A bioinformatics analysis of potential cellular communication networks in non-alcoholic steatohepatitis and colorectal adenoma using scRNA-seq and bulk-seq
Highlight box
Key findings
• NASH/NAFLD may participate in inflammation response by up regulating core genes (including FOS, EGR1, MYC, IL1B, etc.) to promote the progress of CRA/CRC. The specific cell signal is generated in embryonic stem cells, sent by WNT2B in WNT pathway and received by FZD6, LRP5 and LRP6.
What is known and what is new?
• NASH/NAFLD had been shown to significantly increase the risk of CRA and CRC.
• NASH/NAFLD may regulate inflammatory response by mediating WNT2B in the WNT pathway, ultimately promoting the progression of CRA/CRC.
What is the implication, and what should change now?
• We may be able to suppress the progression of CRA/CRC by regulating the inflammatory immune response mediated by core genes and intervening in the signaling of the WNT pathway.
Introduction
Globally, colorectal cancer mortality is the second highest cause of cancer death (9.4%), after lung cancer (1). Colorectal adenoma (CRA) is considered a precancerous lesion of colorectal cancer (CRC) that progresses to malignancy through alterations in DNA repair defects, chromosomal instability, microsatellite instability, serrated pathways, and DNA methylation (2,3), and its high incidence and potential risk of malignancy has become an important reason for widespread health screening.
Non-alcoholic fatty liver disease (NAFLD) is the most common chronic liver disease worldwide. The estimated total prevalence of NAFLD in Asia has reached 27.4% and is expected to increase with obesity rates and an aging population (4). Non-alcoholic steatohepatitis (NASH), an inflammatory subtype of NAFLD, carries a much higher mortality rate than the general population or patients with other inflammatory subtypes of NAFLD (5,6). Hwang et al. first found that NAFLD was associated with an increased risk of colorectal adenomatous polyps (7). Previous research results suggested that patients with advanced CRC had higher levels of steatosis and liver fibrosis staging compared to those with normal or low-grade adenomatous polyps (8). NASH after NAFLD progression is an independent risk factor for colorectal polyps (OR, 2.08; P=0.020) and advanced colorectal tumors (OR, 2.81; P=0.049). Previous studies have shown that NAFLD/NASH may promote the occurrence and development of CRA/CRC through the insulin resistance pathway or the induction of chronic inflammation pathway (9,10). In terms of insulin resistance (IR), NAFLD/NASH aggravates IR and further promotes CRC progress by regulating Insulin-like growth factor (IGF). In addition, there is a chronic inflammatory response in NAFLD, which produces numerous inflammatory mediators such as interleukin-1 (IL-1). For example, IL-1 binds to its receptor and upregulates anti-apoptotic genes by activating nuclear transcription factors to promote the occurrence and development of colorectal cancer. NAFLD patients have an increased risk of developing CRA or CRC due to their chronic inflammation leading to an increase in related inflammatory factors. Although the relationship between NASH and CRA has attracted widespread attention, the biological mechanisms involved are still unclear, and further studies on CRA in the context of NASH and potential therapeutic targets are necessary.
Bioinformatics is a subject that uses computers to collect, sort out, and analyze biological information such as DNA and proteins. To a certain extent, it reveals the potential Mode of action and mechanism of disease at the molecular level. Because the occurrence of a disease may be related to tens of thousands of genes and proteins, we cannot individually determine whether they are related to the target disease. Under the influence of the era of Big data, many patient data will be stored in the database. We can further explore on the basis of previous research to find more relevant potential genes and effect pathways. Bioinformatics analysis is widely used in screening core genes and pathways of diseases (11).
In this study, we combined high-throughput sequencing data in the Gene Expression Omnibus (GEO) database and single-cell data of CRA patients. For high-throughput sequencing data, we screened core targets through gene function classification [Gene Ontology (GO)], pathway enrichment analysis [Kyoto Encyclopedia of Genes and Genomes (KEGG)], and protein-protein interaction (PPI) network analysis, and for single-cell data, used cell annotation analysis and screening of core effect pathways and specific signal pathways of cell communication. The purpose of the study was to find potential biomarkers, therapeutic targets, and related effect pathways of CRA-NASH. Based on previous research, we preliminarily anticipate that NAFLD/NASH may promote the progression of CRA by regulating inflammatory responses, but the specific genes and effector pathways need to be analyzed. We present this article in accordance with the STREGA reporting checklist (available at https://jgo.amegroups.com/article/view/10.21037/jgo-23-502/rc).
Methods
Gene expression profiling data collection
Based on the GPL570 and GPL14951 platforms, two matrix datasets (GSE37364, GSE89632) CRA samples, NASH, and controls were collected from the GEO database. The GSE37364 dataset included gene expression profiles of 38 CRA patients and 29 normal controls, while the GSE89632 dataset included gene expression profiles of 19 patients with NASH and 24 normal controls. The single-cell RNA sequencing data of 10 patients with CRA was also obtained from the GEO database (scRNA-seq, data number GSE201348). The data in the single cell dataset came from normal tissue, CRA tissue, or CRC tissue collected from patients. Based on these data, the changes in cell composition and cell state that occurred during the conversion of healthy colon from CRA to CRC were plotted. The overall design of the data collection process was to separate the nucleus from the sample using the impregnation method. After the nucleus separation, 10× scRNA-seq and scATAC-seq measurements were performed. The study was conducted in accordance with the Declaration of Helsinki (as revised in 2013).
Statistical analysis
Differentially expressed gene (DEG) selection
DEGs were screened using the “GEOquery”, “limma”, “complexheatmap”, “ggplot2”, and “umap” packages in R software (version 3.6.3). Samples of the CRA group, NASH group, and their respective controls were first downloaded from the GEO database with the aid of the GEOquery package, and the difference analysis of the two groups was performed using the limma package. The difference gene screening threshold was set at |log2 FC| ≥1 and adj. P<0.05, where the adjusted P value was used to reduce the false positive rate. The respective DEGs obtained from the two datasets were used to generate heat maps and volcano maps respectively using the “complexheatmap” and “ggplot2” packages in R software. In addition, overlapping DEGs between CRA and NASH were generated using “ggplot2” in R software to generate Venn diagrams, and these overlapping DEGs were retained for subsequent analysis.
Functional classification (GO) analysis
The overlapping DEGs between the above groups were collected and collated, and GO enrichment analysis was performed using the “clusterProfiler” package in R software. The adjusted P value <0.05 for GO enrichment was selected as the output results.
PPI network establishment
The study further explored the interactions between common genes. The intersecting genes obtained above were imported into the Protein Interaction Analysis Platform (http://string-db.org/) for network construction of PPIs, and a minimum interaction score higher than 0.4 was considered significant and processed for PPI network visualization.
Core gene and key molecule screening
Based on the Maximal Clique Centrality (MCC) algorithm, we screened core genes with high connectivity in the PPI network by the cytoHubba plugin (https://apps.cytoscape.org/apps/cytohubba). The MCODE analysis plug-in (MCODE, http://apps.cytoscape.org/apps/mcode) in Cytoscape software was then used to screen out key protein expression molecules.
Cell annotation of CRA scRNA-seq data
The scurat package of R software was used to analyze the scRNA-seq data, and samples with mitochondrial gene percentage greater than five were excluded. Normalized data function was used to standardize data and extract genes with high coefficient of variation between cells. Principal component analysis (PCA) was then conducted and the P value of each principal component was calculated. The principal components with P value <0.05 were selected for subsequent t-distributed stochastic neighbor embedding (t-SNE) analysis, and the cells were divided into different clusters. SingleR R package was used to annotate the cell types of the obtained cell clusters.
Cell distribution analysis of NASH-CRA core gene
We loaded the core gene obtained in “Core gene and key molecule screening”, combined with the cell annotation results of single cells, and used the celldex package, SingleR package, and Monocle package to draw a bubble chart, violin chart, and scatter chart to show the distribution and intensity of the core gene of NASH-CRA in different types of cells.
Analysis of cellular communication
The CellChat package of R software was used for cell-cell interaction analysis. CellChat packet cell communication analysis was used to acquire gene expression data from single cells as input files and simulate the communication between cells by combining gene expression with elements of the interaction between signal ligands, receptors, and their cofactors. Relevant elements were then loaded into the Secreted Signaling and Cell-Cell Contact human databases. We combined the communication between cells with the core genes of NASH-CRA to screen the key pathways and factors in the process of cell communication.
Results
Data downloading arrangement and DEG screening
We downloaded two matrix datasets (GSE37364, GSE89632) for CRA and NASH from the GEO database, and after screening with adjusted thresholds of P value <0.05 and |log2 FC| >1.0, the corresponding volcano and heat maps were plotted for visualizing the DEGs of the two datasets, as shown in Figure 1A-1D. In addition, Venn diagram analysis was performed to evaluate the common DEGs between GSE37364 and GSE89632. As shown in Figure 1E, 63 overlapping DEGs were identified.
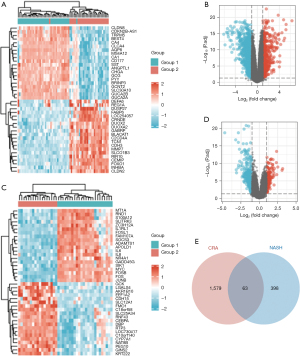
GO analysis of DEGs
Using the clusterProfiler package in R software, we performed GO enrichment analysis to better understand the function of DEGs, and after adjusting the threshold screening of P<0.05, selected the top 10 significantly enriched GO terms. The results are shown in Figure 2.
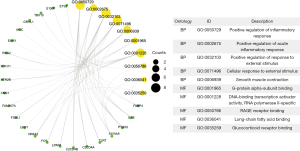
PPI network construction and core gene screening
PPI network analysis and visualization were first performed based on the STRING database (Figure 3) to identify the interactions between overlapping DEGs. The obtained results were then imported into Cytoscape software for cytoHubba analysis to identify core genes. Based on the MCC algorithm, the top 10 genes identified as potential central genes were FOS, EGR1, MYC, PTGS2, FOSL1, IL1B, NR4A2, IER3, ETS2, and TEAD4 (Figure 4A, Table 1). The MCODE plugin was used to identify important gene cluster modules and obtain cluster scores (filtering criteria: degree cutoff =2; node score cutoff =0.2; k-core =2; maximum depth =100), and finally one core gene cluster module was obtained, as shown in Figure 4B. These modules contained six potential core genes: IL1B, FOSL1, EGR1, MYC, PTGS2, and FOS, and the specific cluster scores are shown in Table 2.
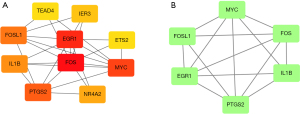
Table 1
Rank | Name | Score |
---|---|---|
1 | FOS | 77 |
2 | EGR1 | 66 |
3 | MYC | 62 |
4 | PTGS2 | 58 |
5 | FOSL1 | 37 |
6 | IL1B | 31 |
7 | NR4A2 | 8 |
8 | IER3 | 7 |
9 | ETS2 | 6 |
9 | TEAD4 | 6 |
MCC, Maximal Clique Centrality.
Table 2
Name | MCODE score |
---|---|
IL1B | 4.00 |
FOSL1 | 4.00 |
EGR1 | 3.73 |
MYC | 3.73 |
PTGS2 | 3.73 |
FOS | 3.73 |
MCODE, Molecular Complex Detection.
Cell annotation of scRNA-seq data
The scRNA-seq data used in this study were from 90,000 cells (9,000 cells per sample) from 10 patients with CRA. Seurat R package was used to analyze the scRNA-seq colorectal cancer cells, and the first 1,500 genes with the largest coefficient of variation of PCA were extracted. The first 20 principal components identified were included in t-SNE analysis, and 90,000 cells were divided into 19 clusters (clusters 0–18). Subsequently, the DEGs in each cluster were identified (P<0.05) and defined as marker genes of corresponding clustering, and the clustering results and corresponding expression amount of DEGs were displayed by thermography. To study the characteristics of cells in each cluster, we used SingleR package to obtain cell type annotations in different clusters. The results showed 19 clusters, including seven cell types. Among them, clusters 0–10th, 12th, and 14th were epithelial cells. Cluster 11 was tissue stem cells, cluster 13 was T cells, cluster 15 was macrophages, cluster 16 was B cells, cluster 17 was embryonic stem cells, and cluster 18 was endothelial cells, as shown in Figures 5-7.
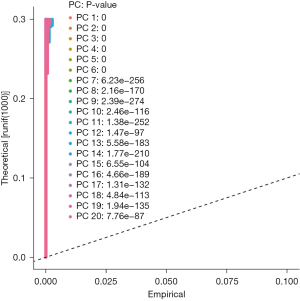
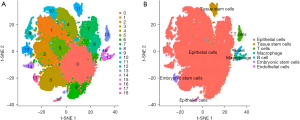
Cell distribution of NASH-CRA core genes
The core genes of NASH-CRA were loaded and the cell annotation results of single cells were combined. The celldex package, SingleR package, and monocle package were then used to analyze the distribution and intensity of the core genes in different types of cells and create a visual display, as shown in Figures 8-10. Based on the visualization results of the three graphs, it could be seen that the distribution and expression of FOS and ETS2 were the most obvious in cells.
Cell communication analysis
We used the “clusterProfiler” package in R software to conduct KEGG signal pathway enrichment analysis on NASH-CRA core genes and selected the adjusted P value <0.05 as the output result (Figure 11).
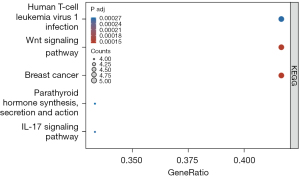
The CellChat package of R software was used to analyze the cell communication of CRA cell samples and identify the cell signal network related to NASH-CRA. Figure 12 shows the overall communication conditions of all cell clusters, Figure 12A shows the number of communication genes in the cell cluster, and Figure 12B shows the communication strength of the cell cluster genes. In addition, from the cell communication network, we identified the signal pathway related to CRA (Figure 13) and intersected the KEGG pathway enrichment results of NASH-CRA core genes before determining the key cell communication pathway of NASH-CRA as the WNT pathway. The visualization of the results in the signal path is shown in Figures 14,15. As shown in Figure 14, the expression of Embryonic stem cells was the most active in the WNT pathway, with the main signal docking channels including WNT2B − (FZD6 + LRP5) and WNT2B − (FZD6 + LRP6). The “transmitter” in Figure 15 referred to the signal source, and the “receiver” referred to the signal target. Embryonic stem cells were both “transmitters” and “receivers” in the WNT pathway. WNT2B existed as a “transmitter” in Embryonic stem cells, sending signals to FZD6, LRP5, and LRP6 of Embryonic stem cells for reception.
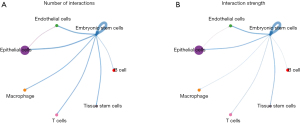
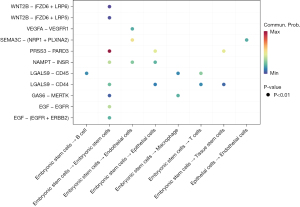
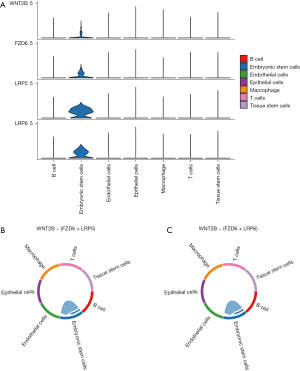
Discussion
Increasingly, studies are confirming the correlation between CRA and NAFLD/NASH. A previous cross-sectional study showed the prevalence of CRA was 40.7% in patients with NAFLD, significantly higher than the 28.1% in patients without NAFLD, and after adjusting for confounding factors of hyperlipidemia, diabetes, and obesity, NAFLD remained independently associated with a higher incidence of adenomas (12). Further, several meta-analyses have shown the severity of NAFLD is one of the independent risk factors for patients with CRA, with a 1.61-fold increase in the incidence of CRAs and a 2.34-fold increase in the incidence of advanced colorectal neoplasms in patients with severe NAFLD (8,9). In addition, the incidence of both NAFLD and colorectal neoplasms was increased in patients with risk factors for the metabolic syndrome (12,13). NASH, the progressive stage of NAFLD, is a critical stage for the development of cirrhosis and hepatocellular carcinoma, and patients with NASH have a higher prevalence of CRA and advanced colorectal tumors (14). In a previous study, it was shown that advance fibrosis was significantly associated with CRA in patients with NAFLD (15). We suggest this may be because the development of advanced fibrotic NAFLD is associated with severe insulin resistance and pro-inflammatory factors (16,17), which in turn cause hyperinsulinemia due to insulin resistance, leading to colorectal tumor growth and resistance to apoptosis (14,18). However, to date, the mechanism of the association between CRA and NAFLD/NASH has not been fully clarified. Exploration of the molecular mechanisms between CRA and NASH can help in early identification and intervention of the disease, which is undoubtedly of great clinical significance.
In this study, a series of raw signal analyses were performed on two independent matrix datasets of CRA and NASH, and 63 overlapping DEGs between CRA and NASH were obtained based on the GEO database. The results of GO enrichment analysis showed that DEGs were mainly enriched in positive regulation of inflammatory response and external stimuli, and contraction of smooth muscle. This result suggests DEGs between CRA and NASH are associated with inflammation and immune function, and is consistent with previous studies (19,20).
Subsequently, we took the results of Cytoscape’s cytoHubba plugin and MCODE plugin analysis and obtained six core genes in the PPI network, including FOS, EGR1, MYC, PTGS2, FOSL1, and IL1B. These six genes were most significantly differentially expressed in CRA patients and NASH patients and may be closely related to the pathogenesis of CRA and NAFLD/NASH.
Separately, FOS and FOSL1 belong to the same FOS family proteins. These proteins are transiently and rapidly expressed in response to external stimuli (21) and form structurally stable heterodimers with JUN family proteins, resulting in AP-1, a transcription factor complex. AP-1 regulates cell proliferation, differentiation, senescence, and apoptosis, and is involved in cell migration, immunity, inflammation, and many other aspects (22,23). Studies show FOS/FOSL1 is overexpressed in colorectal tumors and is an important driver of colorectal cancer metastasis (24-26), and Jones et al. (27) showed the expression of FOS was associated with the development of insulin resistance. In addition, oxidative stress and insulin resistance are major factors in the progression from steatosis to NASH in NAFLD, a process facilitated by DNA binding of AP-1 and NF-κB (28). EGR1 is a member of the zinc-finger transcription factor family and has important regulatory roles in cell growth, proliferation, differentiation, and apoptosis (29,30). Current studies suggest EGR1 can be both a tumor suppressor and a tumor promoter, depending on the cell type and external stimuli (31,32). In colorectal cancer tissues, EGR1 expression is significantly upregulated compared to normal mucosa, probably because EGR1 affects the progression of colorectal cancer by promoting the proliferation of tumor cells (32). Interestingly, in patients with NAFLD, EGR1 is positively correlated with the expression of the FOS gene family and negatively correlated with the degree of steatosis, and is lowly expressed in patients with insulin resistance. These may be important factors contributing to the development and progression of NAFLD (33). MYC is a proto-oncogene, and activation of a series of transcription factors encoded by it is frequently seen in many human tumors (34). These transcription factors can form heterodimers with the bHLH-LZ protein MAX and promote or enhance the transcription of specific target genes through the binding of this complex to the enhancer cassette (E cassette) consensus sequence (35). MYC modulates the host immune system through a mechanism of immune checkpoints, specific receptors, and secreted cytokines that contribute to the development and continued progression of cancer (34). In patients with colorectal tumors, MYC is a major effector of Wnt-β-linked protein pathway activity and a central node linking downstream misexpression to dysregulation of Wnt-β-linked proteins and other signaling pathways (36), which may play an important role in CRA/CRC pathogenesis (37,38). Aberrant MYC is involved in the development of NAFLD by regulating m6A, which is associated with lipid deposition, inflammation, and the immune microenvironment in NAFLD (39). In contrast, activation of the Wnt-β-linked protein signaling pathway strongly accelerates MYC-driven oncogenesis in the liver (40). PTGS2 is expressed as COX-2, a key enzyme for the conversion of arachidonic acid to prostaglandins, is induced by cytokines and growth factors, and is upregulated during inflammation. PTGS2 acts on cell proliferation, angiogenesis, apoptosis receptor, invasion, and the immune response by activating a PGE2 response, among others (41,42). Studies have shown low doses of aspirin reduce the incidence of colorectal cancer and adenoma recurrence, which is associated with high COX-2 expression, and COX-2 inhibitors are now widely used in colorectal cancer chemoprevention (43-45). In contrast, high expression of PTGS2 also interferes with the metabolism of normal substances in the liver, leading to the accumulation of triglycerides in hepatocytes, insulin resistance, and release of inflammatory factors, which affect the development and progression of liver disease (46-49). Of interest is that intestinal flora suppresses tumor immunity by driving the PTGS2 pathway, which in turn promotes NAFLD/NASH progression, while intestinal flora is altered in CRA/colorectal cancer patients by modulating local immune responses and producing genotoxins, which in turn affects tumorigenesis and progression (48,50-52). The mechanism of action of intestinal flora on CRA and NAFLD remains to be further investigated. In contrast, IL1B belongs to the interleukin 1 cytokine family, and its expressed IL-1β is an important pro-inflammatory cytokine involved in a variety of autoimmune inflammatory responses and multiple cellular activities leading to insulin resistance by inhibiting insulin receptor signaling and inducing inflammatory gene transcription (48,53). A study by Proença et al. (54) showed that immune regulation of inflammatory responses to bacterial invasion increased the expression of pro-inflammatory IL1B and other factors and miRNA expression, which may drive the progression of CRA to colorectal cancer. In addition, common variants located in the IL1B gene rs1143634 were shown to be independently associated with advanced histological features of NASH, increasing the risk of liver parenchymal injury in the form of ballooning and increased Mallory vesicles, as well as severe steatosis or cirrhosis (55).
We conducted cell annotation and cell communication analysis of the single-cell data set of patients with CRA, and combined with the matrix data of CRA-NASH, the results showed the key pathway of CRA-NASH may be the WNT pathway, and the main cell signal communication pathways include WNT2B − (FZD6 + LRP5) and WNT2B − (FZD6 + LRP6). Combined with the KEGG pathway enrichment results of CRA-NASH, MYC and FOSL1 were also genes playing a key regulatory role in the WNT pathway. MYC transcription-translated proteins were the main effectors of activity of the Wnt-β connexin pathway, and regulated the production of Wnt-α connexin, which was closely related to the process of CRA progression to colorectal cancer. At the same time, some studies suggest activation of the Wnt-β connexin signal pathway regulated by MYC accelerates the occurrence of liver tumors, which requires close attention in clinical practice (36-40). As an important driving factor of colorectal cancer metastasis, FOSL1 participates in the process of NAFLD developing into NASH through oxidative stress and insulin resistance. It could be seen that the prognosis of CRA and colorectal cancer was closely related to the development of NAFLD/NASH.
Based on the available studies, we hypothesize that these six core genes have an important impact on the development of CRA and NASH, and could be potential diagnostic, therapeutic, or preventive targets in the future. The key pathway of CRA-NASH may be the WNT pathway, and the main cell signal communication pathways include WNT2B − (FZD6 + LRP5) and WNT2B − (FZD6 + LRP6). This send-receive process occurs in embryonic stem cells.
Conclusions
Our study found the core genes of CRA-NASH, including FOS, EGR1, MYC, PTGS2, FOSL1, and IL1B, were up-regulated during the development of CRA and NASH, and might interfere with the pathophysiological processes of CRA and NASH by participating in inflammation and immune responses. In addition, the key pathway of CRA-NASH might be the WNT pathway. The main cell signal communication pathways included WNT2B − (FZD6 + LRP5) and WNT2B − (FZD6 + LRP6). The send-receive process occurred in embryonic stem cells. These findings might help to formulate early diagnosis and treatment strategies for CRA in NASH patients, and further explore corresponding prognostic markers and potential pathways. In summary, the significance of scRNA-seq in exploring tumor heterogeneity lies in promoting our understanding of the expression program of tumor related genes in tumor development patterns. However, it must be acknowledged that the biggest challenge it currently faces is that this analysis may miss out on some biologically significant gene expression programs, such as those that only involve a few genes or exist in rare cells, which requires further exploration by other research methods. Our next research plan is to develop an appropriate basic research protocol to validate the results of this study.
Acknowledgments
Funding: This work was supported by University Scientific Research Project of Guangdong Provincial Department of Education - key field project (No. 2021ZDZX2059), Guangzhou University of Chinese Medicine “Double First-Class” and High-level University Discipline Collaborative Innovation Team (No. 2021xk58), Guangzhou Science and Technology Plan Project (No. 202201020377), Special Support for Clinical Research of Guangdong Hospital of Traditional Chinese Medicine (No. YN1010914), the Special Project of Science and Technology Innovation Strategy of Guangdong Province (No. pdjh2021b0123).
Footnote
Reporting Checklist: The authors have completed the STREGA reporting checklist. Available at https://jgo.amegroups.com/article/view/10.21037/jgo-23-502/rc
Peer Review File: Available at https://jgo.amegroups.com/article/view/10.21037/jgo-23-502/prf
Conflicts of Interest: All authors have completed the ICMJE uniform disclosure form (available at https://jgo.amegroups.com/article/view/10.21037/jgo-23-502/coif). The authors have no conflicts of interest to declare.
Ethical Statement: The authors are accountable for all aspects of the work in ensuring that questions related to the accuracy or integrity of any part of the work are appropriately investigated and resolved. The study was conducted in accordance with the Declaration of Helsinki (as revised in 2013).
Open Access Statement: This is an Open Access article distributed in accordance with the Creative Commons Attribution-NonCommercial-NoDerivs 4.0 International License (CC BY-NC-ND 4.0), which permits the non-commercial replication and distribution of the article with the strict proviso that no changes or edits are made and the original work is properly cited (including links to both the formal publication through the relevant DOI and the license). See: https://creativecommons.org/licenses/by-nc-nd/4.0/.
References
- Sung H, Ferlay J, Siegel RL, et al. Global Cancer Statistics 2020: GLOBOCAN Estimates of Incidence and Mortality Worldwide for 36 Cancers in 185 Countries. CA Cancer J Clin 2021;71:209-49. [Crossref] [PubMed]
- Nguyen LH, Goel A, Chung DC. Pathways of Colorectal Carcinogenesis. Gastroenterology 2020;158:291-302. [Crossref] [PubMed]
- Zhou H, Shen Z, Zhao J, et al. Distribution characteristics and risk factors of colorectal adenomas. Zhonghua Wei Chang Wai Ke Za Zhi 2018;21:678-84. [PubMed]
- Cotter TG, Rinella M. Nonalcoholic Fatty Liver Disease 2020: The State of the Disease. Gastroenterology 2020;158:1851-64. [Crossref] [PubMed]
- Kim JW, Lee CH, Kim BH, et al. Ultrasonographic index for the diagnosis of non-alcoholic steatohepatitis in patients with non-alcoholic fatty liver disease. Quant Imaging Med Surg 2022;12:1815-29. [Crossref] [PubMed]
- Sheka AC, Adeyi O, Thompson J, et al. Nonalcoholic Steatohepatitis: A Review. JAMA 2020;323:1175-83. [Crossref] [PubMed]
- Hwang ST, Cho YK, Park JH, et al. Relationship of non-alcoholic fatty liver disease to colorectal adenomatous polyps. J Gastroenterol Hepatol 2010;25:562-7. [Crossref] [PubMed]
- Cho Y, Lim SK, Joo SK, et al. Nonalcoholic steatohepatitis is associated with a higher risk of advanced colorectal neoplasm. Liver Int 2019;39:1722-31. [Crossref] [PubMed]
- de Kort S, Simons CCJM, van den Brandt PA, et al. Diabetes mellitus, genetic variants in the insulin-like growth factor pathway and colorectal cancer risk. Int J Cancer 2019;145:1774-81. [Crossref] [PubMed]
- Gong Y, Dou LJ, Liang J. Link between obesity and cancer: role of triglyceride/free fatty acid cycling. Eur Rev Med Pharmacol Sci 2014;18:2808-20. [PubMed]
- Jiang H, Du H, Liu Y, et al. Identification of novel prognostic biomarkers for osteosarcoma: a bioinformatics analysis of differentially expressed genes in the mesenchymal stem cells from single-cell sequencing data set. Transl Cancer Res 2022;11:3841-52. [Crossref] [PubMed]
- Blackett JW, Verna EC, Lebwohl B. Increased Prevalence of Colorectal Adenomas in Patients with Nonalcoholic Fatty Liver Disease: A Cross-Sectional Study. Dig Dis 2020;38:222-30. [Crossref] [PubMed]
- Giovannucci E. Metabolic syndrome, hyperinsulinemia, and colon cancer: a review. Am J Clin Nutr 2007;86:s836-42. [Crossref] [PubMed]
- Wong VW, Wong GL, Tsang SW, et al. High prevalence of colorectal neoplasm in patients with non-alcoholic steatohepatitis. Gut 2011;60:829-36. [Crossref] [PubMed]
- Seo JY, Bae JH, Kwak MS, et al. The Risk of Colorectal Adenoma in Nonalcoholic or Metabolic-Associated Fatty Liver Disease. Biomedicines 2021;9:1401. [Crossref] [PubMed]
- Wong VW, Hui AY, Tsang SW, et al. Metabolic and adipokine profile of Chinese patients with nonalcoholic fatty liver disease. Clin Gastroenterol Hepatol 2006;4:1154-61. [Crossref] [PubMed]
- Ahn JS, Sinn DH, Min YW, et al. Non-alcoholic fatty liver diseases and risk of colorectal neoplasia. Aliment Pharmacol Ther 2017;45:345-53. [Crossref] [PubMed]
- Parizadeh SM, Parizadeh SA, Alizade-Noghani M, et al. Association between non-alcoholic fatty liver disease and colorectal cancer. Expert Rev Gastroenterol Hepatol 2019;13:633-41. [Crossref] [PubMed]
- Chang CM, Chia VM, Gunter MJ, et al. Innate immunity gene polymorphisms and the risk of colorectal neoplasia. Carcinogenesis 2013;34:2512-20. [Crossref] [PubMed]
- Anstee QM, Targher G, Day CP. Progression of NAFLD to diabetes mellitus, cardiovascular disease or cirrhosis. Nat Rev Gastroenterol Hepatol 2013;10:330-44. [Crossref] [PubMed]
- Jia J, Ye T, Cui P, et al. AP-1 transcription factor mediates VEGF-induced endothelial cell migration and proliferation. Microvasc Res 2016;105:103-8. [Crossref] [PubMed]
- Bejjani F, Evanno E, Zibara K, et al. The AP-1 transcriptional complex: Local switch or remote command? Biochim Biophys Acta Rev Cancer 2019;1872:11-23. [Crossref] [PubMed]
- Szalóki N, Krieger JW, Komáromi I, et al. Evidence for Homodimerization of the c-Fos Transcription Factor in Live Cells Revealed by Fluorescence Microscopy and Computer Modeling. Mol Cell Biol 2015;35:3785-98. [Crossref] [PubMed]
- Wang HL, Wang J, Xiao SY, et al. Elevated protein expression of cyclin D1 and Fra-1 but decreased expression of c-Myc in human colorectal adenocarcinomas overexpressing beta-catenin. Int J Cancer 2002;101:301-10. [Crossref] [PubMed]
- Cui HY, Wei W, Qian MR, et al. PDGFA-associated protein 1 is a novel target of c-Myc and contributes to colorectal cancer initiation and progression. Cancer Commun (Lond) 2022;42:750-67. [Crossref] [PubMed]
- Iskit S, Schlicker A, Wessels L, et al. Fra-1 is a key driver of colon cancer metastasis and a Fra-1 classifier predicts disease-free survival. Oncotarget 2015;6:43146-61. [Crossref] [PubMed]
- Jones MR, Chazenbalk G, Xu N, et al. Steroidogenic regulatory factor FOS is underexpressed in polycystic ovary syndrome (PCOS) adipose tissue and genetically associated with PCOS susceptibility. J Clin Endocrinol Metab 2012;97:E1750-7. [Crossref] [PubMed]
- Videla LA, Tapia G, Rodrigo R, et al. Liver NF-kappaB and AP-1 DNA binding in obese patients. Obesity (Silver Spring) 2009;17:973-9. [Crossref] [PubMed]
- Arimochi H, Morita K, Kataoka K, et al. Suppressive effect of Clostridium perfringens-produced heat-stable substance(s) on proliferation of human colon adenocarcinoma HT29 cells in culture. Cancer Lett 2006;241:228-34. [Crossref] [PubMed]
- Mahalingam D, Natoni A, Keane M, et al. Early growth response-1 is a regulator of DR5-induced apoptosis in colon cancer cells. Br J Cancer 2010;102:754-64. [Crossref] [PubMed]
- Choi EJ, Yoo NJ, Kim MS, et al. Putative Tumor Suppressor Genes EGR1 and BRSK1 Are Mutated in Gastric and Colorectal Cancers. Oncology 2016;91:289-94. [Crossref] [PubMed]
- Myung DS, Park YL, Kim N, et al. Expression of early growth response-1 in colorectal cancer and its relation to tumor cell proliferation and apoptosis. Oncol Rep 2014;31:788-94. [Crossref] [PubMed]
- Li Z, Yu P, Wu J, et al. Transcriptional Regulation of Early Growth Response Gene-1 (EGR1) is Associated with Progression of Nonalcoholic Fatty Liver Disease (NAFLD) in Patients with Insulin Resistance. Med Sci Monit 2019;25:2293-3004. [Crossref] [PubMed]
- Dhanasekaran R, Deutzmann A, Mahauad-Fernandez WD, et al. The MYC oncogene - the grand orchestrator of cancer growth and immune evasion. Nat Rev Clin Oncol 2022;19:23-36. [Crossref] [PubMed]
- Donati G, Amati B. MYC and therapy resistance in cancer: risks and opportunities. Mol Oncol 2022;16:3828-54. [Crossref] [PubMed]
- Kim J, Woo AJ, Chu J, et al. A Myc network accounts for similarities between embryonic stem and cancer cell transcription programs. Cell 2010;143:313-24. [Crossref] [PubMed]
- Cohen AJ, Saiakhova A, Corradin O, et al. Hotspots of aberrant enhancer activity punctuate the colorectal cancer epigenome. Nat Commun 2017;8:14400. [Crossref] [PubMed]
- Fang Y, Shen ZY, Zhan YZ, et al. CD36 inhibits β-catenin/c-myc-mediated glycolysis through ubiquitination of GPC4 to repress colorectal tumorigenesis. Nat Commun 2019;10:3981. [Crossref] [PubMed]
- Cheng W, Li M, Zhang L, et al. New roles of N6-methyladenosine methylation system regulating the occurrence of non-alcoholic fatty liver disease with N6-methyladenosine-modified MYC. Front Pharmacol 2022;13:973116. [Crossref] [PubMed]
- Bisso A, Filipuzzi M, Gamarra Figueroa GP, et al. Cooperation Between MYC and β-Catenin in Liver Tumorigenesis Requires Yap/Taz. Hepatology 2020;72:1430-43. [Crossref] [PubMed]
- Kunzmann AT, Murray LJ, Cardwell CR, et al. PTGS2 (Cyclooxygenase-2) expression and survival among colorectal cancer patients: a systematic review. Cancer Epidemiol Biomarkers Prev 2013;22:1490-7. [Crossref] [PubMed]
- Wang D, Dubois RN. Prostaglandins and cancer. Gut 2006;55:115-22. [Crossref] [PubMed]
- Benamouzig R, Uzzan B, Martin A, et al. Cyclooxygenase-2 expression and recurrence of colorectal adenomas: effect of aspirin chemoprevention. Gut 2010;59:622-9. [Crossref] [PubMed]
- Benelli R, Venè R, Ferrari N. Prostaglandin-endoperoxide synthase 2 (cyclooxygenase-2), a complex target for colorectal cancer prevention and therapy. Transl Res 2018;196:42-61. [Crossref] [PubMed]
- Zhang Z, Ghosh A, Connolly PJ, et al. Gut-Restricted Selective Cyclooxygenase-2 (COX-2) Inhibitors for Chemoprevention of Colorectal Cancer. J Med Chem 2021;64:11570-96. [Crossref] [PubMed]
- Dieter P, Scheibe R, Jakobsson PJ, et al. Functional coupling of cyclooxygenase 1 and 2 to discrete prostanoid synthases in liver macrophages. Biochem Biophys Res Commun 2000;276:488-92. [Crossref] [PubMed]
- Enomoto N, Ikejima K, Yamashina S, et al. Kupffer cell-derived prostaglandin E(2) is involved in alcohol-induced fat accumulation in rat liver. Am J Physiol Gastrointest Liver Physiol 2000;279:G100-6. [Crossref] [PubMed]
- Liu Y, Liu X, Zhou W, et al. Integrated bioinformatics analysis reveals potential mechanisms associated with intestinal flora intervention in nonalcoholic fatty liver disease. Medicine (Baltimore) 2022;101:e30184. [Crossref] [PubMed]
- Vickers AE. Characterization of hepatic mitochondrial injury induced by fatty acid oxidation inhibitors. Toxicol Pathol 2009;37:78-88. [Crossref] [PubMed]
- Chen F, Dai X, Zhou CC, et al. Integrated analysis of the faecal metagenome and serum metabolome reveals the role of gut microbiome-associated metabolites in the detection of colorectal cancer and adenoma. Gut 2022;71:1315-25. [Crossref] [PubMed]
- Kim M, Vogtmann E, Ahlquist DA, et al. Fecal Metabolomic Signatures in Colorectal Adenoma Patients Are Associated with Gut Microbiota and Early Events of Colorectal Cancer Pathogenesis. mBio 2020;11:e03186-19. [Crossref] [PubMed]
- Loo TM, Kamachi F, Watanabe Y, et al. Gut Microbiota Promotes Obesity-Associated Liver Cancer through PGE(2)-Mediated Suppression of Antitumor Immunity. Cancer Discov 2017;7:522-38. [Crossref] [PubMed]
- Ballak DB, Stienstra R, Tack CJ, et al. IL-1 family members in the pathogenesis and treatment of metabolic disease: Focus on adipose tissue inflammation and insulin resistance. Cytokine 2015;75:280-90. [Crossref] [PubMed]
- Proença MA, Biselli JM, Succi M, et al. Relationship between Fusobacterium nucleatum, inflammatory mediators and microRNAs in colorectal carcinogenesis. World J Gastroenterol 2018;24:5351-65. [Crossref] [PubMed]
- Nelson JE, Handa P, Aouizerat B, et al. Increased parenchymal damage and steatohepatitis in Caucasian non-alcoholic fatty liver disease patients with common IL1B and IL6 polymorphisms. Aliment Pharmacol Ther 2016;44:1253-64. [Crossref] [PubMed]