Role of TAP1 in the identification of immune-hot tumor microenvironment and its prognostic significance for immunotherapeutic efficacy in gastric carcinoma
Introduction
Gastric cancer (GC) represents a highly diverse disease and is the fourth leading cause of mortality related to cancer globally (1,2). Despite the significant progress in clinical diagnosis as well as therapeutic approaches for GC, challenges stemming from low early diagnosis rates and limitations in treating advanced GC have led to a meager 5-year survival rate of <10% (3). Furthermore, due to intratumoral and intertumoral heterogeneity, patients with GC exhibit distinct differences in treatment response (1). Hence, there is an urgent need to explore new and useful biomarkers for drug targeting or predicting therapeutic responses in GC.
With the recent in-depth exploration of immune-related biomarkers and the implementation of immune checkpoint inhibitors (ICIs), such as programmed cell death protein 1 (PD-1) and programmed death-ligand 1 (PD-L1), immunotherapy has rapidly developed, bringing about a revolution in the management of various solid tumors (4,5). Considerable clinical trials of ICIs have demonstrated manageable toxicity and anti-tumor activity in patients with advanced GC (6-10). Moreover, accumulating evidence suggests that the immune system is critical in influencing the response to standard therapy as well as the long-term survival of GC patients (11). For instance, a clinical trial on nivolumab (a PD-1 inhibitor) for advanced GC revealed a longer overall survival (OS) in patients administered nivolumab in contrast with those administered a placebo (5.26 vs. 4.14 months) (8). Although the implementation of immunotherapy utilizing PD-1/PD-L1 blockade has shown promising results, the highly variable objective immunotherapeutic response rates among clinical trials (7-10,12) as well as the innate/acquired resistance to immunotherapy agents in substantial numbers of patients cannot be overlooked (13).
Mounting evidence suggests that the composition of the tumor microenvironment (TME) can impact the therapeutic response, thereby categorizing tumors as either cold or hot. Cold tumors typically demonstrate an immunosuppressive TME, rendering them resistant to chemotherapy or immunotherapy. Conversely, hot tumors exhibit heightened sensitivity to treatments, often associated with T cell (TC) inflammation (14,15). Notably, hot tumors show a favorable reaction to immunotherapy, including anti-PD-1/PD-L1 therapy (16), whereas cold tumors tend not to respond to immunotherapy. Therefore, the differentiation of hot and cold tumors represents a good method for characterizing the responsiveness to immunotherapy.
Extensive studies have highlighted the involvement of transporter associated with antigen processing 1 (TAP1), a member of the ATP-binding cassette (ABC) family, in the transportation of antigens from the cytoplasm to the endoplasmic reticulum for their association with major histocompatibility complex (MHC) class I molecules (17). Acting as the peptide binding molecular scaffold for MHC class I folding, TAP1 also provides endogenous protein peptides essential for the activation of CD8+ cytotoxic TCs (18), indicating its crucial role in maintaining the biological functions of the immune system. Although increased TAP1 has been associated with improved targeting of tumor cells, conflicting findings suggest that tumor cells can potentially evade recognition by cytotoxic immune cells through suppressing peptide delivery via the regulation of TAP1 expression (19-21). Additionally, genetic variants such as an increasing copy number of TAP1 are tightly linked to histological changes among tumor cells (22). The epigenetic silencing of TAP1 among tumor cells also results in enhancing tumor survival and promoting immune evasion (21). Notably, TAP1 is crucial in anti-PD-1 antibody immunotherapy mediated by interleukin 27 (IL-27) (23). The loss of TAP1 expression could remodel the immune status of the TME and participate in reversing resistance to anti-PD-1 therapy. These findings collectively suggest the pivotal role of TAP1 within tumor progression and immune response regulation, implying that TAP1 could serve as a biomarker influencing tumor development as well as the immune status of TME.
Hence, the present study explored the association of TAP1 expression with immunological characteristics, seeking to exploit the value of TAP1 as a biomarker reflecting the inflamed TME and immunotherapeutic response. Initially, we divided GC patients based on TAP1 expression levels alongside the co-expressed genes (CEGs). Several bioinformatics analyses demonstrated that the TAP1-high (TAP1-H) group exhibited an inflamed TME, indicative of an immune-hot tumor phenotype. Notably, within the immunotherapy cohort of GC, patients exhibiting a TAP1-H phenotype demonstrated a more favorable response to immunotherapeutic interventions. Taken together, this study yielded essential insights into the value of TAP1 in the identification of GC patients with an immune-hot TME and the prediction of their response to immunotherapy.
Methods
Dataset acquisition
The standardized RNA-sequencing profile together with the clinical annotations of patients in The Cancer Genome Atlas-stomach adenocarcinoma (TCGA-STAD) cohort, encompassing 389 GC patients, were acquired from the UCSC Xena website (https://xenabrowser.net/datapages/) (24) Additionally, the validation cohort GSE84437 (1) was sourced from the Gene Expression Omnibus (GEO) portal (https://www.ncbi.nlm.nih.gov/geo/). GSE84437 constitutes an array profile based on the GPL6947 platform (Illumina HumanHT-12 V3.0 Expression BeadChip; Illumina, San Diego, CA, USA), comprising 433 GC samples. To preprocess the array profiles, the limma package (25) within Bioconductor (http://www.bioconductor.org/) in R was utilized. Following background correction, quantile normalization, as well as probe summarization, the dataset containing 25,124 genes underwent further processing. In addition, the normalized gene expression profile of GC clinical cohorts subjected to anti-PD-1 therapy (PRJEB25780) was retrieved from Tumor Immune Dysfunction and Exclusion (http://tide.dfci.harvard.edu/). After preprocessing, a dataset with 25,946 genes and 45 diagnostic GC patients who received immunotherapy was prepared for subsequent analysis. Moreover, our study encompassed some immunotherapy cohorts of bladder cancer [GSE176307 (26) and IMvigor210CoreBiologies], breast cancer [GSE194040 (27)] and renal carcinomas (28). For bulk omics datasets, only samples with OS exceeding 0 days were considered in the present study. The study was conducted in accordance with the Declaration of Helsinki (as revised in 2013).
Identification of TAP1 CEGs in the TCGA cohort
As a systems biology approach, weighted gene co-expression network analysis (WGCNA) (29,30) was used to depict the correlation patterns among gene transcriptional levels across microarray samples. This method facilitates the identification of gene modules comprising highly correlated genes, their relationship with various sample traits, and the discovery of potential biomarkers associated with those traits. Recognizing the limitations of solely relying on TAP1 to distinguish between TAP1-H group and TAP1-low (TAP1-L) groups of GC patients, we pursued the identification of TAP1 CEGs to characterize the TAP1-related transcriptional phenotypes more effectively. To achieve this, we initially computed the variance of genes across the samples in the TCGA cohort. Subsequently, we selected the top 25% most variant genes (5,133 genes) to establish a weighted gene co-expression network aimed at identifying the TAP1 CEGs. Within this framework, gene significance (GS) denoted the link of gene expression with TAP1 transcriptional levels, whereas module membership (MM) signified the link of module eigengene with gene expression. Consequently, genes exhibiting GS values of ≥0.5 and MM values of ≥0.5 within the module showcasing the strongest correlation with TAP1 expression were chosen as TAP1 CEGs.
Identification of TAP1-related groups in the TCGA cohort
To ascertain the optimal number of stable TAP1-related subpopulations (31), we conducted consensus clustering and silhouette analysis utilizing the expression matrix of TAP1 in conjunction with the CEGs (Table 1). Consensus clustering, an algorithm providing quantitative/visual stability evidence to determine the number of unsupervised classes in a dataset (32), was employed in this study. We utilized the R package ConsensusClusterPlus (32) with parameters set to 1,000 iterations, 80% resampling, and k values ranging from 2 to 10. Silhouette analysis, a method for assessing clustering outcomes by calculating silhouette coefficients, which range from −1 to 1, confirmed this finding. A larger silhouette coefficient indicates improved clustering quality. Based on the consensus matrices and silhouette analysis, we determined the optimal number of TAP1-related groups. Next, k-means clustering (k=2, default parameters) was performed to divide the individuals in the TCGA cohort into two clusters on the transcriptional profile of TAP1 and its CEGs.
Table 1
RARRES3 | LAG3 | HLA-DMB | PRF1 | IL12RB1 | CD274 |
IDO1 | CXCL11 | CXCL10 | CXCR2P1 | CXCL9 | GZMA |
GZMB | GZMH | CIITA | HLA-DMA | IFNG | FASLG |
HLA-DRA | AIM2 | CD74 | ZNF683 | CCL5 | LOC400759 |
CXCR6 | NKG7 | ZBP1 | GBP5 | GBP4 |
CEGs, co-expressed genes; TAP1, transporter associated with antigen processing 1.
Classification of patients within test datasets
The TAP1-related groups that were identified from the TCGA cohort were further extended to testing datasets as follows: initially, the gene expression profiles of the training dataset as well as the test dataset were integrated. Besides, batch effects were mitigated using the R package “limma” (25). Subsequently, the prediction of patient classification was conducted utilizing the R package “pamr”, which employs a method named nearest shrunken centroids, utilizing “shrunken” centroids as representative prototypes for every group and discerning the genes that can accurately characterize each group (33).
Assessment of immunological characteristics of TME
To evaluate the immunological characteristics of the TME, we employed the Estimation of STromal and Immune cells in MAlignant Tumors using Expression data (ESTIMATE) algorithm (5), a tool that infers tumor purity (TP) as well as stromal/immune cell content from bulk transcriptomic profiles. It allowed for an assessment of TP, ESTIMATE score, immune score (IS), and stromal score (SS). Moreover, we gathered data on immunomodulators, such as MHC signatures, receptors, chemokines, and immune-stimulators, from published studies (34,35). To comprehensively analyze the immune status of each patient, we utilized the specific genes associated with 29 distinct immune cell types alongside pertinent pathways related to the immune system (36) to assess the levels of infiltration by various immune cell populations, as well as the functionality of immune-related pathways and processes for each individual. These calculations were performed utilizing the single-sample gene set enrichment analysis (ssGSEA) function within the R package “GSVA” (37).
Identification of differentially expressed genes (DEGs)
To distinguish DEGs for the TAP1-H group along with TAP1-L group, we conducted a differential expression analysis using the R package “limma” (25). Genes exhibiting a fold-change (FC) of at least 1.5 together with adjusted P values below 0.05 were classified as up-regulated genes in the TAP1-H group. Conversely, those not meeting these criteria were considered up-regulated for the TAP1-L group.
Enrichment analysis of gene functions and pathways
We conducted Gene Ontology (GO) as well as Kyoto Encyclopedia of Genes and Genomes (KEGG) pathway enrichment analyses utilizing the R package “clusterProfiler”. Subsequently, we provided an overview of the top 10 enriched pathways that exhibited significant enrichment based on the most notable P values.
Analysis of single-cell RNA sequencing datasets (scRNA-seq)
Considering the limitation of reflecting the immune cells populations and their activity in the tumor microsegment based on the omics transcriptomics datasets, we obtained scRNA-seq datasets from 29 GC patients through GSE183904 (38). Subsequent analyses were conducted utilizing the Seurat (4.0.4, http://satijalab.org/seurat/) R toolkit (39), encompassing quality control along with all related procedures. To mitigate the impact of either aberrant cells or technical background noise on subsequent analysis, cells were retained if they met the following criteria: (I) the expression level of mitochondrial genes constituted over 10% of the total gene expression; or (II) the number of the detected genes per cell fell within the range of 200 to 5,000. Consequently, 102,561 cells in total were utilized for further investigation.
To address potential impact of technical batches among individuals/experiments, we employed the “RunHarmony” function within the R package harmony (40) to integrate 102,561 cells from 29 GC patients. Besides, to effectively reduce dimensionality, a subset consisting of the top 4,000 genes with the highest variability was selected for principal component analysis (PCA). Subsequently, the integrated data matrix was further reduced to a two-dimensional space utilizing the first 30 principal components (PCs), which was then visualized through t-distributed stochastic neighbor embedding (t-SNE). To identify distinct cell clusters, we utilized a clustering algorithm based on shared nearest neighbor (SNN) modularity optimization. The algorithm was configured with a resolution parameter set at 1. To characterize these cells, existing markers such as von Willebrand factor (VWF) for endothelial cells (EnCs), epithelial cell adhesion molecule (EPCAM) for epithelial cells (EPCs), decorin (DCN) for fibroblasts (FB), and CD3D for TCs were utilized to validate the annotations of cell types.
Statistical analysis
Data analysis was conducted utilizing R software 4.0.4 (R Foundation for Statistical Computing, Vienna, Austria). For continuous variables, we assessed the group disparities based on the Wilcoxon rank-sum test. Meanwhile, difference of categorical variables was measured utilizing the Fisher exact test. To measure the correlation of continuous variables, Pearson’s correlation analysis was employed. Throughout all analyses, P<0.05 (two-tailed) indicated statistical significance, and we displayed the labels as follows: *, P<0.05; **, P<0.01; and ***, P<0.001.
Results
Comparison of TAP1 expression among patients with various clinicopathology characteristics
TAP1 expression exhibited no obvious difference among patients displaying varying clinicopathological characteristics. Consistent with clinical observations (41), GC patients displaying high TAP1 expression demonstrated favorable OS (Figure 1A). Given previous findings indicating the pivotal role of TAP1 in tumorigenesis and progression in certain carcinomas, we proceeded to assess the correlation of TAP1 expression with the clinicopathological features of GC within the TCGA cohort. The results, depicted in Figure 1B, indicated no differences with statistical significance among patients displaying various clinicopathological features. Specifically, TAP1 expression was similar among patients varying clinical/tumor-node-metastasis (TNM) stages (Figure 1C-1G), suggesting TAP1 and any clinicopathological features within the TCGA cohort. Considering the biological roles of TAP1 as reported in previous research, higher transcriptional levels of TAP1 might be linked to an activated immune response. Consequently, we compared the correlation between TAP1 and immune-related characteristics. We adopted the ESTIMATE algorithm to evaluate TP and immune infiltration of TME. In line with previous findings, our findings indicated that TAP1 had a positive link with IS/ESTIMATE score, but a negative link with TP (Figure 1H-1J), suggesting that elevated TAP1 expression may be associated with immune cell infiltration. Importantly, we discovered that the levels of immune infiltration exhibited no variance among patients with differing therapy history and clinicopathological stages (Figure S1A,S1B), implying that these clinical factors did not impact the immune status of the patients.
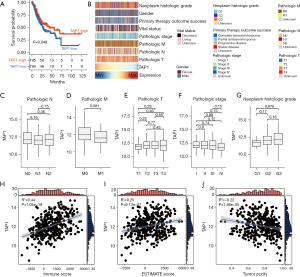
Identification of TAP1-related GC subtypes
Given the absence of significant differences in TAP1 expression among patients with various clinicopathological characteristics and its positive correlation with IS, we explored the link of TAP1 expression with specific immunological characteristics that reflect the immune status of TME. Initially, we categorized patients within the TCGA cohort into distinct TAP1-associated GC subtypes. To ensure a systematic classification, we constructed a weighted gene co-expression network utilizing the R package WGCNA to identify TAP1’s CEGs within the TCGA cohort. Establishing a hierarchical network using the soft threshold—power of β=3 (with a hierarchical network R2=0.9, Figure 2A,2B), we identified nine gene modules coded by colors, excluding the gray module (Figure 2C). Notably, the brown module exhibited the strongest link with TAP1 expression (R2=0.39, P<0.001, Figure 2D). The scatter plots displayed the values of GS as well as MM for the brown module related to TAP1 messenger RNA (mRNA) levels (Figure 2E). CEGs showing GS ≥0.5 as well as MM ≥0.5 were the CEGs chosen for further study. Additionally, the results from enriched biological functions indicated a strong association of TAP1 and its CEGs with signaling pathways related to the immune response, such as MHC-related activities as well as TC activation (Figure S2), aligning with the functional role of TAP1.
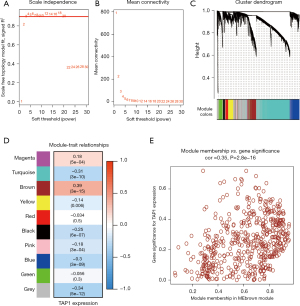
Subsequently, we classified patients within the TCGA cohort in accordance with the expression matrix of TAP1 alongside the CEGs. On account of the consensus clustering matrixes and the number of tests supporting the cluster number from the NbClust testing, we determined the optional cluster number to be two (Figures S3,S4). Silhouette analysis further confirmed the stability of two clusters (Figure 3A). Subsequently, utilizing k-means clustering, the 389 patients within the TCGA cohort were stratified into two subgroups (Figure 3B,3C), comprising 169 and 220 patients in the TAP1-H and TAP1-L groups, respectively. Following this, DEGs were analyzed to identify DEGs for either the TAP1-H group or TAP1-L group (Figure 3D). Significantly, results from enriched biological functions revealed that genes up-regulated among the TAP1-H group were strongly linked with signaling pathways related to the immune response, including the regulation/activation of TCs, cell-cell adhesion of leukocytes, as well as the production of cytokines (Figure 3E). Corresponding to these biological pathways, patients exhibiting the TAP1-H phenotype demonstrated higher IS, SS, and ESTIMATE scores, but lower levels of TP (all P<0.001, Figure 3F-3I). These findings collectively indicate a positive correlation between TAP1 expression and an inflamed TME.
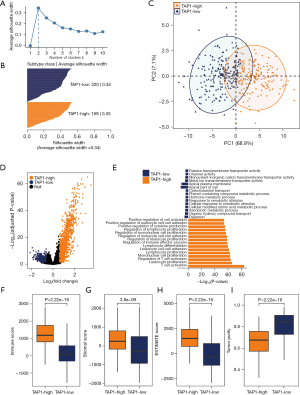
Correlation of TAP1-related GC subtypes with immune infiltration
It was observed that patients with a TAP1-H phenotype displayed an infiltrating TME as well as the activated specific signaling pathways linked to the immune response. Subsequently, we conducted an in-depth analysis of the immunological features of TAP1-H and TAP1-L groups within the TCGA cohort. As shown in Figure 4A-4D, patients exhibiting a TAP1-H phenotype demonstrated notably higher enrichment scores of chemokines, paired receptors, MHC molecules, as well as immunomodulators, all of which are implicated in the recruitment of effector tumor-inflamed immune cells, including CD8+ TCs, macrophages, and antigen-presenting cells (Figure 4E). Furthermore, employing 4 independent algorithms, patients with a TAP1-H phenotype displayed a greater abundance of various immune cell types, including CD8+ TCs, B cells, and macrophages (Figure S5). Simultaneously, the TAP1-H group exhibited heightened activity within immune-related pathways, including up-regulation of MHC class I and promotion of inflammation (Figure 4E), signifying a tendency for these patients to possess a TME that was more inflamed. Moreover, the ICI expressions like PD-1 or PD-L1 was elevated in inflamed TME. Correspondingly, our research revealed a positive correlation between TAP1 and most ICIs, encompassing PD-1, PD-L1, and CTLA4 (Figure 4F).
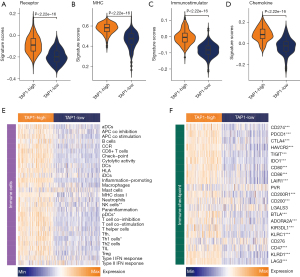
Finally, we further corroborated these findings in the GSE84437 cohort. Utilizing the nearest shrunken centroids method, 166 patients in the GSE84437 cohort were identified as the TAP1-H group, while 267 patients were determined as the TAP1-L group (Figure 5A). Notably, transcriptional levels of TAP1 exhibited favorable accuracy in distinguishing between the TAP1-H and TAP1-L groups within the GSE84437 cohort [area under the curve (AUC) =0.719, Figure S6]. In consistency with the findings observed in the TCGA cohort, TAP1-H group demonstrated markedly higher levels of IS, SS, and ESTIMATE scores; in contrast, a lower level of TP was observed (Figure 5B). Furthermore, individuals displaying the TAP1-H phenotype also exhibited enhanced enrichment scores of immunomodulatory factors as well as immune-related signaling pathways with statistical significance (Figure 5C-5G). Concurrently, a majority of ICIs were highly expressed among the TAP1-H group (Figure 5H). Overall, our results suggest a close correlation of TAP1 with the development of an inflamed TME, potentially helping to identify the immunogenicity of breast cancer.

Considering the limitation of reflecting the immune cells populations and their activity in the tumor microsegment based on the omics transcriptomics datasets, we expanded our study by incorporating scRNA-seq datasets from 29 patients with GC (Figure S7A). This comprehensive dataset consisted of 102,561 individual cells that met quality control criteria, followed by the unsupervised clustering into 37 clusters (Figure S7B). Following this, these clusters were annotated into 9 distinct cell types comprising tumor cells, B cells, plasma cells, EnCs, EPCs, FBs, myeloid cells, mast cells, as well as TCs (Figure 6A-6C) complying with the expression levels of canonical markers. Given the pivotal role of TCs in anti-tumor activity, we further investigated the link of TAP1 expression with the percentage of TCs. Our findings confirmed a positive link of TAP1 expression with TC infiltration (R2=0.32, P=0.06, Figure 6D), further indicating that high TAP1 expression is associated with the infiltration of TCs exhibiting heightened anti-tumor activity, as evidenced by their high expression of cytotoxic signatures such as GNLY and PRF1 (Figure 6E).
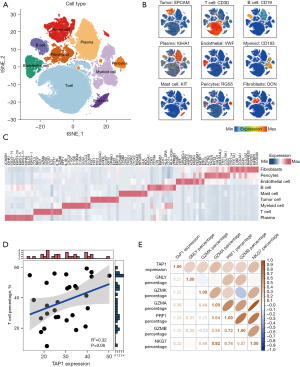
Prediction of immunotherapeutic response by TAP1
Previous research has established the functional role of TAP1 in transporting antigens from the cytoplasm to the endoplasmic reticulum for the association with MHC class I molecules, while also serving as a molecular scaffold for the final stage of MHC class I folding. These findings underline the value of TAP1 in the assessment of anti-tumor activity. Consequently, we investigated the predictive values of TAP1 among GC patients with immunotherapy. Initially, individuals within the PRJEB25780 cohort were categorized into either a TAP1-H group or TAP1-L group on the basis of gene expression profiles (Figure 7A). The AUC results confirmed the efficacy of TAP1 in successfully classifying the TAP1 groups within the PRJEB25780 cohort (AUC =0.760, Figure S8). Besides, the increase of TAP1 expression was accompanied by the enrichment of immune cells in the PRJEB25780 immunotherapy cohort (R2=0.45, P=0.002, Figure 7B). Also, compared to the TAP1-L group, the TAP1-H group displayed higher levels of inflamed immune cell subpopulations as well as ICIs (Figure 7C,7D), consistent with results observed in the TCGA and GSE84437 cohorts. Notably, a significant difference was observed in the composition of TAP1-H and TAP1-L groups between patients who achieved complete remission (CR) after immunotherapy and those who did not (NR) (P=0.04, Figure 7E). Specifically, 75% of patients achieving CR exhibited the TAP1-H phenotype, whereas approximately 65% of patients in the NR groups possessed the TAP1-L subtype (Figure 7E), indicating that TAP1-H group may be more sensitive to immunotherapy and easier to achieve CR following treatment. Additionally, the up-regulation of TAP1 was positively correlated with an increase in PD-L1 expression (R2=0.64, P<0.001, Figure 7F).
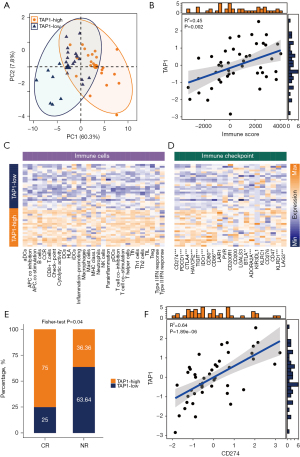
Furthermore, the predictive ability of TAP1 among patients undergoing immunotherapy was explored in other immunotherapy cohorts of bladder cancer, breast cancer, and renal carcinomas, whose tumor cells, akin to those in GC, originated from EPCs. The results indicated that patients achieving remission after immunotherapy exhibited higher levels of TAP1 expression (Figure S9). Significantly, in these immunotherapy cohorts, TAP1 expression demonstrated favorable accuracy in distinguishing patients with varying immunotherapeutic responses (GSE176307: AUC =0.686; IMvigor210CoreBiologies: AUC =0.597; GSE194040: AUC =0.789; renal carcinoma: AUC =0.926; Figure S9).
Altogether, the cumulative findings indicate a positive correlation between TAP1 and an immune-hot TME, revealing its potential as a new biomarker for the prediction of a favorable immunotherapeutic response in GC. Immunohistochemical staining also revealed significant differences in TAP1 protein expression between the TAP1-high and TAP1-low groups (Figure 8). Furthermore, TAP1 overexpression is positively correlated with CD8. Thus, immune cells are enriched in patients with high TAP1 expression, which may result in better outcomes.
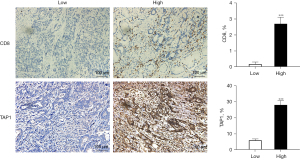
Discussion
GC is one of the most prevalent gastrointestinal malignancies, contributing significantly to cancer-related mortality worldwide (2). Despite a substantial shift in our understanding of GC stemming from the increasingly comprehensive delineation of its molecular characteristics (42,43), considerable controversy persists across all facets of GC treatment (43). Given the widespread use of ICIs in numerous solid tumors, several studies have explored the safety as well as the efficacy of ICIs in GC. Recent clinical investigations have noted that a PD-1 antibody named toripalimab has exhibited a good safety profile as well as promising antitumor activity among individuals with advanced GC, particularly when used in combination with XELOX (7,31,44). Notably, Wang et al. discovered that high tumor mutation burden, rather than PD-1 expression, may serve as a prognostic marker for OS among advanced GC administered with toripalimab as a single agent (45). This finding underscores the limitations of conventional immune checkpoints in predicting the clinical outcomes of individuals with GC.
Based on previous research, tumors represent intricate masses comprising both malignant cells and significant subpopulations of normal cells, including CD8+ TCs along with macrophages. Complicated interactions of these cells, mediated by cytokines, chemokines, and growth factors, shape the TME (46). Through the interplay of diverse cell subpopulations within the TME, this microenvironment may significantly influence treatment response and prognosis (47,48). In addition, tumors may be categorized as either “cold” or “hot” on the basis of their respective TME composition (14). Specifically, cold tumors could manifest an immunosuppressive TME, resulting in the resistance to chemotherapy/immunotherapy (49), whereas hot tumors exhibit greater sensitivity to these mentioned therapies, featured by TC infiltration within an immunosuppressive TME (14,50,51). Overall, hot tumors display a more favorable immunotherapeutic response, including to anti-PD-1/PD-L1 therapy. Hence, the distinguishment of hot tumors with cold tumors represents a good approach for predicting responses to immunotherapy.
In recent decades, bulk transcriptional omics have been extensively employed to identify biomarkers that can differentiate between patients with distinct phenotypes. However, bulk sequencing only yields average expression levels across various cell states and lacks the ability to capture specific characteristics of individual cell subsets. Consequently, biomarkers identified solely based on bulk omics datasets may be predominantly expressed in non-tumor cells, such as immune or stromal subpopulations. This lack of tumor specificity could potentially limit the applicability of these signatures. The advent of single-cell sequencing technologies has enabled the comprehensive quantification of the entire genome or transcriptome of each individual cell within a mixture of tissues, presenting an unprecedented chance to unravel the intricacies of cellular heterogeneity in GC (38,52). Therefore, integrating bulk omics and single-cell datasets enables a more precise identification of tumor-specific signatures capable of distinguishing between immune-hot and -cold tumors, predicting clinical outcomes, and potentially serving as therapeutic targets.
Recent comprehensive studies have shed light on the pivotal role of TAP1, a member of the ABC family, in transporting antigen peptides from the cytoplasm to the endoplasmic reticulum lumen, where they are loaded onto MHC class I molecules (17). Additionally, TAP1 contributes to providing endogenous protein peptides for CD8+ cytotoxic TCs, thereby playing a crucial part in maintaining immune system function (18). Although the up-regulation of TAP1 has been linked to improved targeting of tumor cells by antigen-presenting cells, mounting evidence suggests that TAP1 is closely associated with various carcinomas, potentially tied to tumor cells evading recognition by cytotoxic TCs through peptide delivery shutdown mechanisms (19-21). In prostate cancer, Chow et al. demonstrated a consistent association between amplified TAP1 copy numbers and histological ductal variation (22). Furthermore, the transcriptional levels of TAP1 have been found to be associated with the immune response. Specifically, the downregulation or epigenetic silencing of TAP1 in tumor cells contributes to promoting tumor survival and facilitating immune evasion (20,21). Moreover, down-regulation of TAP1, mediated by miR-200a-5p, has been linked to reduced human leukocyte antigen class I (HLA-I) expression, resulting in tumor cells evading anti-tumor responses and poor prognosis among patients with melanoma tumors (53). Notably, after decitabine treatment, levels of TAP1 as well as CD80 have been shown to have decreased in stem cells of breast cancer, diminishing TC recognition and killing effects while promoting tumor cell growth (21), suggesting TAP1’s potential contribution to tumor progression and drug resistance by influencing tumor immune infiltration. Additionally, TAP1 plays a role in anti-PD-1 antibody immunotherapy mediated by IL-27 (23). Loss of TAP1 expression can change the immune microenvironment and take part in reversing the resistance to anti-PD-1 therapy (54). Cumulatively, these findings indicate an association between TAP1, an active immune microenvironment, and immunotherapeutic response. Nonetheless, the critical role of TAP1 in recognizing the immune status of tumors in GC has yet to be evaluated.
Hence, in this study, we initially observed that the level of TAP1 transcription were not linked to pathological stages; however, they were positively correlated with IS. These results were in line with prior research, indicating no significant difference in TAP1 transcriptional levels between early and advanced GC patients, implying a general rather than subtype-specific role for TAP1. Building on this discovery, patients were methodically allocated into distinct groups on the basis of TAP1 expression. Initially, the algorithm of WGCNA was employed to identify CEGs of TAP1. Subsequently, several bioinformatics methods were conducted utilizing the gene expression matrix. The patients obtained from the TCGA-STAD cohort were then categorized into either a TAP1-H group or TAP1-L group. In-depth exploration of immunological characteristics within these groups revealed that individuals with the TAP1-H phenotype consistently displayed a more active immune TME. Specifically, TAP1 exhibited a positive correlation with the enrichment of immunomodulators as well as tumor-infiltrating immune cells, promoting cell recruitment alongside the advancement of an inflamed TME. Concurrently, it was noted that well-known ICI expressions were markedly high among TAP1-H patients. Subsequent analysis within the GC patients with immunotherapy indicated that high TAP1 expression level was linked to an improved immunotherapeutic response as well as an increased level of PD-L1 expression. Taken together, our collective findings strongly suggest that TAP1 serves as a novel biomarker for the identification of an immune-hot TME and the prediction of a favorable immunotherapeutic response in GC.
Conclusions
Our comprehensive bioinformatics analyses revealed that TAP1-H GC patients exhibited a TME with increased infiltration of immune cell subpopulations alongside the activated immune-related signaling pathways. Additionally, TAP1-H individuals were more sensitive to immunotherapy. Moreover, the up-regulation of TAP1 correlated with heightened transcriptional levels of PD-L1, indicating that TAP1 serves as a novel biomarker capable of identifying an immune-hot TME and predicting a favorable immunotherapeutic response in GC.
Acknowledgments
Funding: This work was partially supported by
Footnote
Peer Review File: Available at https://jgo.amegroups.com/article/view/10.21037/jgo-24-28/prf
Conflicts of Interest: All authors have completed the ICMJE uniform disclosure form (available at https://jgo.amegroups.com/article/view/10.21037/jgo-24-28/coif). The authors have no conflicts of interest to declare.
Ethical Statement: The authors are accountable for all aspects of the work in ensuring that questions related to the accuracy or integrity of any part of the work are appropriately investigated and resolved. The study was conducted in accordance with the Declaration of Helsinki (as revised in 2013).
Open Access Statement: This is an Open Access article distributed in accordance with the Creative Commons Attribution-NonCommercial-NoDerivs 4.0 International License (CC BY-NC-ND 4.0), which permits the non-commercial replication and distribution of the article with the strict proviso that no changes or edits are made and the original work is properly cited (including links to both the formal publication through the relevant DOI and the license). See: https://creativecommons.org/licenses/by-nc-nd/4.0/.
References
- Smyth EC, Nilsson M, Grabsch HI, et al. Gastric cancer. Lancet 2020;396:635-48. [Crossref] [PubMed]
- Sung H, Ferlay J, Siegel RL, et al. Global Cancer Statistics 2020: GLOBOCAN Estimates of Incidence and Mortality Worldwide for 36 Cancers in 185 Countries. CA Cancer J Clin 2021;71:209-49. [Crossref] [PubMed]
- Jim MA, Pinheiro PS, Carreira H, et al. Stomach cancer survival in the United States by race and stage (2001-2009): Findings from the CONCORD-2 study. Cancer 2017;123:4994-5013. [Crossref] [PubMed]
- Emens LA. Breast Cancer Immunotherapy: Facts and Hopes. Clin Cancer Res 2018;24:511-20. [Crossref] [PubMed]
- Li K, Zhang A, Li X, et al. Advances in clinical immunotherapy for gastric cancer. Biochim Biophys Acta Rev Cancer 2021;1876:188615. [Crossref] [PubMed]
- Fuchs CS, Doi T, Jang RW, et al. Safety and Efficacy of Pembrolizumab Monotherapy in Patients With Previously Treated Advanced Gastric and Gastroesophageal Junction Cancer: Phase 2 Clinical KEYNOTE-059 Trial. JAMA Oncol 2018;4:e180013. [Crossref] [PubMed]
- Janjigian YY, Shitara K, Moehler M, et al. First-line nivolumab plus chemotherapy versus chemotherapy alone for advanced gastric, gastro-oesophageal junction, and oesophageal adenocarcinoma (CheckMate 649): a randomised, open-label, phase 3 trial. Lancet 2021;398:27-40. [Crossref] [PubMed]
- Kang YK, Boku N, Satoh T, et al. Nivolumab in patients with advanced gastric or gastro-oesophageal junction cancer refractory to, or intolerant of, at least two previous chemotherapy regimens (ONO-4538-12, ATTRACTION-2): a randomised, double-blind, placebo-controlled, phase 3 trial. Lancet 2017;390:2461-71. [Crossref] [PubMed]
- Shitara K, Ajani JA, Moehler M, et al. Nivolumab plus chemotherapy or ipilimumab in gastro-oesophageal cancer. Nature 2022;603:942-8. [Crossref] [PubMed]
- Zhao JJ, Yap DWT, Chan YH, et al. Low Programmed Death-Ligand 1-Expressing Subgroup Outcomes of First-Line Immune Checkpoint Inhibitors in Gastric or Esophageal Adenocarcinoma. J Clin Oncol 2022;40:392-402. [Crossref] [PubMed]
- Zeng D, Wu J, Luo H, et al. Tumor microenvironment evaluation promotes precise checkpoint immunotherapy of advanced gastric cancer. J Immunother Cancer 2021;9:e002467. [Crossref] [PubMed]
- Nanda R, Chow LQ, Dees EC, et al. Pembrolizumab in Patients With Advanced Triple-Negative Breast Cancer: Phase Ib KEYNOTE-012 Study. J Clin Oncol 2016;34:2460-7. [Crossref] [PubMed]
- Yang Y. Cancer immunotherapy: harnessing the immune system to battle cancer. J Clin Invest 2015;125:3335-7. [Crossref] [PubMed]
- Gajewski TF, Corrales L, Williams J, et al. Cancer Immunotherapy Targets Based on Understanding the T Cell-Inflamed Versus Non-T Cell-Inflamed Tumor Microenvironment. Adv Exp Med Biol 2017;1036:19-31. [Crossref] [PubMed]
- Hu R, Han Q, Zhang J. STAT3: A key signaling molecule for converting cold to hot tumors. Cancer Lett 2020;489:29-40. [Crossref] [PubMed]
- Zemek RM, De Jong E, Chin WL, et al. Sensitization to immune checkpoint blockade through activation of a STAT1/NK axis in the tumor microenvironment. Sci Transl Med 2019;11:eaav7816. [Crossref] [PubMed]
- Leone P, Shin EC, Perosa F, et al. MHC class I antigen processing and presenting machinery: organization, function, and defects in tumor cells. J Natl Cancer Inst 2013;105:1172-87. [Crossref] [PubMed]
- Praest P, Luteijn RD, Brak-Boer IGJ, et al. The influence of TAP1 and TAP2 gene polymorphisms on TAP function and its inhibition by viral immune evasion proteins. Mol Immunol 2018;101:55-64. [Crossref] [PubMed]
- Lage H, Perlitz C, Abele R, et al. Enhanced expression of human ABC-transporter tap is associated with cellular resistance to mitoxantrone. FEBS Lett 2001;503:179-84. [Crossref] [PubMed]
- Ling A, Löfgren-Burström A, Larsson P, et al. TAP1 down-regulation elicits immune escape and poor prognosis in colorectal cancer. Oncoimmunology 2017;6:e1356143. [Crossref] [PubMed]
- Sultan M, Vidovic D, Paine AS, et al. Epigenetic Silencing of TAP1 in Aldefluor(+) Breast Cancer Stem Cells Contributes to Their Enhanced Immune Evasion. Stem Cells 2018;36:641-54. [Crossref] [PubMed]
- Chow K, Bedő J, Ryan A, et al. Ductal variant prostate carcinoma is associated with a significantly shorter metastasis-free survival. Eur J Cancer 2021;148:440-50. [Crossref] [PubMed]
- Carbotti G, Nikpoor AR, Vacca P, et al. IL-27 mediates HLA class I up-regulation, which can be inhibited by the IL-6 pathway, in HLA-deficient Small Cell Lung Cancer cells. J Exp Clin Cancer Res 2017;36:140. [Crossref] [PubMed]
- Yoon SJ, Park J, Shin Y, et al. Deconvolution of diffuse gastric cancer and the suppression of CD34 on the BALB/c nude mice model. BMC Cancer 2020;20:314. [Crossref] [PubMed]
- Ritchie ME, Phipson B, Wu D, et al. limma powers differential expression analyses for RNA-sequencing and microarray studies. Nucleic Acids Res 2015;43:e47. [Crossref] [PubMed]
- Rose TL, Weir WH, Mayhew GM, et al. Fibroblast growth factor receptor 3 alterations and response to immune checkpoint inhibition in metastatic urothelial cancer: a real world experience. Br J Cancer 2021;125:1251-60. [Crossref] [PubMed]
- Wolf DM, Yau C, Wulfkuhle J, et al. Redefining breast cancer subtypes to guide treatment prioritization and maximize response: Predictive biomarkers across 10 cancer therapies. Cancer Cell 2022;40:609-623.e6. [Crossref] [PubMed]
- de Block T, Laumen JGE, Van Dijck C, et al. WGS of Commensal Neisseria Reveals Acquisition of a New Ribosomal Protection Protein (MsrD) as a Possible Explanation for High Level Azithromycin Resistance in Belgium. Pathogens 2021;10:384. [Crossref] [PubMed]
- Langfelder P, Horvath S. WGCNA: an R package for weighted correlation network analysis. BMC Bioinformatics 2008;9:559. [Crossref] [PubMed]
- Zeng L, Wang X, Wang F, et al. Identification of a Gene Signature of Cancer-Associated Fibroblasts to Predict Prognosis in Ovarian Cancer. Front Genet 2022;13:925231. [Crossref] [PubMed]
- Cai Y, Cheng Y, Wang Z, et al. A novel metabolic subtype with S100A7 high expression represents poor prognosis and immuno-suppressive tumor microenvironment in bladder cancer. BMC Cancer 2023;23:725. [Crossref] [PubMed]
- Wilkerson MD, Hayes DN. ConsensusClusterPlus: a class discovery tool with confidence assessments and item tracking. Bioinformatics 2010;26:1572-3. [Crossref] [PubMed]
- Tibshirani R, Hastie T, Narasimhan B, et al. Diagnosis of multiple cancer types by shrunken centroids of gene expression. Proc Natl Acad Sci U S A 2002;99:6567-72. [Crossref] [PubMed]
- Charoentong P, Finotello F, Angelova M, et al. Pan-cancer Immunogenomic Analyses Reveal Genotype-Immunophenotype Relationships and Predictors of Response to Checkpoint Blockade. Cell Rep 2017;18:248-62. [Crossref] [PubMed]
- Mei J, Fu Z, Cai Y, et al. SECTM1 is upregulated in immuno-hot tumors and predicts immunotherapeutic efficacy in multiple cancers. iScience 2023;26:106027. [Crossref] [PubMed]
- Bindea G, Mlecnik B, Tosolini M, et al. Spatiotemporal dynamics of intratumoral immune cells reveal the immune landscape in human cancer. Immunity 2013;39:782-95. [Crossref] [PubMed]
- Ferreira MR, Santos GA, Biagi CA, et al. GSVA score reveals molecular signatures from transcriptomes for biomaterials comparison. J Biomed Mater Res A 2021;109:1004-14. [Crossref] [PubMed]
- Kumar V, Ramnarayanan K, Sundar R, et al. Single-Cell Atlas of Lineage States, Tumor Microenvironment, and Subtype-Specific Expression Programs in Gastric Cancer. Cancer Discov 2022;12:670-91. [Crossref] [PubMed]
- Butler A, Hoffman P, Smibert P, et al. Integrating single-cell transcriptomic data across different conditions, technologies, and species. Nat Biotechnol 2018;36:411-20. [Crossref] [PubMed]
- Korsunsky I, Millard N, Fan J, et al. Fast, sensitive and accurate integration of single-cell data with Harmony. Nat Methods 2019;16:1289-96. [Crossref] [PubMed]
- Segami K, Aoyama T, Hiroshima Y, et al. Clinical Significance of TAP1 and DLL4 Expression in Patients With Locally Advanced Gastric Cancer. In Vivo 2021;35:2771-7. [Crossref] [PubMed]
- Chia NY, Tan P. Molecular classification of gastric cancer. Ann Oncol 2016;27:763-9. [Crossref] [PubMed]
- Joshi SS, Badgwell BD. Current treatment and recent progress in gastric cancer. CA Cancer J Clin 2021;71:264-79. [Crossref] [PubMed]
- Qiu H. Safety and efficacy of toripalimab in advanced gastric cancer: A new clinical trial bringing hope for immunotherapy in gastric cancer. Cancer Commun (Lond) 2020;40:194-6. [Crossref] [PubMed]
- Wang F, Wei XL, Wang FH, et al. Safety, efficacy and tumor mutational burden as a biomarker of overall survival benefit in chemo-refractory gastric cancer treated with toripalimab, a PD-1 antibody in phase Ib/II clinical trial NCT02915432. Ann Oncol 2019;30:1479-86. [Crossref] [PubMed]
- Gout DY, Groen LS, van Egmond M. The present and future of immunocytokines for cancer treatment. Cell Mol Life Sci 2022;79:509. [Crossref] [PubMed]
- Deepak KGK, Vempati R, Nagaraju GP, et al. Tumor microenvironment: Challenges and opportunities in targeting metastasis of triple negative breast cancer. Pharmacol Res 2020;153:104683. [Crossref] [PubMed]
- Jin MZ, Jin WL. The updated landscape of tumor microenvironment and drug repurposing. Signal Transduct Target Ther 2020;5:166. [Crossref] [PubMed]
- Bonaventura P, Shekarian T, Alcazer V, et al. Cold Tumors: A Therapeutic Challenge for Immunotherapy. Front Immunol 2019;10:168. [Crossref] [PubMed]
- Cai Y, Ji W, Sun C, et al. Interferon-Induced Transmembrane Protein 3 Shapes an Inflamed Tumor Microenvironment and Identifies Immuno-Hot Tumors. Front Immunol 2021;12:704965. [Crossref] [PubMed]
- Mao W, Cai Y, Chen D, et al. Statin shapes inflamed tumor microenvironment and enhances immune checkpoint blockade in non-small cell lung cancer. JCI Insight 2022;7:e161940. [Crossref] [PubMed]
- Zhang P, Yang M, Zhang Y, et al. Dissecting the Single-Cell Transcriptome Network Underlying Gastric Premalignant Lesions and Early Gastric Cancer. Cell Rep 2019;27:1934-1947.e5. [Crossref] [PubMed]
- Bianchi G, Cusi D, Vezzoli G. Role of cellular sodium and calcium metabolism in the pathogenesis of essential hypertension. Semin Nephrol 1988;8:110-9. [PubMed]
- Zhang X, Sabio E, Krishna C, et al. Qa-1(b) Modulates Resistance to Anti-PD-1 Immune Checkpoint Blockade in Tumors with Defects in Antigen Processing. Mol Cancer Res 2021;19:1076-84. [Crossref] [PubMed]