsinki (as revised in 2013). The study was approved by committee of the Shanghai Eastern Hepatobiliary Surgery Hospital (No. EHBHKY2022-H063-P001). Individual consent for this retrospective analysis was waived.
Open Access Statement: This is an Open Access article distributed in accordance with the Creative Commons Attribution-NonCommercial-NoDerivs 4.0 International License (CC BY-NC-ND 4.0), which permits the non-commercial replication and distribution of the article with the strict proviso that no changes or edits are made and the original work is properly cited (including links to both the formal publication through the relevant DOI and the license). See: https://creativecommons.org/licenses/by-nc-nd/4.0/.
References
- Zhang CH, Cheng Y, Zhang S, et al. Changing epidemiology of hepatocellular carcinoma in Asia. Liver Int 2022;42:2029-41. [Crossref] [PubMed]
- Omata M, Cheng AL, Kokudo N, et al. Asia-Pacific clinical practice guidelines on the management of hepatocellular carcinoma: a 2017 update. Hepatol Int 2017;11:317-70. [Crossref] [PubMed]
- Manesis EK, Giannoulis G, Zoumboulis P, et al. Treatment of hepatocellular car-cinoma with combined suppression and inhibition of sex hormones: a randomized, con-trolled trial. Hepatology 1995;21:1535-42. [PubMed]
- De Stefano F, Chacon E, Turcios L, et al. Novel biomarkers in hepatocellular car-cinoma. Dig Liver Dis 2018;50:1115-23. [Crossref] [PubMed]
- Shen S, Lin Y, Yuan X, et al. Biomarker MicroRNAs for Diagnosis, Prognosis and Treatment of Hepatocellular Carcinoma: A Functional Survey and Comparison. Sci Rep 2016;6:38311. [Crossref] [PubMed]
- Yin H, Peng X, Ren P, et al. MicroRNAs as a novel class of diagnostic biomarkers in detection of hepatocellular carcinoma: a meta-analysis. Tumour Biol 2014;35:12317-26. [Crossref] [PubMed]
- Han B, Chao J, Yao H. Circular RNA and its mechanisms in disease: From the bench to the clinic. Pharmacol Ther 2018;187:31-44. [Crossref] [PubMed]
- Deryugina EI, Quigley JP. Cell surface remodeling by plasmin: a new function for an old enzyme. J Biomed Biotechnol 2012;2012:564259. [Crossref] [PubMed]
- Danø K, Behrendt N, Høyer-Hansen G, et al. Plasminogen activation and cancer. Thromb Haemost 2005;93:676-81. [Crossref] [PubMed]
- Chikamatsu K, Albers A, Stanson J, et al. P53(110-124)-specific human CD4+ T-helper cells enhance in vitro generation and antitumor function of tumor-reactive CD8+ T cells. Cancer Res 2003;63:3675-81. [PubMed]
- Niu FY, Jin C, Ma L, et al. Urokinase plasminogen activator predicts poor prog-nosis in hepatocellular carcinoma. J Gastrointest Oncol 2021;12:1851-9. [Crossref] [PubMed]
- Zhao X, Liu Z, Ren Z, et al. Triptolide inhibits pancreatic cancer cell prolifera-tion and migration via down-regulating PLAU based on network pharmacology of Trip-terygium wilfordii Hook F. Eur J Pharmacol 2020;880:173225. [Crossref] [PubMed]
- Sun J, Zhou F, Xue J, et al. Long non-coding RNA TRPM2-AS regulates mi-croRNA miR-138-5p and PLAU (Plasminogen Activator, Urokinase) to promote the progression of gastric adenocarcinoma. Bioengineered 2021;12:9753-65. [Crossref] [PubMed]
- Yu T, Liu J, Wang Y, et al. METTL3 promotes colorectal cancer metastasis by stabilizing PLAU mRNA in an m6A-dependent manner. Biochem Biophys Res Commun 2022;614:9-16. [Crossref] [PubMed]
- Wang Y, Zhu M, Li J, et al. Overexpression of PSMC2 promotes the tumorigene-sis and development of human breast cancer via regulating plasminogen activator uro-kinase (PLAU). Cell Death Dis 2021;12:690. [Crossref] [PubMed]
- Hinshaw DC, Shevde LA. The Tumor Microenvironment Innately Modulates Cancer Progression. Cancer Res 2019;79:4557-66. [Crossref] [PubMed]
- Wallace MC, Preen D, Jeffrey GP, et al. The evolving epidemiology of hepato-cellular carcinoma: a global perspective. Expert Rev Gastroenterol Hepatol 2015;9:765-79. [Crossref] [PubMed]
- Poureau PG, Metges JP. Fundamentals of Digestive Cancers Immunology, Especially Gastric and Hepatocellular Carcinomas Fondamentaux de l’immunologie des Cancers Digestifs (Gastriques et Hépatocellulaires). Oncologie 2021;23:47-59. [Crossref]
- Tanaka M, Katayama F, Kato H, et al. Hepatitis B and C virus infection and hepatocellular carcinoma in China: a review of epidemiology and control measures. J Epidemiol 2011;21:401-16. [Crossref] [PubMed]
- Fei Y, Wang Z, Huang M, et al. MiR-155 regulates M2 polarization of hepatitis B virus-infected tumour-associated macrophages which in turn regulates the malignant progression of hepatocellular carcinoma. J Viral Hepat 2023;30:417-26. [Crossref] [PubMed]
- Viot J, Abdeljaoued S, Vienot A, et al. CD8(+) CD226(high) T cells in liver me-tastases dictate the prognosis of colorectal cancer patients treated with chemotherapy and radical surgery. Cell Mol Immunol 2023;20:365-78. [Crossref] [PubMed]
- Liu J, Hu YY, Zhang QY, et al. Attenuated WNV-poly(A) exerts a broad-spectrum oncolytic effect by selective virus replication and CD8+ T cell-dependent immune re-sponse. Biomed Pharmacother 2023;158:114094. [Crossref] [PubMed]
- Hosen SMZ, Uddin MN, Xu Z, et al. Metastatic phenotype and immunosuppres-sive tumour microenvironment in pancreatic ductal adenocarcinoma: Key role of the urokinase plasminogen activator (PLAU). Front Immunol 2022;13:1060957. [Crossref] [PubMed]
- Kelley RK, Rimassa L, Cheng AL, et al. Cabozantinib plus atezolizumab versus sorafenib for advanced hepatocellular carcinoma (COSMIC-312): a multicentre, open-label, randomised, phase 3 trial. Lancet Oncol 2022;23:995-1008. [Crossref] [PubMed]
- Han C, Ye S, Hu C, et al. Clinical Activity and Safety of Penpulimab (Anti-PD-1) With Anlotinib as First-Line Therapy for Unresectable Hepatocellular Carcinoma: An Open-Label, Multicenter, Phase Ib/II Trial (AK105-203). Front Oncol 2021;11:684867. [Crossref] [PubMed]
- Gao S, Hu W, Huang X, et al. Circ_0001178 regulates miR-382/VEGFA axis to facilitate hepatocellular carcinoma progression. Cell Signal 2020;72:109621. [Crossref] [PubMed]
- Zhao Y, Yang Q, Wang X, et al. AnnexinA7 down-regulation might suppress the proliferation and metastasis of human hepatocellular carcinoma cells via MAPK/ ERK pathway. Cancer Biomark 2018;23:527-37. [Crossref] [PubMed]
- Guo H, Zhang C, Tang X, et al. HHLA2 Activates the JAK/STAT Signaling Pathway by Binding to TMIGD2 in Hepatocellular Carcinoma Cells. Inflammation 2022;45:1585-99. [Crossref] [PubMed]
- Dong C, Liu Y, Jiang K, et al. The Nogo-B receptor promotes human hepatocel-lular carcinoma cell growth via the Akt signal pathway. J Cell Biochem 2018;119:7738-46. [Crossref] [PubMed]
- Song M, Kuerban M, Zhao L, et al. Inhibition of RFX6 Suppresses the Invasive Ability of Tumor Cells Through the Notch Pathway and Affects Tumor Immunity in Hepatocellular Carcinoma. Front Oncol 2021;11:801222. [Crossref] [PubMed]
- Ge Y, Zhao R, Li B, et al. Aerobic glycolysis and tumor progression of hepato-cellular carcinoma are mediated by ubiquitin of P53 K48-linked regulated by TRIM37. Exp Cell Res 2022;421:113377. [Crossref] [PubMed]
- Santambrogio R, Salceda J, Costa M, et al. External validation of a simplified BCLC staging system for early hepatocellular carcinoma. Eur J Surg Oncol 2013;39:850-7. [Crossref] [PubMed]
- Su L, Zhou T, Zhang Z, et al. Optimal staging system for predicting the prognosis of patients with hepatocellular carcinoma in China: a retrospective study. BMC Cancer 2016;16:424. [Crossref] [PubMed]
(English Language Editor: B. Draper)