Identification and validation of Cystatin A as a novel promising therapeutic target for gastric cancer
Highlight box
Key findings
• Screening out 11 genes highly correlated with recurrence-free survival (RFS) of gastric cancer (GC).
• The strong correlation between genes related to RFS and early differentiated fibroblasts.
• Cystatin A (CSTA) affects the drug sensitivity of GC cells to cisplatin through the caspase pathway.
What is known and what is new?
• CSTA was found downregulated in tumor tissues with metastasis compared with those without metastasis. Overall survival was found with lower CSTA expression.
• Using functional assays, we found that CSTA prevented in vitro apoptosis and motility of GC cells, as well as, the drug sensitivity to cisplatin through the caspase pathway.
What is the implication, and what should change now?
• Our study suggests that the CSTA could be of therapeutic value for inhibiting GC progression. Further research should be conducted, especially on its function in tumor cells or macrophages and its mechanism of action in the tumor microenvironment
Introduction
Gastric cancer (GC) is a prevalent global malignancy, consistently ranking among the top five most frequently diagnosed cancers worldwide each year. It is estimated that over 1 million new cases of GC are diagnosed annually and that it is the third leading cause of cancer-related death globally (1,2). Data from most countries indicate that drug treatments for GC include chemotherapy, targeted therapy, and immunotherapy, among other modalities (3,4).
Chemotherapy has been shown to improve the survival of patients (5,6). The median overall survival of GC patients who receive combination chemotherapy regimens is approximately 1 year (7,8). Approximately 17–20% of GC patients are human epidermal growth factor receptor 2 (HER2)-positive (9). HER2-positive cancer patients can benefit from targeted therapy with HER2 monoclonal antibodies. However, neither pertuzumab (first-line) nor trastuzumab-emtansine (second-line) treatments have been shown to effectively improve survival in GC patients with high HER2 expression (8). Immune checkpoint inhibitors have been used in the treatment of chemotherapy-refractory GC patients who experience tumor progression after first- or second-line therapy. Results from the phase III ATTRACTION-2 trial showed improved overall survival in Asian patients treated with the anti-programmed cell death protein-1 (PD-1) monoclonal antibody nivolumab (10,11). In the current GC treatment landscape, such drugs have limited biological efficacy and significant side effects, thereby, not as a first-line treatment for GC.
The above-mentioned drug therapies for GC all have their own limitations. Therefore, it is of great scientific significance to explore the molecular mechanisms of GC development in depth and search for new molecules that can significantly prolong the survival period of patients. Recurrence-free survival (RFS) refers to the time from complete remission after anti-tumor treatment until recurrence or the end of follow-up. Longer RFS after anti-tumor treatment indicates better treatment efficacy (12-14). In our quest to identify novel molecules with potential impact on the RFS of GC patients, we employed bioinformatics and discovered that Cystatin A (CSTA) expression exerts a positive influence on patient survival.
CSTA, a member of the cysteine proteinase inhibitor superfamily located on chromosome 3q21.1, is involved in late-stage keratinocyte differentiation and has long been implicated in cell adhesion (15,16). Recent findings demonstrate decreased CSTA expression in cancer tissues, while showing an association with tumor differentiation (17-20). However, most studies have focused on the correlation between CSTA expression levels and tumors rather than its inhibitory mechanisms. This study unveiled that CSTA inhibits cellular motility in vitro and exerts anti-cancer effects by enhancing the Caspase pathway, indicating potential as a therapeutic target for GC.
This study sought to identify the genes associated with RFS in GC patients using bioinformatics methods and to explore their roles and mechanisms. Our findings provide a theoretical basis for GC research and novel insights into the treatment of GC. We present this article in accordance with the MDAR reporting checklist (available at https://jgo.amegroups.com/article/view/10.21037/jgo-23-941/rc).
Methods
Data sources
Microarray data were retrieved from the Gene Expression Omnibus (GEO; https://www.ncbi.nlm.nih.gov/geo/) database. The GSE54129 data set with transcripts per kilobase of exon model per million mapped reads (TPM) transcriptomic comprises 111 tumor samples from GC patients and 21 normal samples. In addition, RNA-sequencing data and corresponding clinical information for GC were obtained from The Cancer Genome Atlas (TCGA) database (https://portal.gdc.com). The single-cell sequencing GSE183904 (21) data set was obtained from the GEO database.
Immune checkpoint blockade immunotherapeutic (ICB) cohorts with Fragments Per Kilobase of exon model per Million mapped fragments (FPKM) transcriptomic were collected from the public database [IMvigor210 (2018, anti-programmed cell death 1-ligand 1 (PD-L1), urothelial cancer (22)]. All patients had immune response in the cohort.
DEGs in GSE54129 data set and TCGA
The ‘limma’ package in R software was used to screen the differentially expressed genes (DEGs) of the GSE54129 and TCGA data sets. Adjusted P values were analyzed to correct for false-positive results. An adjusted P value <0.05 and a log (fold change) >1 for upregulation or a log (fold change) <−1 for downregulation were defined as the screening criteria. Next, a four-set Venn diagram from InteractiVenn (http://www.interactivenn.net/) was used to show the overlapping upregulated and downregulated DEGs. A batch survival analysis was performed on the overlapping genes and genes with significant P values were considered prognostic-related genes. Next, a Venn diagram was used to screen the cross-over genes between the prognostic-related genes and overlapping genes. Subsequently, Gene Ontology (GO) and Kyoto Encyclopedia of Genes and Genomes (KEGG) pathway enrichment analyses of the overlapping genes were performed using the ‘clusterProfiler’ package. P values were adjusted using the Benjamini-Hochberg method. Adjusted P values <0.05 were considered statistically significant.
Development of a prognostic risk-score model in TCGA cohort
To assess the prognostic value of the 23 overlapping genes, we then conducted a least absolute shrinkage and selection operator (LASSO)-Cox regression analysis to evaluate the association between each gene and survival status in TCGA cohort. The candidate genes were screened and prognostic models were developed using LASSO-Cox regression models (R package “glmnet”). Ultimately, 11 genes and their coefficients were identified, and the penalty parameter (λ) was determined by the minimum criterion. The risk-score formula was expressed as follows:
(0.0402) × C3 + (0.0692) × ESRRG + (0.0544) × CSTA + (0.0623) × CIDEC + (0.1013) × NNMT + (0.1491) × RDH12 + (−0.0805) × CXCL1 + (0.0493) × MFAP2 + (0.0792) × SERPINE1 + (−0.0592) × TP53 + (0.0934) × PSAPL1.
TCGA-GC patients were divided into low- and high-risk subgroups according to the median risk score, and the RFS times of the two subgroups were compared by a Kaplan-Meier analysis. A time-dependent receiver operating characteristic (ROC) curve analysis was performed using the “survivalROC” R package to assess the predictive accuracy of the gene signatures.
Pseudo-time trajectory analysis
To investigate the relationship of the cell pseudo-time trajectories, we performed an analysis of single-cell RNA data using the Monocle R (23) package. To identify the highly variable genes, we applied the following filtering criteria: average expression ≥0.1 and empirical dispersion ≥1× dispersion_fit. Dimensionality reduction was performed using the DDR-tree method. Subsequently, we visualized the results using the “plot_pseudotime_heatmap” function, generating a heatmap.
Cell-cell communication and metabolism analysis
Cellchat (24) R package was used to investigate intercellular communication networks derived from single-cell RNA-sequencing data categorized by distinct cell clusters. SCmetabolism R package (25) was used to assess the activity of the cellular metabolic pathways.
Human GC samples and immunohistochemistry (IHC)
For the IHC analysis, human samples were obtained from the Minhang Hospital affiliated to Fudan University. The study was approved by the Medical Ethics Committee of Minhang Hospital affiliated to Fudan University (protocol code: 2019-38; date of approval: 24 July 2019). The study was conducted in accordance with the Declaration of Helsinki (as revised in 2013). Written informed consent was obtained from the patients.
The samples were incubated overnight at 4 ℃ with anti-CSTA antibody (1:200, Abcam, anti-CSTA antibody, Cat. No. ab128948; Cambridge, UK). The slides were then incubated with HRP-conjugated secondary antibodies for 1 h at room temperature. Subsequently, the slides were visualized using 3,3'-diaminobenzidine for 5 minutes and counterstained with hematoxylin to visualize the nucleus. Finally, images were captured using the Olympus BX51 light microscope (Olympus Corporation).
Cell culture
The GC cell line AGS were obtained from the American Type Culture Collection (ATCC), and the GC cell line SGC-7901 were obtained from the CRL-1740 Lncap. The AGS cell line was cultured in Dulbecco’s Modified Eagle Medium (DMEM), while the SGC7901 cell line was cultured in Roswell Park Memorial Institute (RPMI) 1640, along with 10% fetal bovine serum and 1% antibiotics. All the cells were incubated at 37 ℃ with 5% carbon dioxide.
Quantitative real-time-polymerase chain reaction (PCR)
The total RNA was extracted from the test samples using TRIzol® reagent (Invitrogen, USA), and its quality and concentration were assessed with a NanoDrop® 2000 spectrophotometer (Thermo Fisher Scientific, USA). Subsequently, mRNA was reverse-transcribed into cDNA using the PrimeScript™ RT reagent from Takara Bio (Japan). The RNA concentration utilized in the experiment was 1 µg. Following this, FastStart Universal SYBR Green PCR Master Mix Kit (Roche, catalog number 4913914001) was introduced to the sample for conducting RT qPCR to amplify and quantitatively determine the RNA concentration. GAPDH served as an internal control, and gene expression levels were normalized utilizing the 2-ΔΔCq method relative to GAPDH.
The following primers were used: BCL-2 primer: forward: 5'-AGTTCGGTGGGGTCATGTGTG-3', reverse: 5'-CCAGGTATGCACCCAGAGTG-3'; CASP3 primer: forward: 5'-GTAGACGCGTCCAAGTCTCACTGGCTGTCA-3', reverse: 5'-TCCAGTTTAAACGGAACTTCTGCGAGGACTTG-3'.
Western blot
Total protein of all samples was harvested using RIPA reagent (Beyotime Institute of Biotechnology), which contains 1 mM PMSF. After measuring the protein concentration using the BCA assay kit (Beyotime Institute of Biotechnology), 40–80% SDS-PAGE was used to separate the proteins in each lane. Protein was then transferred to PVDF membrane (MilliporeSigma). Next, the membrane was incubated overnight at 4 ℃ with primary antibodies against β-Catenin (CTNNB1; #8480, 1:2,000, Cell Signaling Technologies, Inc., BSN, USA), BCL-2 Apoptosis Regulator (BCL2; #15071, 1:1,000, Cell Signaling Technologies, Inc., BSN, USA), Caspase 3 (CASP3; #14420, 1:2,000, Cell Signaling Technologies, Inc.) and CSTA (Cat. No. ab128948, 1:1,000, Abcam). The membrane was washed three times with 0.05% Tween-20 (TBST), and then incubated with HRP coupled second antibody for 1 hour. Finally, an enhanced chemiluminescence detection system (Bio Rad Laboratories, Inc., Hercules, CA, USA) was used to detect protein bands.
Cell Counting Kit 8 (CCK-8) assays
GC cell viability was detected by CCK-8 assays. Briefly, the groups of Control and CSTA-overexpression (CSTA-OE) GC cells were seeded in 96-well plates. After 1, 2, 3, 4 and 5 days, we added 20 µL of CCK-8 buffer to each well at 37 ℃ for 2 hours. Next, we measured the optical density (OD) value at 450 nm on a microplate reader.
Transwell assays
The transwell experiment was divided into the transwell migration experiment and transwell invasion experiment. We seeded 1×105 cells suspended in 100 µL of serum-free DMEM into the upper chamber. The lower chamber contained 600 µL of DMEM supplemented with 30% fetal bovine serum. After 8 hours of incubation, the cells were fixed with 4% paraformaldehyde for 30 minutes and stained with crystal violet in water (0.5%). The cells were then viewed under a microscope, and images were taken using the microscope.
Cell apoptosis
Apoptosis in GC cells was assessed using the Annexin-V-APC kit following the manufacturer’s protocol. Logarithmic phase cells were plated in 6-well dishes and harvested by trypsinization without EDTA. The collected cells were then suspended in 150 µL of binding buffer, followed by addition of 5 µL of Annexin V staining solution and 10 µL of PI staining solution separately. Subsequently, the cells were pipetted and incubated at room temperature for 15–20 minutes before adding 400 µL of binding buffer to each sample, thoroughly mixed to achieve a final volume of 500 µL for flow cytometry analysis.
Statistical analysis
The data were analyzed using GraphPad Prism 8.0 (GraphPad, Inc., La Jolla, CA, USA) and R (version 4.1.3; R Foundation for Statistical Computing), and the results were expressed as means ± standard deviations (SD). Statistical analyses comprised Student’s t-test, Wilcoxon rank-sum test, Kruskal-Wallis test, and one-way analysis of variance (ANOVA) to evaluate inter-group differences. Survival curves were constructed employing the Kaplan-Meier method alongside Log-rank testing. The threshold of P<0.05 was employed to denote statistically significant differences between cohorts.
Results
Construction of a prognostic model for GC RFS through gene screening
Following the analysis of TCGA-STAD data, we identified 2,532 genes with upregulated expression and 419 genes with downregulated expression. Subsequent examination of gene expression profiles in GSE54129 revealed 1,124 upregulated genes and 1,239 downregulated genes (Figure 1A-1C). The Venn diagram demonstrated an intersection between TCGA-STAD and GSE54129, resulting in a total of 23 GC RFS-related genes (Figure 1D,1E). Functional enrichment analysis indicated that these differentially expressed immune regulatory factors primarily participate in pathways associated with the extracellular matrix (ECM), adhesion, and other relevant processes in cancer, suggesting that these pathways could be pivotal factors impacting the prognosis and survival of patients with GC (Figure 1F,1G).
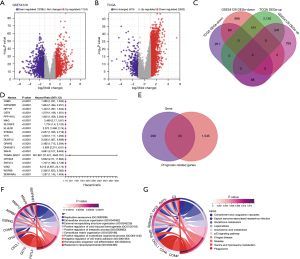
The prognostic model of RFS for GC
Using TCGA-STAD data set, a prognostic model for the RFS of GC was constructed. This model incorporated 23 RFS-associated genes and employed a LASSO regression and multivariate Cox analysis. The risk scores were calculated using the following coefficients, including 11 genes (Figure 2A,2B):
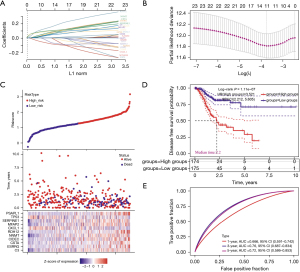
(0.0402) × C3 + (0.0692) × ESRRG + (0.0544) × CSTA + (0.0623) × CIDEC + (0.1013) × NNMT + (0.1491) × RDH12 + (−0.0805) × CXCL1 + (0.0493) × MFAP2 + (0.0792) × SERPINE1 + (−0.0592) × TP53 + (0.0934) × PSAPL1.
Patients with higher risk scores exhibited a poorer prognosis (Figure 2C,2D). The time-dependent area under the curve analysis showed the potential of the 11 GC prognosis-related (GPR) genes prognostic model in predicting RFS among GC patients in TCGA data set (Figure 2E). We further winnowed the 23 RFS-related genes down to 11 that were most pertinent to GC prognosis, thereby expediting subsequent analysis and identification of individually clinically applicable genes.
Functional assessment of the prognostic-related genes at the GC single-cell level
In the GSE183904 data set, which comprised 15 GC and 7 adjacent tissue samples, a total of 54,578 cells were clustered, subsequently, these cells could be clustered into 20 subclusters, and clustering and pathway analysis were shown based on the expression of marker genes (Figure S1A,S1B) (21,26,27). The results revealed that subclusters in the GC single-cell atlas were defined as 13 distinct cell subtypes (Figure 3A). Through the utilization of five distinct methodologies, we assessed the enrichment scores of the 11 GPR genes across all cellular subpopulations and derived a comprehensive score. The results revealed that GC prognostic-related genes were significantly enriched in the stromal and fibroblast subtypes, further analysis indicates that the proportion of stromal cells in the tumor microenvironment (TME) is significantly higher than in adjacent cancer samples (Figure 3B,3C). Further, the gene set variation analysis (GSVA) pathways enrichment highlighted the role of stromal cell types focusing on collagen formation and ECM in the GC TME, which supported our previous findings from the KEGG and GO enrichment analyses (Figure S1C).
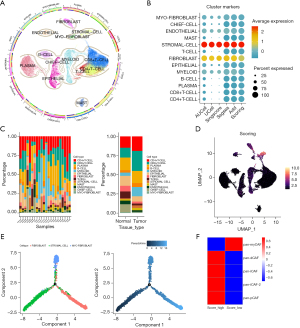
The single-cell atlas analysis of GC of functional enrichment and marker gene expression suggests that the stromal cells of interest exhibit similarities to conventional fibroblasts (Figure S2A). This implies that these stromal cells may represent a subtype of fibroblasts undergoing early-stage differentiation. The enrichment score of GPR genes in stromal cells surpasses that observed in other GC-TME cell subtypes (Figure 3D, Figure S2B). Additionally, validation through pseudo-time analysis confirmed that these stromal cells were indeed in an early stage of differentiation. Our previous analysis also indicated that this early fibroblast subtype was predominantly clustered in cancer tissues, with only a small proportion found in adjacent tissues, indicating active expansion within GC. The temporal expression patterns of the 11 GPR genes further suggested their involvement in regulating differentiation and maturation functions at different stages within these three fibroblast subpopulations (Figure 3E, Figure S2C). Cancer-associated fibroblasts (CAFs) are crucial components of the TME, and based on specific molecular characteristics (28,29), they can be classified into the following subtypes: myofibroblastic CAFs (pan-myCAFs), proliferative fibroblastic CAFs (pan-dCAFs), inflammatory fibroblastic CAFs (pan-iCAFs), and normal fibroblastic CAFs (pan-pCAFs). We divided the stromal cell subgroups into high and low score groups, and found that, apart from the pan-myCAFs subtype, the other subtypes were highly associated with stromal cells (Figure 3F). Using “scMetabolism” to assess the activation levels of KEGG metabolic pathways of two stromal cells subgroups, the results showed that cells in the score_high subgroup had higher metabolic levels (Figure S2D). The cell-cell communication reveals that the score_high subgroup cells exhibited enhanced communication with various subgroups in the intratumoral environment, particularly endothelial cells (ECs). They showed enhanced communication with various T cells through GZMA-F2R ligand-receptor interactions (Figure 4A,4B). The enhanced crosstalk with ECs and T cells may have a potential impact on the TME, thereby influencing patient RFS. Furthermore, by analyzing the DEGs between the groups and conducting a GO pathway enrichment analysis, we identified 15 upregulated genes enriched in the peptide metabolism, hypoxia, and ECM formation pathways in the high score subgroup compared to the low score subgroup. Additionally, 69 downregulated genes were enriched in the RNA splicing pathways (Figure S2E,S2F). Additionally, the pseudo-time analysis revealed that stromal cells within the high score subgroup tended to demonstrate a more differentiated state (Figure 4C).
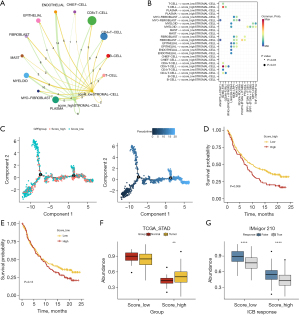
To further investigate the effect of the top 30 gene signatures of stromal cells on patient survival after treatment, we used the IMvigor 210 immunotherapy and TCGA-STAD cohort. The results revealed that in the IMvigor 210 cohort, the top 30 gene signatures of the score_high stromal cell subset had a significant effect on patient survival after treatment, with the low-expression group showing higher survival rates (Figure 4D,4E). In TCGA-STAD cohort, the top 30 gene signatures of the score_high stromal cells were significantly more upregulated in the tumor tissues (Figure 4F). In IMvigor 210 cohort, the top 30 gene signatures of the score_high stromal cells were significantly more downregulated in treatment response group (Figure 4G).
In summary, our assessment of the 11 GPR genes revealed their predominant enrichment in an early fibroblastic stromal cell population. The high-scoring stromal cells exhibited increased activity in various pathways related to metabolism, differentiation, and communication. Additionally, the upregulated genes in the score_high stromal cells were enriched in pathways associated with peptide metabolism and hypoxia, suggesting a potential link to hypoxia-induced processes. Moreover, the signature of these score_high stromal cells had an impact on patient survival after treatment within the bulk cohort. This single-cell analysis provides a theoretical basis for the clinical application of the prognostic model developed earlier in this study.
CSTA inhibits the tumor malignant phenotype of GC cells
The role and mechanism of CSTA in GC remained unclear among the 11 genes. By further sub-grouping the epithelial cell subpopulation into tumor cells and normal cells (Figure 5A,5B). In comparison to normal epithelial cells, the expression of CSTA was elevated in cancerous epithelium (Figure 5C,5D). The IHC results revealed that CSTA was lowly expressed in cancer tissues (Figure 5E). The Western blot showed the expression levels of CSTA in various GC cell lines. The results demonstrated that CSTA was more highly expressed in the normal tissue groups and multiple GC cell lines, particularly in AGS, SGC-7901, and HGC-27 (Figure 5F). The TCGA-STAD data are consistent with our above research results (Figure 5G).
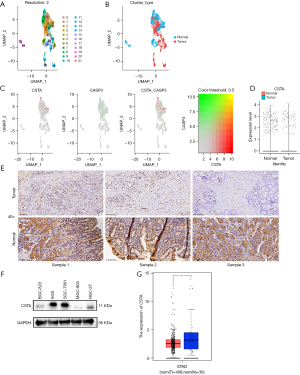
Further, we overexpressed CSTA in the AGS and SGC-7901 cells, designated as CSTA overexpression (CSTA-OE) (Figure 6A,6B). Using CCK-8, transwell assays, colony formation and scratch assays revealed that the overexpression of CSTA significantly suppressed the migration and invasion of the GC cells (Figure 6C-6K).
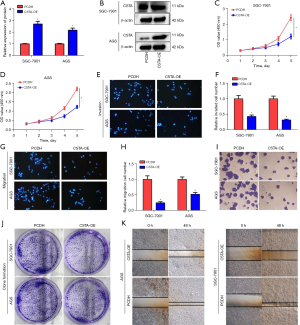
CSTA facilitates GC cell apoptosis by activating the caspase-dependent pathway
Flow cytometry was employed to assess the effect of CSTA-OE on apoptosis in the GC cells. The results revealed a significant alteration in the apoptotic ratio of the AGS and SGC-7901 cells with CSTA-OE compared to the control group (Figure 7A,7B). Further, we investigated the effects of CSTA-OE on pro-apoptotic and anti-apoptotic proteins. Caspase 3, the ultimate executor of the caspase-dependent apoptotic pathway, and apoptosis regulator BCL-2, a classic protein in the anti-apoptotic pathway, were selected as the targets of our study. The results demonstrated that Caspase 3 expression was upregulated and BCL-2 expression was downregulated in response to CSTA-OE; similar results were observed at the messenger RNA level (Figure 7C-7E).
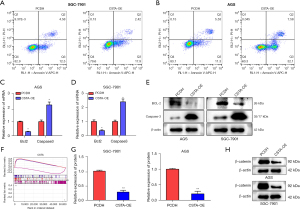
Caspase 3, a key protein and the final executor of the caspase-dependent apoptotic pathway, exerts pro-apoptotic effects by cleaving to downstream cell survival-related proteins. The GSEA pathway analysis revealed a negative correlation between CSTA expression and the Wnt pathway. Therefore, we examined the expression levels of β-catenin, a core protein in the Wnt pathway, in both groups. Interestingly, we observed a decrease in β-catenin expression in the CSTA-OE group, which suggests that CSTA may induce the degradation of β-catenin through the caspase pathway, thereby exerting its inhibitory effects on the malignant phenotype of GC (Figure 7F-7H).
The overexpression of CSTA enhances the apoptotic effect of cisplatin
Combination therapy involving cisplatin and other agents remains a frontline approach in GC treatment, as combining multiple drugs can overcome resistance issues and reduce toxic side effects.
The experimental groups were divided into four categories: the PCDH group (NC), the CSTA-OE group, the PCDH group treated with 5 µM of cisplatin (cis) for 48 hours, and the CSTA-OE group treated with 5 µM of cisplatin for 48 hours (cis + CSTA-OE). The levels of proliferation, migration, invasion, and colony formation among the four groups were assessed using CCK-8, transwell, and colony formation assays. The results demonstrated that CSTA-OE enhanced the tumor-killing ability of cisplatin in the GC cells (Figure 8A-8D). The results of flow cytometry showed that the addition of cis + CSTA-OE increased the level of cell apoptosis in the group remarkably (Figure 8E).
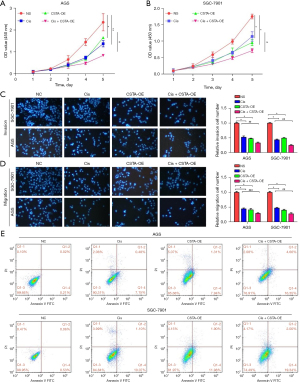
CSTA-OE also increased the sensitivity of the GC cells to cisplatin (Figure S3A). The expression levels of the pro-apoptotic protein Caspase 3 and the anti-apoptotic protein BCL-2 were measured among the four groups. The results showed that CSTA-OE combined with cisplatin significantly increased the expression of Caspase 3 and suppressed the expression of BCL-2 (Figure S3B).
Discussion
GC is one of the most prevalent cancers worldwide, with a high incidence rate in many countries, particularly in Asia (30). Chemotherapy remains the frontline treatment for locally unresectable and metastatic stomach cancer. However, this treatment option has inherent drawbacks, such as a lack of specificity, severe side effects, and tumor resistance (3). Thus, we sought to identify novel potential targets in stomach cancer using bioinformatics approaches.
Firstly, using a bioinformatics analysis, the construction of a prognostic model to predict RFS in patients with GC was undertaken, furthermore, we identified 11 pivotal GC RFS-associated genes (i.e., C3, ESRRG, CSTA, CIDEC, NNMT, RDH12, CXCL1, MFAP2, SERPINE1, TP53, and PSAPL1) and explored their potential functions using the GC single-cell cohort. The findings indicate that an early fibroblast-like stromal cell population may serve as a crucial determinant of GC RFS (31). This population predominantly resides in tumor tissue and possesses the potential to differentiate into mature fibroblasts and myofibroblasts (32).
Numerous studies have unequivocally demonstrated the pivotal role of immune evasion in the initiation and progression of cancer, particularly in GC where CAFs impede immune cell infiltration through paracrine or direct mechanisms, thereby diminishing the immune system’s capacity to recognize and eliminate cancer cells, consequently fostering the onset and advancement of GC (33). CAFs also secrete a myriad of cytokines to induce T cell infiltration, proliferation, differentiation, and apoptosis, thus detrimentally affecting normal T cell immune function (34). Furthermore, CAFs are proficient at remodeling the ECM, establishing a physical barrier that obstructs T lymphocyte infiltration and contributes to tumor immune evasion (35). Additionally, CAF secreted miR-522 suppresses ferroptosis and promotes acquired chemo-resistance in GC (36). The hypoxic conditions within the TME can also stimulate GC CAFs to release vascular endothelial growth factor (VEGF) in order to enhance tumor angiogenesis (37,38).
The expression scores of RFS-associated genes in stromal cells are correlated with their differentiation statuses and the distinct expression patterns of CAF marker genes. Stromal cells with high scores are likely to communicate with various cells, including ECs and T cells, via multiple receptor-ligand pathways, thereby influencing tumor progression. The gene signatures of these cells could serve as prognostic indicators for patient treatment outcomes.
Consequently, our prognostic model and gene set, based on RFS-associated genes, could be employed to assess the activation level of early fibroblasts in the microenvironment of GC patients, offering novel insights into the exploring the mechanism of GC occurrence and progression. The primary obstacle in therapeutic interventions for GC resides in the formidable resistance to traditional chemotherapeutic agents (e.g., cisplatin) and suboptimal response rates to innovative drug modalities (e.g., targeted therapy and immunotherapy) (2). Therefore, our prognostic model and the 11 identified genes hold substantial promise, potentially serving as therapeutic targets that could impede disease progression and augment patient survival, this study provides new evidence of the clinical utility of prognostic genes, and establishes a theoretical foundation and scientific basis for the study and treatment of CSTA in stomach cancer.
CSTA (39), also known as cystatin or acidic cysteine proteinase inhibitor, belongs to the type I or stefin subgroup of known cysteine protease inhibitors. It is an 11-kDa single-chain intracellular cysteine proteinase inhibitor that can inhibit papain, cathepsins B/H/L, and cysteine proteinase activities (40). This study was the first to demonstrate the role of CSTA in stomach cancer. Specifically, this study showed that CSTA is lowly expressed in cancer tissue samples, and exerts anticancer effects by promoting the expression of apoptotic proteins and enhancing the sensitivity of stomach cancer cells to cisplatin.
Conclusions
In conclusion, through the development of a prognostic model for RFS in GC patients, we have successfully pinpointed 11 genes with potential clinical significance. These genes, predominantly enriched in early-stage stromal cells, can indirectly anticipate the abundance of early-stage fibroblasts in cancer tissue and consequently forecast the prognosis of GC patients. Despite the study’s demonstration of CSTA’s antitumor effect through the enhancement of the Caspase-dependent apoptotic pathway in GC cells, further exploration is needed to elucidate the mechanisms of CSTA-mediated tumor inhibition, its specific role in the stomach cancer microenvironment, particularly in macrophages, and its value as a novel target. It should be noted that our exploratory study is limited by the limited profundity of scRNA-sequencing data and the small sample size. The results also need additional validation in more patients.
Acknowledgments
Funding: This work was supported by
Footnote
Reporting Checklist: The authors have completed the MDAR reporting checklist. Available at https://jgo.amegroups.com/article/view/10.21037/jgo-23-941/rc
Data Sharing Statement: Available at https://jgo.amegroups.com/article/view/10.21037/jgo-23-941/dss
Peer Review File: Available at https://jgo.amegroups.com/article/view/10.21037/jgo-23-941/prf
Conflicts of Interest: All authors have completed the ICMJE uniform disclosure form (available at https://jgo.amegroups.com/article/view/10.21037/jgo-23-941/coif). All authors report that this work was supported by National Science Foundation of China (No. 8217100675) subaward. The authors have no other conflicts of interest to declare.
Ethical Statement: The authors are accountable for all aspects of the work in ensuring that questions related to the accuracy or integrity of any part of the work are appropriately investigated and resolved. The study was conducted in accordance with the Declaration of Helsinki and was approved by the Medical Ethics Committee of Minhang Hospital affiliated to Fudan University (protocol code: 2019-38; date of approval: 24 July 2019). Written informed consent was obtained from the patients.
Open Access Statement: This is an Open Access article distributed in accordance with the Creative Commons Attribution-NonCommercial-NoDerivs 4.0 International License (CC BY-NC-ND 4.0), which permits the non-commercial replication and distribution of the article with the strict proviso that no changes or edits are made and the original work is properly cited (including links to both the formal publication through the relevant DOI and the license). See: https://creativecommons.org/licenses/by-nc-nd/4.0/.
References
- Decourtye-Espiard L, Guilford P. Hereditary Diffuse Gastric Cancer. Gastroenterology 2023;164:719-35. [Crossref] [PubMed]
- Guan WL, He Y, Xu RH. Gastric cancer treatment: recent progress and future perspectives. J Hematol Oncol 2023;16:57. [Crossref] [PubMed]
- Choi IJ, Kim CG, Lee JY, et al. Family History of Gastric Cancer and Helicobacter pylori Treatment. N Engl J Med 2020;382:427-36. [Crossref] [PubMed]
- Arnold M, Park JY, Camargo MC, et al. Is gastric cancer becoming a rare disease? A global assessment of predicted incidence trends to 2035. Gut 2020;69:823-9. [Crossref] [PubMed]
- Lordick F. Chemotherapy for resectable microsatellite instability-high gastric cancer? Lancet Oncol 2020;21:203. [Crossref] [PubMed]
- Thrift AP, Wenker TN, El-Serag HB. Global burden of gastric cancer: epidemiological trends, risk factors, screening and prevention. Nat Rev Clin Oncol 2023;20:338-49. [Crossref] [PubMed]
- Murad AM, Santiago FF, Petroianu A, et al. Modified therapy with 5-fluorouracil, doxorubicin, and methotrexate in advanced gastric cancer. Cancer 1993;72:37-41. [Crossref] [PubMed]
- Ajani JA, D'Amico TA, Almhanna K, et al. Gastric Cancer, Version 3.2016, NCCN Clinical Practice Guidelines in Oncology. J Natl Compr Canc Netw 2016;14:1286-312. [Crossref] [PubMed]
- Van Cutsem E, Bang YJ, Feng-Yi F, et al. HER2 screening data from ToGA: targeting HER2 in gastric and gastroesophageal junction cancer. Gastric Cancer 2015;18:476-84. [Crossref] [PubMed]
- Fuchs CS, Doi T, Jang RW, et al. Safety and Efficacy of Pembrolizumab Monotherapy in Patients With Previously Treated Advanced Gastric and Gastroesophageal Junction Cancer: Phase 2 Clinical KEYNOTE-059 Trial. JAMA Oncol 2018;4:e180013. [Crossref] [PubMed]
- Tabernero J, Van Cutsem E, Bang YJ, et al. Pembrolizumab with or without chemotherapy versus chemotherapy for advanced gastric or gastroesophageal junction (G/GEJ) adenocarcinoma: The phase III KEYNOTE-062 study. American Society of Clinical Oncology 2019. Available online:
10.1200/JCO.2019.37.18_suppl.LBA4007 10.1200/JCO.2019.37.18_suppl.LBA4007 - Jun JK, Choi KS, Lee HY, et al. Effectiveness of the Korean National Cancer Screening Program in Reducing Gastric Cancer Mortality. Gastroenterology 2017;152:1319-1328.e7. [Crossref] [PubMed]
- Japanese gastric cancer treatment guidelines 2014 (ver. 4). Gastric Cancer 2017;20:1-19. [Crossref] [PubMed]
- Yuan L, Hu C, Yu P, et al. High HDAC5 expression correlates with a poor prognosis and the tumor immune microenvironment in gastric cancer. Ann Transl Med 2022;10:990. [Crossref] [PubMed]
- Takahashi H, Asano K, Kinouchi M, et al. Structure and transcriptional regulation of the human cystatin A gene. The 12-O-tetradecanoylphorbol-13-acetate (TPA) responsive element-2 site (-272 to -278) on cystatin A gene is critical for TPA-dependent regulation. J Biol Chem 1998;273:17375-80. [Crossref] [PubMed]
- Gupta A, Nitoiu D, Brennan-Crispi D, et al. Cell cycle- and cancer-associated gene networks activated by Dsg2: evidence of cystatin A deregulation and a potential role in cell-cell adhesion. PLoS One 2015;10:e0120091. [Crossref] [PubMed]
- John Mary DJS, Manjegowda MC, Kumar A, et al. The role of cystatin A in breast cancer and its functional link with ERα. J Genet Genomics 2017;44:593-7. [Crossref] [PubMed]
- Shiba D, Terayama M, Yamada K, et al. Clinicopathological significance of cystatin A expression in progression of esophageal squamous cell carcinoma. Medicine (Baltimore) 2018;97:e0357. [Crossref] [PubMed]
- Duivenvoorden HM, Rautela J, Edgington-Mitchell LE, et al. Myoepithelial cell-specific expression of stefin A as a suppressor of early breast cancer invasion. J Pathol 2017;243:496-509. [Crossref] [PubMed]
- Liu ZJ, Zheng LS, Li CZ, et al. Correlated with better prognosis, CSTA inhibits metastasis of nasopharyngeal carcinoma cells via suppressing AKT signaling through promoting METTL3 degradation. Biochim Biophys Acta Mol Basis Dis 2023;1869:166696. [Crossref] [PubMed]
- Kumar V, Ramnarayanan K, Sundar R, et al. Single-Cell Atlas of Lineage States, Tumor Microenvironment, and Subtype-Specific Expression Programs in Gastric Cancer. Cancer Discov 2022;12:670-91. [Crossref] [PubMed]
- Necchi A, Joseph RW, Loriot Y, et al. Atezolizumab in platinum-treated locally advanced or metastatic urothelial carcinoma: post-progression outcomes from the phase II IMvigor210 study. Ann Oncol 2017;28:3044-50. [Crossref] [PubMed]
- Qiu X, Mao Q, Tang Y, et al. Reversed graph embedding resolves complex single-cell trajectories. Nat Methods 2017;14:979-82. [Crossref] [PubMed]
- Jin S, Guerrero-Juarez CF, Zhang L, et al. Inference and analysis of cell-cell communication using CellChat. Nat Commun 2021;12:1088. [Crossref] [PubMed]
- Wu Y, Yang S, Ma J, et al. Spatiotemporal Immune Landscape of Colorectal Cancer Liver Metastasis at Single-Cell Level. Cancer Discov 2022;12:134-53. [Crossref] [PubMed]
- Luecken MD, Theis FJ. Current best practices in single-cell RNA-seq analysis: a tutorial. Mol Syst Biol 2019;15:e8746. [Crossref] [PubMed]
- Jiang D, Turner B, Song J, et al. Comprehensive Analysis of the Unfolded Protein Response in Breast Cancer Subtypes. JCO Precis Oncol 2017;2017:PO.16.00073.
- Galbo PM Jr, Zang X, Zheng D. Molecular Features of Cancer-associated Fibroblast Subtypes and their Implication on Cancer Pathogenesis, Prognosis, and Immunotherapy Resistance. Clin Cancer Res 2021;27:2636-47. [Crossref] [PubMed]
- Zhu GQ, Tang Z, Huang R, et al. CD36(+) cancer-associated fibroblasts provide immunosuppressive microenvironment for hepatocellular carcinoma via secretion of macrophage migration inhibitory factor. Cell Discov 2023;9:25. [Crossref] [PubMed]
- Smyth EC, Verheij M, Allum W, et al. Gastric cancer: ESMO Clinical Practice Guidelines for diagnosis, treatment and follow-up. Ann Oncol 2016;27:v38-49. [Crossref] [PubMed]
- Wang R, Song S, Qin J, et al. Evolution of immune and stromal cell states and ecotypes during gastric adenocarcinoma progression. Cancer Cell 2023;41:1407-1426.e9. [Crossref] [PubMed]
- Kim H, Hwang Y, Sung H, et al. Effectiveness of Gastric Cancer Screening on Gastric Cancer Incidence and Mortality in a Community-Based Prospective Cohort. Cancer Res Treat 2018;50:582-9. [Crossref] [PubMed]
- Yan Y, Wang LF, Wang RF. Role of cancer-associated fibroblasts in invasion and metastasis of gastric cancer. World J Gastroenterol 2015;21:9717-26. [Crossref] [PubMed]
- Ma M, Sun J, Liu Z, et al. The Immune Microenvironment in Gastric Cancer: Prognostic Prediction. Front Oncol 2022;12:836389. [Crossref] [PubMed]
- Chen X, Song E. Turning foes to friends: targeting cancer-associated fibroblasts. Nat Rev Drug Discov 2019;18:99-115. [Crossref] [PubMed]
- Zhang H, Deng T, Liu R, et al. CAF secreted miR-522 suppresses ferroptosis and promotes acquired chemo-resistance in gastric cancer. Mol Cancer 2020;19:43. [Crossref] [PubMed]
- Shah MA, Starodub A, Sharma S, et al. Andecaliximab/GS-5745 Alone and Combined with mFOLFOX6 in Advanced Gastric and Gastroesophageal Junction Adenocarcinoma: Results from a Phase I Study. Clin Cancer Res 2018;24:3829-37. [Crossref] [PubMed]
- Kinoshita H, Yashiro M, Fukuoka T, et al. Diffuse-type gastric cancer cells switch their driver pathways from FGFR2 signaling to SDF1/CXCR4 axis in hypoxic tumor microenvironments. Carcinogenesis 2015;36:1511-20. [Crossref] [PubMed]
- Blaydon DC, Nitoiu D, Eckl KM, et al. Mutations in CSTA, encoding Cystatin A, underlie exfoliative ichthyosis and reveal a role for this protease inhibitor in cell-cell adhesion. Am J Hum Genet 2011;89:564-71. [Crossref] [PubMed]
- Zhang X, Zhuge J, Liu J, et al. Prognostic signatures of sphingolipids: Understanding the immune landscape and predictive role in immunotherapy response and outcomes of hepatocellular carcinoma. Front Immunol 2023;14:1153423. [Crossref] [PubMed]