Multi-omics analysis of the prognostic and biological role of cuproptosis-related gene in gastric cancer
Highlight box
Key findings
• The interactive gene landscape, the prognostic value and biological functions of Cuproptosis-related genes (CRGs) in gastric cancer (GC) were comprehensively and systematically investigated.
What is known and what is new?
• Cuproptosis is the identified copper-dependent regulated cell death form relies on mitochondria respiration, which may affect cancer development and progression. However, the role of CRGs in tumorigenesis and tumor prognosis is still an under-explored topic.
• Our results showed that LIAS, GLS, and CDKN2A were selected as biomarkers in GC patients. Three methylation sites with a significant survival relationship were proposed. Besides, multi-factor regulatory networks were established on the basis of the co-expression network.
What is the implication, and what should change now?
• CRGs may play a key role in the tumor development and progression of GC, which may help guide precision medicine-based decision-making.
Introduction
Gastric cancer (GC) is the fifth most diagnosed malignancy worldwide and remains the third leading cause of cancer-related death due to its frequently advanced stage at diagnosis (1). Despite declining incidence rates in most countries, it can be expected to see more GC cases in the future due to ageing populations. The prognosis of GC patients is poor due to factors such as tumor recurrence, metastasis, tumor heterogeneity, and chemotherapy resistance (2). While the development of immune checkpoint inhibitors plus chemotherapy has significantly enhanced treatment for GC patients, a considerable number of them do not receive benefits from this regimen (3). Therefore, to develop reliable molecular biomarkers for GC diagnosis, prognosis and therapeutics is of vital importance.
Copper is an indispensable mineral nutrient involved in a wide range of physiological processes (4) and is a required cofactor for enzymes that mediate cellular functions (5). Numerous observations pointing out that copper accumulation may promote malignant transformation and serum or tissue levels of copper are elevated in various cancers, at the same time, dysregulation of copper homeostasis can induce oxidative stress and cytotoxicity (6). The underlying mechanisms by which excess copper induces cell death was proposed by Tsvetkov et al. (7). They demonstrated that this copper-dependent cell death way, termed as cuproptosis, was dependent on mitochondrial respiration. It occurred by direct binding copper to lipoylated components of the tricarboxylic acid cycle, resulting in the abnormal lipoylated protein aggregation and subsequent iron-sulfur cluster protein loss, which ultimately leaded to proteotoxic stress and cell death.
In recent years, researches have revealed the significant role of Copper in GC. Tang et al. conducted the first and comprehensive Cu-binding proteins (CBPs) analysis of GC patients and established a clinically feasible CBP signature for predicting survival and response to treatment (8). Feng et al. investigated a novel cuproptosis-related lncRNAs signature for its impacts on the prognosis and immunological features of GC (9). These studies of Copper involvement in GC provide valuable insights for the development of targeted therapeutic strategies. However, studies on cuproptosis-related genes (CRGs) signature in GC patients are limited and the role of CRGs in tumorigenesis and tumor prognosis is still an under-explored topic.
Therefore, we perform a comprehensive multi-omics study to investigate the prognostic value and biological functions of CRGs in GC. The study screens diagnostic, prognostic and therapeutic molecular biomarkers of GC based on CRGs, which may help guide precision medicine-based decision-making in GC patients. We present this article in accordance with the TRIPOD reporting checklist (available at https://jgo.amegroups.com/article/view/10.21037/jgo-23-946/rc).
Methods
Data collection
RNA sequencing (RNA-seq) data of 35 normal stomach and 415 GC samples, copy number variations (CNVs) data of 441 GC samples, and single nucleotide variant (SNV) data of 431 GC samples were obtained from The Cancer Genome Atlas (TCGA) database (http://portal.gdc.cancer.gov/). We removed the samples without survival information such as survival states and survival time, and there were 409 GC samples remained. Differential expression information of CRGs in 14 different cancers was obtained from the “Expression” module in Gene Set Cancer Analysis (GSCA) database (http://bioinfo.life.hust.edu.cn/GSCA/). The cancers included colon adenocarcinoma (COAD), esophageal carcinoma (ESCA), kidney renal clear cell carcinoma (KIRC), head and neck squamous cell carcinoma (HNSC), prostate adenocarcinoma (PRAD), breast invasive carcinoma (BRCA), bladder urothelial carcinoma (BLCA), thyroid carcinoma (THCA), GC, kidney renal papillary cell carcinoma (KIRP), lung adenocarcinoma (LUAD), lung squamous cell carcinoma (LUSC), liver hepatocellular carcinoma (LIHC), and kidney chromophobe (KICH). CRGs were acquired from reference (10). The study was conducted in accordance with the Declaration of Helsinki (as revised in 2013).
Gene expression profiling of CRGs
Expression level of CRGs between normal and tumor samples were acquired from the Pan-Cancer database in TCGA and were visualized as box plots by the R package “ggpubr” (version 0.4.0). The Chi-squared test was adopted to detect the significant differences of CRGs’ expression between carcinoma and adjacent tissue samples according to P<0.05. The expression level of CRGs between GC and normal stomach samples with pairing relationships was also visualized alone as box plots. Besides, the Spearman correlation analysis was used to detect correlation among CRGs via the R package “corrplot” (version 0.91).
Mutation and CNV of CRGs in GC samples
Gene mutation frequencies were obtained by using the R package “maftools” (version 2.6.05) on the basis of SNV data which were downloaded from TCGA (11). The Oncoplot function was adopted to generate a waterfall plot of Gene mutation frequencies. After, CNV data were downloaded from TCGA for further analysis according to the frequencies of chromosomal amplification, chromosomal deletion, and normal diploid of genes. The R package “ggplot2” (version 3.3.3) was used to generate bar diagrams (12).
Survival analyses of CRGs
The expression level of CRGs was merged with the total survival time and survival state of related GC patients. And the patients were divided into high- and low-expression groups according to an optimal threshold that was determined via the R package “survminer” (version 0.4.8). After, survival analyses of overall survival (OS), disease-special survival (DSS), disease-free survival (DFS), and progression-free survival (PFS) between both two groups were performed by using the R package “survminer”.
Clinical analysis of biomarkers
Receiver operating characteristic (ROC) curves of CRGs were generated by adopting the R package “pROC” (version 1.17.0.1) and the area under the curve (AUC) was calculated to assess the clinical value of CRGs (13). Then, differentially expressed CRGs (DE-CRGs) with survival correlation and clinical value were screened out as biomarkers of GC. The influences of clinical characteristics on the expression level of biomarkers were detected via the Chi-squared test according to P<0.05. Next, biomarkers were used to generate a nomogram by R package “Rms” (version 6.2-0) for the prediction of 1-, 3-, and 5-year survival probability. The calibration curves were produced at the same time for verifying the performance of the nomogram. The closer the slope was to 1, the more accurate the prediction was.
Methylation analysis of biomarkers
Methylation data were downloaded from the University of California, Santa Cruz (UCSC) Xena website (http://xena.ucsc.edu/) for Annotating the methylation site of biomarkers by adopting the R package “ChAMP” (version 2.20.1) (14). Then, the methylation sites were visualized via the R package “pheatmap” (version 1.0.12). After, Spearman correlation analysis among methylation sites of biomarkers were performed according to P<0.05 and |logFC| >0.1. Besides, the R package “survival” (version 3.2-3) was used to conduct Kaplan-Meier (K-M) survival analysis to detect the survival correlation of methylation sites and to generate K-M survival curves (15).
Enrichment analysis of biomarkers
Differentially expressed genes (DEGs) between high- and low-expressed groups were screened out by the R package “limma” (version 3.44.3) with P<0.05 and |logFC| >1 (16). Gene set enrichment analysis (GSEA) was performed by the R package “ClusturProfiler” (version 3.18.1) and R package “org.Hs.eg.db” (version 3.12.0) on the basis of Gene ontology (GO) and Kyoto Encyclopedia of Genes and Genomes (KEGG) (PMID: 34557778). GO included biological process (BP), molecular functions (MF), and cellular components (CC). The significance criteria were P<0.05 and count ≥1.
Immune correlation analysis of biomarkers
Immune cell percentages in 409 GC samples were calculated via Cell type Identification By Estimating Relative Subsets Of RNA Transcripts (CIBERSORT) algorithm (version 1.03) and LM22 gene set, and the correlation between biomarkers and immune cell percentage was detected by adopting Spearman correlation analysis. The rank sum test was used to compare the 22 immune cells between high- and low-expression groups. Related bubble charts, box plots, and lollipop diagrams were generated via the R package “ggplot2” and “ggpubr”.
Regulation factors were obtained via multi-factor regulatory networks
RNA-seq data of miRNA in normal and GC samples were obtained from the UCSC Xena website. Targeting correlation of miRNA-mRNA was identified using the miRWalk database (http://mirwalk.umm.uni-heidelberg.de/), and differentially expressed miRNAs (DE-miRNAs) (P<0.05 and |logFC| >0) between normal (n=41) and GC samples (n=387) were screened out via the R package “limma”. Then, miRNAs obtained previously were intersected with the DE-miRNAs, and a miRNA-mRNA network was generated by R package “Cytoscape” (version 3.8.2) (17).
Targeting correlation of TFs-mRNA was identified adopting the NetworkAnalyst database (https://www.networkanalyst.ca/), and DEGs (P<0.05 and |logFC| >1) between normal (n=35) and GC samples (n=415) were sifted out (18). Then, an intersection between DEGs and TFs was produced and it was used to construct a TFs-mRNA network of biomarkers via the R package “Cytoscape”. The interaction among factors in the networks was identified by Spearman correlation analysis and visualized as a heatmap through R package “ggplot2”. Survival analyses of miRNAs in the network between high- and low-expressed groups were performed by the R package “survival”.
Correlation analysis between DEGs and biomarkers was performed to conduct further differential analysis according to P<0.05 and |logFC| >0.3. The R package “Cytoscape” was used to construct a co-expression network of biomarkers, and the enrichment analysis of co-expression genes and biomarkers in the network was implemented by the Metascape tool (https://metascape.org/gp/index.html#/main/step1) (19).
Statistical analysis
Categorical variables were presented as numbers and percentages and analyzed using the Chi-squared test. Continuous variables were expressed as mean ± standard deviation (SD) and assessed using the Wilcoxon rank sum test. The Spearman correlation analyses were used to detect correlation among factors. Survival analyses were performed using the K-M method, and differences in survival times were evaluated using the log-rank test. All statistical analyses were performed using R version 4.3.1, with P values <0.05 indicating a significant difference.
Results
Four differential expressed CRGs between GC and adjacent tissue samples were identified
In order to identify whether cuproptosis has a high correlation with cancers progression, we obtained differential expression information of 10 CRGs including CDKN2A, DLAT, DLD, FDX1, GLS, LIAS, LIPT1, MTF1, PDHA1, and PDHB from GSCA database for differential expression analyses. The result showed that CDKN2A and LIAS had a significant difference in at least seven cancers (Figure 1A). After, the expression level of 10 CRGs between the normal and tumor samples was acquired from the TCGA database. The box plots demonstrated that the expression level of CDKN2A and GLS were significantly up-regulated and LIAS and PDHB were significantly down-regulated in GC samples (Figure 1B). Of note, expression level of DLD significantly changed in BLCA, BRCA, and THCA (Figure 1C-1E). Besides, the box plots visually revealed the normalized expression level of each CRG between GC samples and the paired normal samples (Figure 1F). The heatmap showed that there was no significant correlation among CRGs (Figure 1G). Moreover, tumor-specific CNVs and SNVs were helpful for exploring the molecular mechanisms of GC progression. We analyzed the mutation frequencies of CRGs of GC samples, and the result showed that the LIPT1 and CDKN2A had the highest mutation frequency of 6% and 4% respectively (Figure 1H). After analysis of CNV was performed and we found that the CDKN2A, LIAS, and PHDB frequently experienced the copy number deletion (DEL), and DLD frequently experienced the cope number amplification (AMP) (Figure 1I).
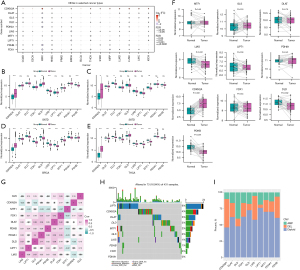
Survival analyses between high- and low-expression groups
A series of survival analyses between high- and low-expression groups were performed to identify the correlation between the expression level of CRGs and GC prognosis. The OS curves showed that the expression level of MTF1, DLAT, and LIAS were positively correlated, while the expression level of GLS was negatively correlated with the prognosis of GC (Figure S1). After, the DSS analysis results demonstrated that the expression level of MTF1, DLAT, PDHA1, DLD, and LIAS were positively correlated, while the expression level of CDKN2A was negatively correlated with the prognosis of GC (Figure S2). Moreover, DFS analysis results illustrated that the expression level of three CRGs including MTF1, PDHA1, and DLD was positively correlated with the prognosis of GC (Figure S3). Finally, progressive-free survival curves showed that the expression level of five CRGs including MTF1, PDHA1, DLD, LIAS, and DLAT was positively correlated with the prognosis of GC (Figure S4). As shown in Table 1, there were four differentials expressed CRGs had a significant survival relationship.
Table 1
Gene | OS | DSS | DFS | PFS |
---|---|---|---|---|
GLS* | True | False | False | False |
LIAS* | True | True | False | True |
CDKN2A* | False | True | False | False |
DLD* | False | True | True | True |
PDHB* | False | False | False | False |
DLAT | True | True | False | True |
MTF1 | True | True | True | True |
FDX1 | False | False | False | False |
LIPT1 | False | False | False | False |
PDHA1 | False | True | True | True |
*, represents differentially expressed genes in GC. True represents survival significance. CRGs, cuproptosis-related genes; GC, gastric cancer; OS, overall survival; DSS, disease-special survival; DFS, disease-free survival; PFS, progression-free survival.
Three biomarkers of clinical prognosis prediction were obtained
We performed a single-gene ROC analysis to evaluate the value of CRGs for GC prognosis, and the ROC curves demonstrated that PDHB, CDKN2A, LIAS, and GLS have great prognosis prediction abilities (Figure 2A-2D). Then, considering the differential expression between normal and GC samples, and the survival correlation with GC, three CRGs including CDKN2A, LIAS, and GLS were chosen as biomarkers of GC prognosis. After, the expression level of biomarkers between high- and low-risk groups in different clinical subgroups including age, gender, vital, state, grade, T stage, N stage, and M stage were compared. We found that the expression level of CDKN2A between G2 and G3 were significantly different. The expression level of GLS in T3 and T4 were significantly higher than in T1, while the expression level of LIAS in T2 and T3 are significantly lower than in T1 (Figure 2E-2G). The total information is provided in Tables S1-S3. Finally, the expression of biomarkers were used to generate a nomogram of GC prognosis prediction (Figure 2H). The efficacy of CDKN2A was relatively low since there was no significant correlation between CDKN2A and OS. The calibration curve demonstrates that the nomogram has a great prediction accuracy of 1-year survival (Figure 2I), but slightly less effective in predicting long-term survival.
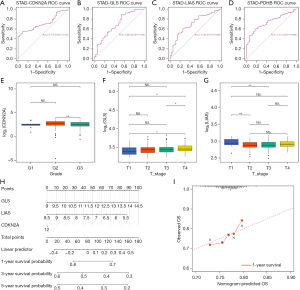
Three methylation sites of CRGs significantly were significantly related to survival
Gene methylation significantly affects gene function. In this study, the methylation site of biomarkers was detected through the UCSC Xena website, and visualized in a heatmap (Figure 3A). The scatter plots show that cg07562918 and cg13601799 were significantly related with CDKN2A, cg07253264 and cg09390371 were significantly associated with LIAS, and cg03962451 and cg19300307 were significantly correlated with GLS (Figure 3B-3G). Total information of the correlation of methylation sites is provided in Table S4. Finally, K-M survival analysis between high- and low-expressed groups was utilized, and we found that cg13601799 and cg07562918 of CDKN2A, and cg07253264 of LIAS had a strongly significant correlation with GC survival (Figure 3H-3J).
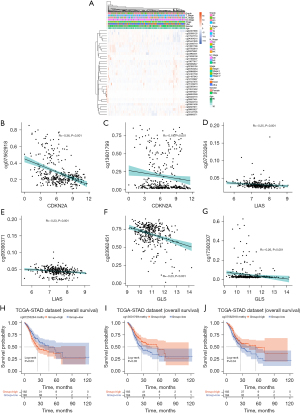
Functional enrichment of CDKN2A, GLS, and LIAS
In order to identify the biological functions of biomarkers, differential expression analysis was implemented to screen DEGs between high- and low-expressed groups based on the expression level of biomarkers, and then DEGs were used for enrichment analysis. Enrichment results of biomarker CDKN2A show that there were 82 functions (such as digestion, antimicrobial humoral response, and thyroid hormone generation) in GO-BP, 35 functions (such as apical plasma membrane, apical part of cell, and chylomicron) in GO-CC, 33 functions (such as endopeptidase activity, cholesterol transfer activity, and sterol transfer activity) in GO-MF (Figure 4A), and 9 KEGG pathways such as Fat digestion and absorption, Cholesterol metabolism, and Proximal tubule bicarbonate reclamation (Figure 4B). Enrichment results of biomarker GLS demonstrated that there were 90 functions (such as digestion, digestive system process, and maintenance of gastrointestinal epithelium) in GO-BP, 12 functions (such as digestion, digestive system process, and maintenance of gastrointestinal epithelium) in GO-CC, 42 functions (such as aspartic-type endopeptidase activity, aspartic-type peptidase activity, and carboxylic acid transmembrane transporter activity) in GO-MF (Figure 4C), and four KEGG pathways included fat digestion and absorption and protein digestion, absorption, pancreatic secretion, and mineral absorption (Figure 4D). Enrichment results of biomarker LIAS illustrated that there were 171 functions (such as uterus development, skin development, and negative regulation of cardiac muscle tissue development) in GO-BP, 51 functions (such as serine-type endopeptidase inhibitor activity, co-receptor binding, and endopeptidase inhibitor activity) in GO-MF (Figure 4E), and three KEGG pathways included cell adhesion molecules, Wnt signaling pathway, and fat digestion and absorption (Figure 4F).
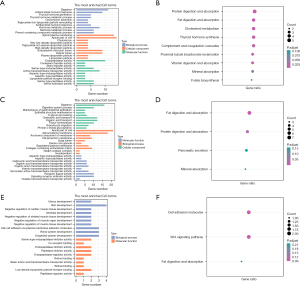
Expression of biomarkers was correlated with immune cells
Immune cells are an important part of the tumor microenvironment (TME) and profoundly influence the progression of GC. We calculated the percentage of the immune cells in GC samples, and the results were visualized as a stacked bar chart (Figure 5A). The bubble charts demonstrated that biomarker GLS had a negative correlation with T cells regulatory (Tregs), NK cells resting, and neutrophils. The biomarker LIAS had a positive correlation with T cells CD4 memory activated, and had a negative correlation with macrophages M0 and macrophages M1. The biomarker CDKN2A had a negative correlation with B cells naive and plasma cells (Figure 5B). The results of differential analyses demonstrated that the percentage of B cells native in high-expressed group of CDKN2A was significant different with low-expression group (Figure 5C). The proportion of B cells naive, T cells regulatory (Tregs), mast cells resting, mast cells activated, and dendritic cells resting in high-expressed group of GLS were significant different with the proportion in low-expressed group (Figure 5D). The percentage of B cells naive, Tregs, mast cells resting, mast cells activated, and dendritic cells resting in high-expressed group of LIAS were significant different with low-expressed group (Figure 5E). The correlations between expression level of biomarkers and immune cells were visualized in lollipop charts (Figure 5F-5H). Besides, we detected the correlation between biomarkers and immune checkpoints, and the result showed that CDKN2A had a negative correlation with IDO1, TIGIT, CD274, PDCD1LG2, ICOS, and HAVCR2, LIAS was negatively related with CD27, and GLS was positively related with ICOS (Figure 5I).
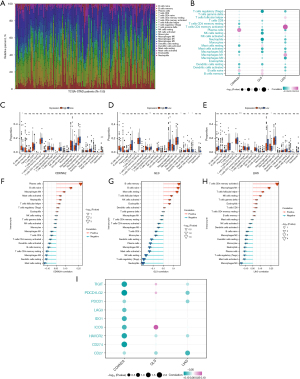
MiRNA-mRNA network of biomarkers
Mi-RNAs can affect the function of biomarkers by degrading mRNA. The volcano plot indicated that there were 724 DE-mRNAs between normal and GC samples (Figure 6A). Then, a miRNA-mRNA network of biomarkers including 22 miRNAs was generated on the basis of correlation of miRNA-mRNA which identified via miRWalk database (Figure 6B). The heatmap of correlation biomarkers and miRNAs indicated that CDKN2A significantly correlate with hsa-miR-330-5P, hsa-miR-181d-5P, hsa-miR-181b-5P, and hsa-miR-1301-3P, and GLS significantly correlates with hsa-miR-34a-5P, hsa-miR-181d-5P, and hsa-miR-1301-3P, and LIAS significantly correlates with 6 miRNAs including hsa-miR-942-5P, hsa-miR-432-5P, hsa-miR-181d-5P, hsa-miR-181b-5P, hsa-miR-125b-5P, and hsa-miR-125a-5P (Figure 6C). Besides, there were eight miRNA significantly correlated with OS of patients, which included hsa-miR-185-5P, hsa-miR-320b, hsa-miR-181b-5P, hsa-miR-34b-5P, hsa-miR-370-3P, hsa-miR-490-3P, hsa-miR-942-5P, and hsa-miR-432-5P (Figure 6D-6K).
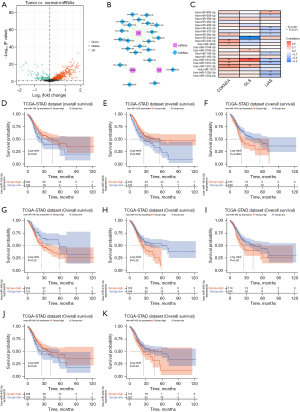
TFs-mRNA network of biomarkers
TFs can affect the function of biomarkers by affecting the transcription process of mRNA. DEG analysis was implemented to obtain 3,489 DEGs between normal and GC samples, and then those DEGs were intersected with TFs identified through the NetworkAnalyst database (Figure 7A,7B). There were five differentially expressed TFs in the intersection and they were used for constructing a TFs-mRNA network (Figure 7C). The heatmap demonstrated that CDKN2A significantly correlated with RCOR2, GLS significantly correlated with SOX5, RCOR2, EZH2, and ELF3, and LIAS was significantly correlated with SOX5, KLF9, EZH2, and ELF3 (Figure 7D). The survival analysis of 5 TFs indicated that 3 TFs including EZH2, SOX5, and KLF9 had a significant correlation with OS of GC patients (Figure 7E-7G).
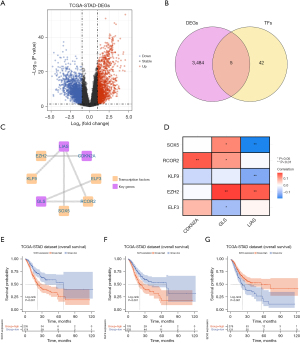
Co-expression network of biomarkers
We constructed a co-expression network to indicate reciprocity between DEGs and biomarkers (Figure 8A), and there were 82 genes co-express with GLS, 21 genes co-express with LIAS, and 19 genes co-express with CDKN2A in the network. Then, we performed enrichment analysis on the basis of the network, and we found that there are 20 functions of biomarkers and co-expressed genes, such as cardiac septum development, collagen fibril organization, and sensory organ morphogenesis (Figure 8B).
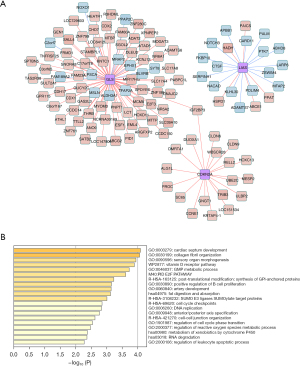
Discussion
GC remains to be one of the most common epithelial cancers while most patients are diagnosed at an advanced stage and still cannot benefit from the developing comprehensive therapeutic strategies. Therefore, developing new potential therapeutic targets is urgently needed and may contribute to guide personalized medicine for GC patients. Cuproptosis is the recently identified pathway which may affect tumor development and progression. Despite increasing studies are emerging to explore the associations between CRGs and typical tumors (20,21). Due to heterogeneity of tumors and corresponding interactions, CRGs signature varied in different cancers. Specifically, there is uncertainty regarding the prognostic accuracy of CRGs and their biological functions in GC. Therefore, it is necessary to perform a full-scale investigation of CRGs in GC patients.
In this study, we used the large-scale public database of GC transcriptome data and clinical data to screen key CRGs that may have potential prognostic, diagnostic and other guidance implications. Moreover, the underlying mechanisms of molecular alterations and clinical relevance of CRGs in GC were further explored and elucidated. In previous studies, 10 genes related to copper-induced cell death pathways were identified (7). Building on this result, the present study aims to further identify copper death genes that play a key role in GC. A relatively low mutation frequency but high frequency of copy number alterations was found in CRGs, consistent with the previous study (22), which implicated that CRGs might be a potential treatment targets and can serve as a prognosis factor in GC patients.
We ultimately identified three molecular biomarkers, GLS, LIAS and CDKN2A, which play a critical role in the copper death pathways of GC patients. Further analysis was conducted on these markers using methods such as methylation analysis, enrichment analysis, immune infiltration analysis, and construction of multi-factor regulatory networks to explore their significance in guiding prognosis and diagnosis of GC patients. GLS is an essential substance for cellular energy metabolism, which is responsible for the conversion of glutamine to glutamate. The high GLS expression is related with poor prognosis in GC patients. LIAS is located in the mitochondrion and encodes the protein of the biotin and lipoic acid synthetases family (23). Decreased LIAS expression is associated with diminished hepatic alpha-lipoic acid and tissue oxidative stress. A high LIAS expression is related to the good prognosis in patients with various cancers (24), which is consistent with the result of our study. CDKN2A is a tumor suppressor gene that encodes two distinct proteins to inhibit the cell cycle and promote apoptosis. The main regulatory pathway for CDKN2A is via the p53 signaling pathway, in cancer, mutations or deletions of CDKN2A are common, leading to loss of its tumor suppressive functions and contributing to tumor growth and progression (25). Our study showed that higher expression of CDKN2A was associated with lower DSS in GC patients, but there was no significant difference in OS or DFS. As shown in the Results section, we analyzed hundreds of enriched pathways for the three biomarkers mentioned above. Moreover, a nomogram of GC prognosis prediction was constructed using clinical features and the expression level of biomarkers, which showed a great prediction accuracy of 1-year survival.
In addition to the above-mentioned biomarkers, we also analyzed other molecules related to prognosis. MTF1 is an essential metal-binding transcription factor (TF) that binds to conserved DNA sequence motifs in the heavy metal response, resulting in the loss of heavy metal response gene transcription and cellular protection (26). Our study firstly revealed that the high expression of MTF1 resulted in better OS in GC patients, which was consistent with the previously reported role for MTF1 and Cu in cell differentiation and gene expression (27).
Immune infiltration analysis and the epigenetic regulation of immune response have been widely applied in clinical research on GC and provide useful guidance for patient treatment selection and prognostic evaluation (28). Considering the important position of immunotherapy in GC, immune infiltration analysis studies were also used to assess the distinct roles of the subclusters and to investigate immune cell dysregulation in GC. GLS has a negative correlation with Tregs, NK cells resting, and neutrophils, LIAS has a positive correlation with T cells CD4 memory activated, and has a negative correlation with macrophages M0 and macrophages M1. CDKN2A has a negative correlation with B cells naive and plasma cells. The results of immune infiltration are largely consistent with some other studies. For example, recent research indicated that GLS was involved in immune-related signaling pathways, such as T-cell receptor signaling pathway, chemokine signaling pathway and hypoxia-related pathways (29). However, further research is needed to determine the optimal immune infiltration analysis method and ways to apply it to personalized treatment decision-making.
Besides, a multifactorial regulatory network was constructed for key genes, and prognostic analysis of miRNAs and TFs was performed. In our study, multiple survival-related miRNAs and TFs were screened out. Eight miRNAs were significantly correlated with OS of patients. And the survival analysis of five TFs indicated that three TFs including EZH2, SOX5, and KLF9 had a significant correlation with OS of GC patients. Accumulating evidence indicates that copper are involved in the regulation of miRNAs and TFs in GC, thereby promoting the proliferation, invasion, and metastasis of cancer cells (30). They contribute to GC as oncogenes or tumour suppressors by inhibiting either directly or indirectly the expression of target genes. In addition to miRNAs, copper ions can also affect the expression and function of some TFs, thereby affecting the development of various cancers (31), while the role remains unclear in GC. Our research provided important reference information for the future development of targeted therapeutic strategies for GC patients.
Our study has some limitations. First, the database of GC samples needs to be expanded for more comprehensive investigation. Second, our study is based on bioinformatics analysis, further in vitro and in vivo research is still warranted to explore the specific mechanism of CRGs affecting tumor development. Even so, our results provide new insights into the diagnosis, prognosis, and treatment of GC patients.
Conclusions
Our study identified possible biomarkers through bioinformatics analysis and systematically investigated the interactive gene landscape, prognosis role and molecular changes of CRGs in GC patients. Multi-factor regulatory networks were constructed to screen out factors with regulating influences of biomarkers. The results indicated that these CRGs may play a key role in the tumor development and progression of GC and highlighted its potential for clinical applications to guide clinical care and improve treatment selection in patients.
Acknowledgments
Funding: This work was supported by
Footnote
Reporting Checklist: The authors have completed the TRIPOD reporting checklist. Available at https://jgo.amegroups.com/article/view/10.21037/jgo-23-946/rc
Peer Review File: Available at https://jgo.amegroups.com/article/view/10.21037/jgo-23-946/prf
Conflicts of Interest: All authors have completed the ICMJE uniform disclosure form (available at https://jgo.amegroups.com/article/view/10.21037/jgo-23-946/coif). R.Z. reports that this work was supported by Guangzhou Basic and Applied Research Project (SL2024A04J01162). W.C. reports that this work was supported by Guangdong Basic and Applied Basic Research Foundation (2023A1515110913). The other authors have no conflicts of interest to declare.
Ethical Statement: The authors are accountable for all aspects of the work in ensuring that questions related to the accuracy or integrity of any part of the work are appropriately investigated and resolved. The study was conducted in accordance with the Declaration of Helsinki (as revised in 2013).
Open Access Statement: This is an Open Access article distributed in accordance with the Creative Commons Attribution-NonCommercial-NoDerivs 4.0 International License (CC BY-NC-ND 4.0), which permits the non-commercial replication and distribution of the article with the strict proviso that no changes or edits are made and the original work is properly cited (including links to both the formal publication through the relevant DOI and the license). See: https://creativecommons.org/licenses/by-nc-nd/4.0/.
References
- Smyth EC, Nilsson M, Grabsch HI, et al. Gastric cancer. Lancet 2020;396:635-48. [Crossref] [PubMed]
- Yang L, Ying X, Liu S, et al. Gastric cancer: Epidemiology, risk factors and prevention strategies. Chin J Cancer Res 2020;32:695-704. [Crossref] [PubMed]
- Janjigian YY, Shitara K, Moehler M, et al. First-line nivolumab plus chemotherapy versus chemotherapy alone for advanced gastric, gastro-oesophageal junction, and oesophageal adenocarcinoma (CheckMate 649): a randomised, open-label, phase 3 trial. Lancet 2021;398:27-40. [Crossref] [PubMed]
- Chen L, Min J, Wang F. Copper homeostasis and cuproptosis in health and disease. Signal Transduct Target Ther 2022;7:378. [Crossref] [PubMed]
- Zhang Z, Zeng X, Wu Y, et al. Cuproptosis-related risk score predicts prognosis and characterizes the tumor microenvironment in hepatocellular carcinoma. Front Immunol 2022;13:925618. [Crossref] [PubMed]
- Ge EJ, Bush AI, Casini A, et al. Connecting copper and cancer: from transition metal signalling to metalloplasia. Nat Rev Cancer 2022;22:102-13. [Crossref] [PubMed]
- Tsvetkov P, Coy S, Petrova B, et al. Copper induces cell death by targeting lipoylated TCA cycle proteins. Science 2022;375:1254-61. [Crossref] [PubMed]
- Tang X, Guo T, Wu X, et al. Clinical Significance and Immune Infiltration Analyses of the Cuproptosis-Related Human Copper Proteome in Gastric Cancer. Biomolecules 2022;12:1459. [Crossref] [PubMed]
- Feng A, He L, Chen T, et al. A novel cuproptosis-related lncRNA nomogram to improve the prognosis prediction of gastric cancer. Front Oncol 2022;12:957966. [Crossref] [PubMed]
- Kahlson MA, Dixon SJ. Copper-induced cell death. Science 2022;375:1231-2. [Crossref] [PubMed]
- Mayakonda A, Lin DC, Assenov Y, et al. Maftools: efficient and comprehensive analysis of somatic variants in cancer. Genome Res 2018;28:1747-56. [Crossref] [PubMed]
- Ito K, Murphy D. Application of ggplot2 to Pharmacometric Graphics. CPT Pharmacometrics Syst Pharmacol 2013;2:e79. [Crossref] [PubMed]
- Robin X, Turck N, Hainard A, et al. pROC: an open-source package for R and S+ to analyze and compare ROC curves. BMC Bioinformatics 2011;12:77. [Crossref] [PubMed]
- Tian Y, Morris TJ, Webster AP, et al. ChAMP: updated methylation analysis pipeline for Illumina BeadChips. Bioinformatics 2017;33:3982-4. [Crossref] [PubMed]
- Mosmann T. Rapid colorimetric assay for cellular growth and survival: application to proliferation and cytotoxicity assays. J Immunol Methods 1983;65:55-63. [Crossref] [PubMed]
- Ritchie ME, Phipson B, Wu D, et al. limma powers differential expression analyses for RNA-sequencing and microarray studies. Nucleic Acids Res 2015;43:e47. [Crossref] [PubMed]
- Shannon P, Markiel A, Ozier O, et al. Cytoscape: a software environment for integrated models of biomolecular interaction networks. Genome Res 2003;13:2498-504. [Crossref] [PubMed]
- Zhou G, Soufan O, Ewald J, et al. NetworkAnalyst 3.0: a visual analytics platform for comprehensive gene expression profiling and meta-analysis. Nucleic Acids Res 2019;47:W234-41. [Crossref] [PubMed]
- Zhou Y, Zhou B, Pache L, et al. Metascape provides a biologist-oriented resource for the analysis of systems-level datasets. Nat Commun 2019;10:1523. [Crossref] [PubMed]
- Bian Z, Fan R, Xie L. A Novel Cuproptosis-Related Prognostic Gene Signature and Validation of Differential Expression in Clear Cell Renal Cell Carcinoma. Genes (Basel) 2022;13:851. [Crossref] [PubMed]
- Chen Y. Identification and Validation of Cuproptosis-Related Prognostic Signature and Associated Regulatory Axis in Uterine Corpus Endometrial Carcinoma. Front Genet 2022;13:912037. [Crossref] [PubMed]
- Han H, Nakaoka HJ, Hofmann L, et al. The Hippo pathway kinases LATS1 and LATS2 attenuate cellular responses to heavy metals through phosphorylating MTF1. Nat Cell Biol 2022;24:74-87. [Crossref] [PubMed]
- Hendricks AL, Wachnowsky C, Fries B, et al. Characterization and Reconstitution of Human Lipoyl Synthase (LIAS) Supports ISCA2 and ISCU as Primary Cluster Donors and an Ordered Mechanism of Cluster Assembly. Int J Mol Sci 2021;22:1598. [Crossref] [PubMed]
- Cai Y, He Q, Liu W, et al. Comprehensive analysis of the potential cuproptosis-related biomarker LIAS that regulates prognosis and immunotherapy of pan-cancers. Front Oncol 2022;12:952129. [Crossref] [PubMed]
- Xu J, Li N, Deng W, et al. Discovering the mechanism and involvement of the methylation of cyclin-dependent kinase inhibitor 2A (CDKN2A) gene and its special locus region in gastric cancer. Bioengineered 2021;12:1286-98. [Crossref] [PubMed]
- Han H, Nakaoka HJ, Hofmann L, et al. The Hippo pathway kinases LATS1 and LATS2 attenuate cellular responses to heavy metals through phosphorylating MTF1. Nat Cell Biol 2022;24:74-87. [Crossref] [PubMed]
- Tavera-Montañez C, Hainer SJ, Cangussu D, et al. The classic metal-sensing transcription factor MTF1 promotes myogenesis in response to copper. FASEB J 2019;33:14556-74. [Crossref] [PubMed]
- Kim W, Chu TH, Nienhüser H, et al. PD-1 Signaling Promotes Tumor-Infiltrating Myeloid-Derived Suppressor Cells and Gastric Tumorigenesis in Mice. Gastroenterology 2021;160:781-96. [Crossref] [PubMed]
- Liu Z, Wang L, Xing Q, et al. Identification of GLS as a cuproptosis-related diagnosis gene in acute myocardial infarction. Front Cardiovasc Med 2022;9:1016081. [Crossref] [PubMed]
- Lee Y, Ahn C, Han J, et al. The nuclear RNase III Drosha initiates microRNA processing. Nature 2003;425:415-9. [Crossref] [PubMed]
- Ramchandani D, Berisa M, Tavarez DA, et al. Copper depletion modulates mitochondrial oxidative phosphorylation to impair triple negative breast cancer metastasis. Nat Commun 2021;12:7311. [Crossref] [PubMed]