A machine learning clinic scoring system for hepatocellular carcinoma based on the Surveillance, Epidemiology, and End Results database
Highlight box
Key findings
• A straightforward and efficient prognostic scoring system for hepatocellular carcinoma (HCC) was devised in this study.
What is known and what is new?
• Demographic and clinical-pathological characteristics serve as pivotal influencing factors in the prognosis of HCC.
• The quantitative analysis of demographic and pathological characteristics is being applied for the first time in prognostic assessments of HCC.
What is the implication, and what should change now?
• The clinical scoring system significantly enhances the management of patients with HCC.
Introduction
Hepatocellular carcinoma (HCC), the third most common cause of cancer-related death globally, predominantly affects individuals in Asia. It is an aggressive malignancy with an unfavorable prognosis (1,2). The prognosis of HCC varies widely based on diverse risk factors. Notably, the primary risk factors for HCC that are currently recognized are hepatitis B virus (HBV) and hepatitis C virus (HCV) infections. Despite advancements in therapeutic interventions, HCC continues to exhibit a poor prognosis, especially in patients with advanced disease at the time of diagnosis.
In recent decades, the integration of information technology and the widespread use of electronic healthcare records have led to the development of several risk stratification systems in medical practice. These include the Barcelona Clinic Liver Cancer (BCLC) staging, The Chinese Society of Clinical Oncology (CSCO), Japan Society of Hepatology (JSH) staging, and the American Joint Committee on Cancer (AJCC) system (3-6). Fan et al. conducted a large cross-sectional study demonstrating that age, male gender, and parameters such as albumin-bilirubin and platelets can accurately predict HCC development (7). These systems primarily rely on biomarkers, pre-operative imaging, and post-operative pathology, which proves challenging for practical application in clinical settings, thereby hindering their widespread adoption. Conversely, clinical scoring systems are preferred in clinical practice due to their simplicity, efficiency, and ease of dissemination. Some notable scoring systems include the Child-Pugh scoring system and the Framingham risk score (8,9).
Machine learning (ML) holds significant promise in aiding clinicians in constructing a straightforward and concise model. ML solutions, such as the AutoScore framework, demonstrate superior performance with greater interpretability and accessibility compared to traditional logistic regression models. This novel ML framework facilitates the automated development of an interpretable clinical scoring system (10,11). In this study, we utilized a retrospective analysis approach to identify risk factors influencing the prognosis of HCC, encompassing overall survival (OS) and cancer-specific survival (CSS). Following this, we developed a robust clinical scoring system, to enhance the clinical management efficacy for patients with HCC. We present this article in accordance with the TRIPOD reporting checklist (available at https://jgo.amegroups.com/article/view/10.21037/jgo-24-230/rc).
Methods
Data sources and research cohort
We obtained data from the Surveillance, Epidemiology, and End Results (SEER), a network for clinical and scientific monitoring of cancer. Eligible patients were adults, aged 20 or older, diagnosed with primary HCC between 2004 and 2020. Only patients in stable condition were enrolled (excluding autopsy and death certificate reporting sources), with available follow-up information and a survival period of ≥1 month. Patients with missing or incomplete data (age, sex, race, number of tumors, T stage, N stage, M stage, surgery, radiotherapy, chemotherapy, annual median household income, Rural-urban geography, and time from diagnosis to treatment) were excluded. Ethics approval and informed consent were waived, as SEER data are freely available, and our investigation was retrospective. The study was conducted in accordance with the Declaration of Helsinki (as revised in 2013).
Demographic characteristics variables
Variables selected for inclusion in the clinical scoring system were predetermined based on clinical relevance to the assessed outcomes. Race/ethnicity categories followed SEER definitions: non-Hispanic Whites, Blacks, Hispanics, Asian/Pacific Islanders, and American Indian/Alaskan Natives. Rural-urban geographic variables, classified by population size and adjacency to metro areas, were developed by the US Department of Agriculture and Office of Management and Budget: (I) metropolitan regions with a population >1 million; (II) metropolitan regions with a population 250,000–1 million; and metropolitan regions with a population <250,000; (III) nonmetropolitan/rural regions. Annual median household income (adjusted to 2018 US dollars) was collected and estimated in a time-dependent manner using US Census American Community Survey data: <$40,000, $40,000–$69,999, and $70,000+. HCC treatments in the SEER database were analyzed using site-specific surgery variables and categorized as no surgical treatment, local regional therapy (including photodynamic therapy, alcohol, heat-radio-frequency ablation, etc.), hepatectomy, and liver transplantation. The time from diagnosis to treatment was measured in months. The pathological tumor stage was characterized according to the seventh edition of the AJCC TNM staging system.
Statistical analysis
Continuous variables were expressed as mean and standard deviation. Categorical variables were expressed as frequencies and percentages. We randomly divided all investigated cases into training and validation cohorts in a 1:1 ratio by caret R packages (12). Cox regression analysis was used to assess the components of the OS and CSS to address risk factors in the training cohort. Then, the model built using the significant risk factors was validated with the data from both the training and validation cohorts. Meanwhile, the minimum Akaikes’s Information Criterion (AIC) was utilized to select the most suitable model for data analysis (13), adjusted for clinical variables. The time-dependent receiver operating characteristic (ROC) curve (14), calibration plots, the index of concordance (C-index), and decision curve analysis (DCA) were used to compare the accuracy between the scoring system and AJCC (15). In addition, the significant risk factors were used to create the clinical score system that was automated and developed by the AutoScore R package. All analyses were conducted using R software version 4.1.1 (www.r-project.org). A two-sided P value <0.05 was considered statistically significant.
Results
Characteristics of the HCC cohort
After applying rigorous inclusion and exclusion criteria, a total of 45,827 and 39,971 HCC patients from the SEER database were included for the analysis of OS and CSS, respectively. The case selection process is illustrated in Figure 1. Among them, 22,914 patients constituted the training cohort for developing the clinical scoring system, while 22,913 patients formed the validation cohort for the same system (Table 1). Generally, the two groups exhibited balance in baseline characteristics. Approximately 77% of the patients were male, and the majority were White (49%). Despite 31,210 patients being staged I−II, the no-surgery rate was around 60%, and the no-radiotherapy rate was approximately 98%. The median follow-up time for the OS cohort was 25 months (95% CI: 25−26), while the 1-, 2-, and 3-year survival rates were 67.4% (95% CI: 67−67.9%), 50.6% (95% CI: 50.2−51.1%) and 40.8% (95% CI: 40.4−41.3%), respectively. As shown in Table 2, the median follow-up time for the CSS cohort was 26 months (95% CI: 26−27), while the 1-, 2-, and 3-year survival rates were 67.6% (95% CI: 67.2−68.1%), 51.6% (95% CI: 51.1−52.1%) and 42.4% (95% CI: 41.9−42.9%), respectively. Statistical data from most countries and regions indicated that the incidence and mortality rates of HCC in males are two to three times higher than in females (1,2).
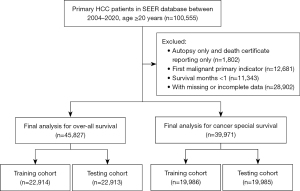
Table 1
Level | Testing (n=22,913) | Training (n=22,914) | P |
---|---|---|---|
Age (years) | 63.12±10.12 | 63.02±10.11 | 0.29 |
Sex | 0.89 | ||
Female | 5,292 (23.1) | 5,279 (23.0) | |
Male | 17,621 (76.9) | 17,635 (77.0) | |
Race | 0.92 | ||
Hispanic | 4,700 (20.5) | 4,709 (20.6) | |
American Indian/Alaska native | 249 (1.1) | 240 (1.0) | |
Asian or Pacific Islander | 4,050 (17.7) | 4,075 (17.8) | |
Black | 2,630 (11.5) | 2,680 (11.7) | |
White | 11,284 (49.2) | 11,210 (48.9) | |
Number of tumors | 1.08±0.30 | 1.07±0.30 | 0.45 |
Delayed treatment | 0.87 | ||
No | 3,911 (17.1) | 3,925 (17.1) | |
Yes | 19,002 (82.9) | 18,989 (82.9) | |
Type | 0.10 | ||
NOS | 22,651 (98.9) | 22,640 (98.8) | |
Clear cell type | 146 (0.6) | 158 (0.7) | |
Fibrolamellar | 57 (0.2) | 78 (0.3) | |
Pleomorphic type | 9 (0.0) | 4 (0.0) | |
Scirrhous | 35 (0.2) | 21 (0.1) | |
Spindle cell variant | 15 (0.1) | 13 (0.1) | |
T stage | 0.43 | ||
T1-2 | 17,232 (75.2) | 17,306 (75.5) | |
T3-4 | 5,681 (24.8) | 5,608 (24.5) | |
N stage | 0.36 | ||
N0 | 21,476 (93.7) | 21,525 (93.9) | |
N1 | 1,437 (6.3) | 1,389 (6.1) | |
M stage | 0.51 | ||
M0 | 20,395 (89.0) | 20,441 (89.2) | |
M1 | 2,518 (11.0) | 2,473 (10.8) | |
AJCC7 | 0.04 | ||
I_II | 15,501 (67.7) | 15,709 (68.6) | |
III_IV | 7,412 (32.3) | 7,205 (31.4) | |
Surgery | 0.52 | ||
None | 13,700 (59.8) | 13,577 (59.3) | |
Local tumor destruction | 4,106 (17.9) | 4,220 (18.4) | |
Hepatectomy | 3,290 (14.4) | 3,279 (14.3) | |
Liver transplant | 1,817 (7.9) | 1,838 (8.0) | |
Radiotherapy | 0.71 | ||
None | 22,390 (97.7) | 22,378 (97.7) | |
Yes | 523 (2.3) | 536 (2.3) | |
Chemotherapy | 0.22 | ||
No/unknown | 9,201 (40.2) | 9,073 (39.6) | |
Yes | 13,712 (59.8) | 13,841 (60.4) | |
Median household income | 0.20 | ||
Lower income | 426 (1.9) | 376 (1.6) | |
Median income | 10,026 (43.8) | 10,055 (43.9) | |
High income | 12,461 (54.4) | 12,483 (54.5) | |
Rural urban | 0.34 | ||
Counties | 14,285 (62.3) | 14,437 (63.0) | |
Nonmetropolitan | 1,988 (8.7) | 1,959 (8.5) | |
Metropolitan | 6,640 (29.0) | 6,518 (28.4) | |
Survival months | 33.82±38.70 | 34.20±38.94 | 0.30 |
Status | >0.99 | ||
Alive | 7,551 (33.0) | 7,552 (33.0) | |
Dead | 15,362 (67.0) | 15,362 (67.0) |
Data are expressed as mean ± SD or n (%). OS, overall survival; NOS, not specified; AJCC, American Joint Committee on Cancer; SD, standard deviation.
Table 2
Level | Testing (n=19,985) | Training (n=19,986) | P |
---|---|---|---|
Age (years) | 62.99±10.08 | 63.03±10.18 | 0.69 |
Sex | 0.38 | ||
Female | 4,579 (22.9) | 4,655 (23.3) | |
Male | 15,406 (77.1) | 15,331 (76.7) | |
Race | 0.46 | ||
Hispanic | 4,136 (20.7) | 4,104 (20.5) | |
American Indian/Alaska native | 228 (1.1) | 197 (1.0) | |
Asian or Pacific Islander | 3,604 (18.0) | 3,563 (17.8) | |
Black | 2,261 (11.3) | 2,326 (11.6) | |
White | 9,756 (48.8) | 9,796 (49.0) | |
Number of tumors | 1.06±0.27 | 1.06±0.28 | 0.86 |
Delayed treatment | 0.13 | ||
No | 3,462 (17.3) | 3,346 (16.7) | |
Yes | 16,523 (82.7) | 16,640 (83.3) | |
Type | 0.35 | ||
NOS | 19,747 (98.8) | 19,743 (98.8) | |
Clear cell type | 127 (0.6) | 144 (0.7) | |
Fibrolamellar | 65 (0.3) | 61 (0.3) | |
Pleomorphic type | 7 (0.0) | 4 (0.0) | |
Scirrhous | 22 (0.1) | 26 (0.1) | |
Spindle cell variant | 17 (0.1) | 8 (0.0) | |
T stage | 0.15 | ||
T1-2 | 14,768 (73.9) | 14,895 (74.5) | |
T3-4 | 5,217 (26.1) | 5,091 (25.5) | |
N stage | 0.22 | ||
N0 | 18,655 (93.3) | 18,718 (93.7) | |
N1 | 1,330 (6.7) | 1,268 (6.3) | |
M stage | 0.34 | ||
M0 | 17,682 (88.5) | 17,620 (88.2) | |
M1 | 2,303 (11.5) | 2,366 (11.8) | |
AJCC7 | 0.12 | ||
I_II | 13,216 (66.1) | 13,365 (66.9) | |
III_IV | 6,769 (33.9) | 6,621 (33.1) | |
Surgery | 0.37 | ||
None | 11,986 (60.0) | 12,132 (60.7) | |
Local tumor destruction | 3,540 (17.7) | 3,530 (17.7) | |
Hepatectomy | 2,953 (14.8) | 2,844 (14.2) | |
Liver transplant | 1,506 (7.5) | 1,480 (7.4) | |
Radiotherapy | 0.95 | ||
None | 19,493 (97.5) | 19,497 (97.6) | |
Yes | 492 (2.5) | 489 (2.4) | |
Chemotherapy | 0.07 | ||
No/unknown | 8,014 (40.1) | 7,836 (39.2) | |
Yes | 11,971 (59.9) | 12,150 (60.8) | |
Median household income | 0.37 | ||
Lower income | 373 (1.9) | 337 (1.7) | |
Median income | 8,703 (43.5) | 8,755 (43.8) | |
High income | 10,909 (54.6) | 10,894 (54.5) | |
Rural urban | 0.71 | ||
Counties | 12,444 (62.3) | 12,516 (62.6) | |
Nonmetropolitan | 1,775 (8.9) | 1,738 (8.7) | |
Metropolitan | 5,766 (28.9) | 5,732 (28.7) | |
Survival months | 33.82±39.13 | 34.07±39.34 | 0.53 |
Status | >0.99 | ||
Alive | 7,551 (37.8) | 7,552 (37.8) | |
Dead | 12,434 (62.2) | 12,434 (62.2) |
Data are expressed as mean ± SD or n (%). CSS, cancer-specific survival; NOS, not specified; AJCC, American Joint Committee on Cancer; SD, standard deviation.
Identification of predictive factors by univariate and multivariate analyses
The Cox proportional hazards regression model was employed to predict OS and CSS in the training cohort by analyzing each variable. Due to the collinearity between AJCC7 and T/N/M, AJCC7 was not included in the Cox regression model analysis. The results of the univariate Cox regression model are presented in Figure 2. Radiation therapy and delayed treatment were not associated with the prognosis of HCC. Age, sex, race, number of tumors, pathology type, T, N, M, surgery, chemotherapy, median household income, and rural-urban status were associated with both OS and CSS in HCC. Consequently, all these variables were included in the multivariate Cox regression analyses. However, based on the minimum AIC and the results of the multivariate Cox regression analyses, chemotherapy showed no significant association with OS and CSS (P=0.98 and P=0.19, respectively). Therefore, chemotherapy is not displayed in Figure 3, while the other variables are shown to be associated with OS and CSS in HCC. The survival analysis results for these 11 variables are presented in Figures S1-S4 (all P<0.001). The recent emphasis on understanding the influence of urban-rural geographical disparities and income on the prognosis of HCC has garnered considerable attention (16,17). These disparities may reflect variations in risk factors, health-related behaviors, and barriers to accessing medical services.
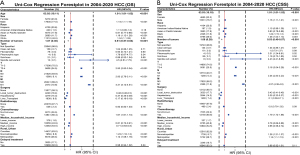
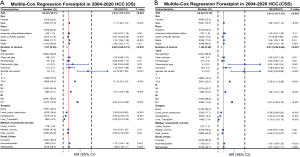
Predictive accuracy
The time-dependent ROC-AUCs were 0.742, 0.746, and 0.754 for the prediction of OS at 1, 2, and 3 years in the training cohort, respectively (Figure 4A). The calibration curve closely resembled the ideal line (Figure 4B-4D). Furthermore, as indicated by the time C-index in Figure 4E, the model consistently exhibited a superior C-index compared to AJCC 7th throughout the investigated period across all settings. The testing cohort also demonstrated consistency in the ROC-AUCs, calibration curve, and C-index (Figure 5).
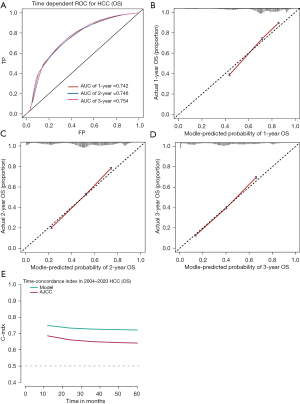
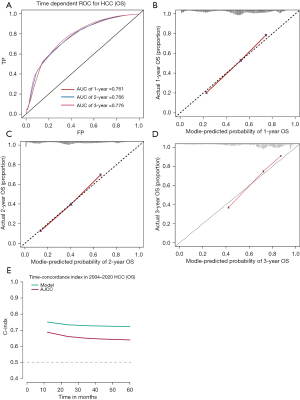
The time-dependent ROC-AUCs were 0.761, 0.763, and 0.770 for the prediction of CSS at 1, 2, and 3 years in the training cohort, respectively (Figure 6A). The calibration curve closely resembled the ideal line (Figure 6B-6D). Furthermore, as indicated by the time C-index in Figure 6E, the model consistently exhibited a superior C-index compared to AJCC 7th throughout the investigated period across all settings. The cohort also demonstrated consistency in ROC-AUCs, the calibration curve, and C-index (Figure 7).

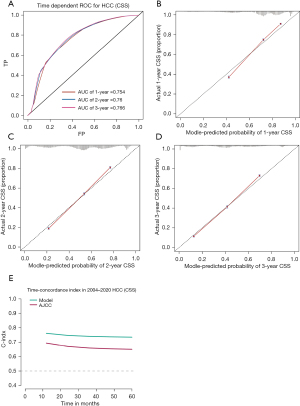
DCA was employed to evaluate the clinical effectiveness of the model, developed in the training cohort and extended to the validation cohort. Demonstrating excellent clinical applicability across a broad range of threshold probabilities, the model effectively predicts CSS and OS in HCC patients. Furthermore, as illustrated in Figure 8, the model consistently achieved greater clinical net benefit at 1, 2, and 3 years for both CSS and OS, surpassing the AJCC staging system.
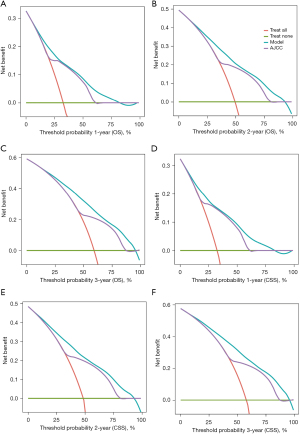
Construction of the clinic scoring system by ML
Data from 45,827 HCC patients were used to construct the OS scoring system. Subsequently, a 7:1:2 ratio was used to randomly divide patients into the training cohort, the validation cohort, and the test cohort respectively according to the AutoScore framework (10,11). According to the 10-fold cross-validation result, it can be seen that the top-11-variable-system (age, sex, race, number of tumors, pathology type, T, N, M, surgery, median household income, and rural-urban status) is a remarkable achievement by the measure of AUC, which achieved 0.688 (Figure 9A,9B). The clinical scoring system is presented in Table 3. A total of 39,971 cases of HCC patients were employed in constructing the CSS scoring system. Utilizing the same methodology for CSS analysis, an 11-feature scoring system exhibited an AUC as high as 0.715 (Figure 9C,9D). The detailed scoring is presented in Table 4.
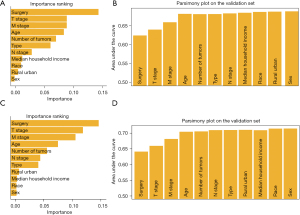
Table 3
Variable | Point |
---|---|
Age (years) | |
<48 | 0 |
48–69 | 2 |
70–79 | 6 |
≥80 | 8 |
Sex | |
Female | 0 |
Male | 2 |
Race | |
Asian or Pacific Islander | 0 |
American Indian/Alaska native | 3 |
Black | 4 |
Hispanic | 2 |
White | 3 |
Number of tumors | |
1 | 6 |
≥2 | 0 |
Type | |
Fibrolamellar | 0 |
NOS | 4 |
Clear cell type | 6 |
Pleomorphic type | 12 |
Scirrhous | 10 |
Spindle cell variant | 18 |
T stage | |
T1-2 | 0 |
T3-4 | 11 |
N stage | |
N0 | 0 |
N1 | 4 |
M stage | |
M0 | 0 |
M1 | 16 |
Surgery | |
None | 25 |
Local tumor destruction | 17 |
Hepatectomy | 11 |
Liver transplant | 0 |
Median household income | |
Lower income | 4 |
Median income | 1 |
High income | 0 |
Rural urban | |
Counties | 0 |
Nonmetropolitan | 1 |
Metropolitan | 1 |
NOS, not specified.
Table 4
Variable | Point |
---|---|
Age (years) | |
<48 | 0 |
48–69 | 1 |
70–79 | 4 |
≥80 | 6 |
Sex | |
Female | 0 |
Male | 1 |
Race | |
Asian or Pacific Islander | 0 |
American Indian/Alaska native | 3 |
Black | 3 |
Hispanic | 1 |
White | 3 |
Number of tumors | |
1 | 8 |
≥2 | 0 |
Type | |
Fibrolamellar | 0 |
NOS | 5 |
Clear cell type | 7 |
Pleomorphic type | 16 |
Scirrhous | 9 |
Spindle cell variant | 22 |
T stage | |
T1-2 | 0 |
T3-4 | 11 |
N stage | |
N0 | 0 |
N1 | 4 |
M stage | |
M0 | 0 |
M1 | 13 |
Surgery | |
None | 28 |
Local tumor destruction | 20 |
Hepatectomy | 15 |
Liver transplant | 0 |
Median household income | |
Lower income | 3 |
Median income | 1 |
High income | 0 |
Rural urban | |
Counties | 0 |
Nonmetropolitan | 1 |
Metropolitan | 1 |
NOS, not specified.
Survival analysis
In the OS dataset, stratification based on the median score (42 scores) yielded two groups. Survival analysis demonstrated a significant correlation between patients with scores above the median and a poorer prognosis in the training set, validation set, and the entire OS dataset, with statistical significance (Figure 10A-10C). Similar observations were noted in the CSS dataset [median score (46 scores)] (Figure 10D-10F).
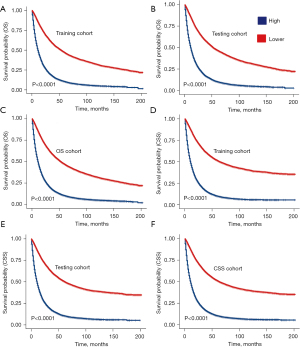
Discussion
In this population-based longitudinal study, we identified 11 (age, sex, race, number of tumors, pathology type, T, N, M, surgery, median household income, and rural-urban status) clinicopathological characteristics that can serve as reference points for predicting the prognosis of HCC. The discrimination and calibration of the 11 clinicopathological characteristics in both internal and external validation indicate that our predictive model demonstrates considerable performance. Furthermore, decision curves and model comparisons suggest its superiority over the AJCC staging system. Based on these findings, we developed a comprehensive scoring system to predict both the OS and CSS of patients with HCC. The novel scoring system holds significant clinical significance, offering a valuable predictive tool that can influence future treatment strategies and guide follow-up investigations for HCC.
Age emerged as an independent risk factor for HCC patients. Despite having liver functional reserves comparable to younger individuals, patients of advanced age (≥55 years) exhibit a poorer prognosis irrespective of the treatment received (18). In a multicenter study, early recurrence rates (≤2 years) after liver resection were found to be unrelated to gender. However, in the late stages, the recurrence rate in males was significantly higher than in females (19,20). In comparison to white individuals, black individuals have a poorer prognosis, while Hispanic and Asian populations exhibit better survival rates. This is associated with lower early diagnosis rates of HCC in black individuals (21). In multiple retrospective studies on liver transplantation, it was found that the number of tumors was not associated with the OS rate and recurrence rate after liver transplantation (22,23). This finding contradicts common knowledge. Interestingly, in our clinical scoring system, we also observed that patients with multiple tumors have higher scores compared to those with a single tumor. This constitutes an intriguing discovery. When the number of tumors was converted into a categorical variable, individuals with multiple tumors showed a significantly lower OS rate than those with a single tumor. However, there is no discernible difference in post-operative recurrence rates between the two groups (24). Surgery is one of the primary treatment modalities for HCC. A retrospective analysis, after clinical feature matching, revealed that patients who underwent surgery had a 55% lower mortality rate compared to those who received non-surgical treatments for HCC. Therefore, active promotion of surgical intervention for HCC is considered one of the most effective means to reduce mortality (25). A retrospective study observed that the incidence of HCC is higher among women from low-income rural households. Furthermore, a higher proportion of cases were diagnosed at advanced stages in this demographic, and these patients received less treatment. This was associated with lower education levels, limited access to medical resources in rural areas, and a higher prevalence of tumors. Consequently, this group of patients exhibited a significantly lower OS rate compared to individuals from higher-income and higher-education demographics (26).
Until now, there has been a lack of dedicated and widely accepted models for predicting the individual survival rate among HCC patients. Staging systems, such as the BCLC and AJCC staging systems, are currently widely utilized in clinical practice. However, these staging systems fail to provide accurate prognostic assessments for individuals (27). First, the significance derived from this study encompasses its potential to serve as a tool to assess individual patient OS and CSS. Secondly, it streamlines the process for clinical practitioners to swiftly identify high-risk patients, enabling timely monitoring. Most importantly, but not limited to, it offers direct guidance for the surgical approach and postoperative interventions for critical patients (median score).
Clinical scoring systems have broad applications in clinical practice. In addition to the previously mentioned scoring systems, the Apgar score (28), pain score (29), and the Glasgow Coma Scale have all played crucial roles as operational guidance tools in clinical settings (30). Given the evolving landscape of diseases and the continual advancement of clinical treatment methods, approaches for assessing the prognosis of HCC must also adapt to modern medical practices.
The primary strengths of the current study include, firstly, that our clinical scoring system is based on a large-scale population from the SEER database, providing rich and detailed data. Variables encompassed clinical characteristics and demographic information. The abundance of data ensured the accuracy of the clinical scoring system. Secondly, the main variables can be obtained before clinical treatment decisions, facilitating the process of making appropriate clinical treatment choices. Thirdly, post-operative management can be personalized, allowing for the timely identification of high-risk patients and optimizing the allocation of clinical resources. Last but not least, using C-index analysis, we found that the established clinical scoring system outperforms the AJCC staging system in assessing both OS and CSS.
Several limitations were encountered during this study. One limitation is that it was conducted through retrospective analysis. Therefore, the applicability of the scoring system has not been validated at other institutions. Furthermore, the critical inclusion and exclusion criteria may have overlooked valuable information, partly because the SEER dataset contains a considerable amount of missing data on several important clinical variables. This contributes to the absence of several crucial variables in the system, introducing considerable bias, as previous evidence suggested (31). For instance, tumor-related characteristics such as tumor size, tumor pathologic grade, as well as vascular invasion are all known risk factors for the poor prognosis of HCC (32-34). Meanwhile, the use of statin medication is also an important prognostic factor (35). Finally, multicenter prospective studies may confirm or improve the accuracy of our scoring system. Overall, our scoring system was designed to assist in the efficient and accurate management of HCC.
Conclusions
After conducting a large-scale retrospective analysis of HCC, we identified 11 clinical variables (age, sex, race, number of tumors, pathology type, T, N, M, surgery, median household income, and rural-urban status) with significant impacts on predicting the accuracy of OS and CSS in HCC. Our results suggest that a scoring system, trained using readily available clinical data, performs well in predicting prognosis. Future research should focus on validating this scoring system’s function in improving the management accuracy and efficiency for clinics, and better personalizing of treatments for HCC patients.
Acknowledgments
We deeply appreciate the statistical assistance (R language) from Dr. Jianming Zeng (biotrainee.com).
Funding: This research was funded by
Footnote
Reporting Checklist: The authors have completed the TRIPOD reporting checklist. Available at https://jgo.amegroups.com/article/view/10.21037/jgo-24-230/rc
Peer Review File: Available at https://jgo.amegroups.com/article/view/10.21037/jgo-24-230/prf
Conflicts of Interest: All authors have completed the ICMJE uniform disclosure form (available at https://jgo.amegroups.com/article/view/10.21037/jgo-24-230/coif). The authors have no conflicts of interest to declare.
Ethical Statement: The authors are accountable for all aspects of the work in ensuring that questions related to the accuracy or integrity of any part of the work are appropriately investigated and resolved. The study was conducted in accordance with the Declaration of Helsinki (as revised in 2013).
Open Access Statement: This is an Open Access article distributed in accordance with the Creative Commons Attribution-NonCommercial-NoDerivs 4.0 International License (CC BY-NC-ND 4.0), which permits the non-commercial replication and distribution of the article with the strict proviso that no changes or edits are made and the original work is properly cited (including links to both the formal publication through the relevant DOI and the license). See: https://creativecommons.org/licenses/by-nc-nd/4.0/.
References
- Sung H, Ferlay J, Siegel RL, et al. Global Cancer Statistics 2020: GLOBOCAN Estimates of Incidence and Mortality Worldwide for 36 Cancers in 185 Countries. CA Cancer J Clin 2021;71:209-49. [Crossref] [PubMed]
- Singal AG, Kanwal F, Llovet JM. Global trends in hepatocellular carcinoma epidemiology: implications for screening, prevention and therapy. Nat Rev Clin Oncol 2023;20:864-84. [Crossref] [PubMed]
- Villanueva A. Hepatocellular Carcinoma. N Engl J Med 2019;380:1450-62. [Crossref] [PubMed]
- Kudo M, Kawamura Y, Hasegawa K, et al. Management of Hepatocellular Carcinoma in Japan: JSH Consensus Statements and Recommendations 2021 Update. Liver Cancer 2021;10:181-223. [Crossref] [PubMed]
- Reig M, Forner A, Rimola J, et al. BCLC strategy for prognosis prediction and treatment recommendation: The 2022 update. J Hepatol 2022;76:681-93. [Crossref] [PubMed]
- Wang FH, Zhang XT, Li YF, et al. The Chinese Society of Clinical Oncology (CSCO): Clinical guidelines for the diagnosis and treatment of gastric cancer, 2021. Cancer Commun (Lond) 2021;41:747-95. [Crossref] [PubMed]
- Fan R, Papatheodoridis G, Sun J, et al. aMAP risk score predicts hepatocellular carcinoma development in patients with chronic hepatitis. J Hepatol 2020;73:1368-78. [Crossref] [PubMed]
- Child CG, Turcotte JG. Surgery and portal hypertension. Major Probl Clin Surg 1964;1:1-85. [PubMed]
- Anderson KM, Odell PM, Wilson PW, et al. Cardiovascular disease risk profiles. Am Heart J 1991;121:293-8. [Crossref] [PubMed]
- Xie F, Chakraborty B, Ong MEH, et al. AutoScore: A Machine Learning-Based Automatic Clinical Score Generator and Its Application to Mortality Prediction Using Electronic Health Records. JMIR Med Inform 2020;8:e21798. [Crossref] [PubMed]
- Xie F, Ning Y, Yuan H, et al. AutoScore-Survival: Developing interpretable machine learning-based time-to-event scores with right-censored survival data. J Biomed Inform 2022;125:103959. [Crossref] [PubMed]
- Kuhn M. Building Predictive Models in R Using the caret Package. Journal of Statistical Software 2008;28:1-26. [Crossref]
- Arnold TW. Uninformative Parameters and Model Selection Using Akaike's Information Criterion. The Journal of Wildlife Management 2010;74:1175-8.
- Blanche P, Dartigues JF, Jacqmin-Gadda H. Estimating and comparing time-dependent areas under receiver operating characteristic curves for censored event times with competing risks. Stat Med 2013;32:5381-97. [Crossref] [PubMed]
- Vickers AJ, Elkin EB. Decision curve analysis: a novel method for evaluating prediction models. Med Decis Making 2006;26:565-74. [Crossref] [PubMed]
- Opneja A, Cioffi G, Alahmadi A, et al. Adoption of single agent anticancer therapy for advanced hepatocellular carcinoma and impact of facility type, insurance status, and income on survival: Analysis of the national cancer database 2004-2014. Cancer Med 2021;10:4397-404. [Crossref] [PubMed]
- Wong RJ, Kim D, Ahmed A, et al. Patients with hepatocellular carcinoma from more rural and lower-income households have more advanced tumor stage at diagnosis and significantly higher mortality. Cancer 2021;127:45-55. [Crossref] [PubMed]
- Xu XS, Chen W, Miao RC, et al. Survival analysis of hepatocellular carcinoma: a comparison between young patients and aged patients. Chin Med J (Engl) 2015;128:1793-800. [Crossref] [PubMed]
- Zhang H, Han J, Xing H, et al. Sex difference in recurrence and survival after liver resection for hepatocellular carcinoma: A multicenter study. Surgery 2019;165:516-24. [Crossref] [PubMed]
- Nevola R, Ruocco R, Criscuolo L, et al. Predictors of early and late hepatocellular carcinoma recurrence. World J Gastroenterol 2023;29:1243-60. [Crossref] [PubMed]
- Rich NE, Carr C, Yopp AC, et al. Racial and Ethnic Disparities in Survival Among Patients With Hepatocellular Carcinoma in the United States: A Systematic Review and Meta-Analysis. Clin Gastroenterol Hepatol 2022;20:e267-88. [Crossref] [PubMed]
- Zavaglia C, De Carlis L, Alberti AB, et al. Predictors of long-term survival after liver transplantation for hepatocellular carcinoma. Am J Gastroenterol 2005;100:2708-16. [Crossref] [PubMed]
- Grasso A, Stigliano R, Morisco F, et al. Liver transplantation and recurrent hepatocellular carcinoma: predictive value of nodule size in a retrospective and explant study. Transplantation 2006;81:1532-41. [Crossref] [PubMed]
- Marelli L, Grasso A, Pleguezuelo M, et al. Tumour size and differentiation in predicting recurrence of hepatocellular carcinoma after liver transplantation: external validation of a new prognostic score. Ann Surg Oncol 2008;15:3503-11. [Crossref] [PubMed]
- Liu JH, Chen PW, Asch SM, et al. Surgery for hepatocellular carcinoma: does it improve survival? Ann Surg Oncol 2004;11:298-303. [Crossref] [PubMed]
- Shen Y, Guo H, Wu T, et al. Lower Education and Household Income Contribute to Advanced Disease, Less Treatment Received and Poorer Prognosis in Patients with Hepatocellular Carcinoma. J Cancer 2017;8:3070-7. [Crossref] [PubMed]
- Gospodarowicz MK, Miller D, Groome PA, et al. The process for continuous improvement of the TNM classification. Cancer 2004;100:1-5. [Crossref] [PubMed]
- Finster M, Wood M. The Apgar score has survived the test of time. Anesthesiology 2005;102:855-7. [Crossref] [PubMed]
- Huskisson EC. Measurement of pain. Lancet 1974;2:1127-31. [Crossref] [PubMed]
- Teasdale G, Maas A, Lecky F, et al. The Glasgow Coma Scale at 40 years: standing the test of time. Lancet Neurol 2014;13:844-54. [Crossref] [PubMed]
- Jeong CW, Washington SL 3rd, Herlemann A, et al. The New Surveillance, Epidemiology, and End Results Prostate with Watchful Waiting Database: Opportunities and Limitations. Eur Urol 2020;78:335-44. [Crossref] [PubMed]
- Sun B, Zhang S, Zhang D, et al. Vasculogenic mimicry is associated with high tumor grade, invasion and metastasis, and short survival in patients with hepatocellular carcinoma. Oncol Rep 2006;16:693-8. [Crossref] [PubMed]
- Torzilli G, Belghiti J, Kokudo N, et al. A snapshot of the effective indications and results of surgery for hepatocellular carcinoma in tertiary referral centers: is it adherent to the EASL/AASLD recommendations?: an observational study of the HCC East-West study group. Ann Surg 2013;257:929-37. [Crossref] [PubMed]
- Levi Sandri GB, Spoletini G, Vennarecci G, et al. Laparoscopic liver resection for large HCC: short- and long-term outcomes in relation to tumor size. Surg Endosc 2018;32:4772-9. [Crossref] [PubMed]
- Vell MS, Loomba R, Krishnan A, et al. Association of Statin Use With Risk of Liver Disease, Hepatocellular Carcinoma, and Liver-Related Mortality. JAMA Netw Open 2023;6:e2320222. [Crossref] [PubMed]