A methylation-related signature for predicting prognosis and sensitivity to first-line therapies in gastric cancer
Highlight box
Key findings
• Two gastric adenocarcinoma subtypes were identified successfully through unsupervised consensus clustering.
• A prognostic model based on five methylation regulators (CHAF1A, CPNE8, PHLDA3, SPARC, and EHF) was established. This model can be used to predict the prognosis and clinical effect of gastric adenocarcinoma patients.
What is known and what is new?
• The dysregulation of methylation is an important epigenetic modification mechanism in cancer, especially in gastrointestinal cancers.
• We established a methylation regulators-related prognostic model to promote the development of precision medicine in gastrointestinal cancers.
What is the implication, and what should change now?
• We clarified the potential clinical application of methylation regulators in gastric adenocarcinoma from a new perspective. It may benefit methylation regulators-targeted therapies in the future.
• Future diagnosis and treatment will further focus on molecular perspectives.
Introduction
Unfortunately, due to most cases being diagnosed at an advanced stage, gastric cancer (GC) has a high mortality, and ranks third as the most common cause of cancer-related death (1). GC’s risk factors consist of Helicobacter pylori gastritis, high salt intake, genetic background, and lack of vegetable and fruit intake (1-3). The main curative therapeutic modality for GC is surgical resection (4). Surgery remains an important intervention against GC, and endoscopic resection has been widely used for most early GC cases (5,6). However, chemotherapy is the first choice for patients with advanced GC (1). A combination of a platinum and a fluoropyrimidine agent constitutes the first-line of chemotherapy (1,7,8). More recently, the addition of a checkpoint inhibitor to chemotherapy brought only modest benefit to the overall population of GC patients (9). Additionally, several targeted therapeutic agents are used for advanced GC (10). About 17–20% patients with GC overexpress the human epidermal growth factor receptor 2 (HER2) protein, meaning that an anti-HER2 antibody, trastuzumab, can assist in treatment against GC (11). Treatment with pembrolizumab/nivolumab is based on testing for microsatellite instability (MSI) or mismatch repair (MMR), PD-L1 expression or tumor mutation burden (TMB) (10). Entrectinib/larotrectinib is based on testing for NTRK gene fusions (10). Despite these choices in therapy, the survival time of patients with advanced disease rarely exceeds 2 years (1). In order to maximize therapeutic effect, the latest findings in molecular biology need to be translated into clinical practice.
Methylation is an important epigenetic modification mechanism in cancer (12), occurring at DNA (13), RNA (14), and protein (15,16) levels. DNA methylation is involved in the regulation of target gene expression (17); RNA methylation is involved in the regulation of transcription, messenger RNA (mRNA) splicing, nuclear export, and translation (18); and protein methylation is involved in the regulation of activity, translation, localization, and signaling of protein (19). In recent years, many studies have demonstrated that the dysregulation of methylation is related to human cancer progression, especially in gastrointestinal cancers (20,21). Silencing of tumor-suppressor genes by aberrant methylation of CpG islands in the promoter of DNA is one of the major mechanisms causing GC (22). Over 150 types of RNA methylation have been identified, with N1-methyladenosine (m1A), 5-methylcytosine (m5C), N6-methyladenosine (m6A), N7-methylguanosine (m7G), and 2-O-dimethyladenosine (m6Am) being the most representative and intensively studied types (12). A study has shown that demethylase ALKBH5 regulates m6A modification of downstream target PKMYT1 to suppress the invasion and metastasis of GC (23). The RNA methyltransferase NSUN2 was demonstrated to inhibit the downstream gene CDKN1C, thus promoting GC cell proliferation via m5C modification (24). Abnormal m7G transfer RNA (tRNA) modification can promote hepatocarcinogenesis (25). Methylation modification of proteins is mainly found in histones, and this posttranslational modification participates in many cancer-related processes, such as transcription and DNA repair (26).
Studies on the epigenetic modification of nucleic acid and protein molecules remain insufficient, with research on crosstalk between different types of methylation in cancer being even less so. Methylation modification plays a great role in the development and diagnosis of gastric adenocarcinoma. In this study, we comprehensively evaluated the role played by methylation in gastric adenocarcinoma at the DNA, RNA, and protein level. The transcriptome, proteome, and genome data from The Cancer Genome Atlas (TCGA) and the Gene Expression Omnibus (GEO) database were collected to perform a series of bioinformatic analyses to investigate the potential application of patients’ classification based on methylation regulation in first-line clinical treatment. The signatures screened through different subtypes could predict patients’ overall survival (OS) and recurrence-free survival (RFS). We aimed to promote the application of precision medicine in GC patients via more refined stratification of gastric adenocarcinoma. The significance and innovation of this study are that it comprehensively considers all possible methylation regulation forms mentioned in existing studies and clarifies the association of methylation modifications with gastric adenocarcinoma-related pathological processes. We present this article in accordance with the TRIPOD reporting checklist (available at https://jgo.amegroups.com/article/view/10.21037/jgo-23-770/rc).
Methods
Acquisition of data of patients with gastric adenocarcinoma
We downloaded the RNA transcriptome datasets (HTSeq-Counts and HTSeq-FPKM) and the relevant clinical data from TCGA database (https://portal.gdc.cancer.gov/). RNA sequencing (RNA-seq) was converted to transcripts per kilobase million (TPM) values. Somatic mutation and copy number variation (CNV) were obtained from UCSC Xena (University California, Santa Cruz; https://xenabrowser.net/datapages/). The clinical information is shown in https://cdn.amegroups.cn/static/public/jgo-23-770-1.xls. In order to increase the accuracy of prognosis model, cases with missing OS values or OS values ≤30 days were excluded. Finally, 335 cases with gene expression values and survival times were obtained. External validation sets GSE84437 (27) and GSE26253 (28) were obtained from GEO database (http://www.ncbi.nlm.nih.gov/geo). GSE84437 contains 433 samples of patients with GC and complete OS and transcriptome data retrieved from the Affymetrix GPL6947 platform (Illumina HumanHT-12 v. 3.0 Expression BeadChips). GSE26253 contains 432 samples from patients with GC, with RFS and transcriptome data being retrieved from the Affymetrix GPL8432 platform (Illumina HumanRef-8 WG-DASL v. 3.0). All data from these platforms are public and with authorization for the study. The study was conducted in accordance with the Declaration of Helsinki (as revised in 2013).
Acquisition of methylation-related genes and mutational analysis
Through a review of the literature, a total of 117 methylation regulators were collected. The detailed information about these methylation regulators is shown in Table 1.
Table 1
Classification | Gene symbol | Source |
---|---|---|
DNA methylation | DNMT1, DNMT3A, DNMT3B, DNMT3L, TET1, TET2, TET3 | (29-31) |
RNA-N6-methyladenosine (m6A) | METTL3, METTL14, METTL16, WTAP, KIAA1429, VIRMA, RBMY1A1, RBM15, RBM15B, ZC3H13, FTO, ALKBH5, YTHDC1, YTHDC2, YTHDF1, YTHDF2, YTHDF3 IGF2BP1, IGF2BP2, IGF2BP3, HNRNPA2B1, HNRNPC, RBMX, LRPPRC, FMR1 | (32-34) |
RNA-N1-methyladenosine (m1A) | TRMT6, TRMT61A, TRMT61B, TRMT10C, BMT2 RRP8, YTHDF1, YTHDF2, YTHDF3, YTHDC1, ALKBH1, ALKBH3 | (20,35) |
RNA-N5-methylcytosine (m5C) | TET1, TET3, DNMT3B, YBX1, NSUN2, NSUN6, NOP2 | (36,37) |
RNA-N7-methylguanosine (m7G) | METTL1, WDR4, NSUN2, DCP2, DCPS, NUDT10, NUDT11, NUDT16, NUDT3, NUDT4, NUDT4B, AGO2, CYFIP1, EIF4E, EIF4E1B, EIF4E2, EIF4E3, GEMIN5, LARP1, NCBP1, NCBP2, NCBP3, EIF3D, EIF4A1, EIF4G3, IFIT5, LSM1, NCBP2L, SNUPN | (38-40) |
Protein methylation | PRMT1, PRMT2, PRMT3, PRMT4, PRMT5, PRMT6, PRMT7, PRMT8, PRMT9, SUV39H1, EHMT2, SETDB2, KMT2A, KMT2B, KMT2C, KMT2D, KMT2E, SETD2, NSD1, SMYD2, SMYD3, NSD2, NSD3, DOT1L, KMT5A, EZH2, SETD7, KDM1A, KDM1B, KDM2A, KDM2B, KDM3A, KDM3B, KDM4A, KDM4B, KDM4C, KDM4D, KDM4E, KDM5A, KDM5B, KDM5C, KDM5D, KDM6A, KDM6B, KDM7A, KDM8 | (26,41) |
The mutation map of methylation regulators in patients with gastric adenocarcinoma was generated by the “maftools” package in R. CNV-altered positions of methylation regulators on 23 chromosomes were mapped with the “RCircos” package in R.
Unsupervised consensus clustering
Unsupervised consensus clustering analysis is a method of providing quantitative evidence to determine the members and number of possible clusters in a dataset (42). This method has been widely applied in cancer genomics to discover new subtypes of disease molecules. It was performed to divide patients into distinct molecular subtypes based on methylation regulators expression via the “ConsensusClusterPlus” R package. The following criteria were used to group patients: first, there was a fluid and progressive growth in the cumulative distribution function curve. Second, there was a sufficient sample size in each group. Thirdly, ensure a balance between intra group correlation and inter group correlation. The “survival” and “survminer” R packages were used to conduct the Kaplan-Meier curve and compare the OS between different subtypes. Clinical data were included and analyzed for differences in molecular subtypes by using the “heatmap” R package.
To investigate the diversity in enrichment status for biological processes of the different subtypes, gene set variation analysis (GSVA) was carried out via the “GSVA” R packages. Gene set “c2.cp.kegg. v7.4” was used for GSVA. We identified differentially expressed genes (DEGs) between different subtypes via the “limma” R package according to a P value of 0. 05 and a |logFC| of 0.5. In order to verify the robustness of clustering, the samples were clustered again based on DEGs. The “clusterProfiler” R package was used to perform functional enrichment analyses on the DEGs.
Analysis of tumor microenvironment and clinical application for different subtypes
Single-sample gene set enrichment analysis (ssGSEA) was used to evaluate the levels of immune cell infiltration. We examined the expression of 47 immune checkpoint genes among different subtypes and selected out significantly differently expressed checkpoints. Data from Tumor Immune Dysfunction and Exclusion (TIDE) was used to predict the potential response of patients to immunotherapy (43). We used the one-class logistic regression (OCLR) machine learning algorithm to calculate the degree of oncogenic dedifferentiation (44). The stemness indices were scaled from 0 (low) to 1 (high). The “pRRophetic” R package was applied to calculate the half-maximal inhibitory concentration (IC50) of common chemotherapy drugs for the clinical treatment of gastric adenocarcinoma.
Establishment of the risk model
A risk model was constructed using the DEGs among the different subtypes. We first performed univariate Cox proportional hazard regression analysis to obtain prognosis-related signatures based on the transcriptome data in TCGA. All patients were randomly divided into training and testing groups in a 1:1 ratio. LASSO regression with 1,000 cycles of 10-fold cross-validation was then used to build the risk model. With the aim of avoiding overfitting, we completed 1,000 random stimulations in each cycle. The computational formula used for this analysis was as follows:
Here, the coef (genek) is the short form of the coefficient of genes correlated with survival, and expr (genek) is the expression of genes. We divided the samples into low-risk and high-risk groups based on the median risk score.
Evaluation of the risk model
We used the “survival” R package to carry out univariate Cox (uni-Cox) and multivariate Cox (multi-Cox) regression analyses to investigate whether the risk score and clinical characteristics were independent variable factors (45). To evaluate the accuracy of the model, we drew receiver operating characteristic (ROC) and concordance index (C-index) curves of different clinical characteristics and stages.
Construction of the nomogram
We used the “regplot” and “rms” R packages to establish a nomogram capable of predicting the 1-, 3-, and 5-year survival of patients with gastric adenocarcinoma. Score, age, sex, and tumor stage were incorporated into the nomogram. We additionally constructed a correction curve according to the Hosmer-Lemeshow test to evaluate the performance of this nomogram.
Analysis of the tumor microenvironment and clinical application for different risk groups
We used ssGSEA to evaluate the levels of immune cell infiltration among the risk groups, calculated the tumor microenvironment score, and compared the expression of immune checkpoints. Response for immunotherapy and chemotherapy was predicted as described above.
Statistical analysis
All statistical tests were performed using R4.1.3, including the two-sample Mann-Whitney test for continuous data, Fisher’s exact test or chi-square test for categorical data, log-rank test for Kaplan-Meier curves, Hosmer-Lemeshow test for nomogram and Cox proportional hazards regression for estimating hazard ratios (HRs) and 95% confidence intervals (CIs). Correlation coefficients between different genes were estimated via Pearson correlation analysis. All statistical P values were two-sided, and P<0.05 was considered statistically significant.
Results
Genomic alterations of methylation regulators in gastric adenocarcinoma
We explored the incidence of somatic mutations and CNVs for 117 methylation regulators in patients with gastric adenocarcinoma. The results showed that genomic alterations in methylation regulators occurred in 248 (57.54%) of 431 samples (Figure 1A). Of note, KMT2D (16%) was the gene with the highest mutation frequency, followed by KMT2C (13%), KMT2B (12%), KMT2A (9%), and ZC3H13 (7%). All methylation regulators had prevalent CNVs (Figure 1B). AGO2, IGF2BP2, NCBP2, SMYD3, VIRMA, and EHMT2 had significant copy number amplification, while EIF4G3, KDM1A, KDM7A, KMT2C, and EZH2 had significant copy number deletions. The locations of significant CNVs in methylation regulators on chromosomes are shown in Figure 1C. Taken together, these results suggested that genomic alterations may create abnormalities in methylation regulators and thus contribute to the development of gastric adenocarcinoma.
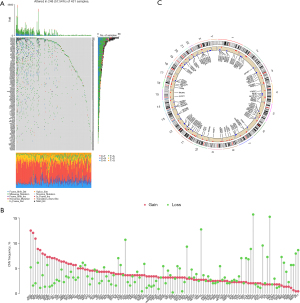
Identification of methylation-related subtypes
Based on the 117 methylation regulators’ expression profiles, we used an unsupervised consensus clustering approach to classify the patients with gastric adenocarcinoma in TCGA cohort. Our analysis indicated that K=2 was the best choice of subtypes with the highest correlation within clusters and the least interference between clusters (Figure 2A,2B). Therefore, we divided patients into two subtypes: cluster A and cluster B. There were 111 patients in cluster A and 224 patients in cluster B. According to the Kaplan-Meier curve, patients in cluster B were found to have a better OS than those in cluster A (P<0.001; Figure 2C). A heatmap revealed differences in methylation regulators’ expression between cluster A and cluster B (Figure 2D), with highly significant difference among the subtypes. Most methylation regulators had a high expression level in cluster B. The univariate and multivariate Cox analyses were performed to explore the independent prognostic factor. Tumor stage and cluster were independent prognostic factors in univariate Cox analysis, while tumor stage, age and cluster were independent prognostic factors in multivariate Cox analysis (Figure S1A,S1B).
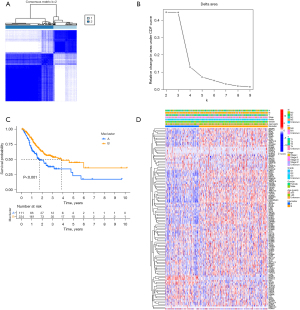
Characterization of the tumor microenvironment and clinical application for different subtypes
We further investigated the differences in molecular characteristics, tumor microenvironment, and first-line therapy between the two subtypes. GSVA enrichment analysis showed that glyoxylate and dicarboxylate metabolism, base excision repair, pyrimidine metabolism, aminoacyl tRNA biosynthesis, non-homologous end joining, homologous recombination, DNA replication, MMR, spliceosome, cell cycle, nucleotide excision repair, and RNA degradation were enriched in cluster B as compared with cluster A (Figure 3A). Most of the pathways enriched in cluster B were related to nucleic acid metabolism, and thus therapies that interfere with related biological processes may be more effective for patients in cluster B. Calcium signaling pathway, vascular smooth muscle contraction, neuroactive ligand-receptor interaction and glycosphingolipid biosynthesis—ganglion series were enriched in cluster A. The result of ssGSEA showed that the infiltration of 17 immune cell subsets were significantly different between the two subtypes (Figure 3B). The expression immune checkpoints of the two subtypes were also investigated: the expression of LAIR1, CD40LG, TNFSF18, CD276, CD28, CD40, BTNL2, CD200, CD48, and NRP1 were higher in cluster A, while LAG3, HHLA2, TNFRSF25, CD80, TNFRSF14, LGALS9, IDO1, CD274, and CTLA4 were higher in cluster B (Figure 3C). TIDE score was employed to predict the treatment effects of immunotherapy. The higher the TIDE score is, the less effective the immune checkpoint blockade (ICB) will be: cluster A showed a higher TIDE score than cluster B (Figure 3D). Tumor mutational burden (TMB) and MSI could be predictive biomarkers for immunotherapeutic response (46): cluster B had a higher level of TMB than cluster A (Figure 3E). A greater proportion of patients in cluster A were microsatellite-stable (MSS), while a greater proportion of patients in cluster B showed high microsatellite instability (MSI-H) (Figure 3F). Stemness score was used to evaluate the similarity between tumor cells and stem cells. A higher stemness score may suggest a greater invasivity and drug resistance of tumor cells. The stemness score of cluster B was higher than that of cluster A (Figure 3G). The expression of target genes with therapeutic promise also differed between the two subtypes. ERBB2 and CDH1 were highly expressed in cluster B, while PIK3CA and VEGFR2 were highly expressed in cluster A (Figure 3H-3K).
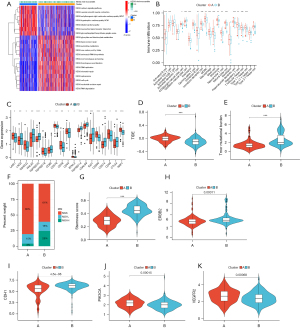
In order to investigate the sensitivity to chemotherapeutic agents, we calculated the IC50 of the commonly used anticancer medicines in gastric adenocarcinoma. We found that patients in in cluster B may be more sensitive to cisplatin, etoposide and paclitaxel (Figure S2A).
Identification of DEGs and validation of clustering
A total of 1,578 DEGs related to the two subtypes were identified via the “limma” R package. We performed functional enrichment analysis based on these DEGs. Gene Ontology (GO) enrichment analysis showed that these DEGs were linked to biological processes such as extracellular matrix organization and cell cycle (Figure 4A). Kyoto Encyclopedia of Genes and Genomes (KEGG) enrichment analysis showed a similar result of cancer-related and cell cycle-related pathways being enriched (Figure 4B). The results demonstrated the significance of the dysregulation of methylation regulators in cancer-related pathological process, especially the cell cycle process. Aiming to verify the stability of clustering, we used a consensus clustering approach based on the DEGs to classify patients again. We obtained two subtypes which showed a significant difference in OS (Figure 4C,4D). Meanwhile, we used external validation sets (GSE84437 with OS and GSE26253 with RFS) to perform consensus clustering and obtained similar results (Figure 4E,4F).
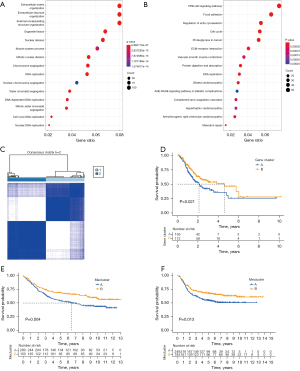
Establishment of the risk model
In the uni-Cox regression analysis, we obtained 66 methylation-related signatures correlated with patients’ OS (Figure 5A). After the LASSO regression analysis, we obtained 5 methylation-related signatures when the first-rank value of Log(λ) was the minimum likelihood of deviance (Figure 5B,5C).
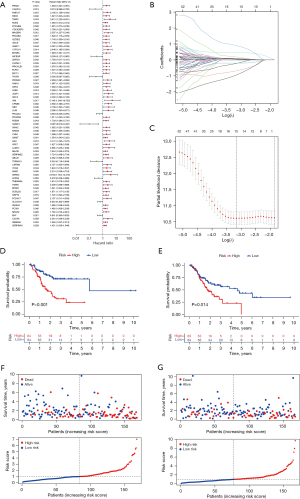
The risk score was calculated with the following formula: risk score = exp CHAF1A × (–1.56998715109262) + exp CPNE8 ×0.870527847113839+ exp PHLDA3 × (–1.41564641545321) + exp SPARC ×2.02578425941195+ exp EHF × (–0.521746288297191).
Via calculation, we obtained the risk score of each sample and divided the samples into a high-risk group (n=174) and low-risk group (n=161). The Kaplan-Meier curves of both the training set (Figure 5D) and test set (Figure 5E) showed that the high-risk group had a significantly poorer prognosis. The survival status and risk curves for the training and test sets are shown in Figure 5F,5G. Meanwhile, this model was also applicable to patients with different clinical characteristics (stage, age, and gender) (Figure 6A-6F). A boxplot showed that cluster B had a significantly lower risk score than cluster A, which illustrated the robustness of the consensus clustering (Figure 6G). The risk model also exhibited excellent ability of classification in the external validation sets (GSE84437 with OS and GSE26253 with RFS) (Figure 6H,6I).
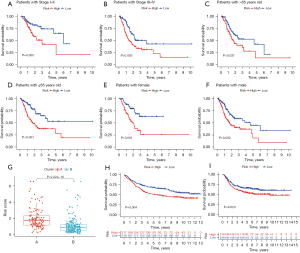
Evaluation of the risk model
In the uni-Cox regression analysis, the HR of the risk score was 1.308 and the 95% CI was 1.169−1.464 (P<0.001) (Figure 7A). In the multi-Cox regression analysis, the HR of the risk score was 1.384 and the 95% CI was 1.227−1.560 (P<0.001) (Figure 7B). This suggested that the risk score could act as an independent prognostic factor. We evaluated the outcomes of ROC using the area under the ROC curve (AUC). In this model, the 1-year AUC of the risk score was 0.712, and the AUCs of age, gender, grade, and stage were 0.573, 0.522, 0.556, and 0.594 respectively (Figure 7C). The 1-, 3-, and 5-year AUC of risk score was 0.712, 0.696, and 0.759 (Figure 7D). Meanwhile, the risk model had the highest c-index among age, gender, stage, and grade (Figure 7E).
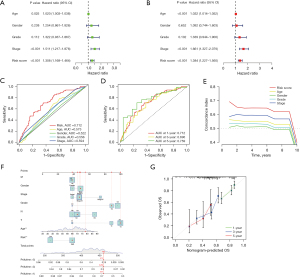
Construction of the nomogram
We constructed a nomogram to predict the 1-, 3-, and 5-year survival probability of patients with gastric adenocarcinoma (Figure 7F). The factors in the nomogram included risk score, age, gender, grade, and TNM stage. We then drew a calibration plot to evaluate the accuracy of the nomogram (Figure 7G), with the results indicating that this nomogram had the ability to predict patients’ risks.
Characterization of the tumor microenvironment and therapy response for the risk model
We evaluated the infiltration levels of immune cells in the tumor microenvironment and found that the levels of activated CD4 T cells, CD56 dim natural killer cells, eosinophil, gamma delta T cells, immature dendritic cells, myeloid-derived suppressor cell (MDSC), macrophages, mast cells, natural killer T cells, plasmacytoid dendritic cells, regulatory T cells, T follicular helper cells, and type 1 T helper cells were significantly different between the two groups (Figure 8A). We then focused on immune checkpoints, which were found to be more active in the high-risk group, except for TNFRSF14, LGALS9, and TNFSF9 (Figure 8B). The high-risk group had a high TMB level (Figure 8C), while the proportion of MSS and MSI-H also differed between the groups: a greater proportion of patients in the high-risk group were MSS, while a greater proportion of patients in the low-risk group were MSI-H (Figure 8D). Meanwhile, the high-risk group had a higher TIDE score compared with the low-risk group (Figure 8E). We explored the sensitivity of the two groups to chemotherapy and found that the IC50 of etoposide and paclitaxel were lower in the low-risk group (Figure S2B) The results of the analysis in the risk model were highly consistent with those in the subtypes, once again demonstrating the robustness of the consensus clustering method.
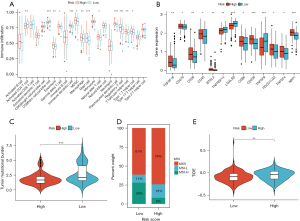
Discussion
GC is one of the most dangerous malignant tumors in the digestive system and is a disease of global concern (1). Gastric adenocarcinoma is the most common histological type of GC. Due to the presence of tumor heterogeneity, the response of patients with gastric adenocarcinoma to clinical treatment varies widely. Therefore, the classification of GC subtypes is crucial for the effectiveness and accuracy of treatment. Methylation plays a significant role in the occurrence and progression of cancer, especially in gastrointestinal cancers (20,47). Recently, many studies have built models based on m6A to predict the prognosis of patients with cancer and achieved good results (48-50). However, few studies have summarized and analyzed all methylation types, and an investigation of the possible link between all methylation types and the clinical characteristics of gastric adenocarcinoma has yet to be reported.
In this study, we identified two distinct methylation-related subtypes based on 117 methylation regulators. There were significant differences in OS, RFS, molecular characteristics, tumor microenvironment, immunotherapy sensitivity, and chemotherapy sensitivity among the different subtypes. According to DEGs between the two subtypes, we constructed a risk model to predict the prognosis of patients. We found 66 DEGs to be relevant to the prognosis of gastric adenocarcinoma in Cox regression analysis. Among these, we identified five signatures (CHAF1A, CPNE8, PHLDA3, SPARC, and EHF) and constructed the risk model using LASSO regression analysis. We calculated the risk score and divided patients into a high-risk group and low-risk group accordingly. The high-risk group had a worse prognosis than the low-risk group. The ROC curve and internal data set verification proved that this model had good predictive performance. The performance of this model was better in predicting the prognosis of patients than were certain clinical indices, such as stage and grade.
The waterfall plot showed that KMT2D, KMT2C, KMT2B, KMT2A, and ZC3H13 had a high level of mutation in patients with gastric adenocarcinoma. The lysine methyltransferase 2 (KMT2) family proteins methylate the Lys-4 position of histone H3, which is an important regulatory region of the genome. Genomic mutations in the KMT2 family may serve as potential predictors of response to immune checkpoint therapy (ICT) in patients with a variety of cancers (51). This may be related to the fact that KMT2 family mutations confer an increased TMB, which enhances tumor immunogenicity (51). ZC3H13 encodes the zinc finger CCCH domain-containing protein 13, acting as a key regulator of m6A methylation by promoting m6A methylation of mRNAs at the 3' untranslated region (3'-UTR) (52). ZC3H13 was found to be able to inactivate the Ras-ERK signaling pathway and suppress invasion and proliferation of colorectal cancer (53). GSVA results showed that pathways associated with cell cycle and nucleic acid processing were enriched in cluster B. Some methylation regulators may participate in these processes. Human DCP2 protein participates in the decapping of mRNA through specifically hydrolyzing methylated-capped RNA to release 7-methylguanosine 5’ diphosphate (m7GDP) (54). Eukaryotic translation initiation factor 4E (EIF4E) can regulate translation, nuclear export, and tumor progression by recognizing the m7G cap of mRNA (55), and this distinctive cap-binding activity makes it a tumor suppressor (55). NUDT10 is a member of the nucleoside diphosphate-linked moiety X (NUDIX) hydroxylases. Research indicates that for patients with GC, NUDT10 expression is related to the disease stage, level of local invasion, and survival outcome (56). POLR2I encodes a subunit of RNA polymerase II, the polymerase responsible for synthesizing mRNA in eukaryotes and has potential as a novel biomarker for colorectal cancer metastasis (57). GTF2F2 encodes general transcription factor IIF subunit 2, promoting transcription elongation. It may regulate neurite outgrowth in neuroblastoma IMR-32 cells (58).
Patients with malignant tumors with a high-level of somatic mutations are more likely to benefit from immunotherapy (59-61). Most patients with GC have a high somatic mutation rate, which indicates that immunotherapy may be of particular benefit (62). However, many clinical studies showed that the response rate of GC patients to immunotherapy was still quite different (63,64). Thus, it is critical to accurately divide patients with GC into different subtypes to improve the response rate of immunotherapy. Programmed death-ligand 1 (PD-L1) combined positive score (CPS), MSI-H, and TMB are regarded as promising signatures for indicating a greater efficacy of immunotherapy (65,66). Methylation regulators are involved in epigenetic regulation and are closely related to the stability of the genome. Classification based on methylation regulators may help to identify those tumors most responsive to immunotherapy (hot tumors) (67). The results of our study indicated that patients in cluster B tended to have a high TMB and MSI-H status. The TIDE score also suggested that the immune checkpoint blockade (ICB) may be more effective in cluster B. Furthermore, immune checkpoint molecules including CD274 (PD-L1) and CTLA4 were highly expressed in cluster B. Thus, we identified cluster B as a hot tumor, and patients in cluster B may be more sensitive to immunotherapy. Meanwhile, the identification of DEGs such as ERBB2, CDH1, PIK3CA and VEGFR2 may help to develop personalized treatment. HER-2 (ERBB2), belonging to the epidermal growth factor receptor (EGFR) family, activates downstream pathways via heterodimer and tyrosine kinase autophosphorylation (68). Trastuzumab, the HER-2 inhibitor, is the only targeted drug for the first-line treatment of advanced Gastric Adenocarcinoma. Based on this, numerous small-molecule drugs targeted to HER-2 are considered to have good prospects (69). Abnormal activation of receptor protein tyrosine kinases (RPTKs)-related pathways leads to GC development (70). Therapies targeting the RPTKs such as PIK3CA and PTEN represent promising treatments for GC. EGFR and VEGFR2 can be targeted by nimotuzumab and AZD4547, and this approach has been shown to achieve good therapeutic effect (70).
Some limitations to our study should be noted. All samples in our study were collected retrospectively from public databases, and our results still need to be validated in large-scale prospective cohorts. Furthermore, despite efforts to ensure its accuracy, some defects and deficiencies may be present in the risk model. Relevant research in the field of molecular biology is ongoing, and the model should be modified according to the most up-to-date findings.
Conclusions
Our study clarified the potential clinical application of methylation regulators in gastric adenocarcinoma from a new perspective. Classification based on methylation regulators could be applied to identify more effective treatments and build models to predict the prognosis of patients with gastric adenocarcinoma. Grouping research based on our study may promote the development of precision medicine in gastric adenocarcinoma and thus potentially benefit these patients.
Acknowledgments
Funding: This project was supported by
Footnote
Reporting Checklist: The authors have completed the TRIPOD reporting checklist. Available at https://jgo.amegroups.com/article/view/10.21037/jgo-23-770/rc
Peer Review File: Available at https://jgo.amegroups.com/article/view/10.21037/jgo-23-770/prf
Conflicts of Interest: All authors have completed the ICMJE uniform disclosure form (available at https://jgo.amegroups.com/article/view/10.21037/jgo-23-770/coif). Z.L. reports funding support from Natural Science Foundation of Hubei Province (No. 2021CFB371). Hua Xiong reports funding support from the Natural Science Foundation of Hubei Province (No. 2021CFB372). The other authors have no conflicts of interest to declare.
Ethical Statement: The authors are accountable for all aspects of the work in ensuring that questions related to the accuracy or integrity of any part of the work are appropriately investigated and resolved. The study was conducted in accordance with the Declaration of Helsinki (as revised in 2013).
Open Access Statement: This is an Open Access article distributed in accordance with the Creative Commons Attribution-NonCommercial-NoDerivs 4.0 International License (CC BY-NC-ND 4.0), which permits the non-commercial replication and distribution of the article with the strict proviso that no changes or edits are made and the original work is properly cited (including links to both the formal publication through the relevant DOI and the license). See: https://creativecommons.org/licenses/by-nc-nd/4.0/.
References
- Smyth EC, Nilsson M, Grabsch HI, et al. Gastric cancer. Lancet 2020;396:635-48. [Crossref] [PubMed]
- Oliveira C, Pinheiro H, Figueiredo J, et al. Familial gastric cancer: genetic susceptibility, pathology, and implications for management. Lancet Oncol 2015;16:e60-70. [Crossref] [PubMed]
- Uemura N, Okamoto S, Yamamoto S, et al. Helicobacter pylori infection and the development of gastric cancer. N Engl J Med 2001;345:784-9. [Crossref] [PubMed]
- Pinheiro RN, Mucci S, Zanatto RM, et al. Quality of life as a fundamental outcome after curative intent gastrectomy for adenocarcinoma: lessons learned from patients. J Gastrointest Oncol 2019;10:989-98. [Crossref] [PubMed]
- Pimentel-Nunes P, Dinis-Ribeiro M, Ponchon T, et al. Endoscopic submucosal dissection: European Society of Gastrointestinal Endoscopy (ESGE) Guideline. Endoscopy 2015;47:829-54. [Crossref] [PubMed]
- Hatta W, Gotoda T, Koike T, et al. History and future perspectives in Japanese guidelines for endoscopic resection of early gastric cancer. Dig Endosc 2020;32:180-90. [Crossref] [PubMed]
- Yamada Y, Higuchi K, Nishikawa K, et al. Phase III study comparing oxaliplatin plus S-1 with cisplatin plus S-1 in chemotherapy-naïve patients with advanced gastric cancer. Ann Oncol 2015;26:141-8. [Crossref] [PubMed]
- Smyth EC, Verheij M, Allum W, et al. Gastric cancer: ESMO Clinical Practice Guidelines for diagnosis, treatment and follow-up. Ann Oncol 2016;27:v38-49. [Crossref] [PubMed]
- Janjigian YY, Shitara K, Moehler M, et al. First-line nivolumab plus chemotherapy versus chemotherapy alone for advanced gastric, gastro-oesophageal junction, and oesophageal adenocarcinoma (CheckMate 649): a randomised, open-label, phase 3 trial. Lancet 2021;398:27-40. [Crossref] [PubMed]
- Ajani JA, D'Amico TA, Bentrem DJ, et al. Gastric Cancer, Version 2.2022, NCCN Clinical Practice Guidelines in Oncology. J Natl Compr Canc Netw 2022;20:167-92. [Crossref] [PubMed]
- Comprehensive molecular characterization of gastric adenocarcinoma. Nature 2014;513:202-9. [Crossref] [PubMed]
- Dai X, Ren T, Zhang Y, et al. Methylation multiplicity and its clinical values in cancer. Expert Rev Mol Med 2021;23:e2. [Crossref] [PubMed]
- Kachroo P, Morrow JD, Vyhlidal CA, et al. DNA methylation perturbations may link altered development and aging in the lung. Aging (Albany NY) 2021;13:1742-64. [Crossref] [PubMed]
- He PC, He C. m(6) A RNA methylation: from mechanisms to therapeutic potential. EMBO J 2021;40:e105977. [Crossref] [PubMed]
- Di Blasi R, Blyuss O, Timms JF, et al. Non-Histone Protein Methylation: Biological Significance and Bioengineering Potential. ACS Chem Biol 2021;16:238-50. [Crossref] [PubMed]
- Zhao S, Chuh KN, Zhang B, et al. Histone H3Q5 serotonylation stabilizes H3K4 methylation and potentiates its readout. Proc Natl Acad Sci U S A 2021;118:e2016742118. [Crossref] [PubMed]
- Robertson KD, Jones PA. DNA methylation: past, present and future directions. Carcinogenesis 2000;21:461-7. [Crossref] [PubMed]
- Frye M, Harada BT, Behm M, et al. RNA modifications modulate gene expression during development. Science 2018;361:1346-9. [Crossref] [PubMed]
- Lanouette S, Mongeon V, Figeys D, et al. The functional diversity of protein lysine methylation. Mol Syst Biol 2014;10:724. [Crossref] [PubMed]
- Xie S, Chen W, Chen K, et al. Emerging roles of RNA methylation in gastrointestinal cancers. Cancer Cell Int 2020;20:585. [Crossref] [PubMed]
- Liang WW, Lu RJ, Jayasinghe RG, et al. Integrative multi-omic cancer profiling reveals DNA methylation patterns associated with therapeutic vulnerability and cell-of-origin. Cancer Cell 2023;41:1567-1585.e7. [Crossref] [PubMed]
- Usui G, Matsusaka K, Mano Y, et al. DNA Methylation and Genetic Aberrations in Gastric Cancer. Digestion 2021;102:25-32. [Crossref] [PubMed]
- Hu Y, Gong C, Li Z, et al. Demethylase ALKBH5 suppresses invasion of gastric cancer via PKMYT1 m6A modification. Mol Cancer 2022;21:34. [Crossref] [PubMed]
- Mei L, Shen C, Miao R, et al. RNA methyltransferase NSUN2 promotes gastric cancer cell proliferation by repressing p57(Kip2) by an m(5)C-dependent manner. Cell Death Dis 2020;11:270. [Crossref] [PubMed]
- Chen Z, Zhu W, Zhu S, et al. METTL1 promotes hepatocarcinogenesis via m(7) G tRNA modification-dependent translation control. Clin Transl Med 2021;11:e661. [Crossref] [PubMed]
- Audia JE, Campbell RM. Histone Modifications and Cancer. Cold Spring Harb Perspect Biol 2016;8:a019521. [Crossref] [PubMed]
- Yoon SJ, Park J, Shin Y, et al. Deconvolution of diffuse gastric cancer and the suppression of CD34 on the BALB/c nude mice model. BMC Cancer 2020;20:314. [Crossref] [PubMed]
- Oh SC, Sohn BH, Cheong JH, et al. Clinical and genomic landscape of gastric cancer with a mesenchymal phenotype. Nat Commun 2018;9:1777. [Crossref] [PubMed]
- Jia D, Jurkowska RZ, Zhang X, et al. Structure of Dnmt3a bound to Dnmt3L suggests a model for de novo DNA methylation. Nature 2007;449:248-51. [Crossref] [PubMed]
- Borgel J, Guibert S, Li Y, et al. Targets and dynamics of promoter DNA methylation during early mouse development. Nat Genet 2010;42:1093-100. [Crossref] [PubMed]
- Smith ZD, Meissner A. DNA methylation: roles in mammalian development. Nat Rev Genet 2013;14:204-20. [Crossref] [PubMed]
- An Y, Duan H. The role of m6A RNA methylation in cancer metabolism. Mol Cancer 2022;21:14. [Crossref] [PubMed]
- Hu BB, Wang XY, Gu XY, et al. N(6)-methyladenosine (m(6)A) RNA modification in gastrointestinal tract cancers: roles, mechanisms, and applications. Mol Cancer 2019;18:178. [Crossref] [PubMed]
- Li Y, Xiao J, Bai J, et al. Molecular characterization and clinical relevance of m(6)A regulators across 33 cancer types. Mol Cancer 2019;18:137. [Crossref] [PubMed]
- He R, Man C, Huang J, et al. Identification of RNA Methylation-Related lncRNAs Signature for Predicting Hot and Cold Tumors and Prognosis in Colon Cancer. Front Genet 2022;13:870945. [Crossref] [PubMed]
- Chen H, Yao J, Bao R, et al. Cross-talk of four types of RNA modification writers defines tumor microenvironment and pharmacogenomic landscape in colorectal cancer. Mol Cancer 2021;20:29. [Crossref] [PubMed]
- Chen YS, Yang WL, Zhao YL, et al. Dynamic transcriptomic m(5) C and its regulatory role in RNA processing. Wiley Interdiscip Rev RNA 2021;12:e1639. [Crossref] [PubMed]
- Tomikawa C. 7-Methylguanosine Modifications in Transfer RNA (tRNA). Int J Mol Sci 2018;19:4080. [Crossref] [PubMed]
- Zhou W, Wang X, Chang J, et al. The molecular structure and biological functions of RNA methylation, with special emphasis on the roles of RNA methylation in autoimmune diseases. Crit Rev Clin Lab Sci 2022;59:203-18. [Crossref] [PubMed]
- Dai Z, Liu H, Liao J, et al. N(7)-Methylguanosine tRNA modification enhances oncogenic mRNA translation and promotes intrahepatic cholangiocarcinoma progression. Mol Cell 2021;81:3339-3355.e8. [Crossref] [PubMed]
- Blanc RS, Richard S. Arginine Methylation: The Coming of Age. Mol Cell 2017;65:8-24. [Crossref] [PubMed]
- Wilkerson MD, Hayes DN. ConsensusClusterPlus: a class discovery tool with confidence assessments and item tracking. Bioinformatics 2010;26:1572-3. [Crossref] [PubMed]
- Jiang P, Gu S, Pan D, et al. Signatures of T cell dysfunction and exclusion predict cancer immunotherapy response. Nat Med 2018;24:1550-8. [Crossref] [PubMed]
- Malta TM, Sokolov A, Gentles AJ, et al. Machine Learning Identifies Stemness Features Associated with Oncogenic Dedifferentiation. Cell 2018;173:338-354.e15. [Crossref] [PubMed]
- Wang Q, Weng S, Sun Y, et al. High DAPK1 Expression Promotes Tumor Metastasis of Gastric Cancer. Biology (Basel) 2022;11:1488. [Crossref] [PubMed]
- Ock CY, Hwang JE, Keam B, et al. Genomic landscape associated with potential response to anti-CTLA-4 treatment in cancers. Nat Commun 2017;8:1050. [Crossref] [PubMed]
- Song P, Tayier S, Cai Z, et al. RNA methylation in mammalian development and cancer. Cell Biol Toxicol 2021;37:811-31. [Crossref] [PubMed]
- Zhang P, Liu G, Lu L. N6-Methylandenosine-Related lncRNA Signature Is a Novel Biomarkers of Prognosis and Immune Response in Colon Adenocarcinoma Patients. Front Cell Dev Biol 2021;9:703629. [Crossref] [PubMed]
- Song W, Ren J, Yuan W, et al. N6-Methyladenosine-Related lncRNA Signature Predicts the Overall Survival of Colorectal Cancer Patients. Genes (Basel) 2021;12:1375. [Crossref] [PubMed]
- Zhao J, Lin X, Zhuang J, et al. Relationships of N6-Methyladenosine-Related Long Non-Coding RNAs With Tumor Immune Microenvironment and Clinical Prognosis in Lung Adenocarcinoma. Front Genet 2021;12:714697. [Crossref] [PubMed]
- Zhang P, Huang Y. Genomic alterations in KMT2 family predict outcome of immune checkpoint therapy in multiple cancers. J Hematol Oncol 2021;14:39. [Crossref] [PubMed]
- Yue Y, Liu J, Cui X, et al. VIRMA mediates preferential m(6)A mRNA methylation in 3'UTR and near stop codon and associates with alternative polyadenylation. Cell Discov 2018;4:10. [Crossref] [PubMed]
- Zhu D, Zhou J, Zhao J, et al. ZC3H13 suppresses colorectal cancer proliferation and invasion via inactivating Ras-ERK signaling. J Cell Physiol 2019;234:8899-907. [Crossref] [PubMed]
- Wang Z, Jiao X, Carr-Schmid A, et al. The hDcp2 protein is a mammalian mRNA decapping enzyme. Proc Natl Acad Sci U S A 2002;99:12663-8. [Crossref] [PubMed]
- Osborne MJ, Volpon L, Kornblatt JA, et al. eIF4E3 acts as a tumor suppressor by utilizing an atypical mode of methyl-7-guanosine cap recognition. Proc Natl Acad Sci U S A 2013;110:3877-82. [Crossref] [PubMed]
- Chen D, Zhang R, Xie A, et al. Clinical correlations and prognostic value of Nudix hydroxylase 10 in patients with gastric cancer. Bioengineered 2021;12:9779-89. [Crossref] [PubMed]
- Long NP, Lee WJ, Huy NT, et al. Novel Biomarker Candidates for Colorectal Cancer Metastasis: A Meta-analysis of In Vitro Studies. Cancer Inform 2016;15:11-7. [Crossref] [PubMed]
- Tong CW, Wang JL, Jiang MS, et al. Novel genes that mediate nuclear respiratory factor 1-regualted neurite outgrowth in neuroblastoma IMR-32 cells. Gene 2013;515:62-70. [Crossref] [PubMed]
- Hodi FS, O'Day SJ, McDermott DF, et al. Improved survival with ipilimumab in patients with metastatic melanoma. N Engl J Med 2010;363:711-23. [Crossref] [PubMed]
- Brahmer JR, Tykodi SS, Chow LQ, et al. Safety and activity of anti-PD-L1 antibody in patients with advanced cancer. N Engl J Med 2012;366:2455-65. [Crossref] [PubMed]
- Donato EM, Fernández-Zarzoso M, De La Rubia J. Immunotherapy for the treatment of Hodgkin lymphoma. Expert Rev Hematol 2017;10:417-23. [Crossref] [PubMed]
- Marrelli D, Polom K, Pascale V, et al. Strong Prognostic Value of Microsatellite Instability in Intestinal Type Non-cardia Gastric Cancer. Ann Surg Oncol 2016;23:943-50. [Crossref] [PubMed]
- Shitara K, Özgüroğlu M, Bang YJ, et al. Pembrolizumab versus paclitaxel for previously treated, advanced gastric or gastro-oesophageal junction cancer (KEYNOTE-061): a randomised, open-label, controlled, phase 3 trial. Lancet 2018;392:123-33. [Crossref] [PubMed]
- Kang YK, Boku N, Satoh T, et al. Nivolumab in patients with advanced gastric or gastro-oesophageal junction cancer refractory to, or intolerant of, at least two previous chemotherapy regimens (ONO-4538-12, ATTRACTION-2): a randomised, double-blind, placebo-controlled, phase 3 trial. Lancet 2017;390:2461-71. [Crossref] [PubMed]
- Mariathasan S, Turley SJ, Nickles D, et al. TGFβ attenuates tumour response to PD-L1 blockade by contributing to exclusion of T cells. Nature 2018;554:544-8. [Crossref] [PubMed]
- Kim ST, Cristescu R, Bass AJ, et al. Comprehensive molecular characterization of clinical responses to PD-1 inhibition in metastatic gastric cancer. Nat Med 2018;24:1449-58. [Crossref] [PubMed]
- Galon J, Bruni D. Approaches to treat immune hot, altered and cold tumours with combination immunotherapies. Nat Rev Drug Discov 2019;18:197-218. [Crossref] [PubMed]
- Tebbutt N, Pedersen MW, Johns TG. Targeting the ERBB family in cancer: couples therapy. Nat Rev Cancer 2013;13:663-73. [Crossref] [PubMed]
- Zhu Y, Zhu X, Wei X, et al. HER2-targeted therapies in gastric cancer. Biochim Biophys Acta Rev Cancer 2021;1876:188549. [Crossref] [PubMed]
- Chia NY, Tan P. Molecular classification of gastric cancer. Ann Oncol 2016;27:763-9. [Crossref] [PubMed]