Metabolomics for early pancreatic cancer detection in plasma samples from a Swedish prospective population-based biobank
Highlight box
Key findings
• Our top candidate diagnostic metabolite biomarkers were indoleacetate, 3-hydroxydecanoate (10:0-OH), and an unidentified metabolite with retention index (RI): 2,745.4. These three metabolites were combined with clinical variables and carbohydrate antigen 19-9 (CA 19-9) into a final logistic regression model that outperformed clinical variables and CA 19-9 alone.
• Seventeen metabolites analyzed in pre-diagnostic pancreatic cancer cases were found to correlate with survival.
What is known and what is new?
• Pancreatic cancer is a very aggressive disease and new biomarkers to enable early detection are urgently needed.
• In this study, we searched for early metabolite biomarkers by using pre-diagnostic pancreatic cancer samples collected up to 6 years before pancreatic cancer diagnosis.
What is the implication, and what should change now?
• The identified diagnostic metabolite profile could have value in early detection of pancreatic cancer but further validation in external pancreatic cancer cohorts is needed.
• Potential prognostic metabolites were identified in a pre-diagnostic cohort and should be confirmed at pancreatic cancer diagnosis.
Introduction
Pancreatic ductal adenocarcinoma (pancreatic cancer) is one of the most aggressive malignancies with a 5-year survival rate of around 12% in the United States (1). Diagnosing pancreatic cancer is challenging due to late-arising and diffuse symptoms, such as back pain, abdominal pain, weight loss, jaundice, and newly-onset diabetes. A majority of pancreatic cancer patients presents with grave metabolic alterations at diagnosis; around 60% have cachexia (2) and 86% have abnormal fasting glucose levels (3), and these metabolic alterations often precede pancreatic cancer diagnosis (4-6). This suggests that identification of specific circulating metabolic changes in pancreatic cancer may aid in early detection strategies.
Despite a suboptimal specificity and sensitivity, carbohydrate antigen 19-9 (CA 19-9) is still the most validated and clinically used pancreatic cancer biomarker today (7). Pre-diagnostic samples allow identification of molecular changes prior to cancer diagnosis. We and others have shown that CA 19-9 increases prior to pancreatic cancer diagnosis but as this occurs close to diagnosis, CA 19-9 has a limited value in early detection (8-10). Several studies analyzing metabolomics in association with pancreatic cancer risk have been performed (11-16). Two separate studies identified elevated branched chain amino acids (leucine, isoleucine, and valine) associated with increased pancreatic cancer risk (13,14), but these findings were neither replicated in five European prospective biobanks (11) nor in the Prostate, Lung, Colorectal, and Ovarian (PLCO) cohort (12). A more recent study found 12 potential circulating metabolite candidates related to pancreatic cancer risk using samples from two prospective biobanks (15). These metabolites were associated with adiposity/insulin resistance, gamma-glutamyl cycle metabolism, and subclinical pancreatic cancer. A nine-metabolic signature identified at pancreatic cancer diagnosis is currently being evaluated in a prospective setting (Deutsche Register Klinischer Studien registration ID: DRKS00010866) and in pre-diagnostic pancreatic cancer samples (17,18).
The primary aim of this study was to identify potential circulating metabolites for early pancreatic cancer detection in plasma samples from individuals that develop pancreatic cancer within 6 years. Secondary aims were to identify altered metabolites in individuals with pre-diagnostic pancreatic cancer symptoms as well as compare metabolites in future pancreatic cancer patients and controls with normal or impaired fasting glucose (IFG). Finally, the prognostic value of pre-diagnostic plasma metabolites in future pancreatic cancer patients was assessed. We present this article in accordance with the REMARK reporting checklist (available at https://jgo.amegroups.com/article/view/10.21037/jgo-23-930/rc) (19).
Methods
Pre-diagnostic pancreatic cancer cohort
We designed a nested case-control study within Northern Sweden Health and Disease Study (NSHDS) to investigate circulating metabolites in future pancreatic cancer. NSHDS consists of three sub-cohorts; Västerbotten Intervention Programme [1985–ongoing], mammography screening project [1995–2006], and the Northern Sweden Monica project [1986–2014]. Plasma samples have been frozen within one hour after sampling and stored at −80 ℃. Inclusion criterion for cases was a future pancreatic cancer diagnosis [International Classification of Diseases (ICD)-10 diagnosis code C25 by December 31st 2017] and exclusion criterion was a previous history of malignancy. One healthy control without a history of malignancy was matched for each case by sex, age (±6 years), and sample date (±6 years). However, there were two exceptions, for one case age differed fifteen years, and for another the sample date differed 9 years. We obtained information on variables such as sex, age, sample date, smoking status, fasting glucose levels, fasting status, and body mass index (BMI) from NSHDS.
Ethylenediamine tetraacetic acid (EDTA)-preserved plasma samples collected closest to pancreatic cancer diagnosis were used. The number of included plasma samples were 100 samples from future pancreatic cancer patients (collected from 82 unique individuals) and 82 controls. This selection was governed by the upper capacity limit of the metabolomics platform used (Figure 1). There were repeated samples collected from fifteen of the 82 future pancreatic cancer patients thus totaling 100 samples overall. Clinical endpoints used in this study were a pancreatic cancer diagnosis or time of death.
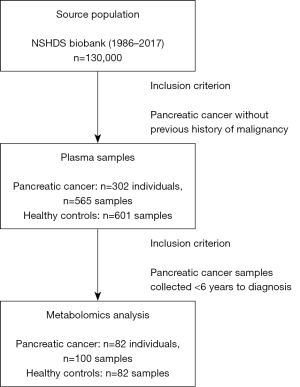
Clinical data
Information on pre-diagnostic pancreatic cancer symptoms was collected with up to 6 years prior to pancreatic cancer diagnosis by reviewing medical records from surgical, medical, orthopedic, and health care centers (general practice) using a standardized case report form. Pre-operative CA 19-9 and carcinoembryonic antigen (CEA) values were collected using the first value available following the date of first radiological pancreatic cancer finding. Symptoms reviewed, as well as inclusion and exclusion criteria for pre-diagnostic symptoms can be found in Table S1. Pre-diagnostic symptoms were divided into different lag-time intervals in relation to pancreatic cancer diagnosis date: ≤0.5, >0.5–2, >2–4, and >4–6 years. Additional clinical variables withdrawn included tumor-node-metastasis (TNM)-stage at diagnosis, surgical treatment, and survival.
Metabolite profiling
Untargeted metabolite profiling was performed at the Swedish Metabolomics Centre (Umeå, Sweden) utilizing liquid chromatography mass spectrometry (LCMS) and gas chromatography mass spectrometry (GCMS). More information can be found in supplementary methods (Appendix 1). Assays were performed blinded to the study endpoint as the tube labels did not indicate case-control status. Run order was randomized with the matched case-control pairs kept together and the order of case or control randomized as well. Some metabolic features are unidentified and thus we do not know the exact overlap of metabolites between the platforms. In this study, we use the terms ‘metabolites’ and ‘metabolic features’ synonymously.
Clinical biomarkers
In addition to the CA 19-9 and CEA values collected from medical charts in pancreatic cancer patients at diagnosis, the levels were also assessed in pre-diagnostic plasma samples using Milliplex Multiplex assays for Luminex kit Human circulating biomarker panel 1 (Merck, Darmstadt, Germany). CA 19-9, CEA, and CA 15-3 were measured in 6 µL plasma. Samples were analyzed in duplicates according to the manufacturer’s instructions. A coefficient of variation (%CV) <10 was accepted between duplicates except for samples with a value below limit of detection, where %CV ≥10 was allowed. Samples outside quantification range were quantified using an extrapolated standard curve. Samples below limit of detection were imputed with lowest value divided by 2.
Statistical analyses
Statistical analyses were performed in R Project for Statistical Computing (RRID:SCR_001905) version 4.1.1 (20). Metabolites raised by 1 and clinical biomarkers were log2-transformed and scaled to unit variance. Imputation was performed for smoking and BMI using R package mice version 3.14.0 (21). In final logistic regression models, BMI and smoking were imputed by mean values. More information can be found in supplementary methods (Appendix 1). Multiple hypothesis correction was performed using the Benjamini-Hochberg’s method (22). A false discovery rate (FDR) <0.1 was considered statistically significant. Figures were generated using R packages ggplot2 version 3.3.5 (RRID:SCR_014601) (23), ggrepel version 0.9.1 (RRID:SCR_017393) (24), and graphic design software Affinity Designer (RRID:SCR_016952) version 1.10.5 (Serif Europe Ltd., Nottinghamshire, UK).
Univariate analysis
We performed logistic regression with adjustment for matching factors sex, age, and sample storage time for each metabolite (25-28). The models were also adjusted for potential confounding variables BMI, smoking status, and fasting status. Unconditional logistic regression was the method of choice to make least absolute shrinkage and selection operator (LASSO) regression models easier to interpret. For matched cases and controls and clinical biomarkers, conditional logistic regression was also performed. Metabolites with nominal P value <0.05 were analyzed using Wilcoxon signed-rank test for longitudinal samples in fifteen pancreatic cancer patients.
LASSO regression
LASSO logistic regression was performed for variable selection using glmnet R package version 4.1-7 (RRID:SCR_015505) (29). Metabolites with a nominal P value <0.05 were included in LASSO analyses. Matching factors age, sex, and sample date were forced into each LASSO regression model. Bootstrapping with replacement, i.e., random draws of case-control sets, was repeated 500 times and the metabolites selected in ≥70% of the resampled datasets were included into a final model. Receiver operating characteristic (ROC) curves were generated using pROC R package version 1.18.0 (30). Area under curves (AUCs) were compared using DeLong’s test implemented in the roc.test function (31).
Multivariate analysis
Multivariate analyses were performed using ropls R package version 1.24.0 (RRID:SCR_016888) (32). A multivariate approach was conducted using orthogonal projections to latent structures-effect projections (OPLS-EP) for paired data as described previously (33). OPLS-EP was performed for the whole pre-diagnostic cohort (82 cases and 82 controls), symptomatic (34 cases and 34 controls), and asymptomatic cohorts (44 cases and 44 controls) by projecting metabolite differences between matched cases and controls against a dummy vector of 1. In OPLS-EP analyses, the metabolites were only scaled to unit variance none (no centering). An unpaired design was used for analyses in impaired and normal fasting glucose (NFG) cohorts, thus OPLS-discriminant analysis (OPLS-DA) was employed as multivariate method in these cohorts. Cases with and without the three most common symptoms were compared with three dummy variables (yes/no) for each symptom as outcome since the outcome of OPLS-DA is binary. To assess the significance of OPLS-EP and OPLS-DA models, analysis of variance (ANOVA) of the cross-validated residuals (CV-ANOVA) was calculated as described previously (34). If the predictive value Q2<0, the P value was set to 1.
Survival analysis
To determine the prognostic value of detected metabolites in 82 future pancreatic cancer cases, time from diagnosis to death was chosen as the time to event. We performed cox regression using survival R package (RRID:SCR_021137) version 3.3-1 (35,36) with adjustment for lag-time to diagnosis and the interaction term lag-time*metabolite. Cox regression models were also further adjusted for the potential confounders BMI, fasting status, age, sex, sample date, TNM stage, type of surgery, and smoking status. All included patients died during follow-up, thus the median follow-up was calculated by taking the median of the survival times from pancreatic cancer diagnosis to death.
Ethical statement
The study was conducted in accordance with the Declaration of Helsinki (as revised in 2013) and the ethics committee at Umeå University (Umeå, Sweden) approved the study (Dnr 09-175M, amendments 2010-191-32, 2011-320-32M, 2012-191-32M, 2013-5-32M, 2015-169-32M, and 2020-02481). Written informed consent was obtained from participants at inclusion into the Northern Sweden Health and Disease Study (NSHDS).
Results
In this study, we have performed metabolomics on pre-diagnostic plasma samples from 82 pancreatic cancer patients, collected 1.6–62.0 months before diagnosis, as well as 82 matched healthy controls (1:1) (Figure 1). A total of 408 LCMS or GCMS metabolites were included in statistical analyses. One extreme outlier was removed from the GCMS data as well as its matched control.
Clinical characteristics and pre-diagnostic biomarkers
Clinical characteristics for our pre-diagnostic cohort can be found in Table 1. Of the three clinical biomarkers analyzed, CA 19-9 was associated with an increased pancreatic cancer risk with an odds ratio (OR) of 1.52 [95% confidence interval (CI): 1.07–2.16] per standard deviation increase (FDR =0.055, Table 2).
Table 1
Variables | Pancreatic cancer cases (n=82) | Healthy controls (n=82) |
---|---|---|
Age at sampling (years) | 57.9±6.9 | 57.6±6.9 |
Female sex | 53 (64.6) | 53 (64.6) |
Follow-up time (years)1 | 2.6±1.4 | 18.6±6.2 |
Sampling year, range | 1986–2014 | 1986–2013 |
Storage time (years)2 | 18.2±6.7 | 19.4±6.2 |
Body mass index (kg/m2)2 | 26.9±4.4 | 26.1±3.8 |
Fasting status3 | ||
0–4 h | 28 (34.1) | 27 (32.9) |
>4 h | 54 (65.9) | 55 (67.1) |
Fasting blood glucose3 | ||
≥6.1 mmol/L | 19 (23.2) | 16 (19.5) |
<6.1 mmol/L | 36 (43.9) | 37 (45.1) |
Information missing | 27 (32.9) | 29 (35.4) |
Smoking status3,4 | ||
Smoker | 24 (29.3) | 15 (18.3) |
Former smoker | 19 (23.2) | 17 (20.7) |
Non-smoker/no answer | 39 (47.6) | 49 (59.8) |
Information missing | 0 | 1 (1.2) |
Age at diagnosis (years) | 60.5±6.5 | NA |
CA 19-9 at diagnosis | ||
≥37 kE/L | 17 (20.7) | NA |
<37 kE/L | 3 (3.7) | NA |
Information missing | 62 (75.6) | NA |
CEA at diagnosis | ||
≥2.5 μg/L | 29 (35.4) | NA |
<2.5 μg/L | 8 (9.8) | NA |
Information missing | 45 (54.9) | NA |
Median survival (months) | 5.7 | NA |
TNM stage at diagnosis | ||
Stage I | 7 (8.5) | NA |
Stage II | 12 (14.6) | NA |
Stage III | 12 (14.6) | NA |
Stage IV | 51 (62.2) | NA |
Surgical treatment | ||
None | 51 (62.2) | NA |
Curative intent | 13 (15.9) | NA |
Palliative intent | 16 (19.5) | NA |
Aborted surgery | 2 (2.4) | NA |
Data are presented as mean ± SD or n (%), unless otherwise specified. 1, time from blood collection to pancreatic cancer diagnosis for cases, time to end of follow-up for controls. 2, non-significant difference (Student’s t-test); 3, non-significant difference (Fisher exact test); 4, combination of sm_status and sm_yes_no variables in NSHDS. Sm_status was kept and sm_yes_no variable converted to sm_status. Occasional smoker was converted to smoker, and former occasional smoker to former smoker. NA, not applicable; CA 19-9, carbohydrate antigen 19-9; CEA, carcinoembryonic antigen; TNM, tumor-node-metastasis; SD, standard deviation; sm, smoking; NSHDS, Northern Sweden Health and Disease Study.
Table 2
Biomarker | Crude model | Adjusted model | |||||
---|---|---|---|---|---|---|---|
OR (95% CI) | P value | FDR | OR (95% CI) | P value | FDR | ||
CA 19-9 | 1.52 (1.07–2.16) | 0.02 | 0.055 | 1.70 (1.16–2.49) | 0.008 | 0.024 | |
CEA | 1.09 (0.79–1.49) | 0.61 | 0.609 | 1.04 (0.74–1.46) | 0.83 | 0.832 | |
CA 15-3 | 1.11 (0.77–1.62) | 0.58 | 0.609 | 1.09 (0.74–1.60) | 0.66 | 0.832 |
BMI, body mass index; OR, odds ratio; CI, confidence interval; FDR, false discovery rate; CA 19-9, carbohydrate antigen 19-9; CEA, carcinoembryonic antigen; CA 15-3, cancer antigen 15-3.
Pre-diagnostic metabolites
Twelve metabolites associated with pancreatic cancer risk at a nominal P value <0.05 were identified by logistic regression (Figure 2A, Figure S1, Table S2), but after multiple hypothesis correction, no metabolite remained significant. Conditional logistic regression was also performed and metabolites with a nominal P value <0.05 overlapped to some extent with metabolites identified using unconditional logistic regression (Table S3). Of the metabolites with a nominal P value <0.05 obtained from unconditional or conditional logistic regression (Tables S2,S3), tagatose levels increase closer to pancreatic cancer diagnosis (FDR =0.04) in longitudinal risk analysis (Figure S2).
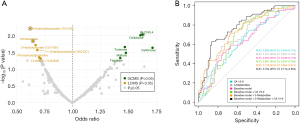
Three metabolites [indoleacetate, 3-hydroxydecanoate (10:0-OH), and retention index (RI): 2,745.4] of the 12 metabolites with a nominal P value <0.05 were selected by LASSO logistic regression in ≥70% of bootstrap iterations (Table S2). A logistic regression model including these three selected metabolites was built together with clinical covariates BMI, fasting status, smoking status, sex, sample date, and age (‘Baseline model + Metabolites’) (Figure 2B). The baseline model in combination with metabolites and CA 19-9 had a significantly better AUC (0.784, 95% CI: 0.714–0.854) than the baseline model together with CA 19-9 only (P value =0.007, AUC: 0.681, 95% CI: 0.597–0.764). No significant OPLS-EP models were detected.
Metabolites associated with pre-diagnostic symptoms
The three most reported pre-diagnostic pancreatic cancer symptoms were abdominal pain, back pain, and weight loss based on the criterion of a symptom being reported at least once up to 6 years before diagnosis (Table S4). No significant OPLS-DA model was generated for the three most common symptoms.
We reasoned that symptomatic individuals might have an affected circulating metabolite profile due to emerging symptoms arising from pancreatic cancer and thus defined a symptomatic and an asymptomatic sub-cohort. The symptomatic sub-cohort included 34 patients (at least one reported symptom as well as a plasma sample taken during the same time interval or after the symptom was reported). The asymptomatic sub-cohort consisted of 44 future pancreatic cancer cases without reported symptoms. Symptomatic (Figure 3A, Table S5) and asymptomatic future pancreatic cancer cases (Figure 3B, Table S6) were compared to their matched controls and different metabolite profiles were found. However, none of these were significant after multiple hypothesis correction and no significant OPLS-EP model was found. No difference in smoking status, BMI, age, sex, or fasting status was identified between symptomatic and asymptomatic patients (P value >0.05 by Fisher’s exact test or Student’s t-test). A total of fifteen metabolites with a nominal P value <0.05 were identified between patients with or without symptoms (Table S7).
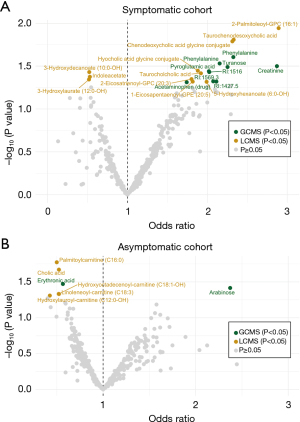
Metabolites associated with fasting glucose
Further, we aimed to identify potential biomarkers for early pancreatic cancer detection in individuals with new-onset diabetes by utilizing information on fasting glucose levels. Twenty cases (future pancreatic cancer patient) and sixteen controls had IFG, whereas 38 cases and 41 controls had NFG. Three individuals (one control, two cases) with a fasting time <4 h were excluded as well as one future pancreatic cancer patient with previously diagnosed diabetes. We identified nineteen metabolites with a nominal P value <0.05 in the IFG cohort (Figure 4A, Table S8) and seventeen in the NFG cohort (Figure 4B, Table S9). However, no metabolite was significant after adjusting for multiple testing. Furthermore, no significant OPLS-DA model was found. A total of 24 nominally altered metabolites were found between patients with or without IFG (Table S10). No difference in smoking status, BMI, age, sex, or fasting status was identified between future pancreatic cancer with impaired or NFG (P value >0.05 by Fisher’s exact test or Mann-Whitney U test).
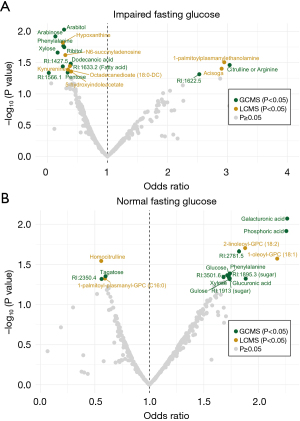
Prognostic metabolites
The end of follow-up for pancreatic cancer patients in survival analysis was the death date of the last pancreatic cancer patient (28th July 2017) and the median follow-up was 5.7 months. We identified seventeen prognostic metabolites associated with survival based on metabolite measurement in pre-diagnostic plasma samples up to 6 years before pancreatic cancer diagnosis (FDR <0.1, Figure 5, Table S11). One amino compound {5-oxoproline [hazard ratio (HR) 2.16; 95% CI: 1.38–3.40]}, two carnitines [laurylcarnitine (C12:0) (HR 2.63; 95% CI: 1.49–4.65) and linoleneoyl-carnitine (C18:3) (HR 2.53; 95% CI: 1.32–4.86)], and thirteen fatty acids [e.g., 13-HODE + 9-HODE (HR 2.77; 95% CI: 1.62–4.71), 3-hydroxydecanoate (10:0-OH) (HR 2.79; 95% CI: 1.61–4.81), and 3-hydroxypalmitate (16:0-OH) (HR 3.22; 95% CI: 1.84–5.62)] were associated with a worse prognosis. The short peptide phenylalanyltryptophan was associated with a better prognosis (HR 0.48; 95% CI: 0.28–0.81).
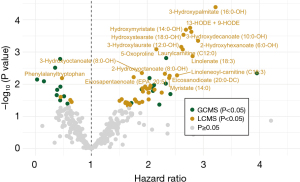
Discussion
The aim of the present study was to identify circulating metabolites that could be used for early detection of pancreatic cancer by untargeted metabolomics in a pre-diagnostic cohort. We combined three LASSO-selected metabolites [indoleacetate, 3-hydroxydecanoate (10:0-OH), and RI: 2,745.4] with baseline variables and CA 19-9 in a final logistic regression model that reached higher discriminative accuracy compared to baseline variables and CA 19-9 alone. Tagatose increases closer to diagnosis based on longitudinal analysis in fourteen future pancreatic cancer patients (FDR =0.04). We identified seventeen metabolites with prognostic potential in pancreatic cancer.
Several previous studies have found altered metabolites in pancreatic cancer at diagnosis and in pre-diagnostic cohorts. A systematic review by Long et al. [2018] included 25 studies of metabolomics in diagnostic pancreatic cancer cohorts (37). Histidine was one of the most frequently downregulated metabolites in seven out of 25 studies included. In our study, none of the metabolites comparing future pancreatic cancer cases and matched healthy controls remained significant after multiple hypothesis correction. This is in line with Fest et al. (11). Based on LASSO regression and bootstrapping of the 12 metabolites with a nominal P value <0.05, three metabolites [indoleacetate, 3-hydroxydecanoate (10:0-OH), and an unidentified metabolite; RI: 2,745.4] were most frequently selected in the LASSO bootstrap iterations (≥70%). We also found the carboxylic acid 3-hydroxydecanoate (HMDB0002203) to be associated with a worse prognosis for future pancreatic cancer patients (Figure 5, Table S11), as opposed to the lower risk for developing pancreatic cancer (OR 0.62; 95% CI: 0.44–0.87, Figure 2) (38). Increased plasma 3-hydroxydecanoate has previously been found in patients with type 2 diabetes and 3-hydroxydecanoate administration to mice induce tissue inflammation (39). The tryptophan metabolite indoleacetate (HMDB0000197) was associated with a lower risk of developing pancreatic cancer in this study (OR 0.65; 95% CI: 0.45–0.91) (38). A recent study found upregulated serum indoleacetate (indole-3-acetic acid) levels in metastatic pancreatic cancer patients who respond to chemotherapy versus non-responders (40). Tagatose (D-tagatose, HMDB0003418) is a sweetener that was not selected in ≥70% of LASSO models, but was found significantly increased in longitudinal analysis closer to pancreatic cancer diagnosis (Figure 2, FDR =0.04). D-tagatose has previously been suggested to have a potential therapeutic role in type 2 diabetes by attenuating intestinal glucose absorption (41,42).
Although not significant, we observed a drop in histidine <2 years to pancreatic cancer diagnosis, similar to Fest et al. 2019 (Figure S3) (11). Furthermore, we could not reproduce the previously reported findings of elevated circulating branched-chain amino acids (BCAAs) prior to pancreatic cancer diagnosis (13,14) (Table S12). Katagiri et al. (13) found this effect to be present in females only, but stratifying our cohort by sex did not show significantly elevated BCAA in either sex. This could be due to differences in the studied cohorts as pre-diagnostic samples can be heterogeneous with characteristics differing at diagnosis and the lag-time to diagnosis.
In early detection strategies, patients with or without symptoms would be treated differently. For symptomatic patients, it is essential with a quick and correct diagnosis to guide the treatment strategies and improve the outcome. However, for asymptomatic patients, a screening biomarker in a high-risk population may be beneficial for detecting pancreatic cancer at an early stage. A distinct strength of our study is not only the use of pre-diagnostic blood samples, but also that we obtained information of prospectively reported pre-diagnostic symptoms in the future pancreatic cancer patients. We used this information to define a pre-diagnostic symptomatic cohort with a reported pre-diagnostic symptom. This cohort was small and heterogenous since all symptoms were included. On the other hand, merging all symptoms into one cohort removes the chance of detecting metabolites associated to a specific symptom rather than the risk for developing pancreatic cancer. We compared cases to their matched controls in our symptomatic and asymptomatic cohorts but found no significantly altered metabolites after multiple hypothesis correction. New-onset diabetes is a risk factor for pancreatic cancer and fasting plasma glucose levels are impaired approximately three years prior to tumor detection (4,6). This indicates an early metabolic event that can be explored using metabolomics. Surprisingly, we found no significantly altered metabolites between pancreatic cancer patients and healthy controls with similar fasting glucose status. In general, there was a low degree of overlap between the metabolite profiles identified in the different subgroups of this study (Figure S4). This could be explained by the fact that the cohort is heterogeneous and that the metabolite profiles vary depending on which subset of samples is being analyzed. None of these metabolites were significant after adjusting for multiple testing, which makes the interpretation of the overlap difficult as we cannot be sure this is just noise or true signals.
Limitations in this study include the small cohort size and lack of an independent validation cohort. In addition, we did not include samples from other conditions such as pancreatitis, cholangitis or other cancer forms. Consequently we are unable to draw conclusions about the specificity of the identified metabolites for pancreatic cancer. Furthermore, it would be essential to validate our findings in a healthy control group to address the risk of false positives. Due to the small sample size in relation to number of metabolites studied, we refrained from splitting the cohort into train and test sets, and we emphasize that this study should be regarded as exploratory and hypothesis-generating. BMI was used as a covariate in regression models, and while obesity is a known risk factor for both diabetes and pancreatic cancer (43), BMI is an insensitive measure for obesity (44). Another limitation is that symptoms were not investigated in healthy controls, as this could not be done on the ethical permit obtained. Furthermore, we used a large window of 6 years storage time between matched cases and controls, which could impact the metabolite levels (45). We did however adjust for storage time in the regression models. Finally, prognostic metabolites were not investigated at time of pancreatic cancer diagnosis since our metabolite measurements were performed in a pre-diagnostic cohort. Lag-time is hard to translate to a clinical setting, where metabolites would instead be measured at the time of pancreatic cancer diagnosis.
Conclusions
We identified three potential metabolites [indoleacetate, 3-hydroxydecanoate (10:0-OH), and RI: 2,745.4] that predispose incident pancreatic cancer. However, these were not significant after adjustment for multiple testing, which could be due to lack of power. Continued efforts are needed to fully evaluate whether analysis of circulating metabolites may be used for early pancreatic cancer detection. Seventeen circulating metabolites were associated with pancreatic cancer survival and might have potential as prognostic biomarkers, but these should be verified in a diagnostic pancreatic cancer cohort.
Acknowledgments
The authors thank Biobanken Norr and Swedish Metabolomics Centre, Umeå, Sweden (www.swedishmetabolomicscentre.se) for performing the metabolomics analyses. We also thank Xiaoshuang Feng at International Agency for Research of Cancer, Lyon, France for assistance in statistical analyses. Parts of this article were reproduced with permission from Emmy Borgmästars’ doctoral thesis (Borgmästars E. In search of early biomarkers in pancreatic ductal adenocarcinoma using multi-omics and bioinformatics. Cityprint i Norr AB, Umeå, Sweden: Umeå University; 2022).
Funding: This work was supported by
Footnote
Reporting Checklist: The authors have completed the REMARK reporting checklist. Available at https://jgo.amegroups.com/article/view/10.21037/jgo-23-930/rc
Data Sharing Statement: Available at https://jgo.amegroups.com/article/view/10.21037/jgo-23-930/dss
Peer Review File: Available at https://jgo.amegroups.com/article/view/10.21037/jgo-23-930/prf
Conflicts of Interest: All authors have completed the ICMJE uniform disclosure form (available at https://jgo.amegroups.com/article/view/10.21037/jgo-23-930/coif). E.B. reports that travel grant was received from JC Kempe Memorial Foundation Scholarship Fund. O.F. reports that funding was obtained from Region Västerbotten, Claes Groschinsky, Bengt Ihre foundation, Swedish Society of Medicine, Lion’s Cancer Research Foundation, and Bengt Ihre Fellowship Research grant as well as travel grant from Cancerforskningsfonden i Norrland. M.S. received research grants from the Swedish Cancer Society, the Swedish Research Council, Region Västerbotten, the Sjöberg Foundation, Finska Läkaresällskapet, the Sigrid Juselius Foundation and Medicinska Understödsföreningen Liv och Hälsa. M.S. is Chairman of the research committee at the Swedish Cancer Foundation (from May 2022–present) and President of the Finnish Surgical Society (from December 2023–present). The funding agents had no role in planning or data analysis. The other authors have no conflicts of interest to declare.
Ethical Statement: The authors are accountable for all aspects of the work in ensuring that questions related to the accuracy or integrity of any part of the work are appropriately investigated and resolved. The study was conducted in accordance with the Declaration of Helsinki (as revised in 2013) and the ethics committee at Umeå University (Umeå, Sweden) approved the study (Dnr 09-175M, amendments 2010-191-32, 2011-320-32M, 2012-191-32M, 2013-5-32M, 2015-169-32M, and 2020-02481). Written informed consent was obtained from participants at inclusion into the Northern Sweden Health and Disease Study.
Disclaimer: Where authors are identified as personnel of the International Agency for Research on Cancer/World Health Organization, the authors alone are responsible for the views expressed in this article and they do not necessarily represent the decisions, policy or views of the International Agency for Research on Cancer/World Health Organization.
Open Access Statement: This is an Open Access article distributed in accordance with the Creative Commons Attribution-NonCommercial-NoDerivs 4.0 International License (CC BY-NC-ND 4.0), which permits the non-commercial replication and distribution of the article with the strict proviso that no changes or edits are made and the original work is properly cited (including links to both the formal publication through the relevant DOI and the license). See: https://creativecommons.org/licenses/by-nc-nd/4.0/.
References
- Siegel RL, Miller KD, Wagle NS, et al. Cancer statistics, 2023. CA Cancer J Clin 2023;73:17-48. [Crossref] [PubMed]
- Kordes M, Larsson L, Engstrand L, et al. Pancreatic cancer cachexia: three dimensions of a complex syndrome. Br J Cancer 2021;124:1623-36. [Crossref] [PubMed]
- Pannala R, Leirness JB, Bamlet WR, et al. Prevalence and clinical profile of pancreatic cancer-associated diabetes mellitus. Gastroenterology 2008;134:981-7. [Crossref] [PubMed]
- Jacobson S, Dahlqvist P, Johansson M, et al. Hyperglycemia as a risk factor in pancreatic cancer: A nested case-control study using prediagnostic blood glucose levels. Pancreatology 2021;S1424-3903(21)00159-9.
- Sah RP, Sharma A, Nagpal S, et al. Phases of Metabolic and Soft Tissue Changes in Months Preceding a Diagnosis of Pancreatic Ductal Adenocarcinoma. Gastroenterology 2019;156:1742-52. [Crossref] [PubMed]
- Sharma A, Smyrk TC, Levy MJ, et al. Fasting Blood Glucose Levels Provide Estimate of Duration and Progression of Pancreatic Cancer Before Diagnosis. Gastroenterology 2018;155:490-500.e2. [Crossref] [PubMed]
- Poruk KE, Gay DZ, Brown K, et al. The clinical utility of CA 19-9 in pancreatic adenocarcinoma: diagnostic and prognostic updates. Curr Mol Med 2013;13:340-51. [PubMed]
- Mason J, Lundberg E, Jonsson P, et al. A Cross-Sectional and Longitudinal Analysis of Pre-Diagnostic Blood Plasma Biomarkers for Early Detection of Pancreatic Cancer. Int J Mol Sci 2022;23:12969. [Crossref] [PubMed]
- Nolen BM, Brand RE, Prosser D, et al. Prediagnostic serum biomarkers as early detection tools for pancreatic cancer in a large prospective cohort study. PLoS One 2014;9:e94928. Erratum in: PLoS One 2015;10:e0117876. [Crossref] [PubMed]
- O'Brien DP, Sandanayake NS, Jenkinson C, et al. Serum CA19-9 is significantly upregulated up to 2 years before diagnosis with pancreatic cancer: implications for early disease detection. Clin Cancer Res 2015;21:622-31. [Crossref] [PubMed]
- Fest J, Vijfhuizen LS, Goeman JJ, et al. Search for Early Pancreatic Cancer Blood Biomarkers in Five European Prospective Population Biobanks Using Metabolomics. Endocrinology 2019;160:1731-42. [Crossref] [PubMed]
- Irajizad E, Kenney A, Tang T, et al. A blood-based metabolomic signature predictive of risk for pancreatic cancer. Cell Rep Med 2023;4:101194. [Crossref] [PubMed]
- Katagiri R, Goto A, Nakagawa T, et al. Increased Levels of Branched-Chain Amino Acid Associated With Increased Risk of Pancreatic Cancer in a Prospective Case-Control Study of a Large Cohort. Gastroenterology 2018;155:1474-1482.e1. [Crossref] [PubMed]
- Mayers JR, Wu C, Clish CB, et al. Elevation of circulating branched-chain amino acids is an early event in human pancreatic adenocarcinoma development. Nat Med 2014;20:1193-8. [Crossref] [PubMed]
- Stolzenberg-Solomon R, Derkach A, Moore S, et al. Associations between metabolites and pancreatic cancer risk in a large prospective epidemiological study. Gut 2020;69:2008-15. [Crossref] [PubMed]
- Zhong H, Liu S, Zhu J, et al. Associations between genetically predicted levels of blood metabolites and pancreatic cancer risk. Int J Cancer 2023;153:103-10. [Crossref] [PubMed]
- Mahajan UM, Oehrle B, Sirtl S, et al. Independent Validation and Assay Standardization of Improved Metabolic Biomarker Signature to Differentiate Pancreatic Ductal Adenocarcinoma From Chronic Pancreatitis. Gastroenterology 2022;163:1407-22. [Crossref] [PubMed]
- Mayerle J, Kalthoff H, Reszka R, et al. Metabolic biomarker signature to differentiate pancreatic ductal adenocarcinoma from chronic pancreatitis. Gut 2018;67:128-37. [Crossref] [PubMed]
- Sauerbrei W, Taube SE, McShane LM, et al. Reporting Recommendations for Tumor Marker Prognostic Studies (REMARK): An Abridged Explanation and Elaboration. J Natl Cancer Inst 2018;110:803-11. [Crossref] [PubMed]
- R Core Team. R: A Language and Environment for Statistical Computing. R Foundation for Statistical Computing; 2021.
- van Buuren S, Groothuis-Oudshoorn K. mice: Multivariate Imputation by Chained Equations in R. Journal of Statistical Software 2011;45:1-67. [Crossref]
- Benjamini Y, Hochberg Y. Controlling the false discovery rate: a practical and powerful approach to multiple testing. Journal of the Royal Statistical Society Series B (Methodological) 1995;289-300. [Crossref]
- Wickham H. ggplot2: Elegant Graphics for Data Analysis: Springer-Verlag New York; 2016. Available online: https://ggplot2.tidyverse.org
- Slowikowski K. ggrepel: Automatically Position Non-Overlapping Text Labels with “ggplot2”. 2021.
- McCullagh P, Nelder JA. Generalized linear models. London: Chapman and Hall; 1989.
- Dobson AJ. An Introduction to Generalized Linear Models. London: Chapman and Hall; 1990.
- Hastie TJ, Pregibon D. Generalized linear models. Statistical Models in S: Wadsworth & Brooks/Cole; 1992.
- Venables WN, Ripley BD. Modern applied statistics with S. New York: Springer; 2002.
- Friedman J, Hastie T, Tibshirani R. Regularization Paths for Generalized Linear Models via Coordinate Descent. J Stat Softw 2010;33:1-22. [Crossref] [PubMed]
- Robin X, Turck N, Hainard A, et al. pROC: an open-source package for R and S+ to analyze and compare ROC curves. BMC Bioinformatics 2011;12:77. [Crossref] [PubMed]
- DeLong ER, DeLong DM, Clarke-Pearson DL. Comparing the areas under two or more correlated receiver operating characteristic curves: a nonparametric approach. Biometrics 1988;44:837-45. [Crossref] [PubMed]
- Thévenot EA, Roux A, Xu Y, et al. Analysis of the Human Adult Urinary Metabolome Variations with Age, Body Mass Index, and Gender by Implementing a Comprehensive Workflow for Univariate and OPLS Statistical Analyses. J Proteome Res 2015;14:3322-35. [Crossref] [PubMed]
- Jonsson P, Wuolikainen A, Thysell E, et al. Constrained randomization and multivariate effect projections improve information extraction and biomarker pattern discovery in metabolomics studies involving dependent samples. Metabolomics 2015;11:1667-78. [Crossref] [PubMed]
- Eriksson L, Trygg J, Wold S. CV-ANOVA for significance testing of PLS and OPLS® models. Journal of Chemometrics 2008;22:594-600. [Crossref]
- Therneau T. A Package for Survival Analysis in R. 2022.
- Therneau T, Grambsch P. Modeling Survival Data: Extending the Cox Model. Springer, New York; 2000.
- Long NP, Yoon SJ, Anh NH, et al. A systematic review on metabolomics-based diagnostic biomarker discovery and validation in pancreatic cancer. Metabolomics 2018;14:109. [Crossref] [PubMed]
- Wishart DS, Guo A, Oler E, et al. HMDB 5.0: the Human Metabolome Database for 2022. Nucleic Acids Res 2022;50:D622-31. [Crossref] [PubMed]
- Mikkelsen RB, Arora T, Trošt K, et al. Type 2 diabetes is associated with increased circulating levels of 3-hydroxydecanoate activating GPR84 and neutrophil migration. iScience 2022;25:105683. [Crossref] [PubMed]
- Tintelnot J, Xu Y, Lesker TR, et al. Microbiota-derived 3-IAA influences chemotherapy efficacy in pancreatic cancer. Nature 2023;615:168-74. [Crossref] [PubMed]
- Donner TW, Wilber JF, Ostrowski D. D-tagatose, a novel hexose: acute effects on carbohydrate tolerance in subjects with and without type 2 diabetes. Diabetes Obes Metab 1999;1:285-91. [Crossref] [PubMed]
- Li J, Dai Q, Zhu Y, et al. Low-calorie bulk sweeteners: Recent advances in physical benefits, applications, and bioproduction. Crit Rev Food Sci Nutr 2023; Epub ahead of print. [Crossref] [PubMed]
- Eibl G, Cruz-Monserrate Z, Korc M, et al. Diabetes Mellitus and Obesity as Risk Factors for Pancreatic Cancer. J Acad Nutr Diet 2018;118:555-67. [Crossref] [PubMed]
- Romero-Corral A, Somers VK, Sierra-Johnson J, et al. Accuracy of body mass index in diagnosing obesity in the adult general population. Int J Obes (Lond) 2008;32:959-66. [Crossref] [PubMed]
- Wagner-Golbs A, Neuber S, Kamlage B, et al. Effects of Long-Term Storage at -80 °C on the Human Plasma Metabolome. Metabolites 2019;9:99. [Crossref] [PubMed]