The prognostic value and potential immunotherapeutic efficacy of ACVR1 in treating gastric cancer
Highlight box
Key findings
• The prognostic value and potential immunotherapeutic efficacy of activin A receptor type-1 (ACVR1) in treating gastric cancer.
What is known and what is new?
• Existing studies have shown that ACVR1 is highly expressed in a variety of cancers (including gastric cancer).
• We study the relationship among the high expression of ACVR1 in gastric cancer, immunity and prognostic value.
What is the implication, and what should change now?
• The results showed that high ACVR1 expression may promote gastric cancer development by suppressing immunity due to the enrichment of related cancer-promoting stromal pathways, high infiltration of inhibitory immune cells, high immune escape, and low tumor mutation burden, low microsatellite instability, and TNN mutation rates. Moreover, the immunotherapy benefit was less in the ACVR1 high-expression group.
• The limitation of this study was that experimental validation was insufficient, and in vivo and in vitro experiments are needed to fully explore the molecular mechanism underlying the relationship between ACVR1 and immunity and the effect of drug therapy on gastric cancer.
Introduction
Gastric cancer is the fourth leading cause of cancer death worldwide and is closely associated with infection (1). The discovery and treatment of Helicobacter pylori have reduced the incidence of gastric cancer. However, genetic risk factors, familial syndromes, environmental factors (2), delayed diagnosis, and lack of effective treatments (3) contribute to the prognosis of gastric cancer. Early symptoms of gastric cancer are usually not obvious; therefore, it is typically detected at an advanced stage. Despite advances in multimodal treatment such as surgery, radiotherapy, and chemotherapy, gastric cancer remains an urgent challenge in malignant tumors (4). The unique pathogenesis and active oncogenic pathways made gastric cancer hard to cure. In recent years, immunotherapy has gradually emerged, providing a new modality for gastric cancer therapy (5). A study has verified that the clinical features of gastric cancer are associated with infiltrating tumor microenvironment (TME) cells, in which the suppression of T cells causes a reduction in the ability to mediate tumor killing and may lead to a poor prognosis (6).
TME includes immune cells, stromal cells and tumor cells. TME enhances tumor cell proliferation, migration ability, and immune escape, promoting tumor development. As the instability of the cancer cell genome predisposes increases the likelihood of drug resistance, targeting nontumor cells in the genetically relatively stable TME has significant advantages. Some patients have been shown to benefit from immunotherapy (7). Therefore, we further analyze the role of activin A receptor type-1 (ACVR1) in TME.
Programmed cell death protein 1 (PD-1)/programmed cell death ligand-1 (PD-L1), two of the biomarkers of gastric cancer, represent an immune checkpoint molecule involved in immunotherapy (8). PD-1 binds to PD-L1 on the surface of tumor cells and inhibits T-cell activation and proliferation, thus, facilitating immune escape (9). Other biomarkers of gastric cancer, including high microsatellite instability (MSI-H)/defective mismatch repair (dMMR), Epstein-Barr virus (EBV) positivity, and high tumor mutation burden (TMB), have also been strongly connected with immunotherapy (10,11). Immune checkpoint inhibitors for gastric cancer, such as sintilimab, pembrolizumab, and nivolumab, have been used in the clinic to benefit patients to a degree but are not sufficient to respond to individual heterogeneity. Moreover, The Cancer Genome Atlas (TCGA) data have confirmed that there is no single target that controls the progression of all gastric cancers. Therefore, it is critical to identify additional biomarkers to better characterize the relationship between gastric cancer and immunotherapy.
Transforming growth factor β (TGF-β) is a ligand family of structurally related pleiotropic cytokines (12) that control cell proliferation, differentiation, migration, and death, whose overexpression leads to extracellular matrix (ECM) deposition, epithelial–mesenchymal transition (EMT), and cancer-associated fibroblast (CAF) formation. These processes are inextricably linked to fibrotic disease and cancer development (13). ACVR1, which has a GS structural domain, a glycine serine–rich juxtamembrane region, and a kinase structural domain, is a type I bone morphogenetic protein (BMP) receptor that encodes TGF-β (14). ACVR1 binds to other TGF-β receptors that together mediate SMAD signaling and is involved in bone growth, retinal angiogenesis, and hepatic cell surface ferroportin production, as well as biological processes such as sweat gland cell proliferation, germ cell genesis, cardiovascular generation, neuropathic pain, and blockade of glial cell differentiation (15-22). ACVR1 has been extensively studied in fibrodysplasia ossificans progressive (FOP) and diffuse endogenous pontine glioma (DIPG), which are two rare diseases (23). In addition, its function is being investigated in other cancer types, including multiple myeloma, breast cancer, endometrial cancer, and polycystic ovary syndrome (24-27). Overexpression of ACVR1 promotes cell proliferation in cancer cells (28), and ACVR1 activates the Wnt/β-linker protein signaling pathway in breast cancer (25) and induces an imbalance in the TGF-β1/BMP-7 pathway in hepatocellular carcinoma (HCC) cells, thereby promoting HCC cell invasion and stemness (29). Increased ACVR1 expression has been shown to lead to increased and prolonged immune infiltration (30). However, to date, the biological functions of ACVR1-related to gastric cancer immunity have not been elucidated.
In this study, through bioinformatics and experimental validation, we provide evidence that ACVR1 expression is upregulated in gastric cancer and is associated with poor prognosis for patients and that aberrant expression of ACVR1 is not only associated with the clinical features, prognosis, TME, immune checkpoints, antitumor drug susceptibility, TMB, MSI, and DNA mismatch repair (MMR) but also participates in the promotion of malignant gastric cancer progression. We present this article in accordance with the REMARK reporting checklist (available at https://jgo.amegroups.com/article/view/10.21037/jgo-23-984/rc).
Methods
Data collection and process
Downloaded pancancer samples were collected from the UCSC Xena database (xenabrowser.net). RNA sequencing data involving 379 gastric cancer tissues and 34 normal tissues as well as the clinical data of 407 cases were obtained from TCGA (https://portal.gdc.cancer.gov/).
Immunohistochemistry (IHC) data were obtained from 212 postoperative patients diagnosed with gastric cancer in Affiliated Hospital of Nantong University from 2010 to 2011. The clinical information including survival status, survival time, date of surgery, tumor-node-metastasis (TNM) stage, tumor stage, grading, age, and gender. The study was conducted in accordance with the Declaration of Helsinki (as revised in 2013). The study was approved by the ethics board of Affiliated Hospital of Nantong University (No. 2023-L067) and informed consent was taken from all the patients. After removal of irrelevant data from the above-described set, the data were analyzed with software and visualized using the “limma” and “ggplot2” packages in R software (The Foundation of Statistical Computing), in which TCGA transcriptome data were converted to transcripts per million (TPM) format for subsequent analysis.
Immunohistochemistry (IHC) staining and scoring
The specific steps for postoperative specimens were as follows:
- Treatment of coverslips and slides: slides and coverslips were soaked in an acid vat for 24 h, rinsed with tap water, rinsed at least 3 times with ddH2O (laboratory-grade water), soaked in 95% alcohol for 24 h, and then baked in an electric thermostatic drying oven and stored for reserve.
- Slicing and baking: tissues were embedded in paraffin, and a tissue slicer was used to make consecutive slices with a thickness of 5 µm, which were affixed to APES-treated slides. The slices were baked in a 60 °C electric thermostatic oven for 6–8 h. The slices were then dried in a thermostatic oven at 60 °C.
- Deparaffinization and hydration of tissue sections: the tissue sections were soaked in xylene for 15 min, placed in fresh xylene, soaked again for 15 min, and then rinsed in phosphate-buffered saline (PBS) for 5 min 3 times, anhydrous ethanol for 10 min 2 times, 95% ethanol for 5 min 2 times, 80% ethanol for 5 min, and 70% ethanol for 5 min.
- Antigen repair: first, a prepared ethylenediaminetetraacetic acid (EDTA) repair solution with a PH of 9.0 was boiled in an autoclave, and the slides were placed in the autoclave, covered with stainless steel lid, and heated with a jet at 300 W for 18 min. Running water was used to make the repair solution naturally cool down to room temperature, which was followed by rinsing with PBS for 5 min 3 times.
- Blocking endogenous peroxidase: a circle was drawn with an IHC pen at the edge of the tissue, 100 µL of 3% H2O2 was added to each section, and incubation was conducted for 15 min at room temperature, which was followed by a rinsing with PBS for 5 min 3 times.
- Closure: slides were gently shaken dry of moisture (blotted dry of surrounding liquid), the specimen was placed in an incubation box, and the tissue was closed with PBS containing 5% bovine serum albumin (BSA) and 0.3% Triton X for 2 h at room temperature.
- Primary antibody: the ACVR1 antibody was diluted at a ratio of 1:300, the diluted antibody was drooped into the circled tissues, incubated for 1 h at room temperature, and rinsed with PBS for 5 min × 3 times.
- Color development: prepared Diaminobutane (DAB) (1:20) was added dropwise onto the tissue for 5 min. The degree of color development was observed under a microscope, and when the reaction was completed, the tissue was rinsed with tap water for 5 min.
- Lining staining: hematoxylin restaining conducted the tissue for 10–20 s. Then rinsed the tissue with running water, and hydrochloric acid–ethanol differentiation was performed for 2–3 s, which was followed by a rinse with running water for 5 min.
- Dehydration: 70% ethanol, 80% ethanol, 95% ethanol, and anhydrous ethanol were used to deal with the tissue in order, then xylene was used to deal with it.
- Sealing: tissue sections were sealed with neutral resin to ensure no air bubbles were produced.
For each section, two experienced pathologists randomly selected ten high-magnification fields of view under the microscope. They counted 100 cells in each field of view to calculate the ratio of the number of positive cells. Scoring was based on the percentage of positive cells, with <5% being 0, 5–25% being 1, 26–50% being 2, 51–75% being 3, and >75% being 4. Scoring was based on staining intensity, with no or light staining being 0, moderate staining being 1, and deep staining being 2. The product of the 2 scores was used as the semiquantitative immunohistochemical score.
Survival analysis
After deleting cases with missing data of downloaded TCGA samples, we calculated the median expression of ACVR1. We then categorized the samples into high- and low-expression groups and examined the survival status, survival time, and the survival curve using Kaplan-Meier analysis. And the IHC data were processed to classify the expression of ACVR1 into high- and low-expression groups based on the median value and cutoff value of the semiquantitative immunohistochemical score. The data were analyzed using the “survival” and “survminer” packages in R software (R version 4.3.0) for prognostic analysis. A P value of less than 0.05 was considered to indicate a statistically significant difference between the 2 groups.
Comprehensive analysis of clinicopathologic characteristics
The downloaded clinical data of TCGA were used to draw heatmaps of clinical traits via the “complexHeatmap” R package; meanwhile, the correlation between clinical characteristics and ACVR1 was plotted using the “ggpubr” R package. The “ggpubr” R package was also used with IHC data to plot the correlation of clinical features with ACVR1. TCGA data were then analyzed using univariate Cox and multifactorial Cox to explore the relationship between clinical traits and ACVR1 expression, while multifactorial Cox was used to examine whether ACVR1 could be used as an independent prognostic indicator for gastric cancer. A P value less than 0.05 was considered to indicate a statistically significant difference between two groups. Based on the results of the prognostic analysis, column line graphs were constructed to assess the survival rates at 1, 3, and 5 years, after which the reliability of the results of the column line graphs was assessed using calibration curves and receiver operating characteristic (ROC) curves.
Biofunctional analysis
ACVR1 coexpressed genes were analyzed with the “ggExtra” R package, and a correlation network diagram was plotted to show the correlation between genes. Additionally, a correlation heat map was plotted using the “corrplot” R package. Gene set enrichment analysis (GSEA) of Kyoto Encyclopedia of Genes and Genomes (KEGG) was conducted for the coexpressed genes, and the results were plotted as bar charts via the “ggplot2” and “clusterProfiler” R packages, and the petal plots in “ggraph” R package were used to show the specific genes related to the pathways. Single-sample GSEA (ssGSEA) was performed using the “Hallmark” gene set to analyze the enrichment of relevant pathways in the ACVR1 high- and low-expression groups, and the results were visualized using the “GSVA”, “GSEABase”, “GSVA”, “GSEABase”, “GSVA”, and “GSEABase” R packages. The results were visualized using “GSVA” and “GSEABase” R packages, and pathways with a P value less than 0.05 were considered to be significantly enriched.
TME analysis
ACVR1-associated immune cells, stromal cell infiltration, and immune function were analyzed by the ESTIMATE algorithm, the CIBERSORT algorithm, the ssGSEA algorithm and Spearman correlation analysis. The results were visualized using R package “ggplot2”. Single-cell data from the Tumor Immune Single-cell Hub 2 (TISCH2) online website were used to analyze immune cell infiltration. Immunotherapy effects were predicted using the tumor immune dysfunction and exclusion (TIDE) score and the IMvigor210 immune cohort. A P value <0.05 was considered statistically significant.
Immune checkpoint and antitumor drug analysis
Differences between ACVR1 and common immune checkpoints were determined using the “ggplot2” package. Immune checkpoint correlation heatmaps and correlation plots of 4 specific common immune checkpoints with ACVR1 were drawn. Online data from The Cancer Imaging Archive (TCIA) were then scored to predict the outcome of anti-immune checkpoint therapy, and violin plots were created using the “ggpubr” R package. Subsequently, we analyzed the correlation between 4 common antitumor drugs and ACVR1. Differences in the 50% reduction in growth concentration (IC50) of chemotherapeutic agents between the ACVR1 high- and low-expression groups were estimated using the “pRRophetic” package. P values less than 0.05 were considered to be statistically significant.
TMB analysis
TMB, which reflects the number of cancer mutations, is defined as the number of somatic mutations per megabase (31). TMB ≥10 mutations per megabase (mut/Mb) is considered to be a high TMB (TMB-H). TMB mutations are processed into neoantigens and presented to T cells via major histocompatibility complex (MHC) proteins. A higher TMB leads to more neoantigens, increases the chances of T-cell recognition, and is clinically associated with better immune checkpoint blockade treatment outcomes (32).
MSI and MMR analysis
MSI can be categorized into 3 states: MSI-H, low microsatellite instability (MSI-L), and microsatellite stable (MSS), while MMR can be categorized into dMMR and proficient MMR (pMMR). In addition, dMMR is equivalent to MSI-H, while pMMR is equivalent to MSI-L or MSS. Human mismatch repair genes (MMR genes) are transcribed and translated to convey the corresponding mismatch repair proteins. A malfunction in the expression of the MMR proteins (including MLH1, MSH2, MSH6, and PMS2) can result in base mismatches during DNA replication, leading to the loss of repair, with dMMR leading to MSI (33).
Statistical analysis
All statistical analyses and graphs were completed with R version 4.3.0. The Wilcoxon rank-sum test and t-test were used to compare numerical differences between ACVR1 high- and low-expression groups, while the log-rank test was conducted to assess differences in survival. Kaplan-Meier survival analysis and the log-rank test were used to evaluate survival distributions. Univariate Cox and multivariate Cox regression analyses were performed to assess the hazard ratios (HRs) and 95% confidence intervals (CIs) for different clinical characteristics and to identify independent prognostic factors. P<0.05 was considered statistically significant.
Results
ACVR1 was highly expressed in multiple cancers and showed differential expression in gastric cancer
The Xena database showed that ACVR1 expression was upregulated in cholangio carcinoma (CHOL), colon adenocarcinoma (COAD), esophageal carcinoma (ESCA), head and neck squamous cell carcinoma (HNSC), kidney renal papillary cell carcinoma (KIRP), lung adenocarcinoma (LUAD), lung squamous cell carcinoma (LUSC), pheochromocytoma and paraganglioma (PCPG), stomach adenocarcinoma (STAD), and thyroid carcinoma (THCA) (Figure 1A). In gastric cancer, TMB and MSI are closely related to the high ACVR1 expression (Figure 1B,1C). The TCGA database showed that in unpaired difference analysis, the expression of ACVR1 was higher in gastric cancer than in normal paracancerous tissues (Figure 1D). In paired differential analysis, the expression level of ACVR1 was also high in the gastric cancer (Figure 1E). The IHC data indicated that the expression of ACVR1 was higher in gastric cancer tissues than in normal tissues (Figure 1F,1G). Above analysis results indicate that ACVR1 was higher in gastric cancer than in normal paracancerous tissues. It may be a procancer factor related to the poor prognosis.
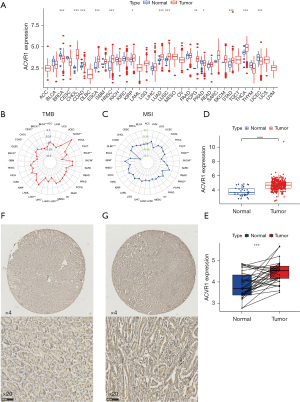
Correlation of ACVR1 with survival prognosis and clinicopathologic features of gastric cancer
The results of previous studies and studies suggest that the high expression of ACVR1 may indicate a poor prognosis in gastric cancer (34,35). In the subsequent survival curves, the difference between high and low ACVR1 expression was noticeable, and the prognosis of the high-expression group was poorer than that of the low-expression group (Figure 2A).
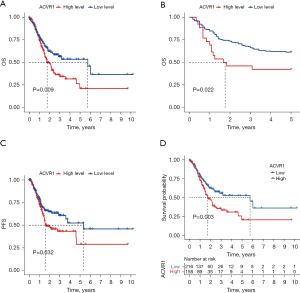
Similarly, the poor prognosis of the ACVR1 high-expression group was again verified by the results of IHC data in Affiliated Hospital of Nantong University (Figure 2B). According to the TCGA data, we found that patients in the ACVR1 high-expression group had a shorter progression-free survival (PFS) time (Figure 2C). We again used the cutoff value of the ACVR1 expression of TCGA data to differentiate between the high- and low-expression groups. The low-expression group has a poorer predicted prognosis (Figure 2D). The chi-squared test was used to assess the clinicopathological characteristics of TCGA data. The results showed that ACVR1 expression was significantly correlated with tumor grade and tumor stage (Figure 3A). According to Spearman analysis, there was a significant difference in the expression of ACVR1 in early (stage I) and advanced (stage III) gastric cancer (Figure 3B). The expression of ACVR1 gradually increased with the increase of tumor T stage (Figure 3C). In the IHC data, the expression of ACVR1 was increased in the advanced stage (stage III) of gastric cancer (Figure 3D). The high expression of ACVR1 was in patients with poorly differentiated gastric cancer (Figure 3E).
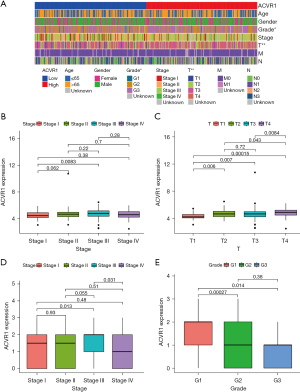
Construction of column line graphs
To further investigate the relationship between ACVR1 and overall survival (OS), we performed univariate Cox and multivariate Cox analyses on TCGA data. Univariate Cox analysis showed that a high expression of ACVR1, T2–4N1–3M1 stage, stage III, IV and old age (>65 years) were all associated with OS (P<0.05) (Figure 4A). Multivariate Cox analysis showed that the high expression of ACVR1 and old age (>65 years) operated as independent prognostic factors (P<0.05) (Figure 4B). Column line plot was constructed for predicting the 1-, 3-, and 5-year survival rates based on TNM stage, pathologic grade, gender, stage, age, and ACVR1 expression, with the results being 0.894, 0.699, and 0.642, respectively (Figure 4C). The calibration curve showed that the actual OS values were more consistent with the predicted OS consequences (Figure 4D). The area under the ROC curve for 1-, 3-, and 5-year survival rates of the forecast OS column line graphs were 0.527, 0.583, and 0.627, respectively, which, taken together, indicated a more satisfactory prediction (Figure 4E).
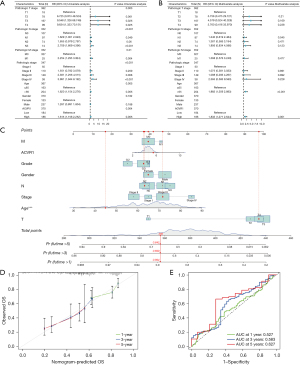
Coexpression analysis and enrichment analysis of ACVR1
We performed a coexpression analysis of ACVR1. Figure 5A shows the network diagram of the top 5 genes most positively and negatively correlated with ACVR1, respectively. Figure 5B shows the correlation heatmap of the top 10 genes most positively and negatively associated with ACVR1. Both images indicate that the extracted coexpressed genes were significantly correlated with ACVR1. To further clarify the underlying mechanisms, we used GSEA of the coexpressed genes of ACVR1 in the KEGG dataset. As can be seen in Figure 5C, these coexpressed genes were significantly correlated with ACVR1 in the areas of leukocyte transendothelial migration, neutrophil extracellular trap formation, PD-L1 expression and PD-1 checkpoint pathway in cancer, B-cell receptor signaling pathway, T helper (Th) 17 cell differentiation, Th1 and Th2 cell differentiation, NK cell–mediated cytotoxicity, T-cell receptor signaling pathway, chemokine signaling pathway, platelet activation, and Toll-like receptor signaling pathway. There was significant enrichment in these immune-related pathways, which may contribute to the development of gastric cancer via the regulation of immune-related functions or immune cells. In addition to the above pathways, these genes were also enriched in nuclear factor (NF)-κB signaling pathway, Hedgehog signaling pathway, focal adhesion, ECM–receptor interaction, Hippo signaling pathway, mTOR signaling pathway, Wnt signaling pathway, TGF-β signaling pathway, JAK-STAT signaling pathway, and cytokine–cytokine receptor interaction, among others, which are involved in cell proliferation, differentiation, apoptosis, migration, and death. ACVR1 is also enriched in metabolism-related pathways such as animal autophagy, phagosome, and lysosome, as well as hypoxia-inducible factor 1 (HIF-1) signaling pathway, which is a hypoxia-related pathway. Figure 5D shows all the genes enriched in the T-cell-related pathways.
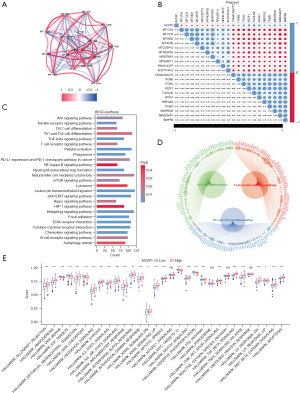
Next, we performed ssGSEA using the “Hallmark” gene set, and as shown in Figure 5E, the high expression group was associated with allograft rejection, angiogenesis, EMT, Hedgehog transition, Hedgehog signaling, hypoxia, IL2-STAT5 signaling, IL6-JAK-STAT3 signaling, inflammatory response, interferon α (IFN-α) response, IFN-γ response, KRAS signaling down-regulation, KRAS signaling up-regulation, mitotic spindle, Notch signaling, P53 pathway, PIK3-AKT-mTOR signaling, TGF-β signaling, TNF-α signaling via NF-κB, ultraviolet response downregulation, ultraviolet response upregulation, Wnt-β catenin signaling, and apoptosis. These are all significantly associated with these stroma-associated pathways of oncogenic activity. Meanwhile, the low-expression group was enriched considerably in DNA repair, E2F targets, MYC target V1, G2M checkpoint, and oxidative phosphorylation, which are cell cycle event–related pathways.
Analysis of the correlation between ACVR1 and TME
Based on TCGA database, we used the ESTIMATE algorithm to produce scores for immune cells, stromal cells, and the availability of immune and stromal cells in the TME. The results showed that the StromalScore, ImmuneScore, and ESTIMATEScore of the high ACVR1 expression group were higher than those of the low-expression group (Figure 6A).
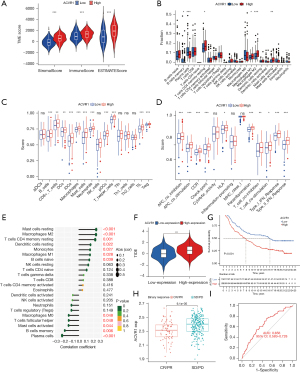
We used the CIBERSORT algorithm to assess the proportion of immune cell infiltration in the ACVR1 high- and low-expression groups. The results showed that the scores of memory B cells and plasma cells were higher in the ACVR1 low-expression group than in the high-expression group. While the scores of resting memory CD4 T cells, M1 macrophages, M2 macrophages, and resting mast cells were higher in the ACVR1 high-expression group than in the low-expression group (Figure 6B).
In addition, we used the ssGSEA algorithm to assess immune cell infiltration in samples of the ACVR1 high- and low-expression groups. We found that B cells, CD8+ T cells, dendritic cells (DCs), interstitial DCs (iDCs), macrophages, mast cells, neutrophils, plasmacytoid DCs (pDCs), Th cells, tumor-infiltrating lymphocytes (TILs), and T regulatory cells (Tregs) had a higher degree of infiltration in the ACVR1 high-expression group than in the low-expression group (Figure 6C). With the upregulation of ACVR1 expression, the immune functions of antigen-presenting cell (APC) coinhibition, APC costimulation, CCR, check-point, human leukocyte antigen (HLA), parainflammation, T-cell coinhibition, T-cell costimulation, type I IFN response, AND type II IFN response were enhanced (Figure 6D). We subsequently investigated the relationship between infiltrating immune cells and ACVR1 expression between the high- and low-expression groups using Spearman correlation analysis. As shown in Figure 6E, high ACVR1 expression was positively correlated with resting mast cells, M2 macrophages, resting memory CD4 T cells, resting DCs, monocytes, M1 macrophages, and memory B cells. However, high ACVR1 expression was negatively correlated with M0 macrophages, follicular Th cells, activated mast cells, memory B cells, and plasma cells.
We also performed TIDE immune escape scoring and assessed complete response (CR)/partial response (PR) and stable disease (SD)/progressive disease (PD) in patients with high ACVR1 expression using the IMvigor210 immune cohort. We used TIDE to identify two mechanisms of tumor immune escape: induction of T-cell dysfunction in tumors with high abundance of cytotoxic T lymphocytes (CTLs) and prevention of T-cell infiltration. It was found that immune escape was more likely to occur in the ACVR1 high-expression group (Figure 6F). Moreover, in the IMvigor210 immunization cohort, the prognosis was worse in the high-expression group than in the low-expression group (Figure 6G). Furthermore, ACVR1 expression was lower in the CR/PR group (Figure 6H), and in the ACVR1 low-expression group, the ROC curve (Figure 6I) indicated that the accuracy of predicting immunotherapy in this IMvigor210 immunization cohort sample was relatively satisfactory. Taken together, these results suggest that high ACVR1 expression may lead to increased infiltration of immunosuppressive cells over antitumor immune cells in the TME, thus promoting gastric cancer progression and resistance to immunotherapy.
Validation of ACVR1 immune infiltration at the single-cell level
We performed single-cell RNA sequencing analysis of ACVR1 based on the gastric cancer database in the TISCH2 online website to explore the expression and distribution of ACVR1 in TME. Two datasets, GSE134520 and GSE167297, were analyzed, revealing that ACVR1 was significantly overexpressed in CD8 T cells, plasma cells, DCs, and mast cells (Figure 7A). Based on the results of GSE167297 data analysis, it was found that ACVR1 was overexpressed and distributed in plasma cells, mast cells, CD8 T cells, DCs, malignant cells, gland mucous cells, and pit mucous cells (Figure 7B,7C). ACVR1 was also overexpressed and distributed in B cells, plasma cells, mast cells, CD8 T cells, DCs, monophages and macrophages, endothelial cells, and epithelial cells (Figure 7D,7E).
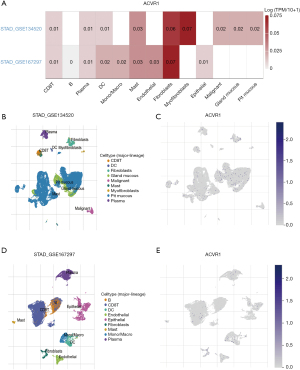
Analysis of ACVR1 with immune checkpoints and antitumor drugs
Immune checkpoint blockers were associated higher survival rates compared to conventional antitumor drugs. Combining immunotherapy and chemotherapy can improve the immune status in TME and thus enhance the antitumor immune response (36). To further predict the response to immunotherapy in high ACVR1 expression patients with gastric cancer, we analyzed the ACVR1-related immune checkpoints, which showed that most of the immune checkpoints were more highly expressed in the ACVR1 high-expression group (Figure 8A). According to Spearman correlation analysis, it was concluded that most of the immune checkpoints were positively regulated with ACVR1. Therefore, patients with high ACVR1 expression experienced better therapeutic efficacy when treated with immune checkpoint inhibitors (Figure 8B). We analyzed PD-1, PD-L1, PD-L2, and CTLA4 among the common immune checkpoints. They were more significantly expressed in the ACVR1–high expression group (Figure 8C-8F). We predicted the therapeutic effects of two immune checkpoint inhibitors, PD-1 and CTLA4. The results showed that the blocking effect of these two immune checkpoints was poor in patients in the ACVR1–high expression group both for the negative or positive expression of PD-1 and CTLA4 (Figure 8G-8J). However, the blocking effect of the other immune checkpoint inhibitors still needs to be investigated. In addition, we also analyzed ACVR1-related drug treatments, and the results showed that the IC50 of four common antitumor drugs, paclitaxel, erlotinib, lapatinib, and sunitinib, were significantly reduced in the ACVR1-high expression group (Figure 8K-8N). In conclusion, although immunotherapy alone is ineffective, combining it with other antitumor drugs may produce promising therapeutic effects. Further investigation into ACVR1 may reveal new strategies for the treatment of gastric cancer.
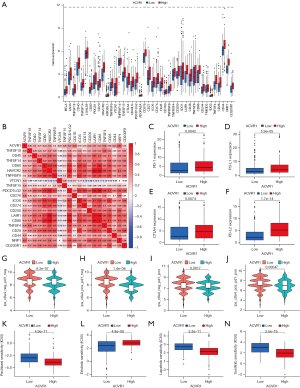
Analysis of the correlation between ACVR1 and TMB
Patients with higher TMB have more prolonged survival after immune checkpoint inhibitor (PD-1/PD-L1) treatment (37). We analyzed the TMB and mutation frequency of ACVR1. The results showed that patients in the ACVR1 high-expression group had lower TMB levels than the low-expression group (Figure 9A). The expression of ACVR1 was negatively correlated with TMB (Figure 9B). Kaplan-Meier analysis showed that patients with gastric cancer with low TMB levels had a shorter survival period with high TMB (Figure 9C). According to stratified survival analysis, patients with gastric cancer in the high TMB and ACVR1 low-expression group had the best survival prognosis, while patients with gastric cancer in the low TMB and ACVR1 high-expression group had the worst survival prognosis (Figure 9D). Among the somatic mutations of ACVR1, the top 20 mutated genes in the high and low ACVR1 expression groups were the same. However, the frequency of gene mutations in the ACVR1 low-expression group was higher than that in the ACVR1 high-expression group, with the gene with the highest mutation frequency being TTN, followed by TP53 and MUC16 (Figure 9E,9F).
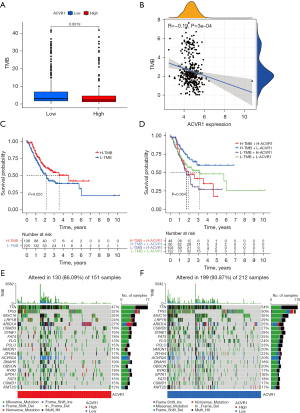
Correlation analysis of ACVR1 with MSI and dMMR
In the comparison of MSS, MSI-L, and MSI-H in the high and low expression of ACVR1, the results showed that the percentage of both MSS and MSI-L was higher in the ACVR1 high-expression group (Figure 10A). Indeed, the differences between MSS, MSI-L, and MSI-H were significant, indicating that the MSI decreased as the expression of ACVR1 increased (Figure 10B). We used Spearman correlation analysis and found that the expression of all 4 MMR proteins (MLH1, MSH2, MSH6, and PMS2) was increased in the ACVR1 high-expression group (Figure 10C-10F).
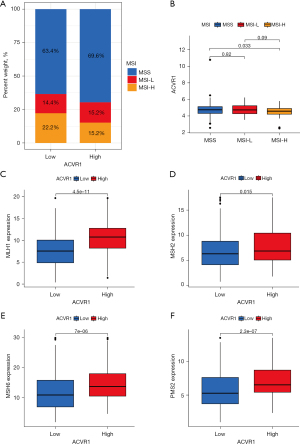
Discussion
Despite a large number of studies being dedicated to eradicating gastric cancer, it remains largely uncured. Some patients receiving improved clinical outcomes and others’ outcomes being unsatisfactory. Therefore, actively exploring biomarkers may help to gain insight into gastric cancer’s molecular mechanisms (38). In this study, the potential biological function of ACVR1 as a novel biomarker for gastric cancer was explored. ACVR1 is highly expressed in various cancers, and this paper focuses on the relationship between ACVR1 and gastric cancer. Compared with normal tissues, gastric cancer tissue showed upregulated ACVR1 expression as demonstrated by the biosignature analysis and IHC data. The high expression of ACVR1 predicted a poor prognosis of gastric cancer correlated with the TME.
ACVR1 was significantly associated with the clinicopathological features of tumor stage and tumor size in gastric cancer. In addition, Kaplan-Meier survival analysis (OS and PFS) of TCGA samples showed that patients with high ACVR1 expression had a worse prognosis in gastric cancer. IHC data in Affiliated Hospital of Nantong University confirmed that patients with high expression of ACVR1 had lower OS than low ACVR1 expression. Univariate Cox, multivariate Cox analysis, and column line graph modeling indicated that high ACVR1 expression could be an independent factor for poor prognosis in gastric cancer. It has been demonstrated that upregulation of ACVR1 expression can promote angiogenesis and lymph node metastasis in gastric cancer (34). Previous in vivo and in vitro experiments have confirmed the role of ACVR1 in the proliferation, invasion, and metastasis of gastric cancer cells, which is closely related to the mechanism of action of H. pylori (35). In summary, a high expression of ACVR1 may be a prognostic biomarker for gastric cancer and promote gastric carcinogenesis.
To further explore the deep mechanism of ACVR1, we identified the coexpressed genes of ACVR1 and performed KEGG enrichment analysis. We found that these genes were significantly enriched in multiple immune-related pathways. The potential mechanism of ACVR1 is that it is involved in regulating the tumor immune microenvironment. Next, the enrichment of immune-related pathways in the ACVR1 high- and low-expression groups was analyzed. And ACVR1 was found to be closely associated with immune cells and stroma. TME in the ACVR1 high-expression group had a higher stroma content as shown by the ESTIMATE and biological analyses. The ACVR1 high-expression group had a higher StromalScore and was significantly enriched in KEGG stroma-associated pathway NF-κB signaling pathway, Hedgehog signaling pathway, focal adhesion, ECM–receptor interaction, Hippo signaling pathway, mTOR signaling pathway, Wnt signaling pathway, TGF-β signaling pathway, JAK-STAT signaling pathway, and cytokine–cytokine receptor interaction. All of them are associated with cell proliferation, differentiation, apoptosis, and migration. Among them, the TGF-β and Wnt signaling pathways promote EMT and tumor development (13). Focal adhesion is an essential step in cell migration (39). Cancer cell proliferation and metastasis depend on a hypoxic environment, and HIF-1 is an adaptive mechanism that arises in response to a hypoxic environment. Cancer cells undergo metabolic reprogramming through the HIF-1 signaling pathway to ensure that the substances required for the growth of cancer cells are provided under hypoxic conditions (40). The ssGSEA in this study showed that the stroma-associated pathway that promotes cancer was enriched in the ACVR1 high-expression group. Therefore, high ACVR1 expression can promote the increase of stromal components in the tumor immune microenvironment, promoting gastric cancer’s invasion and metastasis.
The TME is composed of stromal components and immune cells and their pathways. In the KEGG pathway, neutrophil extracellular trap formation captures circulating cancer cells and enhances the metastatic spread of cancer, in addition to awakening dormant cancer cells and promoting cancer recurrence and metastasis (41). Leukocyte transendothelial migration, PD-L1 expression and PD-1 checkpoint pathway in cancer, B-cell receptor signaling pathway, Th17 cell differentiation, Th1 and Th2 cell differentiation, NK cell–mediated cytotoxicity, T-cell receptor signaling pathway, chemokine signaling pathway, platelet activation, Toll-like receptor signaling pathway, and immune-related pathways immune cells play an essential role in these processes.
The relationship between immune cells and tumors is exceptionally complex, and different immune cells have exerted different functions. On the one hand, immune cells can inhibit gastric cancer. For instance, Tregs are a subpopulation of CD4+ T cells, which secrete immunosuppressive cytokines, granzyme A and granzyme B, to induce apoptosis of T cells, NK cells, NKT cells, APCs, and Th1 cells via cytotoxicity and phagocytosis, but can also activate macrophages, neutrophils, NK cells, and CD8 T cells to fight against tumors. CD8+ T cells are the main effector cells of the antitumor immune response and differentiate into cytotoxic effector T cells after activation. They induce the death of target cells through cytotoxic effects. The direct cytotoxic potential of CD4 T cells can directly kill infected and transformed cells. On the other hand, immune cells can promote gastric cancer progression. For instance, tumor-associated mast cells (TAMCs) are associated with poorer prognosis in patients with colorectal, gastric, or pancreatic ductal adenocarcinoma. In terms of tumor promotion, TAMCs achieve angiogenesis and lymphangiogenesis by secreting angiogenic components and can also suppress T-cell immunity by disrupting antitumor immunity in human gastric cancer through the expression of PD-L1. B cells can activate complement by producing IL-10, which induces protumorigenic activity. B regulatory cells inhibit the immune process by disrupting human gastric cancer’s Th1–Th2 balance. Th2 cells are predominantly associated with protumorigenic activity. Meanwhile, neutrophils and macrophages can be polarized within the TME, and different polarization states exert additional functions. For example, macrophages can be polarized into 2 phenotypes, M1 or M2 macrophages (mostly the M2 type), which can inhibit T-cell and NK-cell functions by inducing the expression of TIM-3, PD-1, and CTLA-4.
On the contrary, M1-type macrophages can enhance antitumor immunity. pDCs have both tumor-promoting and tumor-suppressing effects (42), and IL-2 has been considered a key molecule in promoting T-cell proliferation and differentiation; however, a recent study has shown that IL-2 can induce CD8+ T cell depletion, suppressing antitumor immune responses, thus making IL-2α double-edged sword (43). Combining the results of the immune cell CIBERSORT algorithm, ssGSEA algorithm, and Spearman correlation analysis, we found that tumor-promoting immune cells were more densely infiltrated in the tumor immune microenvironment of the ACVR1 high-expression group. TISCH2 online single-cell data confirmed the increased infiltration abundance of immune cells in gastric cancer with high ACVR1 expression. Prediction of immunotherapy efficacy in high-expression ACVR1 in the IMvigor210 immunotherapy cohort data showed poor treatment efficacy in patients with gastric cancer. The ACVR1 high-expression group had a higher TIDE immune escape score, which predicted that the high-expression group may not benefit from immunotherapy. Cumulatively, the above analysis suggests that high ACVR1 expression may promote the development of gastric cancer by suppressing immunity.
We examined other potential evidence concerning the association of ACVR1 expression with immune checkpoints. Most immune checkpoints had increased expression in the ACVR1 high-risk group and were significantly correlated with ACVR1 expression. Among the immune checkpoint inhibitors, some PD-1 and CTLA-4 inhibitors have been validated for clinical use due to show favorable therapeutic effects (44). However, despite the upregulation of PD-1 and CTLA-4 expression in the high-risk group, predictions of therapeutic efficacy showed better immunotherapy in the low-risk group. PD-1/PD-L1 target inhibitors have proven to be therapeutically effective in a subset of patients, but a significant proportion of patients still do not benefit due to primary or secondary resistance. A study has demonstrated that both aberrant upregulation of PD-L1 expression and deletion of PD-L1 expression may lead to the ineffectiveness of PD-1/PD-L1 target inhibitors. The ineffectiveness of target inhibitors may also be the result of impaired antigen expression, presentation, recognition, activation of T cells, as well as of abnormalities in T cells themselves (45).
Abnormal T-cell immunity includes insufficient T-cell infiltration, dysfunction, and exhaustion, with the latter mostly consisting of CD8+ T cell depletion. Depleted CD8+ T cells (CD8+ Tex) are categorized into PD-1lo and PD-1hi. Immune checkpoint blockade reactivates PD-1lo CD8+ Tex causing CD8+ T cells to expand, thereby promoting antitumor immunity yet leading to the apoptosis of PD-1hi CD8+ Tex. PD-1hi is accompanied by enhanced expression of coinhibitory receptors (including TIM-3, LAG-3, CD160, 2B4, TIGIT, and CTLA-4), yet cells with high expression of coinhibitory receptors cells are more severely depleted (46). Thus, depleted T cells cannot control tumor progression at the late stages due to the presence of immune tolerance and immunosuppressive mechanisms. We further explored common chemotherapeutic and targeted agents in patients with a high expression of ACVR1 and found that the IC50 values were lower in the high-expression group, implying a more pronounced therapeutic benefit in high-expression group. Despite the suboptimal predictive results of immunotherapy, conventional therapies combined with immunotherapy may be more effective. Radiotherapy, chemotherapy, and targeted therapy may improve the immune status, eliminate suppressive immune factors, and even cause distant effects (47). In conclusion, the results suggest that high ACVR1 expression is effective for chemotherapy and targeted therapy and can be combined with immunotherapy.
In recent years, new immunotherapy prognostic biomarkers, such as TMB, MSI, and MMR have attracted extensive research, and some have been applied in clinical practice. Therefore, we explored these biomarkers with ACVR1. The results showed that TMB and MSI-H were negatively correlated in the ACVR1 high-expression group. Four markers of MMR showed upregulated expression, and pMMR was an indicator of MSI-L and MSS. Increased expression of TMB and MSI-H was favorable for immunotherapy (48), whereas low TMB and MSI-L and MSS in the ACVR1 high-expression group suggested a poor immunotherapy effect. In particular, patients with high ACVR1 expression and low TMB had the worst survival and prognosis. In addition, among somatic cell mutations, TNN has the highest mutation frequency. TNN has an increased degree of mutation in gastric cancer, thereby inducing the progression of gastric cancer (49). However, the TNN mutation frequency in our study was lower in the ACVR1 high-risk group than in the low-risk group, indicating that the malignancy of gastric cancer was higher in the low-expression group, which implies better immunotherapy in the low-risk group and poor immunotherapy in the high-risk group (50). In conclusion, these biomarkers reconfirmed that patients with gastric cancer and high expression of ACVR1 have poor prognosis and receive substantially reduced benefit from immunotherapy.
Conclusions
In this study, we analyzed the expression, prognosis, and immune microenvironment of ACVR1 from TCGA database, verified the expression and prognosis of ACVR1 by IHC data, characterized the immune cell infiltration using single-cell data, and predicted the effect of immunotherapy using immune cohort data. The results showed that high ACVR1 expression may promote gastric cancer development by suppressing immunity due to the enrichment of related cancer-promoting stromal pathways, high infiltration of inhibitory immune cells, high immune escape, and low TMB, MSI-L, and TNN mutation rates. Moreover, the immunotherapy benefit was less in the ACVR1 high-expression group. The limitation of this study was that experimental validation was insufficient, and in vivo and in vitro experiments are needed to fully explore the molecular mechanism underlying the relationship between ACVR1 and immunity and the effect of drug therapy on gastric cancer.
Acknowledgments
Funding: This study was supported by
Footnote
Reporting Checklist: The authors have completed the REMARK reporting checklist. Available at https://jgo.amegroups.com/article/view/10.21037/jgo-23-984/rc
Data Sharing Statement: Available at https://jgo.amegroups.com/article/view/10.21037/jgo-23-984/dss
Peer Review File: Available at https://jgo.amegroups.com/article/view/10.21037/jgo-23-984/prf
Conflicts of Interest: All authors have completed the ICMJE uniform disclosure form (available at https://jgo.amegroups.com/article/view/10.21037/jgo-23-984/coif). The authors have no conflicts of interest to declare.
Ethical Statement: The authors are accountable for all aspects of the work in ensuring that questions related to the accuracy or integrity of any part of the work are appropriately investigated and resolved. The study was conducted in accordance with the Declaration of Helsinki (as revised in 2013). The study was approved by the ethics board of Affiliated Hospital of Nantong University (No. 2023-L067) and informed consent was taken from all the patients.
Open Access Statement: This is an Open Access article distributed in accordance with the Creative Commons Attribution-NonCommercial-NoDerivs 4.0 International License (CC BY-NC-ND 4.0), which permits the non-commercial replication and distribution of the article with the strict proviso that no changes or edits are made and the original work is properly cited (including links to both the formal publication through the relevant DOI and the license). See: https://creativecommons.org/licenses/by-nc-nd/4.0/.
References
- Norwood DA, Montalvan-Sanchez E, Dominguez RL, et al. Gastric Cancer: Emerging Trends in Prevention, Diagnosis, and Treatment. Gastroenterol Clin North Am 2022;51:501-18. [Crossref] [PubMed]
- Shah D, Bentrem D. Environmental and genetic risk factors for gastric cancer. J Surg Oncol 2022;125:1096-103. [Crossref] [PubMed]
- Jin X, Liu Z, Yang D, et al. Recent Progress and Future Perspectives of Immunotherapy in Advanced Gastric Cancer. Front Immunol 2022;13:948647. [Crossref] [PubMed]
- Zhang SX, Liu W, Ai B, et al. Current Advances and Outlook in Gastric Cancer Chemoresistance: A Review. Recent Pat Anticancer Drug Discov 2022;17:26-41. [Crossref] [PubMed]
- Zeng Y, Jin RU. Molecular pathogenesis, targeted therapies, and future perspectives for gastric cancer. Semin Cancer Biol 2022;86:566-82. [Crossref] [PubMed]
- Zeng D, Li M, Zhou R, et al. Tumor Microenvironment Characterization in Gastric Cancer Identifies Prognostic and Immunotherapeutically Relevant Gene Signatures. Cancer Immunol Res 2019;7:737-50. [Crossref] [PubMed]
- Xiao Y, Yu D. Tumor microenvironment as a therapeutic target in cancer. Pharmacol Ther 2021;221:107753. [Crossref] [PubMed]
- Wang N, Zhu L, Xu X, et al. Integrated analysis and validation reveal ACAP1 as a novel prognostic biomarker associated with tumor immunity in lung adenocarcinoma. Comput Struct Biotechnol J 2022;20:4390-401. [Crossref] [PubMed]
- Yi M, Zheng X, Niu M, et al. Combination strategies with PD-1/PD-L1 blockade: current advances and future directions. Mol Cancer 2022;21:28. [Crossref] [PubMed]
- Joshi SS, Badgwell BD. Current treatment and recent progress in gastric cancer. CA Cancer J Clin 2021;71:264-79. [Crossref] [PubMed]
- Kono K, Nakajima S, Mimura K. Current status of immune checkpoint inhibitors for gastric cancer. Gastric Cancer 2020;23:565-78. [Crossref] [PubMed]
- Nagar G, Mittal P, Gupta SRR, et al. Multi-omics therapeutic perspective on ACVR1 gene: from genetic alterations to potential targeting. Brief Funct Genomics 2023;22:123-42. [Crossref] [PubMed]
- Peng D, Fu M, Wang M, et al. Targeting TGF-β signal transduction for fibrosis and cancer therapy. Mol Cancer 2022;21:104. [Crossref] [PubMed]
- Ramachandran A, Mehić M, Wasim L, et al. Pathogenic ACVR1(R206H) activation by Activin A-induced receptor clustering and autophosphorylation. EMBO J 2021;40:e106317. [Crossref] [PubMed]
- Aykul S, Huang L, Wang L, et al. Anti-ACVR1 antibodies exacerbate heterotopic ossification in fibrodysplasia ossificans progressiva (FOP) by activating FOP-mutant ACVR1. J Clin Invest 2022;132:e153792. [Crossref] [PubMed]
- Lee HW, Chong DC, Ola R, et al. Alk2/ACVR1 and Alk3/BMPR1A Provide Essential Function for Bone Morphogenetic Protein-Induced Retinal Angiogenesis. Arterioscler Thromb Vasc Biol 2017;37:657-63. [Crossref] [PubMed]
- Chifotides HT, Bose P, Verstovsek S. Momelotinib: an emerging treatment for myelofibrosis patients with anemia. J Hematol Oncol 2022;15:7. [Crossref] [PubMed]
- Lin JB, Chen JF, Lai FC, et al. Involvement of activin a receptor type 1 (ACVR1) in the pathogenesis of primary focal hyperhidrosis. Biochem Biophys Res Commun 2020;528:299-304. [Crossref] [PubMed]
- de Sousa Lopes SM, Roelen BA, Monteiro RM, et al. BMP signaling mediated by ALK2 in the visceral endoderm is necessary for the generation of primordial germ cells in the mouse embryo. Genes Dev 2004;18:1838-49. [Crossref] [PubMed]
- Thomas PS, Rajderkar S, Lane J, et al. AcvR1-mediated BMP signaling in second heart field is required for arterial pole development: implications for myocardial differentiation and regional identity. Dev Biol 2014;390:191-207. [Crossref] [PubMed]
- Yu X, Ton AN, Niu Z, et al. ACVR1-activating mutation causes neuropathic pain and sensory neuron hyperexcitability in humans. Pain 2023;164:43-58. [Crossref] [PubMed]
- Fortin J, Tian R, Zarrabi I, et al. Mutant ACVR1 Arrests Glial Cell Differentiation to Drive Tumorigenesis in Pediatric Gliomas. Cancer Cell 2020;37:308-323.e12. [Crossref] [PubMed]
- Valer JA, Sánchez-de-Diego C, Pimenta-Lopes C, et al. ACVR1 Function in Health and Disease. Cells 2019;8:1366. [Crossref] [PubMed]
- Grcević D, Kusec R, Kovacić N, et al. Bone morphogenetic proteins and receptors are over-expressed in bone-marrow cells of multiple myeloma patients and support myeloma cells by inducing ID genes. Leuk Res 2010;34:742-51. [Crossref] [PubMed]
- Wang Y, Zhang Z, Wang J. MicroRNA-384 inhibits the progression of breast cancer by targeting ACVR1. Oncol Rep 2018;39:2563-74. [Crossref] [PubMed]
- Fukuda T, Fukuda R, Miyazono K, et al. Tumor Promoting Effect of BMP Signaling in Endometrial Cancer. Int J Mol Sci 2021;22:7882. [Crossref] [PubMed]
- Kevenaar ME, Themmen AP, van Kerkwijk AJ, et al. Variants in the ACVR1 gene are associated with AMH levels in women with polycystic ovary syndrome. Hum Reprod 2009;24:241-9. [Crossref] [PubMed]
- Li L, Liu Y, Guo Y, et al. Regulatory MiR-148a-ACVR1/BMP circuit defines a cancer stem cell-like aggressive subtype of hepatocellular carcinoma. Hepatology 2015;61:574-84. [Crossref] [PubMed]
- Ning J, Ye Y, Bu D, et al. Imbalance of TGF-β1/BMP-7 pathways induced by M2-polarized macrophages promotes hepatocellular carcinoma aggressiveness. Mol Ther 2021;29:2067-87. [Crossref] [PubMed]
- Convente MR, Chakkalakal SA, Yang E, et al. Depletion of Mast Cells and Macrophages Impairs Heterotopic Ossification in an Acvr1(R206H) Mouse Model of Fibrodysplasia Ossificans Progressiva. J Bone Miner Res 2018;33:269-82. [Crossref] [PubMed]
- Sha D, Jin Z, Budczies J, et al. Tumor Mutational Burden as a Predictive Biomarker in Solid Tumors. Cancer Discov 2020;10:1808-25. [Crossref] [PubMed]
- Jardim DL, Goodman A, de Melo Gagliato D, et al. The Challenges of Tumor Mutational Burden as an Immunotherapy Biomarker. Cancer Cell 2021;39:154-73. [Crossref] [PubMed]
- He Y, Zhang L, Zhou R, et al. The role of DNA mismatch repair in immunotherapy of human cancer. Int J Biol Sci 2022;18:2821-32. [Crossref] [PubMed]
- Sun Z, Liu C, Jiang WG, et al. Deregulated bone morphogenetic proteins and their receptors are associated with disease progression of gastric cancer. Comput Struct Biotechnol J 2020;18:177-88. [Crossref] [PubMed]
- Chen HY, Hu Y, Xu XB, et al. Upregulation of oncogene Activin A receptor type I by Helicobacter pylori infection promotes gastric intestinal metaplasia via regulating CDX2. Helicobacter 2021;26:e12849. [Crossref] [PubMed]
- Heinhuis KM, Ros W, Kok M, et al. Enhancing antitumor response by combining immune checkpoint inhibitors with chemotherapy in solid tumors. Ann Oncol 2019;30:219-35. [Crossref] [PubMed]
- Valero C, Lee M, Hoen D, et al. The association between tumor mutational burden and prognosis is dependent on treatment context. Nat Genet 2021;53:11-5. [Crossref] [PubMed]
- Sexton RE, Al Hallak MN, Diab M, et al. Gastric cancer: a comprehensive review of current and future treatment strategies. Cancer Metastasis Rev 2020;39:1179-203. [Crossref] [PubMed]
- Paluch EK, Aspalter IM, Sixt M. Focal Adhesion-Independent Cell Migration. Annu Rev Cell Dev Biol 2016;32:469-90. [Crossref] [PubMed]
- Infantino V, Santarsiero A, Convertini P, et al. Cancer Cell Metabolism in Hypoxia: Role of HIF-1 as Key Regulator and Therapeutic Target. Int J Mol Sci 2021;22:5703. [Crossref] [PubMed]
- Masucci MT, Minopoli M, Del Vecchio S, et al. The Emerging Role of Neutrophil Extracellular Traps (NETs) in Tumor Progression and Metastasis. Front Immunol 2020;11:1749. [Crossref] [PubMed]
- Peña-Romero AC, Orenes-Piñero E. Dual Effect of Immune Cells within Tumour Microenvironment: Pro- and Anti-Tumour Effects and Their Triggers. Cancers (Basel) 2022;14:1681. [Crossref] [PubMed]
- Liu Y, Zhou N, Zhou L, et al. IL-2 regulates tumor-reactive CD8(+) T cell exhaustion by activating the aryl hydrocarbon receptor. Nat Immunol 2021;22:358-69. [Crossref] [PubMed]
- Darvin P, Toor SM, Sasidharan Nair V, et al. Immune checkpoint inhibitors: recent progress and potential biomarkers. Exp Mol Med 2018;50:1-11. [Crossref] [PubMed]
- Yuan Y, Adam A, Zhao C, et al. Recent Advancements in the Mechanisms Underlying Resistance to PD-1/PD-L1 Blockade Immunotherapy. Cancers (Basel) 2021;13:663. [Crossref] [PubMed]
- Dolina JS, Van Braeckel-Budimir N, Thomas GD, et al. CD8(+) T Cell Exhaustion in Cancer. Front Immunol 2021;12:715234. [Crossref] [PubMed]
- Chen X, Feng L, Huang Y, et al. Mechanisms and Strategies to Overcome PD-1/PD-L1 Blockade Resistance in Triple-Negative Breast Cancer. Cancers (Basel) 2022;15:104. [Crossref] [PubMed]
- Palmeri M, Mehnert J, Silk AW, et al. Real-world application of tumor mutational burden-high (TMB-high) and microsatellite instability (MSI) confirms their utility as immunotherapy biomarkers. ESMO Open 2022;7:100336. [Crossref] [PubMed]
- Wang Y, Jiang R, Zhao H, et al. TTN-AS1 delivered by gastric cancer cell-derived exosome induces gastric cancer progression through in vivo and in vitro studies. Cell Biol Toxicol 2023;39:557-71. [Crossref] [PubMed]
- Hu X, Wang Z, Wang Q, et al. Molecular classification reveals the diverse genetic and prognostic features of gastric cancer: A multi-omics consensus ensemble clustering. Biomed Pharmacother 2021;144:112222. [Crossref] [PubMed]