Computer-aided diagnostic system with automated deep learning method based on the AutoGluon framework improved the diagnostic accuracy of early esophageal cancer
Highlight box
Key findings
• The computer-aided diagnosis (CAD) system based on the AutoGluon framework can assist doctors to improve the diagnostic accuracy and reading time of early esophageal cancer (EEC) under endoscopy.
What is known and what is new?
• The fusion of the deep learning method and digestive endoscopy has significantly improved the detection rate and accuracy of EEC diagnosis, and can effectively reduce the adverse effects caused by differences in physician experience and equipment.
• The CAD system based on the AutoGluon framework can help endoscopists to improve the detection rate of EEC and the accuracy of endoscopic diagnosis, and improve the efficiency of diagnosis.
What is the implication, and what should change now?
• The CAD system employed in this study exhibits superior accuracy and automation compared to other models, while also offering greater convenience and scalability. Its advantages are particularly pronounced for non-computer-proficient medical personnel.
Introduction
The incidence rate of esophageal malignant tumors is increasing year by year in China; meanwhile, most of them are diagnosed at an advanced stage (1-3). Early diagnosis and treatment of esophageal cancer can significantly prevent the progression of the disease, and some patients can even achieve a radical cure (4,5). Endoscopic examination is the main method for the diagnosis of early esophageal cancer (EEC), but it can easily lead to misdiagnosis due to the lack of specificity under endoscopy compared to other esophageal non-cancerous lesions (6). In recent years, artificial intelligence technology has been widely used in clinical diagnosis and treatment. There have been many reports of the application of artificial intelligence technology in the diagnosis and treatment of esophageal cancer in multiple related fields. Among them, the fusion of the deep learning (DL) method and digestive endoscopy has significantly improved the detection rate and accuracy of EEC diagnosis, and can effectively reduce the adverse effects caused by differences in physician experience and equipment (7,8). However, the DL method poses a great challenge to the programming ability of medical staff and has become the biggest obstacle for front-line clinical doctors without programming experience to carry out artificial intelligence projects. In 2020, the Amazon Science Department (Seattle, WA, USA) released the AutoGluon automatic machine learning framework, which can carry out image classification DL tasks under simple code. This study intends to build a computer-aided diagnosis (CAD) system for EEC endoscopy under this framework, aiming to explore the feasibility of applying automatic DL in clinical computer vision tasks. We present this article in accordance with the TRIPOD reporting checklist (available at https://jgo.amegroups.com/article/view/10.21037/jgo-24-158/rc).
Methods
Data collection
This was a retrospective study collecting endoscopic images of normal esophagus, esophagitis, and EEC from the Digestive Endoscopy Center of The First Affiliated Hospital of Soochow University (September 2015 to December 2021) and the Norwegian HyperKvasir database, including white light imaging (WLI), narrow-band imaging (NBI), and narrow-band imaging combined with magnifying endoscopy (NBI-ME); the detailed flowchart is shown in Figure 1. The study was conducted in accordance with the Declaration of Helsinki (as revised in 2013). This study was approved by the Ethics Committee of The First Affiliated Hospital of Soochow University (ethical approval number: [2022] No. 098). The requirement for informed consent was waived due to the retrospective nature of the study. In this study, EEC was defined as high-grade intraepithelial neoplasia of the esophagus or squamous cell carcinoma confined to the mucosal lining confirmed by endoscopic or surgical pathology. All images of non-cancerous esophageal lesions and EEC in this study were pathologically examined. Images which are blurred, out-of-focus, and unidentifiable, as well as images of esophageal submucosal lesions, advanced esophageal cancer, and other esophageal lesions were removed. All the screened endoscopic images of the esophagus were divided into three tasks for classification and summary as follows: task A: identification of normal esophagus and esophageal lesions (including non-cancerous esophageal lesions and EEC) under non-magnifying endoscopy; task B: identification of non-cancerous esophageal lesions and EEC under non-magnifying endoscopy; task C: identification of non-cancerous esophageal lesions and EEC under magnifying endoscopy. The classification, quantity, and source of endoscopic pictures of each item are detailed below (Table 1).
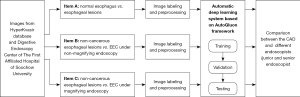
Table 1
Task | Image type | Image classification and quantity | Image source | |
---|---|---|---|---|
Endoscopy center | HK database | |||
A | WLI and NBI | Normal group: 932 | 0 | 932 |
Lesion groups: 1,092 | 429 | 663 | ||
B | WLI and NBI | Non-cancer group: 594 | 594 | 0 |
EEC group: 429 | 429 | 0 | ||
C | NBI-ME | Non-cancer group: 505 | 505 | 0 |
EEC group: 824 | 824 | 0 |
HK, HyperKvasir; WLI, white light imaging; NBI, narrow band imaging; EEC, early esophageal cancer; NBI-ME, narrow-band imaging combined with magnifying endoscopy.
Image labeling and preprocessing
According to the manifestations of esophageal lesions under endoscopy and the pathological biopsy report, three senior physicians (that is, physicians with 15 years or more experience in digestive endoscopy) reviewed, classified, and labelled all pictures in the Endoscopy Center of The First Affiliated Hospital of Soochow University according to guidelines and requirements of Table 1. At the same time, according to the classification labels provided by the HyperKvasir database, we completed the classification and labeling of the database source pictures (Figure 2). Then, all involved pictures were preprocessed as follows: (I) a training set and verification set picture division: in project A, 100 normal group pictures and 100 lesion group pictures were randomly selected as the verification set, and the remaining pictures comprised training set. In project B, 100 non-cancer group pictures and 100 EEC group pictures were randomly selected as the verification set, and the remaining pictures were used as the training set; in project C, 100 non-cancer group pictures and 100 EEC group pictures were randomly selected as the verification set, and the remaining pictures comprised the training set. (II) Picture size adjustment: the size of the original data picture were not the same, which might reduce the accuracy of the CAD system and increase the training time. All images were adjusted to 224×224 pixels. (III) Picture enhancement: the uneven sample size of the 2-category data in the training set could easily lead to bias in the model results, so the training set pictures for each project were enhanced. At the same time, the sample size of the training set increased after enhancement, which was conducive to model fitting. When enhancing, we used non-rigid image changes (flip up and down, flip left and right, rotate).
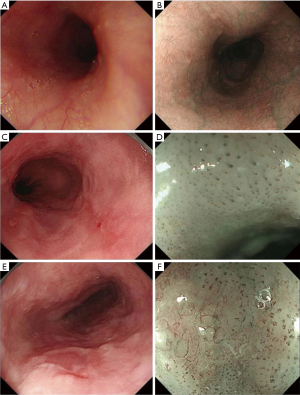
Establishment of the CAD system
The CAD system was established based on the AutoGluon framework; the steps were as follows: (I) load training data: according to the requirements of the architecture, we constructed a suitable data structure. The image data of the three tasks A, B, and C were loaded into the AutoGluon framework in the correct format; (II) the model architecture in the medium performance mode was ResNet50d, the model framework in the high-performance mode was Swin_base_patch4_window7_224, the training round was 50 rounds, and we constructed 32 training batches; the remaining parameters were replaced by the default parameters of the architecture. (III) Save the training model: we saved the high-performance and medium-performance models for each project, that was, the model with the highest accuracy in the test set.
Evaluation of the model
The CAD system was evaluated on the validation set data of each project. At the same time, two endoscopists were selected, determined as either junior or senior endoscopist (junior meant less than 5 years’ experience in digestive endoscopy, and senior meant more than 15 years’ experience in digestive endoscopy), and their interpretation results of the verification set data of each project were collected and used as a reference for model evaluation. The high-performance CAD-assisted endoscopist re-interpreted the pictures of the verification set, which was arranged 4 weeks after the endoscopist independently interpreted them.
Data processing and statistical analysis
The following evaluation indicators were used for the classification results of each project model and junior or senior physicians: accuracy, recall, precision, F1-score, interpretation time, and test subjects. The area under the receiver operating characteristic (ROC) curve (AUC) was defined. The satisfactory level of sensitivity and specificity of CAD models was defined as exceeding 50%. The software used for data analysis was SPSS 23.0 (IBM Corp., Armonk, NY, USA).
Results
The CAD system had high classification accuracy in the three tasks, and the average classification accuracy of its high-performance mode was 0.845, which was slightly lower than that of the junior endoscopists (the average classification accuracy was 0.878). There was a certain gap in the classification accuracy of senior endoscopists (the average classification accuracy was 0.930). In the high-performance mode, the CAD system had the highest classification accuracy of 0.855 in the identification of non-cancerous esophageal lesions and EEC under non-magnifying endoscopy; the highest discrimination was achieved with an AUC of 0.876 (Table 2).
Table 2
Task | Accuracy | Sensitivity | Specificity | Recall rate | Precision | F1 score | AUC | Time (s) |
---|---|---|---|---|---|---|---|---|
Task A: differentiate between normal esophagus and esophageal lesions (including non-cancerous esophageal lesions and EEC) under non-magnifying endoscopy | ||||||||
Mid-performance CAD | 0.790 | 0.810 | 0.770 | 0.810 | 0.779 | 0.794 | 0.813 | 0.026 |
High-performance CAD | 0.845 | 0.850 | 0.840 | 0.850 | 0.842 | 0.846 | 0.864 | 0.037 |
Junior endoscopist | 0.880 | 0.830 | 0.930 | 0.830 | 0.922 | 0.874 | 0.880 | 11.3 |
Senior endoscopist | 0.920 | 0.900 | 0.940 | 0.900 | 0.938 | 0.918 | 0.920 | 6.7 |
High performance CAD + junior | 0.915 | 0.880 | 0.950 | 0.880 | 0.946 | 0.912 | 0.915 | 8.7 |
High-performance CAD + seniority | 0.945 | 0.920 | 0.970 | 0.920 | 0.968 | 0.944 | 0.945 | 5.5 |
Task B: differentiate non-cancerous esophageal lesions from EEC under non-magnifying endoscopy | ||||||||
Mid-performance CAD | 0.825 | 0.820 | 0.830 | 0.820 | 0.828 | 0.824 | 0.831 | 0.028 |
High-performance CAD | 0.855 | 0.860 | 0.850 | 0.860 | 0.851 | 0.856 | 0.873 | 0.038 |
Junior endoscopist | 0.905 | 0.890 | 0.920 | 0.890 | 0.918 | 0.904 | 0.905 | 7.9 |
Senior endoscopist | 0.955 | 0.950 | 0.960 | 0.950 | 0.960 | 0.955 | 0.955 | 4.4 |
High-performance CAD + junior | 0.905 | 0.880 | 0.930 | 0.880 | 0.926 | 0.903 | 0.905 | 6.3 |
High-performance CAD + seniority | 0.960 | 0.960 | 0.960 | 0.960 | 0.960 | 0.960 | 0.960 | 3.8 |
Task C: differentiate non-cancerous esophageal lesions from EEC under magnifying endoscopy | ||||||||
Mid-performance CAD | 0.805 | 0.830 | 0.780 | 0.830 | 0.790 | 0.810 | 0.837 | 0.026 |
High-performance CAD | 0.835 | 0.840 | 0.830 | 0.840 | 0.832 | 0.836 | 0.876 | 0.035 |
Junior endoscopist | 0.850 | 0.830 | 0.870 | 0.830 | 0.865 | 0.847 | 0.850 | 9.5 |
Senior endoscopist | 0.915 | 0.910 | 0.920 | 0.910 | 0.919 | 0.915 | 0.915 | 5.6 |
High-performance CAD + junior | 0.865 | 0.860 | 0.870 | 0.860 | 0.869 | 0.864 | 0.865 | 7.7 |
High-performance CAD + seniority | 0.935 | 0.930 | 0.940 | 0.930 | 0.939 | 0.935 | 0.935 | 3.0 |
Time: the average time for interpretation of each picture in the verification set. CAD, computer-aided diagnosis; AUC, area under the ROC curve; ROC, receiver operating characteristic; EEC, early esophageal cancer.
The high-performance CAD model outperformed the junior doctors in both task A (0.850 vs. 0.830) and task C (0.840 vs. 0.830) in sensitivity comparison. In the comparison of specificity, there was still a large gap between high-performance CAD models and even low-qualified doctors. Likewise, the F1 score and the AUC for high-performance CAD models were still lower than those of junior doctors (Table 2).
In terms of time, the interpretation time of each picture in the verification set of task A with medium performance (0.026 s) and high performance (0.037 s) was much lower than that of low-level (11.3 s) and high-level (6.7 s) endoscopists. The CAD interpretation time in task B and task C was much lower than that of junior and senior endoscopists (Table 2).
After being interpreted by the high-performance CAD system, the endoscopist interpreted the pictures of the verification set again according to the results. The results showed that: in task A, the accuracy of low-seniority and high-seniority physicians were significantly improved with the assistance of CAD pre-interpretation (from 0.880 to 0.915 and from 0.920 to 0.945, respectively); at the same time, the reading time was significantly shortened (low seniority: from 11.3 to 8.7 s; senior seniority: from 6.7 to 5.5 s). In task C, the same low-seniority and high-seniority physicians have significantly improved accuracy with the assistance of CAD pre-interpretation (from 0.850 to 0.865 and from 0.915 to 0.935, respectively), and the reading time was shortened (low-seniority: from 9.5 to 7.7 s; high-seniority: from 5.6 to 3.0 s) (Table 2).
Discussion
In recent years, with the advancement of artificial intelligence and DL technologies, there have been many reports of the application of artificial intelligence technology in the diagnosis and treatment of esophageal cancer in multiple related fields, including computed tomography (CT) imaging diagnosis, surgical pathological diagnosis, and the formulation of radiotherapy plans (9-12).
Significant progress has also been made in CAD in the field of EEC endoscopic diagnosis (13). Ohmori et al. collected endoscopic images of early squamous cell carcinoma of the esophagus and used a 16-layer algorithm based on “Single Shot MultiBox Detector” to construct a DL model. The verification results confirmed that in terms of diagnostic performance, there was no significant difference between the DL system and experienced endoscopists (14). Li et al. obtained endoscopic images of early esophageal squamous cell carcinoma in China and developed a DL model using the backpropagation algorithm. The model demonstrated satisfactory sensitivity and specificity (15). de Groof et al. used 494,364 labeled endoscopic images of the esophagus to construct a classification model for EEC through the “Residual U-Net” model. The results showed that the diagnostic sensitivity and specificity of the model reached 0.90 and 0.88, respectively (16). Liu et al. utilized a dataset of 1,272 esophageal endoscopes from 748 patients to construct a convolutional neural network model consisting of two sub-networks, “O-steam” and “P-steam”. Extensive and detailed features were extracted from the sub-networks, resulting in improved diagnostic accuracy, sensitivity, and specificity compared to the traditional “LBP + SVM + HOG” model (17). In addition to the utilization of the aforementioned DL system in EEC research, ongoing investigations are being conducted on the application of various model building systems such as “Xception”, “NASNet Large”, “ResNet”, and “BigTransfer” in other domains of digestive endoscopy diagnosis (18-21). Remarkably satisfactory predictive outcomes have been achieved across all these endeavors.
However, the aforementioned DL models are associated with drawbacks such as intricate operations, high complexity, steep learning curves, manual debugging, and low accuracy. In response to this predicament, automatic learning amalgamates automation and machine learning disciplines to minimize human intervention and achieve automated machine learning; it has garnered increasing attention (22,23). Amazon has developed AutoGluon, an open-source code library for automatic machine learning that enables developers to build machine learning applications incorporating image, text, or tabular datasets. Compared with other machine learning programs, AutoGluon boasts the advantages of user-friendliness, scalability, and high accuracy (24-26). At present, good application effects have been achieved in multiple medically-related fields, such as the design and development of new drugs, government medical policy formulation, and more commonly assisting clinical physicians in evaluating patient prognosis and formulating appropriate diagnosis and treatment plans (27-29). Seo et al. utilized the AutoGluon framework to facilitate personalized rehabilitation treatment for patients with cerebrovascular accidents, yielding favorable outcomes (30). Liu et al. employed the AutoGluon DL system to predict accidental carbon monoxide poisoning prevalence and aid government agencies in formulating pertinent public health policies (31). Additionally, there has been a report on coronavirus disease 2019 (COVID-19) virus epidemiology based on the AutoGluon framework (32). Currently, there is a lack of research on the application of AutoGluon architecture automatic machine learning for classification modeling in the field of CAD of digestive endoscopy.
This study collected esophageal endoscopy images from the Digestive Endoscopy Center of The First Affiliated Hospital of Soochow University and the Norwegian HyperKvasir database and used the AutoGluon framework to establish a CAD model of EEC under endoscopy. In this study, the CAD system demonstrated its ability to differentiate between normal and diseased esophagi under non-magnifying endoscopy with an accuracy of 84.9% in high-performance mode. Its lesion detection capability is comparable to that of low-qualified endoscopists (88.0%) and slightly lower than that of senior physicians (92.0%). For the detected esophageal lesions, both non-magnifying and magnifying endoscopy demonstrate high accuracy of the CAD system in distinguishing EEC from non-cancerous lesions, with rates reaching 85.5% and 83.5%, respectively. The assistance of CAD pre-interpretation by other endoscopists can significantly improve accuracy while reducing film reading time. Therefore, the CAD system based on the AutoGluon framework can help endoscopists to improve the detection rate of EEC and the accuracy of endoscopic diagnosis, and improve the efficiency of diagnosis. The CAD system employed in this study exhibits superior accuracy and automation compared to other models, while also offering greater convenience and scalability. Its advantages are particularly pronounced for non-computer-proficient medical personnel.
There are still some deficiencies in this study: (I) the sample size of the study is relatively small. In the later stage, it is necessary to accumulate samples from more sources, incorporate multi-center endoscopic data, and introduce external test verification to further improve the accuracy of the model. (II) The classification is relatively simple. In the future, it is necessary to carry out multimodal research in combination with EEC endoscopic findings. (III) It was limited to static pictures for auxiliary diagnosis. In the later stage, it is planned to realize dynamic video-assisted diagnosis, realize real-time auxiliary diagnosis of EEC during the endoscopic examination, and meet clinical needs.
Conclusions
The CAD system based on the AutoGluon framework can assist doctors to improve the diagnostic accuracy and reading time of EEC under endoscopy. This study reveals that automatic DL methods are promising in clinical application.
Acknowledgments
Funding: This study was funded by
Footnote
Reporting Checklist: The authors have completed the TRIPOD reporting checklist. Available at https://jgo.amegroups.com/article/view/10.21037/jgo-24-158/rc
Data Sharing Statement: Available at https://jgo.amegroups.com/article/view/10.21037/jgo-24-158/dss
Peer Review File: Available at https://jgo.amegroups.com/article/view/10.21037/jgo-24-158/prf
Conflicts of Interest: All authors have completed the ICMJE uniform disclosure form (available at https://jgo.amegroups.com/article/view/10.21037/jgo-24-158/coif). The authors have no conflicts of interest to declare.
Ethical Statement: The authors are accountable for all aspects of the work in ensuring that questions related to the accuracy or integrity of any part of the work are appropriately investigated and resolved. The study was conducted in accordance with the Declaration of Helsinki (as revised in 2013). This study was approved by the Ethics Committee of The First Affiliated Hospital of Soochow University (ethical approval number: [2022] No. 098). The requirement for informed consent was waived due to the retrospective nature of the study.
Open Access Statement: This is an Open Access article distributed in accordance with the Creative Commons Attribution-NonCommercial-NoDerivs 4.0 International License (CC BY-NC-ND 4.0), which permits the non-commercial replication and distribution of the article with the strict proviso that no changes or edits are made and the original work is properly cited (including links to both the formal publication through the relevant DOI and the license). See: https://creativecommons.org/licenses/by-nc-nd/4.0/.
References
- Chen W, Zheng R, Baade PD, et al. Cancer statistics in China, 2015. CA Cancer J Clin 2016;66:115-32. [Crossref] [PubMed]
- Constantin A, Constantinoiu S, Achim F, et al. Esophageal diverticula: from diagnosis to therapeutic management-narrative review. J Thorac Dis 2023;15:759-79. [Crossref] [PubMed]
- Ju W, Zheng R, Zhang S, et al. Cancer statistics in Chinese older people, 2022: current burden, time trends, and comparisons with the US, Japan, and the Republic of Korea. Sci China Life Sci 2023;66:1079-91. [Crossref] [PubMed]
- Wander P, Tokar JL. Endoscopic management of early esophageal cancer: a literature review. Ann Esophagus 2023;6:16. [Crossref]
- Codipilly DC, Qin Y, Dawsey SM, et al. Screening for esophageal squamous cell carcinoma: recent advances. Gastrointest Endosc 2018;88:413-26. [Crossref] [PubMed]
- Frazzoni L, Arribas J, Antonelli G, et al. Endoscopists' diagnostic accuracy in detecting upper gastrointestinal neoplasia in the framework of artificial intelligence studies. Endoscopy 2022;54:403-11. [Crossref] [PubMed]
- Merchán Gómez B, Milla Collado L, Rodríguez M. Artificial intelligence in esophageal cancer diagnosis and treatment: where are we now?—a narrative review. Ann Transl Med 2023;11:353. [Crossref] [PubMed]
- Zhu C, Xie Y, Li Q, et al. CPSF6-mediated XBP1 3'UTR shortening attenuates cisplatin-induced ER stress and elevates chemo-resistance in lung adenocarcinoma. Drug Resist Updat 2023;68:100933. [Crossref] [PubMed]
- Su F, Zhang W, Liu Y, et al. The development and validation of pathological sections based U-Net deep learning segmentation model for the detection of esophageal mucosa and squamous cell neoplasm. J Gastrointest Oncol 2023;14:1982-92. [Crossref] [PubMed]
- Wu X, Wu H, Miao S, et al. Deep learning prediction of esophageal squamous cell carcinoma invasion depth from arterial phase enhanced CT images: a binary classification approach. BMC Med Inform Decis Mak 2024;24:3. [Crossref] [PubMed]
- Zou Y, Ye F, Kong Y, et al. The Single-Cell Landscape of Intratumoral Heterogeneity and The Immunosuppressive Microenvironment in Liver and Brain Metastases of Breast Cancer. Adv Sci (Weinh) 2023;10:e2203699. [Crossref] [PubMed]
- Duan Y, Wang J, Wu P, et al. AS-NeSt: A Novel 3D Deep Learning Model for Radiation Therapy Dose Distribution Prediction in Esophageal Cancer Treatment With Multiple Prescriptions. Int J Radiat Oncol Biol Phys 2023;S0360-3016(23)08239-1.
- Zhang JQ, Mi JJ, Wang R. Application of convolutional neural network-based endoscopic imaging in esophageal cancer or high-grade dysplasia: A systematic review and meta-analysis. World J Gastrointest Oncol 2023;15:1998-2016. [Crossref] [PubMed]
- Ohmori M, Ishihara R, Aoyama K, et al. Endoscopic detection and differentiation of esophageal lesions using a deep neural network. Gastrointest Endosc 2020;91:301-309.e1. [Crossref] [PubMed]
- Li B, Cai SL, Tan WM, et al. Comparative study on artificial intelligence systems for detecting early esophageal squamous cell carcinoma between narrow-band and white-light imaging. World J Gastroenterol 2021;27:281-93. [Crossref] [PubMed]
- de Groof AJ, Struyvenberg MR, van der Putten J, et al. Deep-Learning System Detects Neoplasia in Patients With Barrett's Esophagus With Higher Accuracy Than Endoscopists in a Multistep Training and Validation Study With Benchmarking. Gastroenterology 2020;158:915-929.e4. [Crossref] [PubMed]
- Liu G, Hua J, Wu Z, et al. Automatic classification of esophageal lesions in endoscopic images using a convolutional neural network. Ann Transl Med 2020;8:486. [Crossref] [PubMed]
- Hussein M, Everson M, Haidry R. Esophageal squamous dysplasia and cancer: Is artificial intelligence our best weapon? Best Pract Res Clin Gastroenterol 2021;52-53:101723. [Crossref] [PubMed]
- Cui R, Wang L, Lin L, et al. Deep Learning in Barrett's Esophagus Diagnosis: Current Status and Future Directions. Bioengineering (Basel) 2023;10:1239. [Crossref] [PubMed]
- Hosseini F, Asadi F, Emami H, et al. Machine learning applications for early detection of esophageal cancer: a systematic review. BMC Med Inform Decis Mak 2023;23:124. [Crossref] [PubMed]
- Yuan XL, Zeng XH, Liu W, et al. Artificial intelligence for detecting and delineating the extent of superficial esophageal squamous cell carcinoma and precancerous lesions under narrow-band imaging (with video). Gastrointest Endosc 2023;97:664-672.e4. [Crossref] [PubMed]
- Kamboj N, Metcalfe K, Chu CH, et al. Predicting Blood Pressure After Nitroglycerin Infusion Dose Titration in Critical Care Units: A Multicenter Retrospective Study. Comput Inform Nurs 2024;42:259-66. [Crossref] [PubMed]
- Raj R, Kannath SK, Mathew J, et al. AutoML accurately predicts endovascular mechanical thrombectomy in acute large vessel ischemic stroke. Front Neurol 2023;14:1259958. [Crossref] [PubMed]
- Kennedy EE, Davoudi A, Hwang S, et al. Identifying Barriers to Post-Acute Care Referral and Characterizing Negative Patient Preferences Among Hospitalized Older Adults Using Natural Language Processing. AMIA Annu Symp Proc 2023;2022:606-15. [PubMed]
- Jaotombo F, Adorni L, Ghattas B, et al. Finding the best trade-off between performance and interpretability in predicting hospital length of stay using structured and unstructured data. PLoS One 2023;18:e0289795. [Crossref] [PubMed]
- Lin CY, Guo SM, Lien JJ, et al. Combined model integrating deep learning, radiomics, and clinical data to classify lung nodules at chest CT. Radiol Med 2024;129:56-69. [Crossref] [PubMed]
- Tian H, Xiao S, Jiang X, et al. PASSer: fast and accurate prediction of protein allosteric sites. Nucleic Acids Res 2023;51:W427-31. [Crossref] [PubMed]
- Bo Z, Chen B, Zhao Z, et al. Prediction of Response to Lenvatinib Monotherapy for Unresectable Hepatocellular Carcinoma by Machine Learning Radiomics: A Multicenter Cohort Study. Clin Cancer Res 2023;29:1730-40. [Crossref] [PubMed]
- Stoyanova R, Katzberger PM, Komissarov L, et al. Computational Predictions of Nonclinical Pharmacokinetics at the Drug Design Stage. J Chem Inf Model 2023;63:442-58. [Crossref] [PubMed]
- Seo K, Chung B, Panchaseelan HP, et al. Forecasting the Walking Assistance Rehabilitation Level of Stroke Patients Using Artificial Intelligence. Diagnostics (Basel) 2021;11:1096. [Crossref] [PubMed]
- Liu F, Jiang X, Zhang M. Global burden analysis and AutoGluon prediction of accidental carbon monoxide poisoning by Global Burden of Disease Study 2019. Environ Sci Pollut Res Int 2022;29:6911-28. [Crossref] [PubMed]
- Sankaranarayanan S, Balan J, Walsh JR, et al. COVID-19 Mortality Prediction From Deep Learning in a Large Multistate Electronic Health Record and Laboratory Information System Data Set: Algorithm Development and Validation. J Med Internet Res 2021;23:e30157. [Crossref] [PubMed]