Identification of fatty acid oxidation-related subtypes by integrated analysis of bulk- and single-cell transcriptome profiling in colorectal cancer
Highlight box
Key findings
• This study found that patterns vary in patients with colorectal cancer (CRC) in terms of fatty acid oxidation (FAO), and developed a novel risk-scoring system that contributes to the personalized and stratified treatment of CRC.
What is known and what is new?
• The existing studies have proved that changes in FAO metabolites and gene expression levels in the FAO pathway are related to the progress of CRC.
• An efficient corresponding metabolic classifier to disclose the unclear phenotypes of FAO in patients with CRC is of well worth.
What is the implication, and what should change now?
• Patients with CRC were divided into high- and low-enrichment groups by FAO_Cluster. GFAO_Score risk scoring system can evaluate the metabolic enrichment profile of FAO in patients to some extent, which may be conducive to accurate treatment strategies in colorectal malignancy.
Introduction
Ranked third among the most common neoplasm categories, colorectal cancer (CRC), the main cause of cancer-related deaths, had approximately 1.9 million new occurrences in 2020 (1,2). As a standard treatment for resectable CRC, the radical operation followed by adjuvant chemotherapy has been unsatisfactory for advanced CRC (3). CRC is characterized by molecular alterations followed by the dysregulation of various carcinogenic pathways that lead to malignancy invasiveness (4). Therefore, markers related to prognosis are warranted, and it is needed to elucidate potential molecular mechanisms for CRC progression.
Metabolic reprogramming has been proven as a remarkable hallmark of malignant progression (5-7). As for tumor cells, dysregulation of lipid metabolism is one of the vital metabolic characteristics, which enables cancer cells to contend with limitations about energy sources (8,9). The abnormal alteration of fatty acid β-oxidation (FABO) is a frequent feature of metabolic reprogramming in carcinoma, in which fatty acids (FAs) are decomposed to produce acetyl-CoA, participate in Kreb’s Cycle and produce ATP, NADPH, NADH, and FADH2 (10-12). FAs are essential for energy consumption in the tumor microenvironment (TME) (13). TME plays an important role in the occurrence and metastatic progression of malignancy, which consists of numerous categories of infiltrating cells, especially the immune cell types. They regulate tumor progress and metastasis by modifying the construction of TME. The previous research demonstrated that fatty acid oxidation (FAO) favors malignant cell proliferation, and impacts survival via drug resistance (14). It has been illustrated that a metabolic shift toward carbohydrate and lipid metabolism accounts for the adaptation to VEGF blockade. Restricting the lipid synthesis controls distal metastasis after withdrawal of antiangiogenic treatment in anti-tumor therapy (15). Besides, adipocytes facilitate the initial shelter of tumor cells to the omentum by providing FAs, fueling their progression (16). The promotion of self-renewal ability and drug resistance was related to FAO (17,18). Thus, the recognition of the status of FAO in CRC may predict the prognosis of individual patients. However, it is impossible to analyze such an amount of sequencing data to screen the specific patients who may benefit from adjuvant therapies in clinical practice. Therefore, identifying patients for concrete anti-tumor strategies is worthy of exploration in CRC.
In this study, we aimed to construct a novel signature related to FAO to identify certain patients with CRC based on bulk RNA sequencing and single-cell RNA (scRNA) sequencing data, which contributes to the following additional treatments. Furthermore, we explored the predictive capacity and adjuvant therapeutic performance of the signature by external validation cohorts. This study might help identify specific patients with CRC to optimize treatment strategies and enhance their prognosis in chemotherapy and immunotherapy. We present this article in accordance with the TRIPOD reporting checklist (available at https://jgo.amegroups.com/article/view/10.21037/jgo-23-833/rc).
Methods
Publicly databases collection and processing
Transcriptome data of 605 patients and clinical information of 536 patients with CRC were obtained from The Cancer Genome Atlas (TCGA)-Genomic Data Commons (GDC) (https://cancergenome.nih.gov/) and TCGA Pan-Cancer Clinical Data Resource respectively. The gene sets used in this study were all accessed from the molecular signatures database (MSigDB). In order to evaluate the FAO metabolism of patients in multiple dimensions, we collected all the gene sets related to FAO, totally seven gene sets (Table 1). In order to explore the relationship between the colorectal carcinogenesis pathway and FAO metabolism, ten carcinogenic signaling pathway gene sets were collected (the supplementary table available at: https://cdn.amegroups.cn/static/public/10.21037jgo-23-833-1.xlsx). Transcriptome data and survival information of 867 patients with CRC were downloaded from three Gene Expression Omnibus (GEO) datasets, including GSE39582, GSE17536, and GSE72970 (https://www.ncbi.nlm.nih.gov/geo/), as the external verification queues. For further scRNA sequencing analysis and verification, we selected the 10 tumor samples with their corresponding normal samples from GSE132465 (in a total of 23 samples 10 of which had both tumor and normal specimens) with a total of 22,793 cells. The study was conducted in accordance with the Declaration of Helsinki (as revised in 2013).
Table 1
FAO gene sets | Online source | Gene name |
---|---|---|
FATTY_ACID_OXIDATION | https://www.gsea-msigdb.org/gsea/msigdb/human/geneset/FATTY_ACID_OXIDATION | ACADS, ACADVL, ADIPOR1, ADIPOR2, ALOX12, BDH2, CPT1A, CPT1B, ECH1, ECHS1, HACL1, HADHB, HAO1, HAO2, PPARA, PPARD, PPARGC1A |
GOBP_FATTY_ACID_ALPHA_OXIDATION | https://www.gsea-msigdb.org/gsea/msigdb/human/geneset/GOBP_FATTY_ACID_ALPHA_OXIDATION | HACL1, HAO1, ILVBL, PEX13, PHYH, SLC25A17, SLC27A2 |
GOBP_FATTY_ACID_BETA_OXIDATION | https://www.gsea-msigdb.org/gsea/msigdb/human/geneset/GOBP_FATTY_ACID_BETA_OXIDATION | ABCB11, ABCD1, ABCD2, ABCD3, ABCD4, ACAA1, ACAA2, ACACB, ACAD10, ACAD11, ACADL, ACADM, ACADS, ACADVL, ACAT1, ACAT2, ACOT8, ACOX1, ACOX2, ACOX3, ACOXL, ADIPOQ, AKT1, AKT2, ALDH1L2, AMACR, AUH, BDH2, CNR1, CPT1A, CPT1B, CPT1C, CPT2, CRAT, CROT, DECR1, DECR2, ECH1, ECHDC1, ECHDC2, ECHS1, ECI1, ECI2, EHHADH, ETFA, ETFB, ETFBKMT, ETFDH, FABP1, GCDH, HADH, HADHA, HADHB, HSD17B10, HSD17B4, IRS1, IRS2, IVD, LEP, LONP2, MCAT, MFSD2A, MLYCD, MTLN, PEX2, PEX5, PEX7, PLIN5, PPARA, PPARD, SCP2, SESN2, SLC25A17, SLC27A2, TWIST1, TYSND1 |
GOBP_FATTY_ACID_OMEGA_OXIDATION | https://www.gsea-msigdb.org/gsea/msigdb/human/geneset/GOBP_FATTY_ACID_OMEGA_OXIDATION | ADH4, ADH5, ADH7, CYP24A1, CYP4F2, CYP4V2 |
REACTOME_BETA_OXIDATION_OF_VERY_LONG_CHAIN_FATTY_ACIDS | https://www.gsea-msigdb.org/gsea/msigdb/human/geneset/REACTOME_BETA_OXIDATION_OF_VERY_LONG_CHAIN_FATTY_ACIDS | ABCD1, ACAA1, ACOT4, ACOT6, ACOT8, ACOX1, DECR2, ECI2, EHHADH, HSD17B4, MLYCD |
WP_FATTY_ACID_BETAOXIDATION | https://www.gsea-msigdb.org/gsea/msigdb/human/geneset/WP_FATTY_ACID_BETAOXIDATION | ACADL, ACADM, ACADS, ACADVL, ACAT1, ACSL1, ACSL3, ACSL4, ACSL5, ACSL6, ACSS2, CHKB, CPT1A, CPT1B, CPT2, CRAT, DECR1, DLD, ECHS1, ECI1, GCDH, GK, GK2, GPD2, HADH, HADHA, HADHB, LIPC, LIPE, LIPF, LPL, PNPLA2, SLC25A20, TPI1 |
WP_FATTY_ACID_OMEGAOXIDATION | https://www.gsea-msigdb.org/gsea/msigdb/human/geneset/WP_FATTY_ACID_OMEGAOXIDATION | ADH1A, ADH1B, ADH1C, ADH4, ADH6, ADH7, ALDH1A1, ALDH2, CYP1A1, CYP1A2, CYP2A6, CYP2D6, CYP2E1, CYP3A4, CYP4A11 |
FAO, fatty acid oxidation; MsigDB, molecular signatures database.
Construction and validation of the FAO score
Firstly, based on seven gene sets related to FAO, single-sample gene set enrichment analysis (ssGSEA) was used to evaluate the metabolism level of FAO in patients with CRC. We generated seven FAO scores for CRC individuals, which indicated the enrichment degree of gene sets in the samples. We determined the optimal cutpoints of seven FAO scores according to survminer package. Based on the optimal cutpoints, we stratified patients into high and low FAO score groups seven times and performed Kaplan-Meier (K-M) survival analyses to explore the difference in overall survival (OS) each time.
Unsupervised clustering algorithm was applied to cluster analysis of FAO scores in 511 CRC samples. FAO scores consist of seven different FAO scores (GO_FAAO, GO_FABO, GO_FAOO, FAO, WP_FABO, WP_FAOO, and REACTOME_FAO scores) originated from seven gene sets related to FAO. We used the ConsensusClusterPlus package for the above steps and conducted 1000 repetitions to ensure the stability of the classification. Lastly, the patients were divided into two subtypes, Cluster 1 and Cluster 2, according to the parameter kappa 2. Taking the P value less than 0.05 as statistically significant, the OS and progression-free survival (PFS) analyses of the two subtypes were subsequently performed. In accordance with seven different FAO scores, patients with CRC were classified into high and low-score groups by the optimal cutoff value followed by K-M analysis for comparison of OS.
Based on seven FAO scores and the k-means clustering analysis of unsupervised learning, the patients were divided into two subtypes, Cluster 1 and Cluster 2, according to the parameter kappa 2. Taking the P value less than 0.05 as statistically significant, the OS and PFS analyses of the two subtypes were subsequently performed.
To assess the FAO metabolism status between the two subtypes, we additionally compared the enrichment scores of seven FAO metabolic pathways and the expression of key regulatory genes in FAO, including ACADVL, CPT1A, ACADM, CPT2, and ACOX1.
Gene set enrichment analysis (GSEA, http://www.broadinstitute.org/gsea) was used to identify the differences in enrichment degree between Cluster 1 and Cluster 2 of ten classical carcinogenic signaling pathways. Transcriptome data and survival information of 867 patients with CRC from GEO were collected as an external verification cohort, divided into two groups for further gene pathway enrichment and prognosis analyses based on FAO scores.
scRNA sequencing data analysis and validation
Seurat, R package (19) was applied to transfer scRNA-seq data into Seurat objects. We performed quality control (QC) by filtering out genes expressed in less than 3 cells and disregarded cells with less than 300 genes expressed. Cells with fewer than 1,000 or more than 20,000 unique molecular identifiers (UMI) were excluded. The expression of mitochondrial, ribosomal, and hemoglobin genes was calculated. To remove the low-activity cells, cells with a low ribosome (<3%), high mitochondria (>10%), and hemoglobin content (>0.1%) were discarded.
After filtering out poor-quality cells, a total of 16,658 cells were retained for further analysis (Figure S1). For clustering, highly variable genes were selected, and the principal components based on those genes were used to build a graph, which was segmented with a resolution of 0.6. Single-cell clustering was visualized by t-distributed stochastic neighbor embedding (t-SNE). After data homogenization and batch effect removal, cells were clustered by resolution parameter (as 0.5), determined by Clustree package. Further, singleR package was applied to identify and name the clusters. Percentage, ssGSEA, AddModule, and AUC methods were used to calculate the FAO scores after clustering.
We collected 87 genes (FAO 87) from GOBP FATTY ACID OXIDATION in MsigDB (https://amigo.geneontology.org/amigo/term/GO:0019395) and further established a signature to evaluate the status of FAO at cell level. After the selection of the epithelial cells, we performed data normalization, dimensionality reduction, and re-clustering to classify the epithelial cells into two subtypes, C0 and C1, with a resolution of 0.2. The difference between C0 and C1 in terms of FAO 87-gene signature scores and enrichment of carcinogenic signaling pathways were analyzed.
Development and evaluation of GFAO_Score
To enhance the practicability of the classification based on FAO score, we decided to generate a more convenient signature to help detect certain patients with CRC. Therefore, we used three R packages with different methods (DESeq2, edgeR, and Limma) to detect differentially expressed genes (DEGs) by taking the intersection between C0 and C1 that met the screening parameters [log2 fold change |logFC| >1, and false discovery rate (FDR) <0.05]. Venn diagrams were used to determine the intersection of DEGs generated from three methods. DEGs in the above intersection were introduced into a univariate Cox regression along with survival information of patients with CRC to identify differentially expressed prognostic genes, which were defined as hub genes. Using the glmnet R package, least absolute shrinkage and selection operator (LASSO) regression analysis was further performed on hub genes in order to avoid multicollinearity and overfitting as well as develop the final model. Subsequently, scores of hub genes in FAO (GFAO_Score) were defined using the following formula: GFAO_Score = ΣGene expreeion × Coef.
Where gene expression is the expression profile of the hub genes, and Coef is the risk coefficient.
Divisions were established with high and low scores according to the medium value of GFAO_Score. Subsequently, a prognostic assessment was calculated using the receiver operating characteristic (ROC) curve method in the timeROC package. K-M analysis revealed survival curves across the CRC training (TCGA) and validation cohort (GEO). To evaluate the independence of GFAO_Score in prognosis, multivariate Cox regression analyses were conducted. In addition, relationships between GFAO_Score and survival information [vital status and The American Joint Committee on Cancer (AJCC) stages] were also analyzed.
We explored the potential performance of GFAO_Score in anti-tumor treatments including immunotherapy and chemotherapy. To assess the immunotherapy effect, tumor immune dysfunction and exclusion (TIDE) analysis (http://tide.dfci.harvard.edu) web tool was used to predict the responses of patients with CRC. For an indication of systematical assessment of immunological activity and immunotherapy in CRC, we calculated the international prognostic scores (IPS) of high and low score groups of GFAO_Score. Furthermore, we collected 233 patients with CRC in total from GSE39582 who had received adjuvant chemotherapy after surgical resection and 133 patients underwent integrated therapy from GSE63216, in order to evaluate the predictivity of GFAO_Score. Later, the predictive efficacy of GFAO_Score in other solid tumors was also validated in the immunotherapy cohort of 348 patients with bladder cancer in IMvigor210.
The drug analysis datasets were collected from the Genomics of Drug Sensitivity in Cancer (GDSC) (https://www.cancerrxgene.org/) for the drug sensitivity assessment of GFAO_Score in patients with CRC using Oncopredict R package. Based on the GFAO_Score grouping, the relationships between the subgroups and drug sensitivity were determined in the aspect of chemotherapy and immunotherapy using the Wilcox test.
Statistical analysis
Data analyses and graphing were performed using R software (4.1.1). Cox regressions and K–M analyses were conducted using the survival R package with statistically significant differences assessed by the log-rank test. The comparison of count data was assessed by Fisher’s precision probability test and chi-square test. Wilcox test was used to compare non-normal distribution data between subgroups. Spearman analysis was applied to assess the correlations between linearly related variables. The optimal cutoff value was formulated by the survminer package. The time-dependent area under the ROC curve (AUC) for survival variables was plotted using the timeROC package. The value of two-tailed P<0.05 indicates statistically significant differences.
Results
Construction and validation of the FAO score
Construction of the FAO score
Using the ssGSEA to illustrate individual metabolism levels of FA, we generated the seven FAO scores for each patient with CRC according to seven FAO pathway gene sets from MSigDB. The K-M survival curves between the high and low score groups implemented by an optimal cutoff value are depicted in Figure S2. In total, the results indicated that patients with low FAO scores tended to have dismal survival outcomes compared to patients with high scores.
Unsupervised consensus clustering based on the FAO score
Screened by the standard parameters mentioned above, unsupervised consensus clustering was conducted to categorize patients into two clusters based on the seven FAO scores in the training set, with 304 patients in Cluster 1 and 232 patients in Cluster 2 respectively (Figure 1A). We appraised the survival curves by setting OS and PFS as the prognostic endpoints (Figure 1B,1C). It showed that there were significant statistical differences between the two groups of patients. Patients in Cluster 1 had a more favorable survival rate than those in Cluster 2.
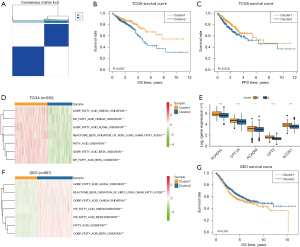
Evaluation and validation of the clustering
The enrichment status of FAO in patients in Cluster 1 and Cluster 2 from the training dataset was assessed and calculated by the ssGSEA in seven FAO pathways. The heatmap of enrichment of FAO metabolism pathways showed that Cluster 1 has a rather higher FAO level, which had been in line with our preliminary results (Figure 1D). Additionally, to depict the expression profiles of key genes of FAO between Cluster 1 and Cluster 2, a boxplot was utilized to display the difference in ACADVL, CPT1A, ACADM, CPT2, and ACOX1, which indicated that the regularity genes were all highly expressed in Cluster 1 (Figure 1E).
GSEA was applied to screen the differences in enrichment pathways between the two clusters through the cell cycle, and classical carcinogenic signaling pathways in the MSigDB. We found that carcinogenic genes were enriched in different statuses between Cluster 1 and Cluster 2. The GSEA results suggested that Cluster 2 exhibited markedly higher transcriptional levels in the period of tumorigenesis, including CELL CYCLE, HIPPO, MAPK, NOTCH, PI3K-AKT, RAS, TGFB, and WNT signaling pathways (Figure S3).
In order to evaluate the stability of the results of our unsupervised consensus clustering, three datasets [GSE39582 (N=566), GSE17536 (N=177), and GSE72970 (N=124)] were collected from the GEO database as the external validation cohort after merging data and removing batch effect. Patients in the merged cohort were clearly categorized into two clusters. We used the heatmap to show that the seven FAO pathways were rather more highly expressed and enriched in Cluster 2 than those in Cluster 1, indicating possession of the preferable survival time (Figure 1F). In addition, the survival analysis presented statistical differences in survival outcomes based on the FAO scores, which was consistent with the results of TCGA cohort (Figure 1G).
Identification of expression profile of FAO at scRNA sequencing analysis
We then sought to determine whether the difference in FAO metabolism between the two clusters can be distinguished at the single-cell level. The single-cell resolution level assists in understanding the precise landscape of subclonal diversity. We selected ten CRC samples from GEO: GSE132465, a total of 22,793 cancer cells. We performed gene filtering, normalization, and dimensionality reduction and finally identified nine distinct clusters, including B cells, endothelial cells, epithelial cells, macrophage, monocyte, natural killer (NK) cell, pre-B cell CD34−, smooth muscle cells, and T cells (Figure 2A-2C). In order to evaluate the difference in FAO enrichment among cell clusters, we used a FAO 87-gene signature (FAO 87; see Methods section) and four common single-cell gene set scoring methods, and it was found that the gene set enrichment score of epithelial cells was significantly higher than that of other cell clusters (Figure 2D,2E and Figure S3). In order to further explore the difference in FAO metabolism in tumor epithelial cells, we extracted epithelial cells and classified to Cluster 0 (C0) and Cluster 1 (C1) (Figure 2F-2H). We found that the FAO 87-gene signature score of the epithelial subtype was higher in C0 than those of C1, while the classical carcinogenic pathway enrichment score and epithelial-mesenchymal transition (EMT) score (AddModule, AUC) were both lower in C0, suggesting that FAO gene enrichment may be associated with better prognosis (Figure 2I-2N), which was consistent with the results in TCGA that patients with lower expression of FAO might lead poor survival outcomes.
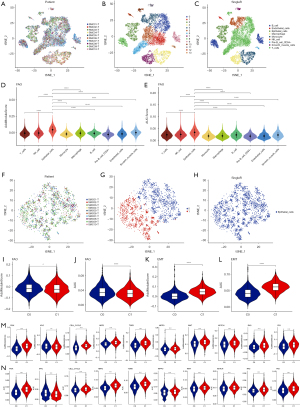
Development and evaluation of the GFAO_Score
Construction and validation of the GFAO_Score
The differences in terms of biological behaviors and survival outcomes between Cluster 1 and Cluster 2 in TCGA were further explored. We applied three commonly used methods of differential gene expression analysis, which include DESeq2, edgeR, and Limma methods, to identify 355, 320, and 644 DEGs respectively (Figure S4). Therefore, a total of 107 genes related to the FAO pathway were screened by taking the intersection of DEG results generated from three different methods between Cluster 1 and Cluster 2 (Figure 3A). Based on the intersection of DEG results, univariate Cox regression was performed to decrease redundancy and three prognosis-related DEGs remained. As a result, ZFHX4, AQP8, and AKR1B10 were further subjected to LASSO regression analysis for the construction of GFAO_Score. The formula for the prognostic risk assessment of the GFAO_Score was defined as follows: GFAO_Score = (0.5323323498) × Exp (ZFHX4) + (0.0058440605) × Exp (AQP8) + (-0.0156539071) × Exp (AKR1B10), where “Exp” represents the expression profile of the individual of selected DEGs. The GFAO_Score in Cluster 2 was significantly higher than that in Cluster 1 (Figure 3B), which indicated that the GFAO_Score could represent the FAO level and detect certain patients with CRC. ROC analysis was performed to assess the efficiency of GFAO_Score with the AUCs for 1-, 3-, and 5-year OS prediction, which was 0.59, 0.60, and 0.70, respectively (Figure 3C). Furthermore, patients in the TCGA training cohort were assigned into two groups, with 255 and 256 patients in high and low GFAO_Score groups sorted by the medium value. Additionally, K-M analysis revealed an unfavorable OS and PFS in the high GFAO_Score group (Figure 3D,3E). In the GEO validation cohort, patients with high GFAO_Score also had the unfavorable prognosis (Figure 3F). Patients in death revealed significantly higher GFAO_Score in vital status analysis. Patients classified in the high GFAO_Score group leaned towards the more advanced AJCC stage and lymph node status with statistical significance (Figure 3G). The multivariate Cox regression analyses including age, gender, AJCC, and TNM, were calculated to show that GFAO_Score was an independent risk factor for CRC (P<0.05) (Figure 3H). In addition, we collected GSE39582 as the validation cohort from the GEO database to evaluate the performance of GFAO_Score. The results also showed that patients in advanced clinical stages in AJCC classification were detected with higher GFAO_Score (Figure 3I), consistent with the previous validation in the TCGA cohort.
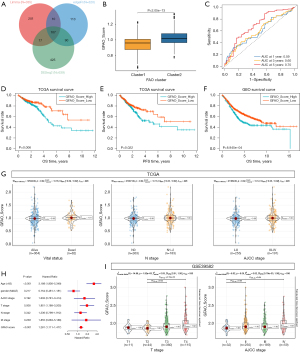
Immunological assessment of the GFAO_Score
The IPS score was used to evaluate the potential treatment outcomes of immunotherapy. Patients with higher IPS values received better responses to immunotherapy with CTLA-4 monoclonal antibody or PD-1 monoclonal antibody. The results showed a tendency for patients with higher IPS to obtain lower GFAO_Score (Figure 4A).
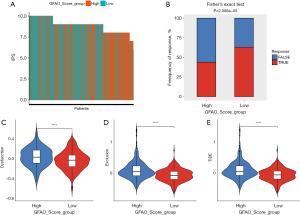
The TIDE score was applied as a predictor of treatment outcomes after immune checkpoint blockade was used. There was a higher proportion of patients possessing lower response rates to the immunotherapy in the high GFAO_Score group (Figure 4B). The violin plots revealed that the TIDE scores, exclusion scores, and dysfunction scores in the low GFAO_Score group were lower than that in the high GFAO_Score group, which meant that patients with high GFAO_Scores might tend to expose to the immune escape and worse response to immune checkpoint therapies (Figure 4C-4E).
Analysis of adjuvant therapies and drug sensitivity
In order to evaluate the value of the GFAO_Score in integrated anti-tumor therapy, 233 and 133 patients with CRC from GSE39582 and GSE63216 were collected for survival analysis respectively. The results illustrated that in GSE39582, patients with lower GFAO_Scores would prefer a better prognosis in adjuvant chemotherapy (Figure 5A). Besides, patients with low GFAO_Score tended to possess longer survival time receiving capecitabine, oxaliplatin, and bevacizumab treatments in GSE63216 (Figure 5B). In order to evaluate the predictive performance in other tumors, we also assessed the GFAO_Score of 348 patients with bladder cancer in the IMvigor210 cohort. Patients with low GFAO_Score had better survival outcomes, whereas those with high GFAO_Score presented the opposite results in K-M analysis (Figure 5C).
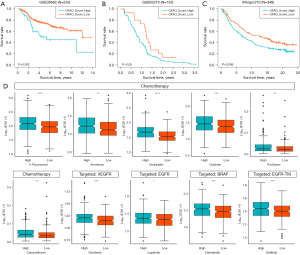
In addition, we calculated the IC50 (50% inhibition concentration) of 198 medications from GDSC for high and low GFAO_Score groups in the training cohort. Filtering the abnormalities, six widely applied chemotherapy drugs are shown in Figure 5D, including 5-fluorouracil, irinotecan, oxaliplatin, cisplatin, paclitaxel, and camptothecin in the low GFAO_Score group had significantly lower IC50 values than those in the high GFAO_Score group, which revealed that patients with lower GFAO_Score might benefit from chemotherapy. Inhibitors of tumor activation pathways were further explored to identify the differences between the two subgroups. Screening for target drugs, we analyzed the IC50 and found the lower values of sorafenib (the anti-VEGFR drug), lapatinib (the anti-EGFR/HER2 drug), dabrafenib (the anti-BRAF drug), gefitinib (the EGFR-TKI drug) in the low GFAO_Score group (Figure 5D), indicating the preferable vital status in patients in target therapy.
Discussion
The incidence of CRC in younger adults has increased in recent years (1). Inconclusive conventional approaches may lead to inappropriate prescription-making and surveillance (20). Personalized assessment approaches exhibited various survival outcomes in CRC. With an in-depth understanding of the complexity of tumors, it was found that metabolic reprogramming is an important marker of tumors. In recent years, the change in energy metabolism is generally regarded as an important sign of cancer occurrence, development, and treatment resistance, in which the imbalance of lipid metabolism has been proven to be an important feature of tumor cell metabolism (19).
FA metabolism influences biological behaviors in different ways, typically including the synthesis of lipid building blocks for membranes, that is, glycerophospholipids, and signaling intermediates such as phosphatidylinositol (4,5)-bisphosphate, diacylglycerol (DAG) and phosphatidate to facilitate mitogenic and/or oncogenic signaling (21). FAs are a large, essential, energy source that can meet the substantial fuel needs of rapid invasiveness of carcinoma (22). Plenty of epidemiological evidence reveals that the recurrence and mortality rates of patients with CRC increase in obese people (23). The obesity-related impacts on tumor incidence, progression, and therapeutical efficacy will increasingly challenge malignant disease arrangement, which underscored the significance of FAO in CRC pathogenesis. Abnormal expression levels of metabolic enzymes involved in FA uptake, synthesis, and FAO have been found in many tumors, leading to FA metabolism reprogramming and promoting tumorigenesis and development (24). However, the mechanism of lipid metabolism reprogramming in CRC has not been fully explored, especially the alteration in FAO metabolism in patients with CRC. In this study, we identified the enrichment and inner interaction of FAO in individuals with CRC via TCGA and GEO databases. Development of the classifications according to bulk RNA and scRNA sequencing analysis indicated that patients who differed in levels of FAO tended to possess a variety of prognoses.
The role that FA metabolism plays in carcinogenesis has been illustrated in many studies. Tumor FA metabolism adapts to ‘macro-level’ host attributes and, at the local microenvironment level, influences disease behavior. Recent research related to gynecologic malignancy showed that adipocytes provide FAs to procedures of metastasis and activate the FAO pathway, which serves as an energy source to facilitate distal metastasis. Many categories of tumors co-localize with adipose tissue and exhibit a close relationship, such as breast cancer, prostate cancer, and ovarian cancer that grow or invade the adipose-rich areas (25-28). FAs can act as substrates for lipid synthesis and storage in cancer cells which serve as the energy pool. Some research showed that CPT1A, one of the key proteins of the FAO pathway, whose expression profile in breast cancer was required to metabolize adipocyte-derived FAs and supported the further invasion and EMT induced by adipocytes (29). In melanoma cancer cells, the increase of CPT1A remained more stable, which might remind the differences among cancer types (30). It has been suggested that the accumulation of FAs can promote the growth of CRC cells and liver metastasis. Among them, saturated FAs can participate in the tumor-related inflammatory microenvironment and promote the occurrence and development of CRC. Reducing the content of ultra-long chain FAs can inhibit the growth of tumor cells. In addition, FA metabolism is also closely related to immune cells in the TME. Tumor cells can release FAs for lipid communication with tumor-related macrophages, making tumor-related macrophages become “accomplices” to assist tumor cells in liver metastasis.
In CRC, we calculated the enrichment status of FAO in individuals using ssGSEA and classified patients into two subgroups by unsupervised consensus clustering. In order to verify its stability and repeatability, we conducted validation in several aspects: visualizing the differences in enrichment scores; clarifying the expression profile of key genes in FAO; exploring the enrichment of some gene pathways; and requiring other external data to support and verify the conclusion. According to the results, patients with high enrichment levels in FAO (Cluster 1) showed more favorable OS and PFS. The heterogeneity and vitro experimental model design may contribute to such a situation. However, we generated the FAO scores to evaluate the individual level via the bulk RNA sequencing data, which focused on the integrity of the genomic landscape and inner interaction about the enrichment of lipid metabolism in bioinformatics. To unclose the differences between Cluster 1 and Cluster 2, we drew a systematic analysis in terms of the clinical characteristics, and biological behaviors related to the FAO metabolism. The results suggested that compared to Cluster 1, Cluster 2 possessed higher transcriptional profiles about tumorigenesis, including CELL CYCLE, HIPPO, MAPK, NOTCH, PI3K-AKT, RAS, TGFB, and WNT pathways. Similar situations have been found in other cancer types like non-small cell lung cancer. A metabolic transition that inhibited FA synthesis and promoted energy provision has been identified as a crucial component of TGFβ1-induced EMT and metastasis (31). We subsequently used GEO data for external verification. The results suggested that the samples could also be clearly divided into two groups. Cluster 2 in GEO had a better prognosis than Cluster 1, labeled by a higher FAO enrichment score. It was also the case that the group with a high enrichment score in TCGA cohorts had a better survival outcome. Therefore, the performance of our clustering was stable and repeatable. Animal models are indispensable work in the study of CRC, which helps explore the biological progress of tumor growth and subsequently develop anti-tumor drugs. The mouse CRC model has been widely used in various malignancy research in the world. A previous study has verified that the alteration of FAO affects the progress of CRC through experiments of the mouse model in vivo (32). In addition, the signaling pathways of intestinal development, regeneration, and disease between drosophila and mammals are highly conserved (33). The previous study has proved that the change of expression of FAO-related genes has a significant influence on intestinal stem cells (ISC) based on a new CRISPR-Cas9 fly model, in which ISC is the established cell of origin for CRC in the mammalian intestine (34).
Following the previous analysis, we further focused on the scRNA sequencing method to clarify the correlation between FAO and tumor progression in CRC. The epithelial cells were screened for high expression of the FAO 87-gene signature, related to the oxidation of FAs in mitochondria. The epithelial cells were then extracted and clustered again into two clusters. The classical carcinogenic pathway label enrichment score and EMT score were apt to be lower in C0, suggesting that poor FAO enrichment may lead to disease deterioration.
However, it is of high cost and unavailable to conduct the bulk RNA sequencing procedure for the whole seven FAO gene sets to perform detection for patients with CRC. Considering the clinical practicality, we decided to generate a new signature based on the unsupervised consensus clustering of FAO. Therefore, three genes, ZFHX4, AQP8, and AKR1B10 were identified and subjected to the LASSO algorithm for the development of GFAO_Score, which showed excellent efficacy in prognosis and treatment response in chemotherapy and target therapy. According to the classification of GFAO_Score, we found that the high GFAO_Score group had an unoptimistic survival time compared to the low-score group. Adjuvant therapy response and external validation were subsequently evaluated.
Besides, considering the relationship between heterogeneity and clinical benefits of the two groups, the predictive performance of GFAO_Score in chemotherapy and target therapy was further assessed. Patients in the low-score group who had higher IPS values received better responses to immunotherapy with CTLA-4 or PD-1 application. In addition, we calculated the GFAO_Score in order to evaluate the immunotherapy response in the IMvigor210 cohort as external validation data, which also indicated that patients in the high GFAO_Score group presented unfavorable survival outcomes and poor sensitivity to immunotherapy. The resistance to immunotherapy challenges the standard treatment strategies nowadays. A long-chain fatty acyl-CoA synthetase inhibitor, triacsin C, that blocks FA activation and thereby lipid droplet biogenesis, which means lower the status of FAO, can enhance the sensitivity of chemotherapy of CRC cells in vitro and in mouse xenografts (35). Tumoral CPT1A expression was associated with poorer OS in patients with gastric cancer, a similar situation in CRC (18). Pharmacological regulation of FAO like CPT1 inhibitors, might consistently chemosensitized tumor cells. While further shreds of evidence in the real world are necessary to validate the efficacy of GFAO_Score.
As for drug sensitivity analysis, six commonly utilized chemotherapy drugs including 5-fluorouracil, irinotecan, oxaliplatin, paclitaxel, and camptothecin, were screened with the lower IC50 values in the low-score group, suggesting that patients who obtained the lower GFAO-Sore might benefit from chemotherapy. GFAO_Score also showed a good performance in the discrimination of patients sensitive to tumor pathway inhibitors in our study. Our signature has the potential to be a surrogate for prognostic and predictive assessment and drug benefit selection. This may also offer guidance for clinical medicine, such as decision-making strategies for immunotherapy based on our medication sensitivity analysis.
Moreover, we identified the three genes related to FA metabolism in CRC, which included ZFHX4, AQP8, and AKR1B10. With the max coefficient and highest expression level in the signature, AKR1B10, a member of the aldosterone reductase superfamily, is mainly expressed in the human small intestine and colon epithelial tissues. It has been previously mentioned that high expression of AKR1B10 in breast cancer is significantly positively correlated with genes related to FAO (36). In breast cancer, AKR1B10 can promote tumor migration and metastasis by activating the MAPK pathway (37) but regulates FAO to enhance the prognosis of CRC. However, there is no research exploring the regulation mechanism of FAO mediated by AKR1B10 in patients with CRC.
ZFHX4 is one of the DNA repair pathway genes, and somatic mutations in this gene are associated with poor survival in esophageal squamous cell carcinoma and multiple myeloma (38,39). Moreover, ZFHX4 encodes a zinc finger protein and is associated with the maintenance of tumor-initiating cells in glioblastoma (40). However, the role of this gene in CRC is not clear. Based on the derivation of GFAO_Score, patients with overexpression of ZFHX4 are more likely to be stratified into the high-risk group. It will be a valuable research direction to explore the relationship between ZFHX4 mutation and the prognosis of CRC. Besides, the aquaporins are a family of small membrane transport proteins, and AQP8 functions as water-selective transporters (41). The dysregulation of AQP8 has been proven to be involved in tumorigenesis. For example, AQP8 increases viability, inhibits apoptosis, and facilitates metastasis in cervical cancer cells (42). In CRC, AQP8 represses progression by regulating PI3K/AKT signaling and PCDH7 expression (43). However, the association between AQP8 expression and FA metabolism pathways in CRC remains unexplored.
Although the remarkable prognosis and prediction of GFAO_Score in CRC have been evaluated in this study, some limitations remain to be addressed. First, because the datasets were collected from online retrospective public databases, it is anticipated that large prospective clinical studies and experiments in vivo and in vitro should be validated. Second, real immunotherapy and target therapy cohorts were recruited by the study, which limited its efficacy in prediction. The subsequent downstream mechanism of FAO needs to be explored at cellular or subcellular levels to clarify the underlying bio function of AKR1B10.
Conclusions
We explored the predictive efficacy and prognostic performance of the clustering related to FAO metabolism. Using the bulk RNA and scRNA sequencing analysis, a promising prognostic signature was constructed, the GFAO_Score, based on three determined DEGs related to the enrichment of FAO, which showed good evaluation of survival outcomes and adjuvant therapies. Our research provided a new angle to connect the inner interaction of lipid metabolism and tumor progression, serving as a tool for precise screening and strategy-making in chemotherapy and immunotherapy in patients with CRC when transferred to actual application in the future.
Acknowledgments
The authors gratefully acknowledge the data shared in TCGA, and GEO projects with compelling appreciation for the researchers.
Funding: None.
Footnote
Reporting Checklist: The authors have completed the TRIPOD reporting checklist. Available at https://jgo.amegroups.com/article/view/10.21037/jgo-23-833/rc
Peer Review File: Available at https://jgo.amegroups.com/article/view/10.21037/jgo-23-833/prf
Conflicts of Interest: All authors have completed the ICMJE uniform disclosure form (available at https://jgo.amegroups.com/article/view/10.21037/jgo-23-833/coif). The authors have no conflicts of interest to declare.
Ethical Statement: The authors are accountable for all aspects of the work in ensuring that questions related to the accuracy or integrity of any part of the work are appropriately investigated and resolved. Ethics approval is not required since the data of transcriptome expression in this study were accessed from online available databases. The study was conducted in accordance with the Declaration of Helsinki (as revised in 2013).
Open Access Statement: This is an Open Access article distributed in accordance with the Creative Commons Attribution-NonCommercial-NoDerivs 4.0 International License (CC BY-NC-ND 4.0), which permits the non-commercial replication and distribution of the article with the strict proviso that no changes or edits are made and the original work is properly cited (including links to both the formal publication through the relevant DOI and the license). See: https://creativecommons.org/licenses/by-nc-nd/4.0/.
References
- Siegel RL, Miller KD, Goding Sauer A, et al. Colorectal cancer statistics, 2020. CA Cancer J Clin 2020;70:145-64. [Crossref] [PubMed]
- Miller KD, Nogueira L, Devasia T, et al. Cancer treatment and survivorship statistics, 2022. CA Cancer J Clin 2022;72:409-36. [Crossref] [PubMed]
- Dekker E, Tanis PJ, Vleugels JLA, et al. Colorectal cancer. Lancet 2019;394:1467-80. [Crossref] [PubMed]
- Vogelstein B, Fearon ER, Hamilton SR, et al. Genetic alterations during colorectal-tumor development. N Engl J Med 1988;319:525-32. [Crossref] [PubMed]
- Vaupel P, Schmidberger H, Mayer A. The Warburg effect: essential part of metabolic reprogramming and central contributor to cancer progression. Int J Radiat Biol 2019;95:912-9. [Crossref] [PubMed]
- Sun L, Suo C, Li ST, et al. Metabolic reprogramming for cancer cells and their microenvironment: Beyond the Warburg Effect. Biochim Biophys Acta Rev Cancer 2018;1870:51-66. [Crossref] [PubMed]
- Pavlova NN, Thompson CB. The Emerging Hallmarks of Cancer Metabolism. Cell Metab 2016;23:27-47. [Crossref] [PubMed]
- Carracedo A, Cantley LC, Pandolfi PP. Cancer metabolism: fatty acid oxidation in the limelight. Nat Rev Cancer 2013;13:227-32. [Crossref] [PubMed]
- Li Q, Wang Y, Wu S, et al. CircACC1 Regulates Assembly and Activation of AMPK Complex under Metabolic Stress. Cell Metab 2019;30:157-173.e7. [Crossref] [PubMed]
- Bian X, Liu R, Meng Y, et al. Lipid metabolism and cancer. J Exp Med 2021;218:e20201606. [Crossref] [PubMed]
- Houten SM, Wanders RJ. A general introduction to the biochemistry of mitochondrial fatty acid β-oxidation. J Inherit Metab Dis 2010;33:469-77. [Crossref] [PubMed]
- Houten SM, Violante S, Ventura FV, et al. The Biochemistry and Physiology of Mitochondrial Fatty Acid β-Oxidation and Its Genetic Disorders. Annu Rev Physiol 2016;78:23-44. [Crossref] [PubMed]
- Martinez-Outschoorn UE, Peiris-Pagés M, Pestell RG, et al. Cancer metabolism: a therapeutic perspective. Nat Rev Clin Oncol 2017;14:11-31. [Crossref] [PubMed]
- Ma Y, Temkin SM, Hawkridge AM, et al. Fatty acid oxidation: An emerging facet of metabolic transformation in cancer. Cancer Lett 2018;435:92-100. [Crossref] [PubMed]
- Sounni NE, Cimino J, Blacher S, et al. Blocking lipid synthesis overcomes tumor regrowth and metastasis after antiangiogenic therapy withdrawal. Cell Metab 2014;20:280-94. [Crossref] [PubMed]
- Nieman KM, Kenny HA, Penicka CV, et al. Adipocytes promote ovarian cancer metastasis and provide energy for rapid tumor growth. Nat Med 2011;17:1498-503. [Crossref] [PubMed]
- Han J, Qu H, Han M, et al. MSC-induced lncRNA AGAP2-AS1 promotes stemness and trastuzumab resistance through regulating CPT1 expression and fatty acid oxidation in breast cancer. Oncogene 2021;40:833-47. [Crossref] [PubMed]
- He W, Liang B, Wang C, et al. MSC-regulated lncRNA MACC1-AS1 promotes stemness and chemoresistance through fatty acid oxidation in gastric cancer. Oncogene 2019;38:4637-54. [Crossref] [PubMed]
- Stuart T, Butler A, Hoffman P, et al. Comprehensive Integration of Single-Cell Data. Cell 2019;177:1888-902.e21. [Crossref] [PubMed]
- Salazar R, Tabernero J. New approaches but the same flaws in the search for prognostic signatures. Clin Cancer Res 2014;20:2019-22. [Crossref] [PubMed]
- Zhu J, Thompson CB. Metabolic regulation of cell growth and proliferation. Nat Rev Mol Cell Biol 2019;20:436-50. [Crossref] [PubMed]
- De Rosa A, Pellegatta S, Rossi M, et al. A radial glia gene marker, fatty acid binding protein 7 (FABP7), is involved in proliferation and invasion of glioblastoma cells. PLoS One 2012;7:e52113. [Crossref] [PubMed]
- Calle EE, Rodriguez C, Walker-Thurmond K, et al. Overweight, obesity, and mortality from cancer in a prospectively studied cohort of U.S. adults. N Engl J Med 2003;348:1625-38. [Crossref] [PubMed]
- Snaebjornsson MT, Janaki-Raman S, Schulze A. Greasing the Wheels of the Cancer Machine: The Role of Lipid Metabolism in Cancer. Cell Metab 2020;31:62-76. [Crossref] [PubMed]
- Marien E, Meister M, Muley T, et al. Phospholipid profiling identifies acyl chain elongation as a ubiquitous trait and potential target for the treatment of lung squamous cell carcinoma. Oncotarget 2016;7:12582-97. [Crossref] [PubMed]
- Park J, Morley TS, Kim M, et al. Obesity and cancer--mechanisms underlying tumour progression and recurrence. Nat Rev Endocrinol. 2014;10:455-65. [Crossref] [PubMed]
- Balaban S, Lee LS, Schreuder M, et al. Obesity and cancer progression: is there a role of fatty acid metabolism? Biomed Res Int 2015;2015:274585. [Crossref] [PubMed]
- Avery CL, Howard AG, Nichols HB. Comparison of 20-Year Obesity-Associated Cancer Mortality Trends With Heart Disease Mortality Trends in the US. JAMA Netw Open 2021;4:e218356. [Crossref] [PubMed]
- Wang YY, Attané C, Milhas D, et al. Mammary adipocytes stimulate breast cancer invasion through metabolic remodeling of tumor cells. JCI Insight 2017;2:e87489. [Crossref] [PubMed]
- Lazar I, Clement E, Dauvillier S, et al. Adipocyte Exosomes Promote Melanoma Aggressiveness through Fatty Acid Oxidation: A Novel Mechanism Linking Obesity and Cancer. Cancer Res 2016;76:4051-7. [Crossref] [PubMed]
- Jiang L, Xiao L, Sugiura H, et al. Metabolic reprogramming during TGFβ1-induced epithelial-to-mesenchymal transition. Oncogene 2015;34:3908-16. [Crossref] [PubMed]
- Dai W, Xiang W, Han L, et al. PTPRO represses colorectal cancer tumorigenesis and progression by reprogramming fatty acid metabolism. Cancer Commun (Lond) 2022;42:848-67. [Crossref] [PubMed]
- Tafesh-Edwards G, Eleftherianos I. The role of Drosophila microbiota in gut homeostasis and immunity. Gut Microbes 2023;15:2208503. [Crossref] [PubMed]
- Zipper L, Batchu S, Kaya NH, et al. The MicroRNA miR-277 Controls Physiology and Pathology of the Adult Drosophila Midgut by Regulating the Expression of Fatty Acid β-Oxidation-Related Genes in Intestinal Stem Cells. Metabolites 2022;12:315. [Crossref] [PubMed]
- Cotte AK, Aires V, Fredon M, et al. Lysophosphatidylcholine acyltransferase 2-mediated lipid droplet production supports colorectal cancer chemoresistance. Nat Commun 2018;9:322. [Crossref] [PubMed]
- Ma J, Luo DX, Huang C, et al. AKR1B10 overexpression in breast cancer: association with tumor size, lymph node metastasis and patient survival and its potential as a novel serum marker. Int J Cancer 2012;131:E862-71. [Crossref] [PubMed]
- Li J, Guo Y, Duan L, et al. AKR1B10 promotes breast cancer cell migration and invasion via activation of ERK signaling. Oncotarget 2017;8:33694-703. [Crossref] [PubMed]
- Qing T, Zhu S, Suo C, et al. Somatic mutations in ZFHX4 gene are associated with poor overall survival of Chinese esophageal squamous cell carcinoma patients. Sci Rep 2017;7:4951. [Crossref] [PubMed]
- Pawlyn C, Davies FE. Toward personalized treatment in multiple myeloma based on molecular characteristics. Blood 2019;133:660-75. [Crossref] [PubMed]
- Chudnovsky Y, Kim D, Zheng S, et al. ZFHX4 interacts with the NuRD core member CHD4 and regulates the glioblastoma tumor-initiating cell state. Cell Rep 2014;6:313-24. [Crossref] [PubMed]
- Walz T, Fujiyoshi Y, Engel A. The AQP structure and functional implications. Handb Exp Pharmacol 2009;31-56. [PubMed]
- Li W, Song Y, Pan C, et al. Aquaporin-8 is a novel marker for progression of human cervical cancer cells. Cancer Biomark 2021;32:391-400. [Crossref] [PubMed]
- Wu Q, Yang ZF, Wang KJ, et al. AQP8 inhibits colorectal cancer growth and metastasis by down-regulating PI3K/AKT signaling and PCDH7 expression. Am J Cancer Res 2018;8:266-79. [PubMed]