Upregulated expression of PTTG1 is associated with progression of pancreatic cancer
Highlight box
Key findings
• This study demonstrates that pituitary tumor transforming gene 1 (PTTG1) was highly expressed in pancreatic cancer (PC) tissues based on The Cancer Genome Atlas, Gene Expression Omnibus and immunohistochemistry databases. Meanwhile, we also demonstrate that PTTG1 may serve as a driving gene associated with the occurrence and progression of PC.
What is known and what is new?
• Research has confirmed that PTTG1 participates in the occurrence and development of many tumors, such as glioma, lung cancer, laryngeal cancer, and prostate cancer.
• The upregulated expression of PTTG1 plays an essential role in PC’s progression as a biomarker.
What is the implication, and what should change now?
• Our finding may contribute to prolong patients’ survival time with PC.
Introduction
Pancreatic cancer (PC), as one of the most aggressive human solid malignancies, is the 4th-highest cause of cancer morbidity and mortality in the USA and the 7th worldwide. Patients with PC, including in China, face a very high risk of mortality and extremely poor prognosis, and the incidence of PC has displayed a clear ascendant trend throughout the years. In America, for the patients with PC, overall survival (OS) is dismal and the 5-year survival rate of approximately 9% has not shown significant improvement compared with other cancer types. In addition, PC is anticipated to become the 2nd or 3rd most common cause of cancer deaths in high-income countries in the next years (1). However, PC is often silent as most patients remain asymptomatic in its early stages and are initially diagnosed at an advanced stage, for which few effective therapies exist (2-5). Currently, therapeutic strategies such as surgical excision and chemotherapy are the main treatment options for patients, but they remain unsatisfactory due to the relatively few operation opportunities, drug resistance, and cancer recurrence (6,7). Molecular markers that could be used to accurately predict the course of the disease or response to therapy have not yet been applied in the treatment of PC. Therefore, molecular biomarkers and targeted therapy may bring new hope for early diagnosis and management of PC (7). For instance, Du et al. utilized the overexpression of SGLT-2 to predict the prognosis of the patients with pancreatic ductal adenocarcinoma (8). There is a critical need at this juncture for new strategies to explore the molecular mechanism of PC and to improve its diagnosis and treatment.
Pituitary tumor transforming gene 1 (PTTG1), a recently characterized protooncogene, resides at human chromosome 5q33.3 and encodes the mammalian protein securin, originally isolated from rat pituitary tumor cells (9). PTTG1 has an essential role in cell-cycle regulation and sister chromatid separation during mitosis (10) and its activation has been associated with the regulation of growth and progression in many types of cancer cells (11). Accumulating evidence suggests that PTTG1 is a potential biomarker for cancer malignancy and a cell-cycle regulatory protein involved in a variety of cellular processes (12). A published study has shown that PTTG1 in hepatocellular carcinoma may be an independent prognostic indicator and potential therapeutic target by upregulating c-myc (13). One previous study has shown that PTTG1 expression has an association with the expression of bFGF and VEGF, therefore contributing to cell proliferation and migration (14). In addition, aberrant expression of PTTG1 is highly immunogenic and is an important target for immunotherapy of multiple myeloma (15). Previous data reported that altered levels of PTTG1 are expressed in breast cancer cells, suggesting that PTTG1 has a role in breast tumorigenesis (16). Similarly, a recent paper showed that PTTG1 is known to be down-regulated in thyroid cancer cells (17). PTTG1 has been verified to be abundantly expressed in many tumors, such as glioma (18), lung cancer (19), laryngeal cancer (20), and prostate cancer (21).
For the present PTTG1 is ascertained to be highly correlated with various aspects of PC, including gender, clinical stage, and prognosis. Long et al. found that higher expression of PTTG1 was associated with higher clinical stages and worse prognosis of PC, the potential mechanism of which was the enhanced OAd5 transduction into PC cells by increasing CXADR expression on the cell surface (22). In a study of human PC tissues, the scholars discovered that PTTG1 was highly expressed in PC, and its expression was related to the gender of PC patients (23). However, no significant correlation was found between PTTG1 expression and perineural infiltration as well as age, tumor sizes, pathological styles, and distant metastases in PC patients. Since PTTG1 plays an essential role in PC progression and in the present study, we analyzed the expression of PTTG1 messenger RNA (mRNA) and protein and their relation to progression in PC to demonstrate the role of PTTG1 in the development of PC and its value as a molecular target for cancer therapy. We present this article in accordance with the REMARK reporting checklist (available at https://jgo.amegroups.com/article/view/10.21037/jgo-23-979/rc).
Methods
Study design
Hence, a new mining method of high-throughput analysis based on sequence data such as microarrays, RNA-sequencing (RNA-seq), and all available published documents was presented to evaluate the potential of the PTTG1 gene in early diagnosis and prompt treatment. The mining of various databases, and bioinformatics analyses are required to verify the different expression levels, clinical value, and potential pathological role of PTTG1 in PC. Additionally, further validation of the protein expression level of PTTG1 was conducted using in-house immunohistochemistry (IHC). Then, Gene Ontology (GO) and Kyoto Encyclopedia of Genes and Genomes (KEGG) analysis and a protein-protein interaction (PPI) network were constructed and analyzed to harvest the enrichment functions, potential pathways, and hub genes. In addition, comprehensive meta-analyses of hazard ratio (HR) and Kaplan-Meier (KM) survival were performed to examine the potential prognostic value of PTTG1 expression in patients with PC. The study design is presented in Figure 1.
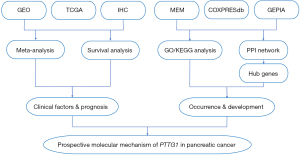
Data extraction of PTTG1 expression from Gene Expression Omnibus (GEO) and The Cancer Genome Atlas (TCGA) databases
Initially, PC-related RNA-seq data were searched in the GEO (https://www.ncbi.nlm.nih.gov/gds/) database using the search strategy ‘pancreas’ OR ‘pancreatic’, AND ‘carcinoma’, ‘adenocarcinoma’, ‘cancer’, ‘neoplasm’, ‘tumor’, ‘tumour’, ‘malignan*’, ‘neoplas*’, ‘PDAC’, ‘or’, ‘PAAD’ OR ‘PC’. ‘Homo sapiens’ was used to limit the search range. The criteria for the chip filter conditions were as follows: (I) human pancreatic tissue; (II) comparative RNA expression data chip with PC tissue and paracancer or normal pancreatic tissue; and (III) patients without adjuvant therapies, including radiotherapy and chemotherapy. The exclusion criteria were as follows: (I) samples based on cell line; (II) methylation; (III) samples that have been treated with adjuvant therapies; and (IV) cancer samples without a comparison of paracancer or normal tissue. The mRNA expression data associated with PC of TCGA was extracted from the University of California Santa Cruz (UCSC) Xena (https://xena.ucsc.edu/). Therefore, we obtained the mRNA profiles of 179 PC tissues and four non-cancerous tissues.
In-house IHC
The tissue microarray that included 79 cases of PC tissues and 73 adjacent non-cancerous tissues (HPanA170Su04) was purchased from Shanghai Outdo Biotech Company (Shanghai, China) and some clinical information for each sample, such as age, gender, clinical stage, surgery and OS were also provided. OS was defined as the time interval from surgery to cancer-related death or the last follow-up. In the IHC analysis, PTTG1 was detected with anti-securin antibody (at a 1/100 dilution; JB37-37; HUABIO, Woburn, MA, USA). The PTTG1 expression intensity for each sample was evaluated based on the score, and the score was calculated as the product of the proportion of stained cells among all cells (0, <5%; 1, 5–10%; 2, 11–50%; 3, 51–75%; 4, >75%) and the staining degree of the positive cells (0, no staining; 1, light yellow or yellow; 2, brown; 3, dark brown). Finally, a total expression score was given ranging from 0 to 12 (23). Based on the analysis results by X-tile software (Yale School of Medicine, New Haven, CT, USA), PTTG1 protein expression was regarded as negative expression in PC tissues when the score was 0–6, and as positive expression when the score was ≥6. The scoring of IHC staining was performed by two experienced pathologists without knowing the patient’s clinical information. The study was approved by the Ethics Committee of Shanghai Outdo Biotech Company (No. YB M-05-02) and carried out in accordance with the Declaration of Helsinki (as revised in 2013).
Acquisition of co-expressed genes of PTTG1 in PC
The Multi Experiment Matrix (MEM; https://biit.cs.ut.ee/mem/index.cgi) website is a web-based tool employed in multi experiment gene expression query. The COXPRESdb (https://coxpresdb.jp/) is a database providing co-expression relationships for 11 animal species such as human and mouse (24). Putative co-expressed genes of PTTG1 were determined based on the MEM and COXPRESdb. In MEM, a P value of less than 0.05 was considered statistically significant. In COXPRESdb, the upper limit number was set to output 2,000 genes. The Gene Expression Profiling Interactive Analysis database (GEPIA; http://gepia.cancer-pku.cn/) is a cancer data mining website, based on the RNA sequencing data expression of 9,736 tumors and 8,587 normal samples from TCGA and the Genotype-Tissue Expression (GTEx) projects. The differentially expressed genes (DEGs) of PC were further studied when the |log2(fold change)| ≥1.5 and adjusted P value <0.05 in the GEPIA database. Intersecting genes of the three dataset parts were treated as co-expressed genes of PTTG1 in PC (25).
Bioinformatics analysis of overlapping genes
The Database for Annotation, Visualization and Integrated Discovery tool (DAVID 6.8; https://david.ncifcrf.gov/) was used to perform GO functional annotation and KEGG biological pathway analyses by using the overlapped genes of PTTG1. Statistical significance was indicated when the pathways and enrichment significance level were set as a P value <0.05. GO is an ontology used extensively in this field of bioinformatics for annotating genes, gene products, and sequences. It covers three aspects of biology: biological process (BP), cellular component (CC), and molecular function (MF) (26). The top 10 significant function enrichment analyses included 10 BP, CC, and MF entries as well as 10 KEGG pathways, which were visualized using the ‘GOplot’ package of R software v.3.5.2 to reveal possible enrichment of candidate target genes. A PPI network was established and forecast by the Search Tool for the Retrieval of Interacting Genes (STRING) database (https://string-db.org/). Based on the degree of nodes, hub co-expressed genes of PTTG1 were identified. Hub genes encoded protein expression and IHC staining in PC and normal pancreatic tissues were searched in The Human Protein Atlas (HPA; https://www.proteinatlas.org/) database to present the expression pattern.
Statistical analysis
In the statistical analysis, continuous variable results of PTTG1 expression were presented as means ± standard deviation (SD). The measurement data of normal distribution were compared by t-test between the two groups and analysis of variance between multiple groups; The measurement data with non-normal distribution were compared by Mann-Whitey U test between the two groups and Kruskal-Walls H test between multiple groups. Additionally, receiver operating characteristic (ROC) curve analyses were constructed to compare the predictive performance of PTTG1 in the study groups. ROC curves were plotted with sensitivity vs. 1 − specificity and were then used to obtain the cut-off points for optimal sensitivity and specificity. The area under the curve (AUC) was performed to assess the overall discriminative capability of the variables. The best cut-off value was determined as the point with a maximum Youden index. The diagnostic value parameters of true positivity (TP), false positivity (FP), false negativity (FN), and true negativity (TN) cases were calculated from the ROC curve. Further, we summarized these analyses and drew a summary ROC (sROC) curve to investigate the accuracy of PTTG1 in discriminating between cancerous and normal tissue. The statistical analysis was carried out using SPSS 22.0 software (IBM Corp., Armonk, NY, USA). Additionally, we conducted this meta-analysis to explore the association between PTTG1 and the risk of PC. Stata 12.0 (StataCorp., College Station, TX, USA) was utilized to perform the forest plots with a standard mean difference (SMD) and 95% confidence interval (CI), and funnel plots based on TCGA and GEO data. A funnel plot was carried out to evaluate the publication bias. The heterogeneity of the meta-analysis was evaluated with Higgins I2. When the P≥0.05 or I2≤50%, it was deemed there was less or no heterogeneity, and the fixed effects model was used. A random effect model should be selected if P<0.05 and I2>50%. A value of P<0.05 (two-tailed) was considered statistically significant in all analyses. GraphPad Prism v.8.0 software (GraphPad Software, San Diego, CA, USA) was used to obtain violin plots and ROC curves with the RNA-seq and microarray data.
Evaluation of the prognostic value of PTTG1 in PC
Cox regression was employed to calculate the HR and 95% CIs for PC. The prognostic data downloaded from TCGA and GEO were analyzed using SPSS 22.0 to estimate HR and 95% CIs. An observed HR >1 indicated that patients with high PTTG1 expression were more likely to have an unfavorable prognosis. A comprehensive meta-analysis was conducted on the HR to evaluate the efficiency of PTTG1 in PC prognosis. The prognostic data from TCGA and GEO were analyzed with the Cox regression model to estimate the effect of PTTG1 on survival time. A funnel plot was performed to evaluate publication bias by using SelnHR as the abscissa and lnHR as the ordinate. We then conducted the sensitivity analyses using Stata 12.0. In addition, the KM plotter survival analysis tool (https://kmplot.com/) with detailed survival including gene chip and RNA-seq data from GEO, European Genome-phenome Archive (EGA), and TCGA databases, was used to validate the effect of PTTG1 in PC. In this research, the expressions and prognostic values of PTTG1 were carried out through GEPIA and KM online datasets.
Results
PTTG1 expression in PC from the GEO, TCGA, and IHC databases
A total of 29 GEO microarray datasets containing PTTG1 were included in the current study, namely, GSE14245, GSE11838, GSE15471, GSE16515, GSE19279, GSE22780, GSE28735, GSE32676, GSE32688, GSE36076, GSE41368, GSE43288, GSE46234, GSE49515, GSE55643, GSE56560, GSE58561, GSE60646, GSE60980, GSE62165, GSE62452, GSE63158, GSE71729, GSE71989, GSE74629, GSE91035, GSE101448, GSE101462, and GSE107610. The final analysis included 1,056 PC samples and 394 non-cancer samples. In the overall data analysis of 29 GEO microarrays, the results showed that 19 of the selected PTTG1 microarrays (GSE15471, GSE16515, GSE28735, GSE32676, GSE32688, GSE41368, GSE43288, GSE55643, GSE56560, GSE60646, GSE60980, GSE62165, GSE62452, GSE63158, GSE71729, GSE71989, GSE91035, GSE101462, and GSE107610) had remarkably increased expression in cancer tissues compared to normal tissues. The mRNA expression data of TCGA associated with PC were downloaded from the UCSC Xena database. We extracted the mRNA profiles of 179 PC tissues and four non-cancerous tissue expression data together with corresponding clinical parameters. For the IHC data, PTTG1 was found to be upregulated in 79 PC tissues (6.47±3.042) compared to 73 normal tissues (4.67±2.404, P<0.001). The expression of PTTG1 in the GEO microarrays and the TCGA and IHC databases is shown in Tables 1,2.
Table 1
Datasets | Platform | Year | Country | Patients | Normal | t value | P value | |||||
---|---|---|---|---|---|---|---|---|---|---|---|---|
Number | Mean | SD | Number | Mean | SD | |||||||
GSE14245 | GPL570 | 2008 | USA | 12 | 4.5883 | 4.23926 | 12 | 13.0092 | 13.35287 | 2.082 | 0.057 | |
GSE11838 | GPL6977 | 2002 | USA | 28 | 7.5244 | 9.60781 | 4 | 2.1813 | 0.41225 | −1.097 | 0.282 | |
GSE15471 | GPL570 | 2009 | Romania | 36 | 8.2642 | 0.85627 | 36 | 7.2728 | 1.04296 | −4.408 | <0.001 | |
GSE16515 | GPL570 | 2009 | USA | 36 | 296.8483 | 293.835 | 16 | 79.1056 | 71.5034 | −2.911 | 0.005 | |
GSE19279 | GPL96 | 2013 | United Kingdom | 4 | 6.178 | 0.54797 | 3 | 5.4217 | 0.13092 | −2.290 | 0.071 | |
GSE22780 | GPL570 | 2011 | USA | 8 | 754.8165 | 336.3105 | 8 | 624.235 | 489.5187 | −0.622 | 0.544 | |
GSE28735 | GPL6244 | 2012 | USA | 45 | 4.9654 | 0.5298 | 45 | 4.3872 | 0.53832 | −5.135 | <0.001 | |
GSE32676 | GPL570 | 2011 | USA | 25 | 8.0265 | 1.38649 | 7 | 6.253 | 2.08149 | −2.675 | 0.012 | |
GSE32688 | GPL570 | 2011 | USA | 25 | 8.0265 | 1.38649 | 7 | 6.253 | 2.08149 | −2.675 | 0.012 | |
GSE36076 | GPL570 | 2014 | Singapore | 3 | 8.1048 | 1.33284 | 10 | 8.1048 | 0.29178 | 0.000 | 1.000 | |
GSE41368 | GPL6244 | 2013 | Italy | 6 | 88.5152 | 31.78445 | 6 | 37.3604 | 7.93625 | −3.825 | 0.010 | |
GSE43288 | GPL96 | 2013 | United Kingdom | 4 | 6.5067 | 0.43875 | 3 | 5.7663 | 0.1229 | −2.781 | 0.039 | |
GSE46234 | GPL570 | 2017 | Norway | 4 | 2,544.08 | 2,180.275 | 4 | 687.6562 | 719.012 | −1.617 | 0.157 | |
GSE49515 | GPL570 | 2013 | Singapore | 3 | 8.1048 | 1.33284 | 10 | 8.1048 | 0.29178 | 0.000 | 1.000 | |
GSE55643 | GPL6480 | 2014 | United Kingdom | 45 | 12.181 | 1.2603 | 8 | 10.9756 | 1.70234 | −2.363 | 0.022 | |
GSE56560 | GPL5175 | 2014 | United Kingdom | 28 | 128.3821 | 53.96653 | 6 | 59.7369 | 13.82657 | −3.060 | 0.004 | |
GSE58561 | GPL14550 | 2014 | Norway | 3 | 12.3301 | 0.73458 | 2 | 12.2394 | 0.30142 | −0.159 | 0.884 | |
GSE60646 | GPL5175 | 2015 | USA | 10 | 5.821 | 1.28669 | 10 | 4.426 | 0.95763 | −2.750 | 0.013 | |
GSE60980 | GPL14550 | 2015 | Norway | 49 | 10.7902 | 0.92524 | 12 | 9.0403 | 1.5351 | −3.784 | 0.002 | |
GSE62165 | GPL13667 | 2016 | Belgium | 118 | 7.6285 | 1.01979 | 13 | 5.1483 | 1.17441 | −8.199 | <0.001 | |
GSE62452 | GPL6244 | 2016 | USA | 69 | 4.8242 | 0.51968 | 61 | 4.3376 | 0.4681 | −5.616 | <0.001 | |
GSE63158 | GPL5175 | 2014 | United Kingdom | 28 | 128.3821 | 53.96653 | 6 | 59.7369 | 13.82657 | −3.060 | 0.004 | |
GSE71729 | GPL20769 | 2015 | USA | 145 | 7.1439 | 0.7425 | 46 | 6.4856 | 0.56642 | −5.521 | <0.001 | |
GSE71989 | GPL570 | 2015 | USA | 13 | 9.4416 | 0.8487 | 8 | 8.0801 | 0.2303 | −5.466 | <0.001 | |
GSE74629 | GPL10558 | 2015 | Spain | 36 | 7.0844 | 0.08333 | 14 | 7.0775 | 0.06365 | −0.278 | 0.782 | |
GSE91035 | GPL22763 | 2016 | USA | 25 | 10.2488 | 1.12223 | 8 | 7.5965 | 0.39864 | −10.007 | <0.001 | |
GSE101448 | GPL10558 | 2018 | Germany | 24 | 7.9148 | 0.34089 | 19 | 8.1349 | 0.5504 | 1.610 | 0.115 | |
GSE101462 | GPL10558 | 2018 | Germany | 6 | 6.5341 | 0.15836 | 4 | 6.2645 | 0.04729 | −3.250 | 0.012 | |
GSE107610 | GPL15207 | 2018 | Japan | 39 | 10.56 | 0.65089 | 2 | 9.3773 | 1.16649 | −2.438 | 0.019 | |
TCGA | 179 | 8.0081 | 1.01655 | 4 | 7.083 | 0.7361 | −1.807 | 0.072 | ||||
IHC | 79 | 6.47 | 3.042 | 73 | 4.67 | 2.404 | 4.056 | <0.001 |
PTTG1, pituitary tumor transforming gene 1; IHC, immunohistochemistry; GEO, Gene Expression Omnibus; TCGA, The Cancer Genome Atlas; SD, standard deviation.
Table 2
Datasets | Sensitivity (%) | Specificity (%) | TP | FP | FN | TN |
---|---|---|---|---|---|---|
GSE14245 | 100.00 | 25.00 | 12 | 9 | 0 | 3 |
GSE11838 | 75.00 | 100.00 | 21 | 0 | 7 | 4 |
GSE15471 | 100.00 | 50.00 | 36 | 18 | 0 | 18 |
GSE16515 | 88.90 | 81.20 | 32 | 3 | 4 | 13 |
GSE19279 | 75.00 | 100.00 | 3 | 0 | 1 | 3 |
GSE22780 | 75.00 | 75.00 | 6 | 2 | 2 | 6 |
GSE28735 | 88.90 | 62.20 | 40 | 17 | 5 | 28 |
GSE32676 | 92.00 | 71.40 | 23 | 2 | 2 | 5 |
GSE32688 | 92.00 | 71.40 | 23 | 2 | 2 | 5 |
GSE36076 | 33.30 | 100.00 | 1 | 0 | 2 | 10 |
GSE41368 | 100.00 | 100.00 | 6 | 0 | 0 | 6 |
GSE43288 | 100.00 | 100.00 | 4 | 0 | 0 | 3 |
GSE46234 | 75.00 | 100.00 | 3 | 0 | 1 | 4 |
GSE49515 | 33.30 | 100.00 | 1 | 0 | 2 | 10 |
GSE55643 | 84.40 | 75.00 | 38 | 2 | 7 | 6 |
GSE56560 | 75.00 | 100.00 | 21 | 0 | 7 | 6 |
GSE58561 | 33.30 | 100.00 | 1 | 0 | 2 | 2 |
GSE60646 | 90.00 | 90.00 | 9 | 1 | 1 | 9 |
GSE60980 | 98.00 | 75.00 | 48 | 3 | 1 | 9 |
GSE62165 | 98.30 | 84.60 | 116 | 2 | 2 | 11 |
GSE62452 | 60.90 | 86.90 | 42 | 8 | 27 | 53 |
GSE63158 | 85.70 | 100.00 | 24 | 0 | 4 | 6 |
GSE71729 | 41.40 | 97.80 | 60 | 1 | 85 | 45 |
GSE71989 | 84.60 | 100.00 | 11 | 0 | 2 | 8 |
GSE74629 | 38.90 | 78.60 | 14 | 3 | 22 | 11 |
GSE91035 | 96.00 | 100.00 | 24 | 0 | 1 | 8 |
GSE101448 | 100.00 | 10.50 | 24 | 17 | 0 | 2 |
GSE101462 | 83.30 | 100.00 | 5 | 0 | 1 | 4 |
GSE107610 | 71.80 | 100.00 | 28 | 0 | 11 | 2 |
TCGA | 82.70 | 75.00 | 148 | 1 | 31 | 3 |
IHC | 92.41 | 44.58 | 73 | 40 | 6 | 33 |
PTTG1, pituitary tumor transforming gene 1; PC, pancreatic cancer; TP, true positive; FP, false positive; FN, false negative; TN, true negative; TCGA, The Cancer Genome Atlas; IHC, immunohistochemistry.
Based on the obtained RNA-seq and microarray data, GraphPad Prism v.8.0 was used for statistical analysis and violin plots. The software was applied to compare the expression levels of PTTG1 in PC and non-cancerous tissues (P<0.05, Figures 2,3). ROC curves were also generated (Figures 4-6). In the overall data analysis of 29 GEO chips, the results revealed that 19 chips had significant upregulation in PC tissues, compared with normal tissues. The box chart showed the mRNA expression of the PTTG1 gene in the PC tissues (179 cases) and non-PC tissues (171 cases) in GEPIA. The results indicated that the expression level of PTTG1 in PC tissues was significantly higher than that in non-PC tissues (P<0.05, Figure 7A). GEPIA analysis of high and low expression levels of PTTG1 in PC on the pathological stage of patients is shown in Figure 7B. PTTG1 expression levels did not significantly differ between the different pathological stages (P=0.07).
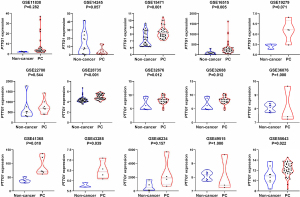
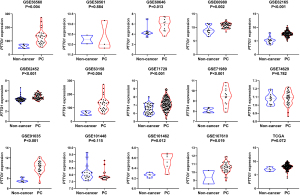
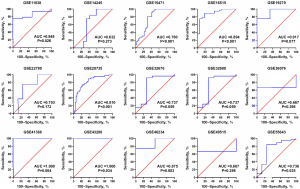
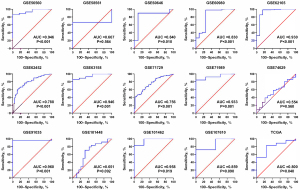
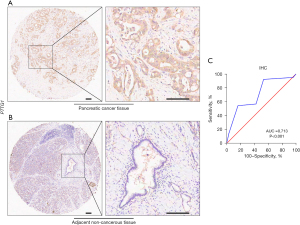
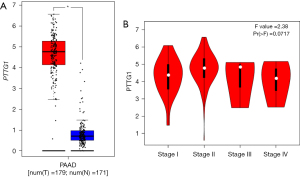
Correlation between PTTG1 expression and clinicopathological parameters
The Cox regression analysis was applied to analyze the prognostic factors. According to the IHC data, the univariate analysis indicated that there was no significant relationship between PTTG1 expression and OS (P=0.317), as shown in Table 3. Other clinical parameters including gender (HR: 0.48, 95% CI: 0.30–0.76, P=0.002), size (HR: 1.91, 95% CI: 1.20–3.02, P=0.006), T stage (HR: 2.13, 95% CI: 1.33–3.40, P=0.002), N stage (HR: 1.64, 95% CI: 1.14–2.34, P=0.007), and M stage (HR: 5.61, 95% CI: 3.22–9.76, P<0.001) were also associated with poorer OS. Multivariate analysis showed that gender (HR: 0.36, 95% CI: 0.21–0.60, P<0.001) and M staging (HR: 4.71, 95% CI: 2.47–9.01, P<0.001) were independently associated with OS. In addition, the association between PTTG1 and some clinical parameters was also calculated using TCGA data, and the results indicated that the expression of PTTG1 was associated with the histological grade (Table 4, F=4.020, P=0.009). As the grade of PC increased, PTTG1 expression also increased.
Table 3
Characteristics | Univariate Cox regression | Multivariate Cox regression | |||
---|---|---|---|---|---|
HR (95% CI) | P value | HR (95% CI) | P value | ||
Gender (male vs. female) | 0.48 (0.30–0.76) | 0.002 | 0.36 (0.21–0.60) | <0.001 | |
Age (≥60 vs. <60 years) | 0.87 (0.56–1.37) | 0.554 | |||
CEA (≥5 vs. <5 ng/mL) | 1.59 (0.97–2.60) | 0.064 | 1.34 (0.77–2.33) | 0.301 | |
CA199 (≥37 vs. <37 U/mL) | 0.97 (0.56–1.70) | 0.926 | |||
Size (≥4 vs. <4 cm) | 1.91 (1.20–3.02) | 0.006 | 1.55 (0.63–3.77) | 0.337 | |
T stage (T3&T4 vs. T1&T2) | 2.13 (1.33–3.40) | 0.002 | 1.29 (0.56–2.99) | 0.551 | |
N stage (N2 vs. N1 vs. N0) | 1.64 (1.14–2.34) | 0.007 | 0.87 (0.54–1.41) | 0.576 | |
M stage (M1 vs. M0) | 5.61 (3.22–9.76) | <0.001 | 4.71 (2.47–9.01) | <0.001 | |
Venous invasion (present vs. absent) | 1.37 (0.87–2.15) | 0.176 | |||
Nervous invasion (present vs. absent) | 1.25 (0.78–2.01) | 0.357 | |||
PTTG1 (high vs. low) | 0.79 (0.50–1.25) | 0.317 |
IHC, immunohistochemistry; HR, hazard ratio; CI, confidence interval; CEA, carcinoembryonic antigen; CA199, carbohydrate antigen 199; T, tumor; N, node; M, metastasis; PTTG1, pituitary tumor transforming gene 1.
Table 4
Clinicopathological parameters | Number | PTTG1 expression† | H/Z/F value | P value |
---|---|---|---|---|
Age | −0.072 | 0.943 | ||
<60 years | 55 | 8.096 (7.419–8.552) | ||
≥60 years | 124 | 8.045 (7.475–8.616) | ||
Gender | −0.036 | 0.971 | ||
Female | 80 | 8.040 (7.479–8.616) | ||
Male | 99 | 8.120 (7.453–8.585) | ||
Tumor size | −1.503 | 0.133 | ||
<4 cm | 102 | 8.008 (7.435–8.466) | ||
≥4 cm | 77 | 8.169 (7.510–8.870) | ||
Histological grade | 4.020 | 0.009 | ||
G1 | 32 | 7.484±1.343 | ||
G2 | 96 | 8.082±0.877 | ||
G3 | 48 | 8.169±0.937 | ||
G4 | 3 | 8.672±0.968 | ||
T stage | 4.054 | 0.256 | ||
T1 | 7 | 7.873 (6.710–8.310) | ||
T2 | 24 | 7.816 (7.095–8.631) | ||
T3 | 143 | 8.096 (7.508–8.617) | ||
T4 | 5 | 8.348 (6.288–9.229) | ||
N stage | 1.837 | 0.399 | ||
N0 | 51 | 8.070 (7.249–8.794) | ||
N1–N1b | 124 | 8.063 (7.482–8.477) | ||
NX | 4 | 7.220 (6.150–8.509) | ||
M stage | 3.282 | 0.194 | ||
M0 | 80 | 8.091 (7.461–8.670) | ||
M1 | 5 | 7.503 (6.249–7.942) | ||
MX | 94 | 8.047 (7.474–8.625) | ||
Tumor stage | 6.891 | 0.075 | ||
I–IB | 21 | 7.794 (6.828–8.401) | ||
IIA–IIB | 147 | 8.096 (7.508–8.666) | ||
III | 6 | 8.571 (6.413–9.191) | ||
IV | 5 | 7.503 (6.249–7.942) |
†, data are presented as HR (95% CI) or mean ± SD. PTTG1, pituitary tumor transforming gene 1; TCGA, The Cancer Genome Atlas; G, grade; T, tumor; N, node; M, metastasis; HR, hazard ratio; CI, confidence interval; SD, standard deviation.
Meta-analysis of GEO and TCGA
We used Stata 12.0 for the meta-analysis of continuous variables to conduct a holistic evaluation of PTTG1 expression levels. A total of 1,056 PC cases and 394 non-cancerous tissue cases were collected in our study from 29 GEO microarrays and TCGA sequencing data. PTTG1 in PC tissues was significantly higher than that in non-cancerous controls (SMD: 1.02, 95% CI: 0.74–1.30, Figure 8A). Due to the high heterogeneity in this meta-analysis (I2=71.4%, P<0.001), a random effects model was used for the analysis. As shown by the sensitivity analysis in Figure 8B, there was no significant heterogeneity in the study. At the same time, funnel plots (Figure 8C) did not find publication bias. The results of the diagnostic analysis of GEO microarrays and TCGA sequencing data showed that the pooled specificity and sensitivity of PTTG1 for the diagnosis of PC were 0.86 (95% CI: 0.77–0.92) and 0.87 (95% CI: 0.77–0.93), respectively, sROC (AUC) =0.93 (95% CI: 0.90–0.95) (Figure 8D).
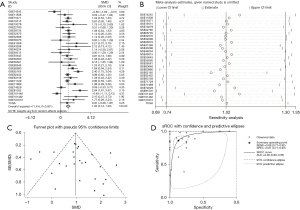
The prognostic value of PTTG1 in PC
Concerning the prognostic value, an elevated PTTG1 level was remarkably correlated with worse OS (HR: 1.36, 95% CI: 1.07–1.75, Figure 9A) and with unobserved heterogeneity (I2=0.0%, P=0.852) when employing the fixed-effect model. Furthermore, a sensitivity analysis was conducted, and the result indicated the pooled HR was stable (Figure 9B). As shown in Figure 9C, Begg’s regression plot revealed no statistically significant publication bias in the eligible studies. Additionally, GEPIA showed a correlation between survival and PTTG1 gene expression levels in PC patients. The OS (HR: 2.0, P<0.001, Figure 10A) and disease-free survival (DFS; HR: 1.7, P=0.013, Figure 10B) of PC patients with high expression of PTTG1 (n=89) was significantly lower than that of patients with low expression of PTTG1 (n=89). However, KM survival curves showed that there was no significance between PTTG1 mRNA expression with the OS of PC patients (HR: 1.254, P=0.3104, Figure 10C) based on the IHC data.
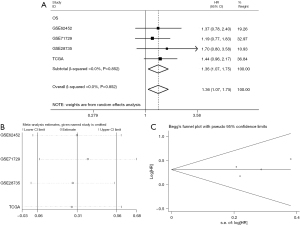
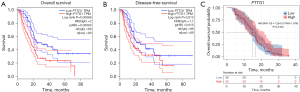
Genes co-expressed with PTTG1
We collected 1,574 related genes from the MEM database and identified 2,000 genes from COXPRESdb. From GEPIA, the 5,076 DEGs between PC and non-cancer tissues were selected. We eventually identified 367 overlapping genes (Figure 11A).
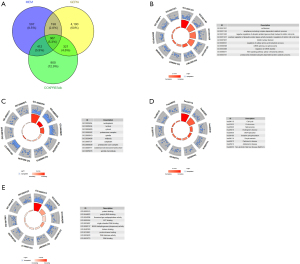
Bioinformatics analysis
To explore the mechanisms and pathways of PTTG1, the 367 overlapping genes were analyzed by using the DAVID and STRING online tools. According to the GO enrichment analysis, in terms of BP, the top 3 most significant processes were cell division, anaphase-promoting complex-dependent catabolic process, and negative regulation of ubiquitin-protein ligase activity involved in the mitotic cell cycle. As for the analysis of CC, the topmost significant annotations were nucleoplasm, nucleus, and cytosol. In the annotations of MF, the top 3 most significant functions were protein binding, poly(A) RNA binding, and threonine-type endopeptidase activity (Table 2, Figure 11B-11D). The results of the KEGG pathway analysis indicated that DEGs were centralized in these pathways, such as cell cycle, proteasome, and spliceosome (Table 5, Figure 11E). We placed the top 367 overlapping genes into STRING and generated the PPI network (Figure 12). The PPI network analysis of PTTG1 targets indicated that four hub genes (CDK1, CCNA2, CDC20, and MAD2L1) were found at the highest level.
Table 5
Geneset | Description | Count | P value |
---|---|---|---|
BP | |||
GO:0051301 | Cell division | 55 | 4.61E−31 |
GO:0031145 | Anaphase-promoting complex-dependent catabolic process | 30 | 1.24E−28 |
GO:0051436 | Negative regulation of ubiquitin-protein ligase activity involved in mitotic cell cycle | 28 | 3.18E−27 |
GO:0051437 | Positive regulation of ubiquitin-protein ligase activity involved in regulation of mitotic cell cycle transition | 28 | 2.79E−26 |
GO:0007067 | Mitotic nuclear division | 37 | 4.93E−20 |
GO:0006521 | Regulation of cellular amino acid metabolic process | 19 | 7.31E−18 |
GO:0000398 | mRNA splicing, via spliceosome | 32 | 6.50E−17 |
GO:0043488 | Regulation of mRNA stability | 23 | 2.49E−16 |
GO:0060071 | Wnt signaling pathway, planar cell polarity pathway | 22 | 2.85E−16 |
GO:0043161 | Proteasome-mediated ubiquitin-dependent protein catabolic process | 30 | 3.63E−16 |
CC | |||
GO:0005654 | Nucleoplasm | 184 | 2.29E−57 |
GO:0005634 | Nucleus | 211 | 3.60E−30 |
GO:0005829 | Cytosol | 159 | 3.74E−30 |
GO:0000502 | Proteasome complex | 20 | 2.43E−18 |
GO:0005819 | Spindle | 23 | 2.24E−15 |
GO:0030496 | Midbody | 22 | 9.55E−14 |
GO:0005737 | Cytoplasm | 168 | 4.57E−13 |
GO:0005839 | Proteasome core complex | 11 | 2.32E−12 |
GO:0000777 | Condensed chromosome kinetochore | 17 | 1.28E−11 |
GO:0005876 | Spindle microtubule | 13 | 3.52E−11 |
MF | |||
GO:0005515 | Protein binding | 277 | 1.45E−24 |
GO:0044822 | Poly(A) RNA binding | 67 | 1.90E−14 |
GO:0004298 | Threonine-type endopeptidase activity | 11 | 4.16E−12 |
GO:0005524 | ATP binding | 68 | 1.77E−09 |
GO:0003697 | Single-stranded DNA binding | 13 | 5.45E−07 |
GO:0008137 | NADH dehydrogenase (ubiquinone) activity | 10 | 5.78E−07 |
GO:0042393 | Histone binding | 14 | 1.71E−06 |
GO:0019901 | Protein kinase binding | 24 | 4.84E−06 |
GO:0003678 | DNA helicase activity | 7 | 7.97E−06 |
GO:0003723 | RNA binding | 28 | 3.68E−05 |
KEGG | |||
hsa04110 | Cell cycle | 32 | 1.69E−20 |
hsa03050 | Proteasome | 20 | 5.45E−18 |
hsa03040 | Spliceosome | 21 | 2.86E−09 |
hsa05016 | Huntington’s disease | 24 | 1.62E−08 |
hsa03030 | DNA replication | 11 | 7.54E−08 |
hsa00190 | Oxidative phosphorylation | 18 | 5.25E−07 |
hsa04114 | Oocyte meiosis | 16 | 1.22E−06 |
hsa05012 | Parkinson’s disease | 18 | 1.34E−06 |
hsa05010 | Alzheimer’s disease | 19 | 3.31E−06 |
hsa04932 | NAFLD | 17 | 1.37E−05 |
GO, Gene Ontology; KEGG, Kyoto Encyclopedia of Genes and Genomes; PTTG1, pituitary tumor transforming gene 1; BP, biological process; mRNA, messenger RNA; CC, cellular component; MF, molecular function; ATP, adenosine triphosphate; NADH, Nicotinamide adenine dinucleotide; hsa, homo sapiens; NAFLD, non-alcoholic fatty liver disease.
Protein levels of the hub genes from the HPA database
We put the four hub genes into the HPA database to understand the protein expression pattern of these genes: CDK1 (antibody HPA003387), CDC20 (antibody CAB004525), CCNA2 (antibody CAB000114), and MAD2L1 (antibody HPA003348). CDK1 exhibited low staining and weak intensity in normal pancreatic tissues and showed medium staining and moderate intensity in PC tissues. CCNA2 displayed undetected staining and negative intensity in normal pancreatic tissues, whereas it displayed medium staining and strong intensity in PC tissues. CDC20 showed undetected staining and negative intensity in normal pancreatic tissues, and high staining and strong intensity in PC tissues. MAD2L1 presented low staining and weak intensity in normal pancreatic tissues while recording low staining and moderate intensity in PC tissues.
Discussion
PC is the 4th most common cause of cancer death and is the 12th most common cause of cancer (27). To make things worse, diagnosis of advanced stage, recurrence, and metastasis shatter the treatment window of opportunity for PC patients (28). The tumor microenvironment (TME) in PC is known to influence tumor progression and can be influenced by different tumor characteristics, leading to diverse mechanisms of immune evasion (29). Current therapeutic strategies targeting the TME of PC have been designed to modulate the cancer-associated fibroblast and immune compartments. These strategies include the use of CD40 agonistic monoclonal antibodies, chemotherapy, and immune checkpoint inhibitors (30). As an essential factor influencing TME, PTTG1 has been associated with tumorigenesis and poor prognosis in a variety of endocrine-related tumors including breast cancer (16) and thyroid cancer (17), as well as nonendocrine-related cancers involving the central nervous, pulmonary, and gastrointestinal systems such as hepatocellular carcinoma (13), multiple myeloma (15), and glioma (18). Though the overexpression of PTTG1 is controversial in promoting or inhibiting cell proliferation in various kinds of tumors, PTTG1 is confirmed to be tumorigenic via cell transforming by the induction of chromosomal instability and aneuploidy (31). Moreover, overexpressed PTTG1 may act as a paracrine/autocrine activator, enhancing expression of growth factors that in turn further sustain tumor growth and contribute to the tumorigenic microenvironment. Interestingly, PTTG1 is highly expressed in PC, and the positive expression of PTTG1 is related to the gender of PC patients (23). The discovery of molecular markers can improve diagnosis, evaluation of prognosis, and individualized treatment, including molecularly targeted therapies. To the best of our knowledge, few studies to date have investigated the relationship between PTTG1 regulation and PC development. On the molecular level, the precise mechanism has not yet been fully clarified, and intensive scientific research is currently underway to identify potential therapeutic targets for further therapies. Thus, our study sought to validate how PTTG1 was expressed in PC and explored the potential molecular mechanism and clinical pathological features. In the current study, we observed that the expression of PTTG1 in PC was dramatically up-regulated compared with non-tumor tissues. We first assessed PTTG1 expression in PC from GEO and TCGA databases, and it was observed that PTTG1 expression was significantly upregulated in PC tissues compared to non-cancerous tissues. Then, we validated this finding by IHC. We also performed a meta-analysis to exhaustively evaluate the prognostic role of PTTG1 expression in patients with PC and the results further verified that PTTG1 is an independent prognostic marker. To verify overlapping genes from the perspective of the underlying biological mechanism, we used the DAVID database to perform the GO functional analysis and KEGG pathway analyses. Functional analysis of the overlapping genes revealed a significant enrichment in BP that included cell division, anaphase-promoting complex-dependent catabolic process, and negative regulation of ubiquitin-protein ligase activity involved in mitotic cell cycle and in the MF that included protein binding, poly(A) RNA binding, and threonine-type endopeptidase activity. In the KEGG pathway network, we found that PTTG1 was involved in regulating multiple cancer-related pathways, including cell cycle, DNA replication, and spliceosome. The results suggested that the cell cycle might be actively involved in oncogenesis and progression. Deregulation of the cell cycle induces uncontrolled cell growth and loss of cell cycle checkpoint control accelerates genetic instability, which are key characteristics of cancer (32). Uncontrolled cell division is the primary key in the progression of tumors and it has previously been shown that IRAK1 over-expression promotes endometrial carcinoma tumorigenesis by activating the mitotic cell cycle and cell division pathways (33). It has also been reported that PTTG1 is highly involved in many other cellular processes, such as regulation of the cell cycle, growth, DNA repair, and even cell senescence (34).
Xu et al. revealed that dysregulation of cell cycle control is a hallmark of melanoma tumorigenesis (35). Oi et al. reported that LTA4H is an important regulator of the cell cycle at the G0/G1 phase in skin cancer acting by negatively regulating p27 expression (36). Cao et al. investigated the promotion effect of DDX21 on malignant growth accompanied by an effect on the cell cycle and found that DDX21 stimulated the growth of gastric cancer cells by promoting G1/S conversion (37). Zhu et al. showed that SNAP23 inhibited the proliferation and progression of cervical cancer and induced cell cycle G2/M arrest by upregulating p21cip1 and downregulating CyclinB1 (38). A recent study demonstrated that long non-coding RNA EPIC1 promotes the growth of PC cells by regulating the cell cycle via interacting with YAP1 (39). Similarly, our enrichment analysis showed that PTTG1 and its co‑expressed genes were involved in the cell cycle pathway and played an integral role in PC. This prompted the hypothesis that abnormal PTTG1 expression may play a distinct role in the occurrence, progression, and prognosis of PC. Taken together, cell cycle regulation is closely associated with tumorigenicity; this perspective could be exploited to develop new treatment strategies. The findings of the current research can provide a new orientation for deepening our understanding of PC. According to our research, a total of four hub genes (CDK1, CCNA2, CDC20, and MAD2L1) were identified, as determined from co-expression and PPI network analysis.
CDK1 (also known as CDC2), is a protein-coding gene and is crucial for a transition during the G1/S and G2/M phase of the eukaryotic cell cycle (40). In addition, entering into mitosis is primarily governed by the activity of CDK1 (41). Dysregulation of the cell cycle is an uncontrolled proliferative signal that acts as a marker for cancer (42). Meanwhile, CDK1 has been reported to phosphorylate YAP at multiple sites such as sites T119 and S289 at the G2/M phase of the cell cycle, promoting invasion and migration in cancer cells (43). Moreover, CDK1 expression has also been described in pancreatic ductal adenocarcinoma (44), epithelial ovarian cancer (45), hepatocellular carcinoma (46), and melanoma (47).
The protein coding gene CCNA2 resides on chromosome four which promotes G1/S and G2/M phase transition by modulating the cell cycle (48). Specifically, one previous study has found that the accumulation of CCNA2 in the S phase is up-regulated in the S, G2, and early M phases (49). Similarly, CCNA2, belonging to the highly conserved cyclin family, is identified as a regulator of CDK kinases (50). Deficiency and overabundance of CCNA2 have been observed in human cancers where it may affect cell proliferation, tumorigenesis (51), and poor clinical prognosis (52). Mounting evidence has revealed that the expression of CCNA2 is abnormally increased in a variety of cancers, including esophageal squamous cell carcinoma (53), colon cancer (54), hepatocellular carcinoma (55), PC (56), osteosarcoma (57), and cervical carcinoma (58).
CDC20, located in chromosome 1 (59), plays a critical role in cell cycle progression as an essential activator of the anaphase-promoting complex/cyclosome (APC/C) that controls the protein overexpression levels of vital regulators of mitosis and DNA replication (60,61). In addition to its main functions in mitosis, recent research had linked CDC20-APC/C to various cellular processes beyond the cell cycle such as stem cell expansion, neurogenesis, apoptosis, and epigenetic regulation (62). Up-regulated CDC20 along with clinicopathological parameters has been detected and verified in several cancers. We suspect that inhibiting CDC20 will play an important role in anticancer treatment (63). In addition, there is evidence that CDC20 is a carcinogenesis factor associated with cell growth, motility, apoptosis, and metastasis (64). A study has shown that increased expression of CDC20 eliminated the cytotoxic functions induced by curcumin and enhanced cellular proliferation and invasion in PC cells (65).
MAD2L1, a member of the spindle assembly mitotic checkpoint, is gene that assures the proper segregation of the sister chromatids at the metaphase plate during cell division to maintain genomic stability (66). Dysregulation of MAD2L1 has been shown to result in chromosomal instability and aneuploidy, which ultimately culminate in tumor development (67). MAD2L1 as a potential target for the treatment of several tumors plays a critical role in breast cancer, lung cancer, liver cancer, and gastric cancer (68). Li et al. demonstrated that a significant up-regulation of MAD2L1 in lung adenocarcinoma tissues could promote cell proliferation, migration, and invasion and its high expression is correlated with poor OS in lung adenocarcinoma patients (69). Lu et al. reported that MAD2L1 was associated with poor OS and event-free survival in rhabdomyosarcoma (70). Also, Wang et al. found that MAD2L1 was up-regulated in gastric cancer, and the miR-30a-3p can down-regulate the MAD2L1 expression to suppress the proliferation of gastric cancer cells and regulate the cell cycle (71).
In summary, PTTG1 may serve as a novel prognostic biomarker and a therapeutic target for patients with PC. However, there were still some limitations in this study that should be acknowledged. Although expression of PTTG1 was observed to be significantly upregulated in PC tissue after validation in GEO, TCGA, and IHC databases, the association of PTTG1 and its prognosis showed variation between public databases and real-world data. In the future, more laboratory experiments and clinical trials need to be performed to further validate the findings in this study. To substantiate PTTG1 as an independent prognostic factor, comprehensive research is needed. With multivariate analysis, we will conduct prospective trials or retrospective studies to accurately assess the prognostic value of PTTG1 independent of other confounding factors. Mendelian randomization analysis can also be applied for proving the causal relationship between PTTG1 and poor prognosis of PC.
Conclusions
PTTG1 may serve as a driving gene associated with the occurrence and progression of PC.
Acknowledgments
Funding: This work was supported by
Footnote
Reporting Checklist: The authors have completed the REMARK reporting checklist. Available at https://jgo.amegroups.com/article/view/10.21037/jgo-23-979/rc
Data Sharing Statement: Available at https://jgo.amegroups.com/article/view/10.21037/jgo-23-979/dss
Peer Review File: Available at https://jgo.amegroups.com/article/view/10.21037/jgo-23-979/prf
Conflicts of Interest: All authors have completed the ICMJE uniform disclosure form (available at https://jgo.amegroups.com/article/view/10.21037/jgo-23-979/coif). The authors have no conflicts of interest to declare.
Ethical Statement: The authors are accountable for all aspects of the work in ensuring that questions related to the accuracy or integrity of any part of the work are appropriately investigated and resolved. This study was performed in line with the principles of the Declaration of Helsinki (as revised in 2013). Approval was granted by the Ethics Committee of Shanghai Outdo Biotech Company (No. YB M-05-02).
Open Access Statement: This is an Open Access article distributed in accordance with the Creative Commons Attribution-NonCommercial-NoDerivs 4.0 International License (CC BY-NC-ND 4.0), which permits the non-commercial replication and distribution of the article with the strict proviso that no changes or edits are made and the original work is properly cited (including links to both the formal publication through the relevant DOI and the license). See: https://creativecommons.org/licenses/by-nc-nd/4.0/.
References
- Lambert A, Schwarz L, Borbath I, et al. An update on treatment options for pancreatic adenocarcinoma. Ther Adv Med Oncol 2019;11:1758835919875568. [Crossref] [PubMed]
- Bray F, Ferlay J, Soerjomataram I, et al. Global cancer statistics 2018: GLOBOCAN estimates of incidence and mortality worldwide for 36 cancers in 185 countries. CA Cancer J Clin 2018;68:394-424. [Crossref] [PubMed]
- Siegel RL, Miller KD, Wagle NS, et al. Cancer statistics, 2023. CA Cancer J Clin 2023;73:17-48. [Crossref] [PubMed]
- Lin QJ, Yang F, Jin C, et al. Current status and progress of pancreatic cancer in China. World J Gastroenterol 2015;21:7988-8003. [Crossref] [PubMed]
- Brunner M, Wu Z, Krautz C, et al. Current Clinical Strategies of Pancreatic Cancer Treatment and Open Molecular Questions. Int J Mol Sci 2019;20:4543. [Crossref] [PubMed]
- Deng PC, Chen WB, Cai HH, et al. LncRNA SNHG14 potentiates pancreatic cancer progression via modulation of annexin A2 expression by acting as a competing endogenous RNA for miR-613. J Cell Mol Med 2019;23:7222-32. [Crossref] [PubMed]
- Zhou Q, Andersson R, Hu D, et al. Quantitative proteomics identifies brain acid soluble protein 1 (BASP1) as a prognostic biomarker candidate in pancreatic cancer tissue. EBioMedicine 2019;43:282-94. [Crossref] [PubMed]
- Du J, Gu J, Deng J, et al. The expression and survival significance of sodium glucose transporters in pancreatic cancer. BMC Cancer 2022;22:116. [Crossref] [PubMed]
- Xiang W, Wu X, Huang C, et al. PTTG1 regulated by miR-146a-3p promotes bladder cancer migration, invasion, metastasis and growth. Oncotarget 2017;8:664-78. [Crossref] [PubMed]
- Chi HC, Chen CY, Tsai MM, et al. Molecular functions of thyroid hormones and their clinical significance in liver-related diseases. Biomed Res Int 2013;2013:601361. [Crossref] [PubMed]
- Li WH, Chang L, Xia YX, et al. Knockdown of PTTG1 inhibits the growth and invasion of lung adenocarcinoma cells through regulation of TGFB1/SMAD3 signaling. Int J Immunopathol Pharmacol 2015;28:45-52. [Crossref] [PubMed]
- Caporali S, Alvino E, Lacal PM, et al. Targeting the PTTG1 oncogene impairs proliferation and invasiveness of melanoma cells sensitive or with acquired resistance to the BRAF inhibitor dabrafenib. Oncotarget 2017;8:113472-93. [Crossref] [PubMed]
- Lin X, Yang Y, Guo Y, et al. PTTG1 is involved in TNF-α-related hepatocellular carcinoma via the induction of c-myc. Cancer Med 2019;8:5702-15. [Crossref] [PubMed]
- Jiang T, Si L. Identification of the molecular mechanisms associated with acute type A aortic dissection through bioinformatics methods. Braz J Med Biol Res 2019;52:e8950. [Crossref] [PubMed]
- Chiriva-Internati M, Ferrari R, Prabhakar M, et al. The pituitary tumor transforming gene 1 (PTTG-1): an immunological target for multiple myeloma. J Transl Med 2008;6:15. [Crossref] [PubMed]
- Meng C, Zou Y, Hong W, et al. Estrogen-regulated PTTG1 promotes breast cancer progression by regulating cyclin kinase expression. Mol Med 2020;26:33. [Crossref] [PubMed]
- Chintharlapalli S, Papineni S, Lee SO, et al. Inhibition of pituitary tumor-transforming gene-1 in thyroid cancer cells by drugs that decrease specificity proteins. Mol Carcinog 2011;50:655-67. [Crossref] [PubMed]
- Genkai N, Homma J, Sano M, et al. Increased expression of pituitary tumor-transforming gene (PTTG)-1 is correlated with poor prognosis in glioma patients. Oncol Rep 2006;15:1569-74. [Crossref] [PubMed]
- Rehfeld N, Geddert H, Atamna A, et al. The influence of the pituitary tumor transforming gene-1 (PTTG-1) on survival of patients with small cell lung cancer and non-small cell lung cancer. J Carcinog 2006;5:4. [Crossref] [PubMed]
- Zhang Z, Wang X, Tan X. Correlation of serum PTTG1 expression level with clinicopathological features and prognosis in patients with laryngeal cancer. Lin Chuang Er Bi Yan Hou Tou Jing Wai Ke Za Zhi 2020;34:1128-31. [PubMed]
- Fraune C, Yehorov S, Luebke AM, et al. Upregulation of PTTG1 is associated with poor prognosis in prostate cancer. Pathol Int 2020;70:441-51. [Crossref] [PubMed]
- Long L, Gao J, Zhang R. PTTG1 Enhances Oncolytic Adenovirus 5 Entry into Pancreatic Adenocarcinoma Cells by Increasing CXADR Expression. Viruses 2023;15:1153. [Crossref] [PubMed]
- Lin H, Chen QL, Wang XY, et al. Clinical significance of pituitary tumor transforming gene 1 and transgelin-2 in pancreatic cancer. Int J Immunopathol Pharmacol 2013;26:147-56. [Crossref] [PubMed]
- Obayashi T, Kagaya Y, Aoki Y, et al. COXPRESdb v7: a gene coexpression database for 11 animal species supported by 23 coexpression platforms for technical evaluation and evolutionary inference. Nucleic Acids Res 2019;47:D55-62. [Crossref] [PubMed]
- Tang Z, Li C, Kang B, et al. GEPIA: a web server for cancer and normal gene expression profiling and interactive analyses. Nucleic Acids Res 2017;45:W98-W102. [Crossref] [PubMed]
- Chen J, Liu C, Cen J, et al. KEGG-expressed genes and pathways in triple negative breast cancer: Protocol for a systematic review and data mining. Medicine (Baltimore) 2020;99:e19986. [Crossref] [PubMed]
- Chu LC, Goggins MG, Fishman EK. Diagnosis and Detection of Pancreatic Cancer. Cancer J 2017;23:333-42. [Crossref] [PubMed]
- Wang Y, Yang G, You L, et al. Role of the microbiome in occurrence, development and treatment of pancreatic cancer. Mol Cancer 2019;18:173. [Crossref] [PubMed]
- Karamitopoulou E. Tumour microenvironment of pancreatic cancer: immune landscape is dictated by molecular and histopathological features. Br J Cancer 2019;121:5-14. [Crossref] [PubMed]
- Skorupan N, Palestino Dominguez M, Ricci SL, et al. Clinical Strategies Targeting the Tumor Microenvironment of Pancreatic Ductal Adenocarcinoma. Cancers (Basel) 2022;14:4209. [Crossref] [PubMed]
- Vlotides G, Eigler T, Melmed S. Pituitary tumor-transforming gene: physiology and implications for tumorigenesis. Endocr Rev 2007;28:165-86. [Crossref] [PubMed]
- Williams GH, Stoeber K. The cell cycle and cancer. J Pathol 2012;226:352-64. [Crossref] [PubMed]
- Wang Y, Wang Y, Duan X, et al. Interleukin-1 receptor-associated kinase 1 correlates with metastasis and invasion in endometrial carcinoma. J Cell Biochem 2018;119:2545-55. [Crossref] [PubMed]
- Lim SM, Jang HY, Lee JE, et al. Alteration of Pituitary Tumor Transforming Gene-1 Regulates Trophoblast Invasion via the Integrin/Rho-Family Signaling Pathway. PLoS One 2016;11:e0149371. [Crossref] [PubMed]
- Xu W, McArthur G. Cell Cycle Regulation and Melanoma. Curr Oncol Rep 2016;18:34. [Crossref] [PubMed]
- Oi N, Yamamoto H, Langfald A, et al. LTA4H regulates cell cycle and skin carcinogenesis. Carcinogenesis 2017;38:728-37. [Crossref] [PubMed]
- Cao J, Wu N, Han Y, et al. DDX21 promotes gastric cancer proliferation by regulating cell cycle. Biochem Biophys Res Commun 2018;505:1189-94. [Crossref] [PubMed]
- Zhu B, Zhang Q, Wu Y, et al. SNAP23 suppresses cervical cancer progression via modulating the cell cycle. Gene 2018;673:217-24. [Crossref] [PubMed]
- Xia P, Liu P, Fu Q, et al. Long noncoding RNA EPIC1 interacts with YAP1 to regulate the cell cycle and promote the growth of pancreatic cancer cells. Biochem Biophys Res Commun 2020;522:978-85. [Crossref] [PubMed]
- Asghar U, Witkiewicz AK, Turner NC, et al. The history and future of targeting cyclin-dependent kinases in cancer therapy. Nat Rev Drug Discov 2015;14:130-46. [Crossref] [PubMed]
- Schmidt M, Rohe A, Platzer C, et al. Regulation of G2/M Transition by Inhibition of WEE1 and PKMYT1 Kinases. Molecules 2017;22:2045. [Crossref] [PubMed]
- Qian JY, Gao J, Sun X, et al. KIAA1429 acts as an oncogenic factor in breast cancer by regulating CDK1 in an N6-methyladenosine-independent manner. Oncogene 2019;38:6123-41. [Crossref] [PubMed]
- Hsu PC, Yang CT, Jablons DM, et al. The Crosstalk between Src and Hippo/YAP Signaling Pathways in Non-Small Cell Lung Cancer (NSCLC). Cancers (Basel) 2020;12:1361. [Crossref] [PubMed]
- Piao J, Zhu L, Sun J, et al. High expression of CDK1 and BUB1 predicts poor prognosis of pancreatic ductal adenocarcinoma. Gene 2019;701:15-22. [Crossref] [PubMed]
- Yang W, Cho H, Shin HY, et al. Accumulation of cytoplasmic Cdk1 is associated with cancer growth and survival rate in epithelial ovarian cancer. Oncotarget 2016;7:49481-97. [Crossref] [PubMed]
- Dou C, Sun L, Jin X, et al. Long non-coding RNA colon cancer-associated transcript 1 functions as a competing endogenous RNA to regulate cyclin-dependent kinase 1 expression by sponging miR-490-3p in hepatocellular carcinoma progression. Tumour Biol 2017;39:1010428317697572. [Crossref] [PubMed]
- Ravindran Menon D, Luo Y, Arcaroli JJ, et al. CDK1 Interacts with Sox2 and Promotes Tumor Initiation in Human Melanoma. Cancer Res 2018;78:6561-74. [Crossref] [PubMed]
- Li X, Ma XL, Tian FJ, et al. Downregulation of CCNA2 disturbs trophoblast migration, proliferation, and apoptosis during the pathogenesis of recurrent miscarriage. Am J Reprod Immunol 2019;82:e13144. [Crossref] [PubMed]
- Akef A, McGraw K, Cappell SD, et al. Ribosome biogenesis is a downstream effector of the oncogenic U2AF1-S34F mutation. PLoS Biol 2020;18:e3000920. [Crossref] [PubMed]
- Cai Y, Mei J, Xiao Z, et al. Identification of five hub genes as monitoring biomarkers for breast cancer metastasis in silico. Hereditas 2019;156:20. [Crossref] [PubMed]
- Gopinathan L, Tan SL, Padmakumar VC, et al. Loss of Cdk2 and cyclin A2 impairs cell proliferation and tumorigenesis. Cancer Res 2014;74:3870-9. [Crossref] [PubMed]
- Kanakkanthara A, Jeganathan KB, Limzerwala JF, et al. Cyclin A2 is an RNA binding protein that controls Mre11 mRNA translation. Science 2016;353:1549-52. [Crossref] [PubMed]
- Ma Q. MiR-219-5p suppresses cell proliferation and cell cycle progression in esophageal squamous cell carcinoma by targeting CCNA2. Cell Mol Biol Lett 2019;24:4. [Crossref] [PubMed]
- Iemoto T, Nishiumi S, Kobayashi T, et al. Serum level of octanoic acid predicts the efficacy of chemotherapy for colorectal cancer. Oncol Lett 2019;17:831-42. [PubMed]
- Li C, Xu J. Identification of Potentially Therapeutic Target Genes of Hepatocellular Carcinoma. Int J Environ Res Public Health 2020;17:1053. [Crossref] [PubMed]
- Wang F, Tian X, Zhang Z, et al. Demethylzeylasteral (ZST93) inhibits cell growth and enhances cell chemosensitivity to gemcitabine in human pancreatic cancer cells via apoptotic and autophagic pathways. Int J Cancer 2018;142:1938-51. [Crossref] [PubMed]
- Shekhar R, Priyanka P, Kumar P, et al. The microRNAs miR-449a and miR-424 suppress osteosarcoma by targeting cyclin A2 expression. J Biol Chem 2019;294:4381-400. [Crossref] [PubMed]
- Chen ZH, Jing YJ, Yu JB, et al. ESRP1 Induces Cervical Cancer Cell G1-Phase Arrest Via Regulating Cyclin A2 mRNA Stability. Int J Mol Sci 2019;20:3705. [Crossref] [PubMed]
- Chen X, Wang L, Su X, et al. Identification of potential target genes and crucial pathways in small cell lung cancer based on bioinformatic strategy and human samples. PLoS One 2020;15:e0242194. [Crossref] [PubMed]
- Manchado E, Eguren M, Malumbres M. The anaphase-promoting complex/cyclosome (APC/C): cell-cycle-dependent and -independent functions. Biochem Soc Trans 2010;38:65-71. [Crossref] [PubMed]
- Cheng S, Castillo V, Sliva D. CDC20 associated with cancer metastasis and novel mushroom-derived CDC20 inhibitors with antimetastatic activity. Int J Oncol 2019;54:2250-6. [Crossref] [PubMed]
- Chi JJ, Li H, Zhou Z, et al. A novel strategy to block mitotic progression for targeted therapy. EBioMedicine 2019;49:40-54. [Crossref] [PubMed]
- Zhao L, Zhang J, Liu Z, et al. Identification of biomarkers for the transition from low-grade glioma to secondary glioblastoma by an integrated bioinformatic analysis. Am J Transl Res 2020;12:1222-38. [PubMed]
- Zhang Q, Huang H, Liu A, et al. Cell division cycle 20 (CDC20) drives prostate cancer progression via stabilization of β-catenin in cancer stem-like cells. EBioMedicine 2019;42:397-407. [Crossref] [PubMed]
- Zhang Y, Xue YB, Li H, et al. Inhibition of Cell Survival by Curcumin Is Associated with Downregulation of Cell Division Cycle 20 (Cdc20) in Pancreatic Cancer Cells. Nutrients 2017;9:109. [Crossref] [PubMed]
- Wei R, Wang Z, Zhang Y, et al. Bioinformatic analysis revealing mitotic spindle assembly regulated NDC80 and MAD2L1 as prognostic biomarkers in non-small cell lung cancer development. BMC Med Genomics 2020;13:112. [Crossref] [PubMed]
- Zhu XF, Yi M, He J, et al. Pathological significance of MAD2L1 in breast cancer: an immunohistochemical study and meta analysis. Int J Clin Exp Pathol 2017;10:9190-201. [PubMed]
- Zhou G, Zhao M, Liang R, et al. A Study of the Mechanism of Binding between Neratinib and MAD2L1 Based on Molecular Simulation and Multi-spectroscopy Methods. Curr Pharm Des 2019;25:4287-95. [Crossref] [PubMed]
- Li J, He X, Wu X, et al. miR-139-5p Inhibits Lung Adenocarcinoma Cell Proliferation, Migration, and Invasion by Targeting MAD2L1. Comput Math Methods Med 2020;2020:2953598. [Crossref] [PubMed]
- Lu S, Sun C, Chen H, et al. Bioinformatics Analysis and Validation Identify CDK1 and MAD2L1 as Prognostic Markers of Rhabdomyosarcoma. Cancer Manag Res 2020;12:12123-36. [Crossref] [PubMed]
- Wang Y, Wang F, He J, et al. miR-30a-3p Targets MAD2L1 and Regulates Proliferation of Gastric Cancer Cells. Onco Targets Ther 2019;12:11313-24. [Crossref] [PubMed]