Predicting bowel function after diverting stoma closure in patients with rectal cancer
Highlight box
Key findings
• The interval between stoma creation and closure, neoadjuvant therapy, and body mass index were identified as the three most crucial characteristics associated with patients experiencing major low anterior resection syndrome (LARS) in this analysis.
What is known and what is new?
• LARS frequently occurs after rectal cancer surgery, impairing the quality of life for patients.
• This model can effectively identify patients with high-risk major LARS in rectal cancer patients after diverting stoma closure, aiding their decision-making and counseling.
What is the implication, and what should change now?
• The model aids patients in informed decisions on stoma closure. Personalized estimates guide surgical management, enhancing patient understanding. Additionally, it identifies high-risk patients, enabling targeted post-operative care for improved outcomes.
Introduction
A diverting stoma can effectively prevent potential or even life-threatening clinical consequences, such as anastomotic leakage, especially in patients with mid-to-low rectal cancer after radical resection (1,2). However, it carries risks such as infection, ulceration, parastomal hernias, stomal obstruction and even psychological disorders (3-8). Patients frequently require regular follow-up at a wound ostomy clinic to monitor the stoma and ensure their overall well-being. Moreover, some patients report a negative impact on their quality of life, such as toilet dependence and are eager to close the stoma as soon as possible (7,9,10). Previous randomized clinical trials showed that closing diverting stoma 8–13 days after primary surgery is feasible and safe in selected patients (11,12). However, bowel dysfunction, also known as low anterior resection syndrome (LARS), frequently occurs after diverting stoma closure and consists of a variable symptom spectrum that typically includes incontinence of flatus, incontinence of liquid stool, frequency, clustering, urgency and impairs quality of life (13,14). Furthermore, these bowel functional changes reduce the expectations of the patients after diverting stoma closure owing to a significant decline in quality of life (15). A qualitative study of bowel function in patients with rectal cancer after stoma closure found that patients often need to deal with acute and troublesome intestinal symptoms and it is difficult for them to control their defecation function, resulting in toilet dependence (15). Therefore, patients with severe bowel dysfunction often need to weigh the pros and cons and re-examine the decision to undergo stoma closure prior to the actual procedure.
Currently, the machine learning algorithm based on artificial intelligence is widely used to predict disease prognosis by combining clinical multidimensional nonlinear features and assist doctors or patients in making their decisions, achieving accuracy beyond the traditional linear model (16-20). The present study aimed to create and validate a machine learning model that could predict the risk probabilities of developing major LARS before stoma closure by combining clinicopathological features. We present this article in accordance with the TRIPOD reporting checklist (available at https://jgo.amegroups.com/article/view/10.21037/jgo-23-1019/rc).
Methods
Study design and participants
A total of 636 patients diagnosed as mid-low rectal cancer (cases located less than 10 cm from the anal verge), who underwent laparoscopic anterior resection at Tongji Hospital affiliated with Huazhong University of Science and Technology between January 1, 2012, and December 31, 2020, were included in the present retrospective study. All patients’ surgeries were performed by senior surgeons, and during the procedures, comprehensive measures were taken to ensure total nerve preservation. Patients with incomplete medical records, ≤18 years old, lost to follow-up or death were excluded. Patients whose diverting stoma was not closed or had been closed for <1 year were also excluded. The study was conducted in accordance with the Declaration of Helsinki (as revised in 2013) and approved by the ethics committees of Tongji Hospital, Huazhong University of Science and Technology (No. TJ-IRB20230364). The requirement for informed consent was waived due to the retrospective nature of the study.
Predictors
Only clinicopathological data before diverting stoma closure were selected as predictors, including age, sex, body mass index (BMI), hypertension, diabetes, neoadjuvant therapy, the length (cm) of the removed bowel, pathological stage of tumor, anastomotic height (cm), the interval (days) between stoma creation and closure, American Society of Anesthesiologists (ASA) classification, operation time, tumor obstruction and tumor size. All the predictors were discussed and determined by senior physicians. Tumor obstruction indicated that the endoscope with a maximum insertion portion width of 12.8 mm could not pass through the space between the tumor and the bowel lumen before the primary surgery. Anastomotic height, which indirectly reflects the distance from the lower edge of the tumor to the anal verge, was assessed during follow-up by digital inspection, rigid sigmoidoscopy, or magnetic resonance imaging. The pathological stage of the tumor was reassessed and reviewed based on the 8th edition of the American Joint Committee on Cancer (AJCC) guidelines. Neoadjuvant therapy in the present study conformed to “The Standard for Diagnosis and Treatment of Chinese Colorectal Cancer” and its revised version. Whether employing a short-course neoadjuvant therapy approach (administering 5 Gy × 5 fractions to the primary tumor and high-risk areas) or a long-course neoadjuvant therapy approach (administering a total radiation dose of 45.0–50.4 Gy to the primary tumor and high-risk areas, with each fraction ranging from 1.8 to 2.0 Gy, totaling 25–28 fractions, and concurrently administering 5-fluorouracil (5-FU) or capecitabine monotherapy during radiotherapy), both were categorized as preoperative neoadjuvant therapy. Neoadjuvant treatment, length of the removed bowel, pathological stage of the tumor, ASA, operation time, tumor obstruction and tumor size were associated with the primary surgery, whereas age, BMI and the interval between stoma creation and closure were associated with the stoma closure surgery.
Evaluation of bowel function
The Chinese version of the LARS questionnaire was used to evaluate the intestinal function of patients. It is a proven short and effective tool for assessing intestinal function after rectal cancer surgery (13,21), including the five most prominent aspects of intestinal dysfunction, such as gas incontinence, liquid fecal incontinence, frequency, clustering and urgency. According to the scoring criteria, the following score was assigned based on LARS severity: 0–20 points without LARS; 21–29 points for minor LARS; and 30–42 points for major LARS. To accurately evaluate bowel function (22,23), the eligible patients were followed up using the LARS score questionnaire by phone, text message, outpatient or hospitalization 1 year after diverting stoma closure. To highlight major LARS, patients with major LARS were classified into one group, while patients without and with minor LARS were into another group.
Model development
The collected patient data were randomly divided into a training (70%, n=277) and a test set (30%, n=119). An ensemble learning random forest (RF) classifier was trained with 14 input features in the training set to calculate the probability of developing major LARS in patients with rectal cancer after diverting stoma closure. During the training of the model, the probability (Youden index) to balance the sensitivity and specificity of the receiver operating characteristic (ROC) curves was found to divide the continuous probability generated by RF into the predicted major LARS and non-major LARS groups. If the probability value exceeded the Youden index, the patient would be considered as “major LARS”, thus avoiding the low actual positive rate or high false positive rate caused by the default cutoff value of 0.5 and resolving the low sensitivity or specificity of the prediction results of the model. In addition, to further evaluate the performance of the model, positive predictive values (PPV), negative predictive values (NPV), accuracy, the area under the ROC curves (AUC) and area under the precision-recall curves (AUPRC), Brier score and concordance index (C-index) of the model were calculated. To compare machine learning models with traditional linear models, we additionally constructed a logistic regression (LR) model. We calculated the AUC, sensitivity, specificity, positive predictive PPV, and NPV of the LR model in the training set to evaluate its predictive ability.
Model validation
To test the discrimination power of the Youden index, the latter was used to validate the model in the test set and obtain the accuracy, specificity, sensitivity, PPV, NPV, AUC, AUPRC, Brier score and C-index of the model. The AUC and C-index were used to evaluate the ability of the model to discriminate major LARS after diverting stoma closure. The calibration degree of the model was assessed using the calibration curve and the Brier score (24). The Brier score is always between 0 and 1, and the closer to 0 the better the calibration of the model and vice versa. Accuracy, specificity, sensitivity, PPV, NPV and AUPRC were used to evaluate the accuracy of the model.
Feature importance analysis
The influence of each feature on the prediction of major LARS was evaluated using the SHapley Additive exPlanations (SHAP) method (25,26). The SHAP method has proven to be a valuable tool for interpreting the predictions of machine learning models and can enhance our understanding of the underlying mechanisms that drive the model’s predictions by estimating the contribution of each feature to the model prediction outcomes. Furthermore, the importance of each feature in the training set was assessed based on the contributions to the model output. By ranking the features according to their importance, we were able to identify the most influential factors in the prediction of major LARS.
Model utility
Decision curve analysis (DCA) was used to evaluate the potential clinical net benefits of the model under various thresholds and further verify the clinical application value (27). DCA examined the relative effects of false negatives (underdiagnosed major LARS) and false positives (misdiagnosed major LARS) on predictive outcomes in a range of threshold probabilities. This approach allows for a more thorough comprehension of the clinical impact of a predictive model and can aid in guiding clinical decision-making.
Statistical analysis
Continuous variables are expressed as mean ± standard deviation, whereas categorical variables are expressed as counts (percentage). One-way analysis of variance (ANOVA) or nonparametric Mann-Whitney U test was adopted to compare continuous variables between two groups. The χ2 test was used to compare categorical variables between two groups. The tests were two-tailed and P<0.05 was considered to indicate a statistically significant difference. Statistical analysis was performed using SPSS 27.0 (IBM Corp.) and the online tool Vassar Stats (http://vassarstats.net/index.html). All machine learning algorithms were implemented using Python version 3.9.7 (https://www.python.org/) and the Scikit-learn package version 0.24.2 (https://scikit-learn.org).
Results
Participants
The queue selection process is shown in Figure 1. The present study included 396 eligible patients diagnosed as mid-low rectal cancer, with an average anastomotic height of 3.6 cm (range, 1 to 10 cm). The age of the patients ranged from 26 to 82 years, with a mean age of 56 years; there were 154 female patients (38.9%). A total of 162 patients with diverting stoma developed major LARS within 1 year after diverting stoma closure, with an incidence of 40.9% (41.2% and 40.3% in the training and test set, respectively). The baseline characteristics of the two groups are shown in Table 1.
Table 1
Variables | Training cohort (n=277) | Test cohort (n=119) | P value |
---|---|---|---|
Age (years) | 56.22±10.2 | 56.68±9.8 | 0.67 |
Male | 164 (59.2) | 78 (65.5) | 0.14 |
BMI (kg/m2) | 22.83±2.8 | 23.12±2.9 | 0.35 |
Neoadjuvant | 48 (17.3) | 12 (10.1) | 0.06 |
Hypertension | 54 (19.5) | 31 (26.1) | 0.09 |
Diabetes | 18 (6.5) | 13 (10.9) | 0.09 |
Tumor obstruction | 8 (2.9) | 7 (5.9) | 0.12 |
Anastomotic height (cm) | 3.61±1.61 | 3.62±1.56 | 0.95 |
Interval surgery-closing stoma (days) | 147.44±112.4 | 144.40±134.6 | 0.81 |
ASA | 0.06 | ||
1 | 43 (15.5) | 22 (18.5) | |
2 | 223 (80.5) | 86 (72.3) | |
3 | 11 (4.0) | 11 (9.2) | |
Operation time (min) | 211.96±53.2 | 208.78±57.2 | 0.59 |
Specimen length (cm) | 10.82±3.0 | 11.03±3.2 | 0.54 |
Tumor size (cm) | 3.45±1.34 | 3.39±1.3 | 0.64 |
Stage | 0.72 | ||
1 | 104 (26.3) | 41 (34.5) | |
2 | 84 (30.3) | 35 (29.4) | |
3 | 89 (32.1) | 43 (36.1) | |
LARS | 0.48 | ||
Minor/no | 163 (58.8) | 71 (59.7) | |
Major | 114 (41.2) | 48 (40.3) |
Data are presented as mean ± SD or n (%). BMI, body mass index; ASA, American society of Anesthesiologists classification; LARS, low anterior resection syndrome; SD, standard deviation.
Model performance
In the training set, 14 clinicopathological factors were used as input features and major LARSs were used as the target to train the machine learning RF model. First, the probability of developing major LARS in patients with rectal cancer after stoma closure was calculated. These continuous probabilities generated by the RF algorithm were significantly correlated with the occurrence of major LARS in the training set (Figure 2A, P<0.001) and the best cutoff value of the Youden index was 0.450 (Figure 2B). When the probability exceeded the Youden index, the patients were classified as major LARS group. The AUC and AUPRC were 0.781 and 0.728, respectively (Figure 2C,2D). In addition, the classification results based on this cut-off value are shown in Figure 2E. Figure 2F shows the sensitivity, specificity, PPV, NPV and accuracy of the model in the training set. The Brier score and C-index of the model were 0.20 and 0.75, respectively (Table 2). Compared with the LR model, the RF classifier exhibited superior performance across the multiple evaluation metrics in the training set, including AUC, AUPRC, Brier score, C-index, sensitivity, PPV, NPV and accuracy (Figure 3A,3B and Table 2; AUC 0.78 for RF versus 0.75 for LR; AUPRC 0.73 for RF versus 0.71 for LR; Brier 0.20 for RF versus 0.21 for LR; C-index 0.75 for RF versus 0.70 for LR; sensitivity 0.66 for RF versus 0.62 for LR; PPV 0.63 for RF versus 0.61 for LR; NPV 0.75 for RF versus 0.73 for LR; accuracy 0.70 for RF versus 0.68 for LR). These results demonstrated that the trained RF model showed good discrimination and calibration.
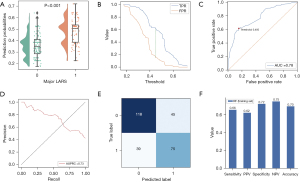
Table 2
Variables | Training set (n=277) | Test set (n=119) | |
---|---|---|---|
RF | LR | ||
Sensitivity (95% CI) | 0.66 (0.56–0.74) | 0.62 (0.53–0.71) | 0.67 (0.51–0.79) |
Specificity (95% CI) | 0.72 (0.65–0.79) | 0.72 (0.65–0.79) | 0.66 (0.54–0.77) |
PPV (95% CI) | 0.63 (0.53–0.71) | 0.61 (0.52–0.70) | 0.57 (0.43–0.70) |
NPV (95% CI) | 0.75 (0.68–0.82) | 0.73 (0.66–0.80) | 0.75 (0.62–0.84) |
Accuracy (95% CI) | 0.70 (0.64–0.75) | 0.68 (0.62–0.74) | 0.66 (0.57–0.75) |
AUC (95% CI) | 0.78 (0.74–0.83) | 0.75 (0.70–0.79) | 0.74 (0.70–0.79) |
AUPRC | 0.73 | 0.71 | 0.69 |
Brier | 0.20 | 0.21 | 0.20 |
C-index | 0.75 | 0.70 | 0.72 |
RF, random forest; LR, logistic regression; CI, confidence interval; PPV, positive predictive value; NPV, negative predictive value; AUC, area under the receiver operating characteristic curve; AUPRC, area under the precision-recall curve; C-index, concordance index.
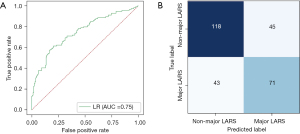
Model assessment
In the test set, the probabilities generated by the RF model were significantly associated with major LARS (Figure 4A). The model achieved satisfactory performance, with AUC and AUPRC of 0.74 (95% CI: 0.70–0.79) and 0.69, respectively (Figure 4B,4C). In addition, the model had a Brier score and C-index of 0.21 and 0.70, respectively (Table 2). The classification results of the model are shown in Figure 4D. Sensitivity (0.67; 95% CI: 0.51–0.79), specificity (0.66; 95% CI: 0.54–0.77), PPV (0.57; 95% CI: 0.43–0.70) and NPV (0.75; 95% CI: 0.62–0.84) and accuracy (0.66; 95% CI: 0.57–0.75) are shown in Figure 4E and Table 2. The concordance between the model’s predictions results and the observed values was evaluated by plotting calibration curves (Figure 4F). Briefly, in the test set, the RF model still showed good discrimination and calibration.
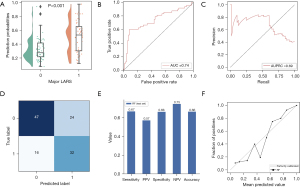
Feature importance
Figure 5A illustrates the relationship between each patient characteristic and the major LARS, as determined by the feature importance analysis. To evaluate the impact of individual patient features on major LARS, we employed the SHAP value method and assigned importance values to each feature, as presented in Figure 5B. Moreover, in Figure 5C, the ranking of feature importance is presented, emphasizing the salient patient characteristics correlated with major LARS. The analysis identified the interval (days) between stoma creation and closure, neoadjuvant therapy, and BMI as the foremost three significant patient characteristics. Figure S1 further illustrates the disparities in the intervals between stoma creation and closure within the major and non-major LARS cohorts, as well as the variations in neoadjuvant therapy status and BMI. Additionally, the intervals between stoma creation and closure were stratified into three distinct categories: ≤30, >30 to <60, and ≥60 days. In our study population, there was no statistically significant difference in the incidence of major LARS after the diverting stoma closure between the ≤30 and >30 to <60 days groups (P=0.30). Nevertheless, the incidence of major LARS after the diverting stoma closure was significantly higher within the ≥60 days group compared to the >30 to <60 days group (P<0.001), as showed in Figure S2.
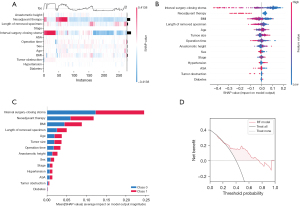
Clinical application value
Finally, the DCA results indicated that our model had good clinical application value, as shown in Figure 5D. Specifically, within the threshold probability range of 0.2–0.8, patients would benefit from comparing all or no treatments using the model. The DCA results provide evidence that our model can be a helpful tool for personalized treatment decision-making within this range in patients with rectal cancer who are considering stoma closure.
Discussion
The present study constructed a predictive model for major LARS after diverting stoma closure based on a supervised machine-learning RF classifier. Thirteen clinicopathological features were imputed to predict the risk probability of major LARS in patients with rectal cancer after stoma closure. The model demonstrated good discrimination, calibration and clinical application value. Furthermore, the study identified the top five crucial features affecting the predictive results of the model, including the interval between creation and stoma closure, neoadjuvant therapy, BMI, the length of removed bowel, and age.
Creating a diverting stoma to protect anastomosis is considered standard practice by most rectal surgeons after anterior resection or low anterior resection for rectal cancer, especially in patients with middle and low rectal cancer (28). Recent analysis has shown an increase in the proportion of diverting stomas due to an increase in sphincter-preserving surgery (29). Snijders et al. (30) found that the proportion of diverting stomas increased from 57% in 1999 to 70% in 2010 and creating diverting stomas significantly reduced the incidence of symptomatic anastomotic leakage and the associated mortality (31,32). However, bowel dysfunction after stoma closure in patients with rectal cancer is common and significantly impacts the quality of life because diverting stoma interrupts intestinal function, leading to diverting colitis (33). Taylor and Bradshaw (15) found that patients with rectal cancer have frequent intestinal movement, urgency and fecal fragmentation and incontinence after diverting stoma closure. Changes in bowel function restrict the daily life and work routine of the patients, affecting their mental and physical health and thus altering their expectations after diverting stoma closure. Jansen et al. (34) reported that bowel dysfunction damaged the quality of life of the patient within 1 year after stoma closure. Similarly, Engel et al. (35) pointed out in a 4-year prospective study that bowel dysfunction, such as diarrhea, urgency and fecal incontinence, significantly impacted the quality of life of postoperative patients with rectal cancer.
Given the decline in patient expectations after diverting stoma closure (36), it is crucial to inform patients of the risk of postoperative bowel dysfunction before diverting stoma closure. Although several previous studies have attempted to develop predictive models for LARS following rectal cancer surgery, these models have only a moderate ability to discriminate major LARS due to various methodological limitations. For example, Paku et al. (37) established a nomogram model to predict major LARS based on the traditional LR algorithm, but the model did not include patients after diverting stoma closure. The pre-operative LARS score tool developed and externally validated by Battersby et al. (38) to predict bowel dysfunction following restorative rectal cancer resection was also based on the traditional regression algorithm. However, Essangri et al. (39) observed that when applied to other populations, this tool showed a Kendall coefficient of 0.433 and a Kappa coefficient of 0.327, which indicated weak concordance. Moreover, the comparison of the predicted and actual LARS scores, by category and degree of agreement, resulted in only 18.09% perfect agreement for major LARS. In summary, the moderate discriminatory accuracy of these models is attributable to the conventional regression algorithm’s dependence on a linear correlation between prediction variables and predicted outcomes (39), which may not hold true for multidimensional clinical variables (16).
The current study developed a machine learning algorithm that accurately predicts the risk of major LARS in patients with rectal cancer after stoma closure (AUC, 0.75; 95% CI: 0.69–0.79). Patients with diverting stomas should benefit from the model, according to DCA. The predictive factors included in the model were reported in previous literature, such as the interval between the creation and stoma closure, neoadjuvant therapy, anastomotic height, age, sex, and BMI (40-42). In addition, our model identified several pre-operative variables that were significantly associated with post-operative bowel function recovery. These variables include the length (cm) of the removed bowel, pathological stage of tumor, ASA classification, hypertension, diabetes, operation time, tumor obstruction and tumor size. Of these variables, the interval between the creation and stoma closure was the most important predictor of post-operative bowel function recovery. The distance between the anastomosis and the anal margin was included because a diverting stoma was always performed in patients with mid-low rectal cancer who have risk factors for anastomotic leakage. The model’s clinical utility was evaluated, and the DCA indicated that the trained model would be helpful in clinical practice. The predictive model may improve patient outcomes by facilitating more informed and personalized surgical decision-making, reducing the risk of postoperative complications, and ultimately improving patient satisfaction.
The present study features several limitations. First, like for any other single-center retrospective observational study, information and selection biases may exist. Second, the model needs to be validated by external datasets at different times and locations. Third, assessing the surgical impact on the anal sphincter and pelvic plexus, including the degree of damage, presented challenges. Additionally, obtaining precise data on patients’ self-administered medications to improve bowel function, along with dosages and compliance with pelvic floor rehabilitation exercises, proved to be significantly challenging. Finally, the generalization ability of the model may need to be improved. Our study only included patients with colorectal cancer who underwent prophylactic stoma closure, which may limit the applicability of the predictive model to other patient populations. Although the model included 14 predictors, these may still be a relatively small number. To overcome these limitations, a multicenter prospective study will be performed to further evaluate the clinical impact of using the present model. Additionally, further dedicated prospective studies are required to explicitly elucidate the relationship between diverting stoma, LARS, and the timing of closure.
Conclusions
In conclusion, our study successfully developed a machine learning model that can predict major LARS in rectal cancer patients before diverting stoma closure. The model demonstrated reliability and has the potential to aid patients in making informed decisions about diverting stoma closure. The personalized estimates provided by the model can help clinicians guide patients in their surgical management and improve their understanding of the potential outcomes. Moreover, this model can be used to identify patients at high risk of poor bowel function recovery, enabling clinicians to provide targeted post-operative care to improve their outcomes.
Acknowledgments
I would like to thank all the medical staff of the Gastrointestinal Surgery Department of Tongji Hospital for providing convenient conditions for implementing this study.
Funding: This work was supported by
Footnote
Reporting Checklist: The authors have completed the TRIPOD reporting checklist. Available at https://jgo.amegroups.com/article/view/10.21037/jgo-23-1019/rc
Data Sharing Statement: Available at https://jgo.amegroups.com/article/view/10.21037/jgo-23-1019/dss
Peer Review File: Available at https://jgo.amegroups.com/article/view/10.21037/jgo-23-1019/prf
Conflicts of Interest: All authors have completed the ICMJE uniform disclosure form (available at https://jgo.amegroups.com/article/view/10.21037/jgo-23-1019/coif). The authors have no conflicts of interest to declare.
Ethical Statement: The authors are accountable for all aspects of the work in ensuring that questions related to the accuracy or integrity of any part of the work are appropriately investigated and resolved. The study was conducted in accordance with the Declaration of Helsinki (as revised in 2013) and approved by Ethics Committee of the Tongji Hospital, Huazhong University of Science and Technology (No. TJ-IRB20230364). The requirement for informed consent was waived due to the retrospective nature of the study.
Open Access Statement: This is an Open Access article distributed in accordance with the Creative Commons Attribution-NonCommercial-NoDerivs 4.0 International License (CC BY-NC-ND 4.0), which permits the non-commercial replication and distribution of the article with the strict proviso that no changes or edits are made and the original work is properly cited (including links to both the formal publication through the relevant DOI and the license). See: https://creativecommons.org/licenses/by-nc-nd/4.0/.
References
- Myrseth E, Nymo LS, Gjessing PF, et al. Diverting stomas reduce reoperation rates for anastomotic leak but not overall reoperation rates within 30 days after anterior rectal resection: a national cohort study. Int J Colorectal Dis 2022;37:1681-8. [Crossref] [PubMed]
- Schlesinger NH, Smith H. The effect of a diverting stoma on morbidity and risk of permanent stoma following anastomotic leakage after low anterior resection for rectal cancer: a nationwide cohort study. Int J Colorectal Dis 2020;35:1903-10. [Crossref] [PubMed]
- Tsujinaka S, Suzuki H, Miura T, et al. Diagnosis, Treatment, and Prevention of Ileostomy Complications: An Updated Review. Cureus 2023;15:e34289. [Crossref] [PubMed]
- Omar M, Tarek A, Abdeslam B, et al. Risk factors of acute renal failure in patients with protective ileostomy after rectal cancer surgery. BMC Surg 2023;23:107. [Crossref] [PubMed]
- Rud CL, Baunwall SMD, Bager P, et al. Patient-Reported Outcomes and Health-Related Quality of Life in People Living With Ileostomies: A Population-Based, Cross-Sectional Study. Dis Colon Rectum 2022;65:1042-51. [Crossref] [PubMed]
- Babakhanlou R, Larkin K, Hita AG, et al. Stoma-related complications and emergencies. Int J Emerg Med 2022;15:17. [Crossref] [PubMed]
- Keane C, Sharma P, Yuan L, et al. Impact of temporary ileostomy on long-term quality of life and bowel function: a systematic review and meta-analysis. ANZ J Surg 2020;90:687-92. [Crossref] [PubMed]
- Ge Z, Zhao X, Liu Z, et al. Complications of preventive loop ileostomy versus colostomy: a meta-analysis, trial sequential analysis, and systematic review. BMC Surg 2023;23:235. [Crossref] [PubMed]
- Denost Q, Sylla D, Fleming C, et al. A phase III randomized trial evaluating the quality of life impact of a tailored versus systematic use of defunctioning ileostomy following total mesorectal excision for rectal cancer-GRECCAR 17 trial protocol. Colorectal Dis 2023;25:443-52. [Crossref] [PubMed]
- Tsunoda A, Tsunoda Y, Narita K, et al. Quality of life after low anterior resection and temporary loop ileostomy. Dis Colon Rectum 2008;51:218-22. [Crossref] [PubMed]
- Park J, Angenete E, Bock D, et al. Cost analysis in a randomized trial of early closure of a temporary ileostomy after rectal resection for cancer (EASY trial). Surg Endosc 2020;34:69-76. [Crossref] [PubMed]
- Alves A, Panis Y, Lelong B, et al. Randomized clinical trial of early versus delayed temporary stoma closure after proctectomy. Br J Surg 2008;95:693-8. [Crossref] [PubMed]
- Emmertsen KJ, Laurberg S. Low anterior resection syndrome score: development and validation of a symptom-based scoring system for bowel dysfunction after low anterior resection for rectal cancer. Ann Surg 2012;255:922-8. [Crossref] [PubMed]
- Keane C, Fearnhead NS, Bordeianou LG, et al. International Consensus Definition of Low Anterior Resection Syndrome. Dis Colon Rectum 2020;63:274-84. [Crossref] [PubMed]
- Taylor C, Bradshaw E. Tied to the toilet: lived experiences of altered bowel function (anterior resection syndrome) after temporary stoma reversal. J Wound Ostomy Continence Nurs 2013;40:415-21. [Crossref] [PubMed]
- Rajkomar A, Dean J, Kohane I. Machine Learning in Medicine. N Engl J Med 2019;380:1347-58. [Crossref] [PubMed]
- Mitsala A, Tsalikidis C, Pitiakoudis M, et al. Artificial Intelligence in Colorectal Cancer Screening, Diagnosis and Treatment. A New Era. Curr Oncol 2021;28:1581-607. [Crossref] [PubMed]
- Shao S, Liu L, Zhao Y, et al. Application of Machine Learning for Predicting Anastomotic Leakage in Patients with Gastric Adenocarcinoma Who Received Total or Proximal Gastrectomy. J Pers Med 2021;11:748. [Crossref] [PubMed]
- Shao S, Zhao Y, Lu Q, et al. Artificial intelligence assists surgeons' decision-making of temporary ileostomy in patients with rectal cancer who have received anterior resection. Eur J Surg Oncol 2023;49:433-9. [Crossref] [PubMed]
- Topol EJ. High-performance medicine: the convergence of human and artificial intelligence. Nat Med 2019;25:44-56. [Crossref] [PubMed]
- Hou XT, Pang D, Lu Q, et al. Validation of the Chinese version of the low anterior resection syndrome score for measuring bowel dysfunction after sphincter-preserving surgery among rectal cancer patients. Eur J Oncol Nurs 2015;19:495-501. [Crossref] [PubMed]
- Nguyen TH, Chokshi RV. Low Anterior Resection Syndrome. Curr Gastroenterol Rep 2020;22:48. [Crossref] [PubMed]
- Afshari K, Smedh K, Wagner P, et al. Risk factors for developing anorectal dysfunction after anterior resection. Int J Colorectal Dis 2021;36:2697-705. [Crossref] [PubMed]
- Yang W, Jiang J, Schnellinger EM, et al. Modified Brier score for evaluating prediction accuracy for binary outcomes. Stat Methods Med Res 2022;31:2287-96. [Crossref] [PubMed]
- Nohara Y, Matsumoto K, Soejima H, et al. Explanation of machine learning models using shapley additive explanation and application for real data in hospital. Comput Methods Programs Biomed 2022;214:106584. [Crossref] [PubMed]
- Rodríguez-Pérez R, Bajorath J. Interpretation of machine learning models using shapley values: application to compound potency and multi-target activity predictions. J Comput Aided Mol Des 2020;34:1013-26. [Crossref] [PubMed]
- Vickers AJ, van Calster B, Steyerberg EW. A simple, step-by-step guide to interpreting decision curve analysis. Diagn Progn Res 2019;3:18. [Crossref] [PubMed]
- Hamabe A, Ito M, Nishigori H, et al. Preventive effect of diverting stoma on anastomotic leakage after laparoscopic low anterior resection with double stapling technique reconstruction applied based on risk stratification. Asian J Endosc Surg 2018;11:220-6. [Crossref] [PubMed]
- Grupa VEM, Kroon HM, Ozmen I, et al. Current practice in Australia and New Zealand for defunctioning ileostomy after rectal cancer surgery with anastomosis: Analysis of the Binational Colorectal Cancer Audit. Colorectal Dis 2021;23:1421-33. [Crossref] [PubMed]
- Snijders HS, van den Broek CB, Wouters MW, et al. An increasing use of defunctioning stomas after low anterior resection for rectal cancer. Is this the way to go? Eur J Surg Oncol 2013;39:715-20. [Crossref] [PubMed]
- Gadan S, Brand JS, Rutegård M, et al. Defunctioning stoma and short- and long-term outcomes after low anterior resection for rectal cancer-a nationwide register-based cohort study. Int J Colorectal Dis 2021;36:1433-42. [Crossref] [PubMed]
- Yang S, Lin Y, Zhong W, et al. Impact of ileostomy on postoperative wound complications in patients after laparoscopic rectal cancer surgery: A meta-analysis. Int Wound J 2023; Epub ahead of print. [Crossref] [PubMed]
- Dilke S, Segal J, Tozer PJ, et al. Diversion colitis: aetiology, diagnosis and treatment. A systematic review. GastroHep 2020;2:266-71. [Crossref]
- Jansen L, Herrmann A, Stegmaier C, et al. Health-related quality of life during the 10 years after diagnosis of colorectal cancer: a population-based study. J Clin Oncol 2011;29:3263-9. [Crossref] [PubMed]
- Engel J, Kerr J, Schlesinger-Raab A, et al. Quality of life in rectal cancer patients: a four-year prospective study. Ann Surg 2003;238:203-13. [Crossref] [PubMed]
- Siassi M, Hohenberger W, Lösel F, et al. Quality of life and patient's expectations after closure of a temporary stoma. Int J Colorectal Dis 2008;23:1207-12. [Crossref] [PubMed]
- Paku M, Miyoshi N, Fujino S, et al. Development and evaluation of a Japanese prediction model for low anterior resection syndrome after rectal cancer surgery. BMC Gastroenterol 2022;22:239. [Crossref] [PubMed]
- Battersby NJ, Bouliotis G, Emmertsen KJ, et al. Development and external validation of a nomogram and online tool to predict bowel dysfunction following restorative rectal cancer resection: the POLARS score. Gut 2018;67:688-96. [PubMed]
- Essangri H, Majbar MA, Benkabbou A, et al. Do we have enough Foreknowledge to predict the low anterior resection syndrome (LARS) score preoperatively? Colorectal Dis 2020;22:1445. [Crossref] [PubMed]
- Hughes DL, Cornish J, Morris C, et al. Functional outcome following rectal surgery-predisposing factors for low anterior resection syndrome. Int J Colorectal Dis 2017;32:691-7. [Crossref] [PubMed]
- Nicotera A, Falletto E, Arezzo A, et al. Risk factors for Low Anterior Resection Syndrome (LARS) in patients undergoing laparoscopic surgery for rectal cancer. Surg Endosc 2022;36:6059-66. [Crossref] [PubMed]
- Liang Z, Zhang Z, Wu D, et al. Effects of Preoperative Radiotherapy on Long-Term Bowel Function in Patients With Rectal Cancer Treated With Anterior Resection: A Systematic Review and Meta-analysis. Technol Cancer Res Treat 2022;21:15330338221105156. [Crossref] [PubMed]