ZNF326 as a potential prognostic and predictive biomarker in stage II colorectal cancer
Highlight box
Key findings
• Our study identified ZNF326 as a potential prognostic and predictive biomarker in stage II colorectal cancer (CRC) patients. Stage II CRC patients with low ZNF326 expression levels exhibited a poor 5-year overall survival (OS) rate compared to those with high ZNF326 expression. Furthermore, patients in stage II with high ZNF326 expression levels are more likely to derive benefits from adjuvant chemotherapy compared to low ZNF326 expression.
What is known and what is new?
• Current stratification algorithms are still insufficient to identify stage II CRC patients with poor prognostic risk factors.
• We implemented a screening strategy utilizing quantitative proteomics to identify ZNF326 in 11 paired CRC patients selected by a nested case-control design. Subsequently, we investigated the association between ZNF326 expression level with the prognosis of stage II CRC patients and the benefit from adjuvant chemotherapy.
What is the implication, and what should change now?
• ZNF326 holds promise for clinical application in risk classification among stage II CRC patients.
Introduction
Colorectal cancer (CRC) is the third most common malignancy worldwide with more than 1.9 million new cases diagnosed annually (1) and the second leading cause of cancer-related death (1) with a 5-year mortality rate of about 40% (2); it represents a great health burden in China (3,4). Based on current guidelines, most stage II CRC patients are treated surgically without adjuvant chemotherapy. Whether a stage II CRC patient should receive adjuvant chemotherapy after surgery largely depends on the recurrence risk assessed by a group of factors (5,6). Still, a considerable proportion of stage II patients who are evaluated as low-risk later experience recurrence and progression. The decision to treat stage II CRC patients with adjuvant chemotherapy has been one of the most challenging (7-10) and controversial issues in oncology over the past 20 years. Therefore, biomarkers are needed to more accurately identify patients with stage II CRC who are suitable for adjuvant chemotherapy.
Many specialist bodies have published separate guidelines for the adjuvant treatment of stage II CRC and there is considerable variation (11-13). The guidelines recommend considering adjuvant chemotherapy for stage II patients with high-risk clinicopathologic features, such as T4 tumors, poorly differentiated histology, lymphatic/vascular/perineural invasion, obstruction, perforation, and inadequate lymph node sampling, although the definition of "high-risk" is not standardized (14). However, with the exception of T4 tumors, these factors are not sufficient to identify those patients with stage II CRC who may be candidates for adjuvant chemotherapy (15) Significant efforts have been made to identify new biomarkers, including gene expression signatures, microRNA profiling and circulating tumor DNA, to assess patients’ risk of relapse over the past few decades.. However, they often struggle to become routine clinical tests, perhaps because of low technical reproducibility, the requirement of fresh-frozen tissues, or the lack of validation in large prospective trials (16-20). As a result, there is a clinical need for more precise biomarkers to determine which patients will benefit from adjuvant chemotherapy.
As the effector molecules of genes, proteins carry out biological functions of the genes in the cell. Thus, proteomics could provide more direct evidence and better solutions to cancer problems (21). Compared with genomics, the verification and interpretation provided by proteomics are closer to phenotype, and virtually all existing drug targets are proteins (22,23). We have previously reported that at least 5 proteins, including the ZNF326, varied on the tumor tissues between the recurrence and non-recurrence CRC patients through a nested case-control cohort that combined the proteomics (24). However, the prognostic value of ZNF326 in stage II CRC patients is unclear.
In this study, ZNF326 was selected as a candidate biomarker from our last study. We evaluated its prognostic impact using 2 gene expression data sets for training and validation in different cohorts of CRC patients. We also assessed the association of ZNF326 expression status and the benefit from adjuvant chemotherapy in stage II CRC patients. We present this article in accordance with the REMARK reporting checklist (available at https://jgo.amegroups.com/article/view/10.21037/jgo-23-908/rc).
Methods
Study design
ZNF326 was selected as a candidate biomarker based on previous proteomics analyses and literature reviews (24). Briefly, we established a Yunnan CRC cohort comprising stage I–III CRC patients at Yunnan Cancer Hospital between December 2010 and February 2019. Then, propensity score matching (PSM) was used to adjust for critical variables between post-operative and non-post-operative metastatic patients. A nested case-control cohort was employed, and 11 pairs of patients were selected through PSM. Subsequently, we focused on the intersection of differentially expressed proteins identified using Student’s t-test and metastasis-related protein verified by univariate Cox regression analysis. ZNF326 was selected for further analysis. According to the literature search and our basic research, these genes are rarely reported in most cancers. The study was conducted in accordance with the Declaration of Helsinki (as revised in 2013). The study was approved by the Yunnan Cancer Hospital Ethics Committee (No. KY2019141). The requirement for informed consent was waived by the Ethics Committee owing to the retrospective nature of the study.
The following processes were employed to analyze ZNF326. Firstly, we used X-Tile (Yale School of Medicine, New Haven, CT, USA) to identify an optimal cutoff for ZNF326 expression level in the discovery cohort of the stage II CRC patients. Secondly, we tested its association with the prognosis of stage II CRC patients in a discovery data set, and verified the association in a different cohort of stage II CRC patients as the validation data set. Then, we analyzed the correlation between the ZNF326 expression level and existing prognostic factors. Subgroup analyses were used to assess the robustness of the risk estimations. Thirdly, a pooled database was used for analyses of the association of ZNF326 and the benefit from adjuvant chemotherapy in stage II CRC patients. Figure 1 shows the study flowchart.
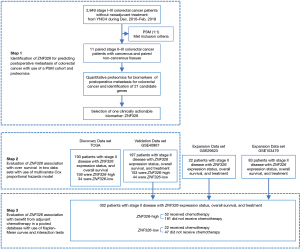
Datasets collection
CRC tissue gene expression profiles, annotated with clinical and pathological information, were obtained from two independent sources: The Cancer Genome Atlas (TCGA) for the discovery dataset and GSE40967 in the National Center for Biotechnology Information Gene Expression Omnibus (NCBI-GEO) for the validation dataset. Combined data pooled by three data sets, namely, GSE29623, GSE40967, and GSE103479, were found to satisfy our criteria [including pathological stage, available information on ZNF326 gene expression, adjuvant chemotherapy, date of overall survival (OS), and follow-up duration] was used to explore the relationship with adjuvant chemotherapy. The detailed workflows are described in Figures S1-S4. A comprehensive depiction of the patient cohorts from the 2 independent sources is available in Table S1.
We downloaded from the TCGA portal (https://portal.gdc.cancer.gov/) and the NCBI-GEO dataset (https://www.ncbi.nlm.nih.gov/geo/) transcriptome profiles in fragments per kilobase million (FPKM) format and corresponding clinical information. The NCBI-GEO datasets used for the multiple dataset analysis were based on different platforms. Therefore, we combined the three datasets by normalizing them using the Robust Multichip Average (RMA) algorithm (25) and removing batch effects using the affy and sva R packages (26,27) to increase the sample size and avoid less reliable results. Meanwhile, we averaged the probes corresponding to the same gene.
Identification of the optimal cutoff for ZNF326 expression level
Due to the variation of sequencing between the TCGA and NCBI-GEO datasets, we used the Z-score to balance the difference before the data analysis. ZNF326 expression levels were stratified into ZNF326-high and ZNF326-low subgroups according to the ZNF326 expression. X-tile (28) was used to determine the threshold of ZNF326 expression in TCGA dataset and we applied this threshold to the NCBI-GEO dataset.
Association analysis of ZNF326 with prognosis in the discovery and validation data sets
We explored the association between ZNF326 expression levels and OS in both of these data sets. Subgroup analyses were performed based on adjuvant chemotherapy, age, gender, tumor location, genetic mutations in the BRAF, KARS, and TP53 genes, and mismatch repair (MMR), with tests for interaction by the Cox regression model in GSE40967.
Correlation analyses of ZNF326 expression with existing prognostic factors
The relationship between ZNF326 expression with prognostic factors, including age, gender, genetic mutations in the BRAF, KARS, and TP53 genes, and MMR, was investigated in GSE40967. The distribution of ZNF326 expression in binary prognostic factors was compared using violin plots and t-test, and the correlations between ZNF326 expression and age were analyzed based on scatterplot and Pearson’s correlation analysis. Meanwhile, we explored the expression of ZNF326 in the tumor samples and normal samples based on the Human Protein Atlas (HPA) database (https://www.proteinatlas.org/). Many articles have demonstrated that chemokines and their receptors regulate tumor progression and metastasis. Correlations also were calculated between ZNF326 expression and chemokines across human cancers based on Tumor and Immune System Interaction Database (29) (TISIDB, http://cis.hku.hk/TISIDB).
ZNF326 expression and benefit from adjuvant chemotherapy
By pooling 3 datasets (GSE40967, GSE29623, and GSE103479), we investigated the association between ZNF326 expression, assessed either at the messenger RNA (mRNA) level, and OS in patients who received or did not receive adjuvant chemotherapy in the NCBI-GEO dataset to assess whether patients with ZNF326-low tumors might benefit from adjuvant chemotherapy.
Statistical analysis
Qualitative and quantitative variables were compared between the 2 patient groups using χ2 test and t-test, respectively. Univariate and multivariate survival analyses were conducted using the Cox proportional hazards regression model. Survival plots were generated with the use of Kaplan-Meier method and compared by log-rank test. Statistical analyses were performed using R version 3.6.0 (R Foundation for Statistical Computing, Vienna, Austria) and corresponding R packages.
Results
Optimal cutoff for ZNF326 expression level
According to our last study involving a nested case-control cohort combining the proteomics, 21 proteins varied on the tumor tissues between the recurrence and non-recurrence CRC patients. Based on this, we selected ZNF326 as a candidate biomarker. Previous studies had indicated that ZNF326 expression was associated with specific disease processes, such as in non-small cell lung cancer (NSCLC), triple-negative breast cancer (TNBC), glioma, and schizophrenia (30-34). Figure S5 shows the distribution in metastatic and non-metastatic patients, tumor and tumor-adjacent tissues.
The optimal cutoff value for ZNF326-scaled expression count at dissection was identified using X-tile software in the discovery cohort of the stage II CRC patients (n=193). As shown in Figure S6, the optimal cutoff value of ZNF326 was −0.72. Then, the level of ZNF326 was divided into ZNF326-high and ZNF326-low groups using cutoff value: there were 159 (82.4%) patients with ZNF326 above −0.72 and 34 (17.6%) patients with ZNF326 less than or equal to −0.72, which indicated significant differences among the cutoff value.
ZNF326 expression and OS in the TCGA discovery data set
We aimed to explore the association between ZNF326 expression and OS among stage II patients in the TCGA discovery data set. The 5-year OS of the 2 groups was compared using Kaplan-Meier curves. As shown in Figure 2, the rate of 5-year OS was lower among 34 patients (17.6%) with ZNF326-low level than among those 159 (82.4%) patients with ZNF326-high level (P=0.008; hazard ratio (HR): 3.13, 95% confidence interval (CI): 1.29–7.58]. In a multivariate analysis that includes age and sex as confounding variables, shown in Table 1, the HR for OS among stage II patients with ZNF326-low versus ZNF326-high was 2.77 (95% CI: 1.11–6.94; P=0.029).
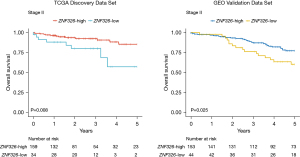
Table 1
Dataset | Variable | Univariate analysis | Multivariate analysis | |||
---|---|---|---|---|---|---|
HR (95% CI) | P value | HR (95% CI) | P value | |||
TCGA | Agea | 1.12 (1.05–1.18) | <0.001 | 1.11 (1.05–1.18) | <0.001 | |
Male vs. female | 1.09 (0.46–2.58) | 0.849 | 1.20 (0.49–2.92) | 0.690 | ||
ZNF326_low vs. ZNF326_high | 3.13 (1.29–7.58) | 0.012 | 2.77 (1.11–6.94) | 0.029 | ||
GSE40967 | Agea | 1.03 (1.00–1.05) | 0.041 | 1.03 (1.00–1.06) | 0.032 | |
Male vs. female | 1.07 (0.59–1.94) | 0.817 | 1.22 (0.66–2.24) | 0.521 | ||
ZNF326_low vs. ZNF326_high | 1.98 (1.08–3.65) | 0.028 | 2.21 (1.19–4.10) | 0.012 | ||
Adjuvant chemotherapyb | 0.76 (0.35–1.63) | 0.484 | 0.83 (0.37–1.85) | 0.641 |
a, continuous variable. b, yes vs. no. TCGA, The Cancer Genome Atlas; HR, hazard ratio; CI, confidence interval.
ZNF326 expression and survival in the NCBI-GEO validation data set
To evaluate the robustness of our findings, we performed analysis within the GSE40967 in NCBI-GEO data set. As shown in Figure 2, low ZNF326 expression was associated with poorer 5-year OS in stage II patients (P=0.025; HR: 1.98, 95% CI: 1.08–3.65). After adjustment for sex, age, and adjuvant chemotherapy, as shown in Table 1, multivariate analysis confirmed that low ZNF326 expression status was associated with poor prognosis and an independent prognostic factor for 5-year OS (HR: 2.21, 95% CI: 1.19–4.10).
Correlation analyses of ZNF326 expression level with existing prognostic factors and chemokines
To evaluate the prognostic ability of ZNF326, we assessed the correlation between the expression of ZNF326 and clinical and pathological features from the GSE40967 cohort (age, sex, genetic mutations in the BRAF, KARS, and TP53 genes, and MMR), and ZNF326 was found to be independent of those prognostic factors (all P>0.05, Figure S7). Next, the expression of ZNF326 was validated in the tumor samples and normal samples in HPA database. As shown in Figure 3, ZNF326 was lowly expressed in normal colon and rectum tissues. We also explored correlations between ZNF326 expression and chemokines across human cancers based on the TISIDB database. As shown in Figure S8, most chemokines were negatively correlated with the expression of ZNF326 and CCL23 was significantly associated with ZNF326 expression in CRC (r=−0.59, P<0.001).
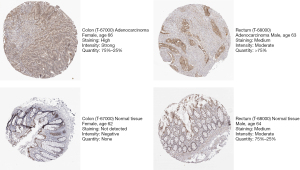
Subgroup analyses
We performed subgroup analysis to further investigate the association between clinical and pathological characteristics with ZNF326 expression levels in GSE40967. As displayed in Figure 4, the ZNF326-low expression level was significantly associated with the lower 5-year OS in patients treated with adjuvant chemotherapy (HR: 6.13, 95% CI: 1.23–30.46), age less than 60 years old (HR: 3.75, 95% CI: 1.06–13.30), and female patients (HR: 3.46, 95% CI: 1.40–8.52). It was also correlated to the 5-year OS in patients with wild-type BRAF gene (HR: 2.31, 95% CI: 1.20–4.45) and patients with mutated KRAS gene (HR: 3.53, 95% CI: 1.36–9.16).
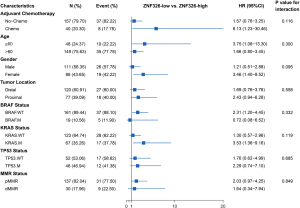
ZNF326 expression and benefit from adjuvant chemotherapy
To further clarify whether stage II patients with different ZNF326 expression levels might benefit from adjuvant chemotherapy, we explored the association between ZNF326 status and OS among stage II patients who either did or did not accept adjuvant chemotherapy in the NCBI-GEO database. As displayed in Figure 5, treatment with adjuvant chemotherapy was associated with a higher rate of OS in all stage II patients (P=0.021; HR: 0.46, 95% CI: 0.24–0.90) and the ZNF326-high patients (P=0.011; HR: 0.28, 95% CI: 0.10–0.80). Meanwhile, adjuvant chemotherapy was independent of a higher rate of OS in ZNF326-low patients (P=0.472; HR: 0.71, 95% CI: 0.28–1.81), which highlights that stage II patients with ZNF326-high status are more likely to benefit from adjuvant chemotherapy than stage II patients with ZNF326-low level.
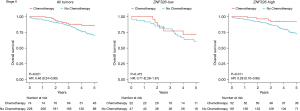
Discussion
Although current published clinical guidelines suggest that adjuvant chemotherapy may be appropriate for stage II CRC patients with poor prognostic features, it is estimated that the adjuvant chemotherapy only provides a relatively small absolute benefit (3–4% patients with survival improvement) for stage II CRC patients (14,35). Accurately predicting the prognosis at the time of diagnosis is critical for clinicians to tailor the treatment plan for maximal efficacy and to determine surveillance strategies (36). However, prognostic markers in stage II CRC have been sparse and the available ones are not easily translated into the clinical setting (37).
We employed a nested case-control design combining the proteomics to discover a prognostic biomarker in CRC patients. Based on literature search and previous basic experiments, ZNF326 was selected as a biomarker. It was first discovered in Drosophila, where it is thought to play an important role in the differentiation of nerve cells (38). ZNF326 has been shown to promote the epithelial-mesenchymal transition (EMT) and invasiveness of CRC cells, and CRC patients with high ZNF326 expression level were positively correlated with tumor differentiation, tumor-node-metastasis (TNM) staging, and lymph node metastasis (39). Several previous studies have demonstrated that ZNF326 expression levels are associated with prognosis of other cancers, including that it promotes the proliferation of NSCLC cells by regulating the expression of ERCC1 (30), plays a vital role in promoting the malignant phenotype of breast cancer cells by interacting with DBC1 and is associated with poor prognosis (31,40), and expresses highly in glioma cell lines and tissues and is closely related with advanced tumor grade in the patients (33).
Our results revealed that stage II CRC and ZNF326-low expression level were both associated with poor OS with adjustment of the existing prognostic factors in the discovery dataset and validation dataset. Hence, ZNF326 was an independent prognostic biomarker. A study conducted by Uhlen et al. reported that ZNF326 is a favorable prognostic gene symbol (P=0.007) in CRC patients based on a pathology atlas of the human cancer transcriptome (41), which is consistent with our finding. Besides, we detected an association of ZNF326 expression level and the benefit from adjuvant chemotherapy in stage II CRC patients. Our finding confirmed that ZNF326 was a predictive biomarker for the adjuvant chemotherapy.
To exclude existing prognostic factors that may impact ZNF326 expression, correlation analyses were performed between ZNF326 expression and prognostic factors based on t-test or Pearson’s correlation analysis. Of those factors, age (r=0.1, P=0.16), sex (P=0.76), MMR (P=0.38), mutational status of BRAF gene (P=0.70), KRAS gene (P=0.67), and TP53 gene (P=0.38) were not significantly correlated with ZNF326 expression; ZNF326 was found to be independent of those prognostic factors. Different immune cell subsets are recruited into the tumor microenvironment via interactions between chemokines and chemokine receptors, and these populations have distinct effects on tumor progression and therapeutic outcomes (42). In this regard, we employed correlation analyses between ZNF326 gene expression and chemokines across human cancers using TISIDB and found that expression of ZNF326 in most cancers is negatively correlated with chemokines. Importantly, CCL23 was significantly associated with ZNF326 expression in CRC patients (r=−0.59, P<0.001), which indicated that ZNF326 may affect the prognosis of CRC patients by participating in immune processes.
Our findings suggest that stage II CRC patients with ZNF326-high expression status might benefit from adjuvant chemotherapy, therefore, ZNF326 might be potential predictive biomarker for stage II CRC patients in the current clinical setting. Indeed, subgroup analysis was used to explore the relationship between other clinical and pathological features with adjuvant chemotherapy. It is worth noting that ZNF326 expression demonstrated significant prognostic value among less than 60 years old and female patients, it was also correlated to the 5-year OS in patients with wild-type BRAF gene and mutated KRAS gene. Therefore, ZNF326 expression status alone or in combination with conventional features, such as tumor age, sex, and genetic mutation status in BRAF and KRAS has the potential to improve prognosis and influence postoperative decisions.
Our study had several limitations. Firstly, as certain CRC patients were lacking recurrence-free survival (RFS) information in the discovery dataset, our current study focused on OS; thus, to a certain extent, the effect of ZNF326 expression on RFS could not be explained. Second, information on some important clinicopathological features of patients in public cohorts, including TCGA and GEO, is incomplete. Third, because of the exploratory and retrospective nature of the study, a prospectively designed study is necessary to further validate the prognostic value of ZNF326.
Conclusions
With the use of independent cohort and public datasets comprising stage II CRC patients, extensive protein screening was performed and validated in conjunction with public databases. Our results indicated that the rate of 5-year OS was lower among stage II CRC patients with low ZNF326 expression level than those with ZNF326-high status. Stage II patients with ZNF326-high status are more likely to benefit from adjuvant chemotherapy than those with low ZNF326 expression level. In conclusion, ZNF326 has the potential to be used in clinical practice for risk classification. However, given the prospectively designed of our study, our results need to be further validated.
Acknowledgments
The authors thank the patients for making this study possible.
Funding: This study was supported by
Footnote
Reporting Checklist: The authors have completed the REMARK reporting checklist. Available at https://jgo.amegroups.com/article/view/10.21037/jgo-23-908/rc
Data Sharing Statement: Available at https://jgo.amegroups.com/article/view/10.21037/jgo-23-908/dss
Peer Review File: Available at https://jgo.amegroups.com/article/view/10.21037/jgo-23-908/prf
Conflicts of Interest: All authors have completed the ICMJE uniform disclosure form (available at https://jgo.amegroups.com/article/view/10.21037/jgo-23-908/coif). The authors have no conflicts of interest to declare.
Ethical Statement: The authors are accountable for all aspects of the work in ensuring that questions related to the accuracy or integrity of any part of the work are appropriately investigated and resolved. The study was conducted in accordance with the Declaration of Helsinki (as revised in 2013). The study was approved by the Yunnan Cancer Hospital Ethics Committee (No. KY2019141). The requirement for informed consent was waived by the Ethics Committee owing to the retrospective nature of the study.
Open Access Statement: This is an Open Access article distributed in accordance with the Creative Commons Attribution-NonCommercial-NoDerivs 4.0 International License (CC BY-NC-ND 4.0), which permits the non-commercial replication and distribution of the article with the strict proviso that no changes or edits are made and the original work is properly cited (including links to both the formal publication through the relevant DOI and the license). See: https://creativecommons.org/licenses/by-nc-nd/4.0/.
References
- Sung H, Ferlay J, Siegel RL, et al. Global Cancer Statistics 2020: GLOBOCAN Estimates of Incidence and Mortality Worldwide for 36 Cancers in 185 Countries. CA Cancer J Clin 2021;71:209-49. [Crossref] [PubMed]
- Siegel RL, Miller KD, Jemal A. Cancer statistics, 2020. CA Cancer J Clin 2020;70:7-30. [Crossref] [PubMed]
- Feng RM, Zong YN, Cao SM, et al. Current cancer situation in China: good or bad news from the 2018 Global Cancer Statistics? Cancer Commun (Lond) 2019;39:22. [Crossref] [PubMed]
- Wang ZX, Yao YC, Mai ZJ, et al. Temporal Change in Treatment Patterns of Metastatic Colorectal Cancer and Its Association with Patient Survival: A Retrospective Cohort Study Based on an Intelligent Big-Data Platform. Engineering 2021;7:526-33.
- Oñate-Ocaña LF, Montesdeoca R, López-Graniel CM, et al. Identification of patients with high-risk lymph node-negative colorectal cancer and potential benefit from adjuvant chemotherapy. Jpn J Clin Oncol 2004;34:323-8. [Crossref] [PubMed]
- O'Connor ES, Greenblatt DY, LoConte NK, et al. Adjuvant chemotherapy for stage II colon cancer with poor prognostic features. J Clin Oncol 2011;29:3381-8. [Crossref] [PubMed]
- Dotan E, Cohen SJ. Challenges in the management of stage II colon cancer. Semin Oncol 2011;38:511-20. [Crossref] [PubMed]
- Kelley RK, Venook AP. Prognostic and predictive markers in stage II colon cancer: is there a role for gene expression profiling? Clin Colorectal Cancer 2011;10:73-80. [Crossref] [PubMed]
- Vicuna B, Benson AB 3rd. Adjuvant therapy for stage II colon cancer: prognostic and predictive markers. J Natl Compr Canc Netw 2007;5:927-36. [Crossref] [PubMed]
- Meropol NJ. Ongoing challenge of stage II colon cancer. J Clin Oncol 2011;29:3346-8. [Crossref] [PubMed]
- Benson AB 3rd, Schrag D, Somerfield MR, et al. American Society of Clinical Oncology recommendations on adjuvant chemotherapy for stage II colon cancer. J Clin Oncol 2004;22:3408-19. [Crossref] [PubMed]
- Schmoll HJ, Van Cutsem E, Stein A, et al. ESMO Consensus Guidelines for management of patients with colon and rectal cancer. a personalized approach to clinical decision making. Ann Oncol 2012;23:2479-516. [Crossref] [PubMed]
- Watanabe T, Muro K, Ajioka Y, et al. Japanese Society for Cancer of the Colon and Rectum (JSCCR) guidelines 2016 for the treatment of colorectal cancer. Int J Clin Oncol 2018;23:1-34. [Crossref] [PubMed]
- Dienstmann R, Salazar R, Tabernero J. Personalizing colon cancer adjuvant therapy: selecting optimal treatments for individual patients. J Clin Oncol 2015;33:1787-96. [Crossref] [PubMed]
- Baxter NN, Kennedy EB, Bergsland E, et al. Adjuvant Therapy for Stage II Colon Cancer: ASCO Guideline Update. J Clin Oncol 2022;40:892-910. [Crossref] [PubMed]
- Gröne J, Lenze D, Jurinovic V, et al. Molecular profiles and clinical outcome of stage UICC II colon cancer patients. Int J Colorectal Dis 2011;26:847-58. [Crossref] [PubMed]
- Dalerba P, Sahoo D, Paik S, et al. CDX2 as a Prognostic Biomarker in Stage II and Stage III Colon Cancer. N Engl J Med 2016;374:211-22. [Crossref] [PubMed]
- Kopetz S, Tabernero J, Rosenberg R, et al. Genomic classifier ColoPrint predicts recurrence in stage II colorectal cancer patients more accurately than clinical factors. Oncologist 2015;20:127-33. [Crossref] [PubMed]
- Salazar R, Roepman P, Capella G, et al. Gene expression signature to improve prognosis prediction of stage II and III colorectal cancer. J Clin Oncol 2011;29:17-24. [Crossref] [PubMed]
- Di Narzo AF, Tejpar S, Rossi S, et al. Test of four colon cancer risk-scores in formalin fixed paraffin embedded microarray gene expression data. J Natl Cancer Inst 2014;106:dju247. [Crossref] [PubMed]
- Huang W, Zhan D, Li Y, et al. Proteomics provides individualized options of precision medicine for patients with gastric cancer. Sci China Life Sci 2021;64:1199-211. [Crossref] [PubMed]
- Imming P, Sinning C, Meyer A. Drugs, their targets and the nature and number of drug targets. Nat Rev Drug Discov 2006;5:821-34. [Crossref] [PubMed]
- Ishizuka JJ, Manguso RT, Cheruiyot CK, et al. Loss of ADAR1 in tumours overcomes resistance to immune checkpoint blockade. Nature 2019;565:43-8. [Crossref] [PubMed]
- You D, Zhang S, Yan S, et al. SAMHD1 as a prognostic and predictive biomarker in stage II colorectal cancer: A multicenter cohort study. Front Oncol 2022;12:939982. [Crossref] [PubMed]
- Irizarry RA, Hobbs B, Collin F, et al. Exploration, normalization, and summaries of high density oligonucleotide array probe level data. Biostatistics 2003;4:249-64. [Crossref] [PubMed]
- Gautier L, Cope L, Bolstad BM, et al. affy--analysis of Affymetrix GeneChip data at the probe level. Bioinformatics 2004;20:307-15. [Crossref] [PubMed]
- Leek JT, Johnson WE, Parker HS, et al. The sva package for removing batch effects and other unwanted variation in high-throughput experiments. Bioinformatics 2012;28:882-3. [Crossref] [PubMed]
- Camp RL, Dolled-Filhart M, Rimm DL. X-tile: a new bio-informatics tool for biomarker assessment and outcome-based cut-point optimization. Clin Cancer Res 2004;10:7252-9. [Crossref] [PubMed]
- Ru B, Wong CN, Tong Y, et al. TISIDB: an integrated repository portal for tumor-immune system interactions. Bioinformatics 2019;35:4200-2. [Crossref] [PubMed]
- Wu J, Zhang X, Han Q, et al. ZNF326 promotes proliferation of non-small cell lung cancer cells by regulating ERCC1 expression. Lab Invest 2019;99:169-79. [Crossref] [PubMed]
- Rangel R, Guzman-Rojas L, Kodama T, et al. Identification of New Tumor Suppressor Genes in Triple-Negative Breast Cancer. Cancer Res 2017;77:4089-101. [Crossref] [PubMed]
- Rengasamy M, Zhang F, Vashisht A, et al. The PRMT5/WDR77 complex regulates alternative splicing through ZNF326 in breast cancer. Nucleic Acids Res 2017;45:11106-20. [Crossref] [PubMed]
- Yu X, Wang M, Wu J, et al. ZNF326 promotes malignant phenotype of glioma by up-regulating HDAC7 expression and activating Wnt pathway. J Exp Clin Cancer Res 2019;38:40. [Crossref] [PubMed]
- Barrera-Conde M, Ausin K, Lachén-Montes M, et al. Cannabis Use Induces Distinctive Proteomic Alterations in Olfactory Neuroepithelial Cells of Schizophrenia Patients. J Pers Med 2021;11:160. [Crossref] [PubMed]
- Quasar Collaborative Group. Adjuvant chemotherapy versus observation in patients with colorectal cancer: a randomised study. Lancet 2007;370:2020-9. [Crossref] [PubMed]
- Ji D, Qiao M, Yao Y, et al. Serum-based microRNA signature predicts relapse and therapeutic outcome of adjuvant chemotherapy in colorectal cancer patients. EBioMedicine 2018;35:189-97. [Crossref] [PubMed]
- Carey LA, Berry DA, Cirrincione CT, et al. Molecular Heterogeneity and Response to Neoadjuvant Human Epidermal Growth Factor Receptor 2 Targeting in CALGB 40601, a Randomized Phase III Trial of Paclitaxel Plus Trastuzumab With or Without Lapatinib. J Clin Oncol 2016;34:542-9. [Crossref] [PubMed]
- Lee JY, Nakane Y, Koshikawa N, et al. Characterization of a zinc finger protein ZAN75: nuclear localization signal, transcriptional activator activity, and expression during neuronal differentiation of P19 cells. DNA Cell Biol 2000;19:227-34. [Crossref] [PubMed]
- Yang Y, Yan T, Han Q, et al. ZNF326 promotes colorectal cancer epithelial-mesenchymal transition. Pathol Res Pract 2021;225:153554. [Crossref] [PubMed]
- Yu X, Wang M, Han Q, et al. ZNF326 promotes a malignant phenotype of breast cancer by interacting with DBC1. Mol Carcinog 2018;57:1803-15. [Crossref] [PubMed]
- Uhlen M, Zhang C, Lee S, et al. A pathology atlas of the human cancer transcriptome. Science 2017;357:eaan2507. [Crossref] [PubMed]
- Nagarsheth N, Wicha MS, Zou W. Chemokines in the cancer microenvironment and their relevance in cancer immunotherapy. Nat Rev Immunol 2017;17:559-72. [Crossref] [PubMed]