MMP11 as a prognostic biomarker correlated with immune infiltrates in pancreatic adenocarcinoma
Highlight box
Key findings
• The expression of matrix metalloproteinase 11 (MMP11) is closely related to the prognosis of pancreatic cancer patients, and it can regulate the tumor immune microenvironment.
What is known and what is new?
• Current research indicates that MMP11 is highly expressed in various cancers, including pancreatic cancer.
• We study the relationship between high expression, immune function, and prognostic value of MMP11 in pancreatic cancer.
What is the implication, and what should change now?
• MMP11 may be a new target for the treatment of pancreatic adenocarcinoma, but further research is still needed through in vivo and in vitro experiments.
Introduction
Pancreatic adenocarcinoma (PAAD) is known for its strong metastatic capability, high recurrence rate, and poor prognosis. Radical resection is the main treatment modality for PAAD; however, at the time of diagnosis, most patients are in the advanced stage and have distant organ metastasis, and within 2 years of surgery, 80% of patients have local recurrence or distant metastasis (1-3). Despite rapid advancements in chemoradiotherapy, immunotherapy, and targeted therapies, their effectiveness is still not satisfactory (4), highlighting the need to explore PAAD’s mechanisms and to identify new therapeutic targets.
Matrix metalloproteinase 11 (MMP11), also known as matrix hemolysin-3, is a key member of the MMP family and belongs to the stromelysin subfamily of MMPs, a subpopulation initially discovered in the stromal cells of breast cancer (5). The abnormal expression of MMP11 plays a role in the occurrence and development of several kinds of cancers, including lung, laryngeal, breast, and colorectal cancer (6-9). However, MMP11 expression and its role in PAAD need to be further explored.
This study investigates MMP11 expression and its association with the prognosis of PAAD patients using various databases. In addition, the Tumor Immune Estimation Resource (TIMER) and Tumor-Immune System Interactions and Drug Bank (TISIDB) databases were used to investigate the relationship between MMP11 and immune-related cells in different tumor microenvironments (TMEs). This study revealed the critical involvement of MMP11 in PAAD, as well as the possible connection and mechanism by which MMP11 may regulate tumor-infiltrating immune cells. We present this article in accordance with the REMARK reporting checklist (available at https://jgo.amegroups.com/article/view/10.21037/jgo-24-425/rc).
Methods
Acquisition of patients and data
All messenger RNA (mRNA) information and clinical data of patients were obtained from The Cancer Genome Atlas (TCGA) (https://www.cancer.gov/ccg/research/genome-sequencing/tcga) and Genotype-Tissue Expression (GTEx) (https://commonfund.nih.gov/GTEx) databases. The study was conducted in accordance with the Declaration of Helsinki (as revised in 2013).
MMP11 gene expression analysis
We analyzed MMP11 expression levels across normal and tumor tissues in a comprehensive data set that integrates data from TCGA and GTEx databases. For standardized and bioinformatics analyses of RNA-sequencing (RNA-Seq) data from these sources, we employed the Gene Expression Profiling Interactive Analysis (GEPIA)—an online interactive database specifically designed for this purpose (10) (http://gepia.cancer-pku.cn/). Additionally, GEPIA was utilized to investigate variations in MMP11 gene expression across different cancer stages.
Clinical association and prognostic analysis
We examined the association between MMP11 gene expression and survival rates across 21 tumor types using the Kaplan-Meier plotter database (http://kmplot.com/analysis/). Furthermore, GEPIA was used to assess the prognostic significance of MMP11 in 33 different cancer types, encompassing analyses of overall survival (OS) and disease-free survival (DFS). To enhance diagnostic accuracy, we generated receiver operating characteristic (ROC) curves and time-dependent curves of diagnosis, and nomogram models using the pROC, timeROC, and survival packages.
Functional enrichment analysis
The Limma package was used to investigate the differential expression of mRNAs. False-positive results were corrected using the adjusted P value. A P value <0.05 and a |log2 (fold change)| >1 were established as the cut-off points for screening differentially expressed genes (DEGs). Multi-omics data for 10 clinical proteomics tumor analysis collaboration cancer cohorts and all 32 TCGA cancer types are available on the Linkedomics site (11) (http://www.linkedomics.org/login.php). To derive biological insights from the correlation results, the Linkinterpreter module was used to perform an enrichment analysis according to the Gene Ontology (GO), biological pathway, network module, and other functional categories. To better understand the carcinogenesis of MMP11, we accessed protein-protein interaction (PPI) network information for MMP11 using the Search Tool for the Retrieval of Interacting Genes/Proteins (STRING) database (12). A Reactome pathway analysis and GO term enrichment analysis were conducted to examine the functions of MMP11 using the open access WebGestalt tool (http://www.webgestalt.org). An adjusted P<0.05 in the enrichment results indicated a significant pathway.
Mutation and immune infiltration analysis
The cBioPortal was used to evaluate the mutation frequency of MMP11 in PAAD (http://www.cbioportal.org/). The Catalogue of Somatic Mutations in Cancer (COSMIC) database was used to further identify the mutation types of MMP11 in PAAD (http://cancer.sanger.ac.uk) (13).
TIMER is a database that examines immune infiltration in different cancer types in a methodical manner (https://cistrome.shinyapps.io/timer/). In this study, we examined the connection between MMP11 expression and immune infiltration in PAAD using TIMER. The TISIDB (http://cis.hku.hk/TISIDB) database connects multiple data sources that provide information about tumors and the immune system. We used it to examine whether MMP11 is involved in regulating immune infiltration in PAAD and to examine the relationship between MMP11 expression and tumor-infiltrating lymphocytes (TILs), immunoinhibitors, immunostimulators, and major histocompatibility complex (MHC) molecules.
Statistical analysis
Statistical analyses were conducted by R (V 3.6.3). The Wilcoxon rank-sum test and t-test were used to compare numerical differences between MMP11 high- and low-expression groups. The effect of MMP11 expression on survival was compared with other clinical characteristics (e.g., age, gender, grading, staging) using univariate and multifactorial Cox analysis. Survival curves were generated by Kaplan-Meier plots. P<0.05 was considered to be statistically significant.
Results
MMP11 was more highly expressed in cancerous than non-cancerous tissues
We first examined MMP11 expression in 33 different human cancer types to investigate its potential roles. As Figure 1A,1B shows, compared to the corresponding normal tissues, MMP11 mRNA levels were significantly upregulated in 28 cancer types, including adrenocortical carcinoma, bladder urothelial carcinoma, breast invasive carcinoma, cervical squamous cell carcinoma, cholangio carcinoma, colon adenocarcinoma, lymphoid neoplasm diffuse large B-cell lymphoma, esophageal carcinoma, glioblastoma multiforme (GBM), head and neck squamous cell carcinoma (HNSC), kidney renal clear cell carcinoma (KIRC), kidney renal papillary cell carcinoma, acute myeloid leukemia, brain lower grade glioma (LGG), liver hepatocellular carcinoma (LIHC), lung adenocarcinoma, lung squamous cell carcinoma, ovarian serous cystadenocarcinoma, PAAD, prostate adenocarcinoma (PRAD) rectum adenocarcinoma, skin cutaneous melanoma, stomach adenocarcinoma, testicular germ cell tumor, thyroid carcinoma, thymoma (THYM), uterine corpus endometrial carcinoma (UCEC), and uterine carcinosarcoma. However, no significant difference was observed in kidney chromophobe (KICH).
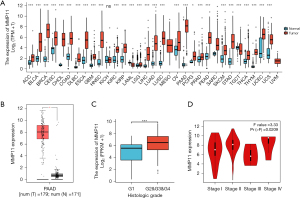
We also evaluated the expression of MMP11 in patients with different G stages of PAAD, and the expression of MMP11 was higher in the G2, G3, and G4 stages than the G1 stage (Figure 1C, P<0.001). In addition, we discovered that MMP11 and the pathological stage of PAAD patients were correlated (Figure 1D, P=0.02).
Prognostic significance of MMP11 expression in human cancers
Following this, we used the Kaplan-Meier plotter database to assess the prognostic implications of MMP11 expression in various human malignancies. Elevated MMP11 expression was correlated with a poorer prognosis in bladder carcinoma [OS: hazard ratio (HR) =1.5; 95% confidence interval (CI): 1.06 to 2.12; P=0.02], KIRC (OS: HR =1.43; 95% CI: 1.04 to 1.95; P=0.03), LIHC (OS: HR =1.61; 95% CI: 1.07 to 2.42; P=0.02), PAAD [OS: HR =1.91, 95% CI: 1.26 to 2.9, P=0.002; recurrence-free survival (RFS): HR =3.55, 95% CI: 1.51 to 8.35; P=0.002], and sarcoma (OS: HR =1.73, 95% CI: 1.15 to 2.6, P=0.007; RFS: HR =1.84, 95% CI: 1.11 to 3.04, P=0.02). Moreover, lower MMP11 expression was linked to a worse prognosis in THYM (OS: HR =0.1; 95% CI: 0.02 to 0.49; P<0.001) and UCEC (OS: HR =0.52, 95% CI: 0.35 to 0.79, P=0.002; RFS: HR =0.44, 95% CI: 0.26 to 0.73, P=0.001) (Figure 2A-2J). However, MMP11 expression was not associated with RFS in bladder carcinoma, KIRC, LIHC, and THYM.
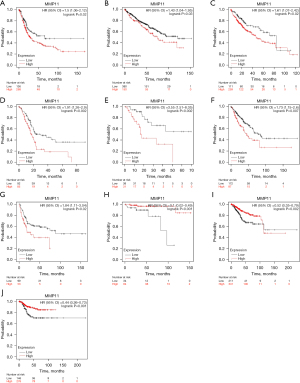
Additionally, we analyzed the prognostic potential of the level of MMP11 mRNA expression in various cancers using the TCGA RNA-Seq data from the GEPIA database. MMP11 expression was associated with poorer OS in GBM, KICH, LGG, mesothelioma (MESO), PAAD, and uveal melanoma (UVM), and poorer DFS in KICH, LGG, PRAD, and UVM. However, a higher expression of MMP11 was correlated with better DFS in HNSC and UCEC (Figure 3A-3L). Thus, MMP11 expression is important in the prognosis of a variety of human cancers; however, the link between MMP11 expression and prognosis may differ according to the type of cancer.
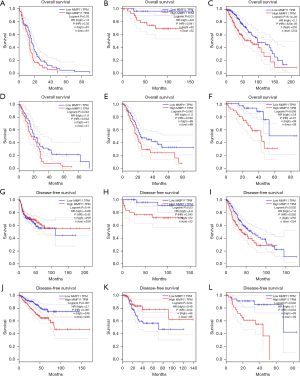
Value of MMP11 overexpression in diagnosing and predicting prognosis in PAAD
The analysis of the diagnostic ROC curve indicated that MMP11 expression could accurately differentiate between tumors and normal tissues [area under the curve (AUC) =0.980] (Figure 4A). In addition, we conducted ROC curve analyses for pathological stage, tumor (T) stage, node (N) stage, metastasis (M) stage and grade (G) stage in the subgroup of PAAD patients. The subgroup ROC curve analysis suggested that MMP11 overexpression might have diagnostic significance in PAAD patients with pathological stage I/(II + III + IV) (Figure 4B, AUC =0.634), T1/(T2 + T3 + T4) (Figure 4C, AUC =0.633), N0/N1 stage (Figure 4D, AUC =0.521), M0/M1 stage (Figure 4E, AUC =0.703), G1/(G2 + G3 + G4) stage (Figure 4F, AUC =0.710).
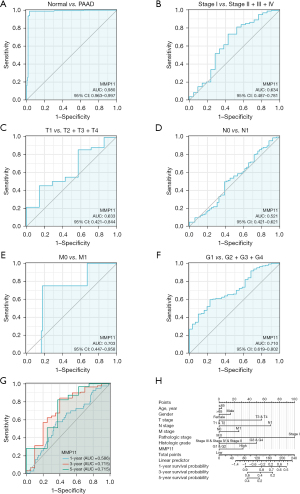
Further, the AUCs determined using the ROC curve assessment were used to predict 1-, 3-, and 5-year risk in PAAD patients (1-year AUC =0.586; 3-year AUC =0.715; 5-year AUC =0.715) (Figure 4G). By integrating the clinicopathologic factors (including age, gender, T stage, N stage, M stage, pathologic stage, and histologic grade) and the MMP11 level, a nomogram model was created that could be used to predict the probability of survival of patients over 1-, 3- and 5-year in clinical practice (Figure 4H). These findings showed that MMP11 may be useful for diagnosing PAAD patients.
Mutations of MMP11 in PAAD
The mutation frequency of MMP11 in PAAD was evaluated in the cBioPortal database. Five data sets [International Cancer Genome Consortium (ICGC), Queensland Centre for Medical Genomics (QCMG), University of Texas Southwestern Medical Center (UTSW), Clinical Proteomic Tumor Analysis Consortium (CPTAC), and TCGA-PanCancer Atlas], comprising 988 samples, were selected for analysis. The somatic mutation frequency of MMP11 in PAAD was 0.1%, which was mainly composed of missense mutations (Figure 5A). With only 1 in 1,000 samples, the mutation frequency was comparatively low. The MMP11 mutation types were then further assessed using COSMIC. For the sake of clarity, Figure 5 displays two pie charts of the mutation types. Approximately 55.41% of the samples were missense substitutions, 17.20% were synonymous substitutions, and 4.46% were nonsense substitutions (Figure 5B). The following substitution mutations were observed: C>T (43.88%), followed by G>A (25.74%), G>T (10.13%), and C>A (7.17%) (Figure 5C).
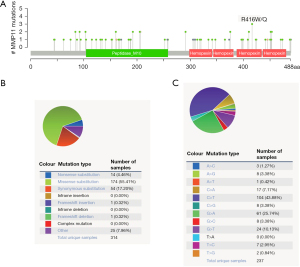
MMP11 methylation in PAAD
The DNA methylation levels of MMP11 in relation to each CpG (cytosine-phosphate-guanine) were analyzed using the MetSurv tool. The MethSurv results showed that there were 13 methylated CpG sites, of which cg27598806 had the highest degree of DNA methylation (Figure 6). Methylation levels at two CpG sites (i.e., cg27598806 and cg12395479) were associated with prognosis (Table 1). The OS rate of MMP11 hypermethylation patients at these CpG sites was better than that of MMP11 hypomethylated patients.
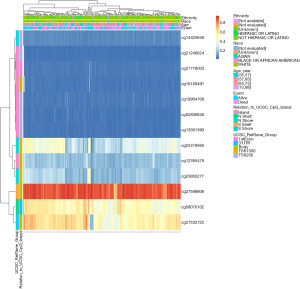
Table 1
CpG | HR | P value |
---|---|---|
Body-S_Shelf-cg27598806 | 0.431 | 0.003 |
Body-Island-cg12395479 | 0.566 | 0.03 |
PAAD, pancreatic adenocarcinoma; CpG, cytosine-phosphate-guanine; HR, hazard ratio.
Functional inference of MMP11 in PAAD
In disease research, DEGs are a popular tool for investigating potential biological effects through enrichment analyses. A functional enrichment analysis of transcriptome data from TCGA was conducted to further validate the potential biological role of MMP11 in PAAD. Based on the critical criterion of the median MMP11 expression |log2 (fold change)| >1, and adjusted P value <0.05, an analysis of TCGA RNA-Seq data between MMP11-high and -low groups identified 2,231 DEGs, of which 392 were upregulated and 1,839 were downregulated genes (Figure 7A).
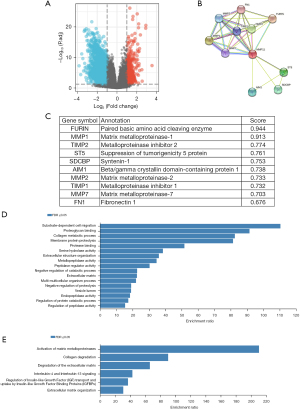
The biological process of MMP11 was identified by an overrepresentation enrichment analysis of the Linkedomics. We conducted a series of enrichment analyses to further determine the potential function of MMP11. First, using the STRING database, the PPI network of MMP11 was established. Next, 10 functional chaperone genes with high connectivity were selected (Figure 7B,7C), including FURIN, MMP1, TIMP2, ST5, SDCBP, AIM1, MMP2, TIMP1, MMP7, and FN1.
Next, we used the WebGestalt database to conduct an enrichment analysis of the above 10 related genes. The GO enrichment analysis identified three main functions: biological processes, cellular components, and molecular functions (P<0.05) (Table 2). The biological processes mainly included “substrate-dependent cell migration”, “collagen metabolic process”, “membrane protein proteolysis”, “extracellular structure organization”, “negative regulation of catabolic process”, “multi-multicellular organism process”, “negative regulation of proteolysis”, “regulation of protein catabolic process”, and “regulation of peptidase activity”. The cellular components mainly included the “extracellular matrix”, and “vesicle lumen”. The molecular functions mainly included “proteoglycan binding”, “protease binding”, “serine hydrolase activity”, “metallopeptidase activity”, “peptidase regulator activity”, and “endopeptidase activity”.
Table 2
Ontology | ID | Description | Gene ratio | Bg ratio | P value | P. adjust | q value |
---|---|---|---|---|---|---|---|
BP | GO:0022617 | Extracellular matrix disassembly | 6/7 | 80/18,670 | 3.57e−14 | 7.85e−12 | 3.42e−12 |
BP | GO:0043062 | Extracellular structure organization | 7/7 | 422/18,670 | 2.87e−12 | 3.16e−10 | 1.37e−10 |
BP | GO:0030198 | Extracellular matrix organization | 6/7 | 368/18,670 | 3.88e−10 | 2.84e−08 | 1.24e−08 |
BP | GO:0030574 | Collagen catabolic process | 4/7 | 47/18,670 | 1.23e−09 | 6.75e−08 | 2.94e−08 |
BP | GO:0032963 | Collagen metabolic process | 4/7 | 115/18,670 | 4.71e−08 | 2.07e−06 | 9.03e−07 |
CC | GO:0034774 | Secretory granule lumen | 3/7 | 321/19,717 | 1.43e−04 | 0.003 | 0.002 |
CC | GO:0060205 | Cytoplasmic vesicle lumen | 3/7 | 338/19,717 | 1.66e−04 | 0.003 | 0.002 |
CC | GO:0031983 | Vesicle lumen | 3/7 | 339/19,717 | 1.68e−04 | 0.003 | 0.002 |
CC | GO:0062023 | Collagen-containing extracellular matrix | 3/7 | 406/19,717 | 2.85e−04 | 0.004 | 0.002 |
CC | GO:0005796 | Golgi lumen | 2/7 | 102/19,717 | 5.47e−04 | 0.006 | 0.004 |
MF | GO:0004222 | Metalloendopeptidase activity | 3/7 | 103/17,697 | 6.59e−06 | 1.37e−04 | 7.05e−05 |
MF | GO:0004175 | Endopeptidase activity | 4/7 | 427/17,697 | 1.10e−05 | 1.37e−04 | 7.05e−05 |
MF | GO:0002020 | Protease binding | 3/7 | 128/17,697 | 1.27e−05 | 1.37e−04 | 7.05e−05 |
MF | GO:0008191 | Metalloendopeptidase inhibitor activity | 2/7 | 16/17,697 | 1.61e−05 | 1.37e−04 | 7.05e−05 |
MF | GO:0004252 | Serine-type endopeptidase activity | 3/7 | 160/17,697 | 2.47e−05 | 1.37e−04 | 7.05e−05 |
KEGG | hsa05219 | Bladder cancer | 1/3 | 41/8,076 | 0.02 | 0.07 | 0.030 |
KEGG | hsa04912 | GnRH signaling pathway | 1/3 | 93/8,076 | 0.03 | 0.07 | 0.030 |
KEGG | hsa01522 | Endocrine resistance | 1/3 | 98/8,076 | 0.04 | 0.07 | 0.030 |
KEGG | hsa04933 | AGE-RAGE signaling pathway in diabetic complications | 1/3 | 100/8,076 | 0.04 | 0.07 | 0.030 |
KEGG | hsa04066 | HIF-1 signaling pathway | 1/3 | 109/8,076 | 0.04 | 0.07 | 0.030 |
GO, Gene Ontology; KEGG, Kyoto Encyclopedia of Genes and Genomes; Bg, background; BP, biological process; CC, cellular component; MF, molecular function; GnRH, gonadotropin-releasing hormone; AGE-RAGE, advanced glycation end products-receptor for advanced glycation end products; HIF-1, hypoxia inducible factor-1.
The outcomes of the Reactome pathway enrichment analysis primarily encompassed MMP activation, collagen degradation, extracellular matrix (ECM) degradation, interleukin (IL)-4 and IL-13 signaling, insulin-like growth factor (IGF) regulation, and ECM organization (Figure 7D,7E). These results show that the MMP11 expression network has widespread effects on the prognosis and immune activation in PAAD.
Correlation of MMP11 expression with immune characteristics
Many studies have shown that the immune infiltration of different tumor types is related to prognosis and therapy response (14-16). We assessed whether MMP11 expression was associated with the degree of immune infiltration in human malignancies using TIMER and TISIDB data sets. We first used the CIBERSORT algorithm to assess the correlation between MMP11 expression and tumor-infiltrating immune cells in the PAAD microenvironment. As Figure 8A shows, there were significant differences in the composition of the 22 immune cell types in each sample.
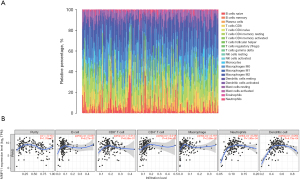
We examined the proportion of several immune cell types that infiltrate tumors in PAAD. According to the TIMER database, the MMP11 levels were positively correlated with the infiltrating levels of cluster of differentiation (CD)4+ T cells (R=0.218, P=4.34e−03), macrophages (R=0.167, P=0.03), neutrophils (R=0.282, P=1.90e−04), and dendritic cells (DCs) (R=0.264, P=4.73e−04) in the PAAD samples (Figure 8B).
We then evaluated the correlation between MMP11 expression and the abundance of 28 TILs using the TISIDB database. Figure 9A shows the relationship between MMP11 expression and TILs in different types of cancer. Figure 9B shows the associations between MMP11 and TILs, such as Tcm_CD8, Tem_CD8, Tcm_CD4, Tfh, Tgd, Th1, Th2, regulatory T cells (Tregs), natural killer (NK) cells, CD56bright, CD56dim, myeloid-derived suppressor cell (MDSC), natural killer T cell (NKT), Act_DC, plasmacytoid dendritic cell (pDC), macrophages, and eosinophils. These results suggest that MMP11 plays an important role in immune infiltration in PAAD.
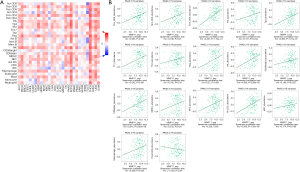
Further, we examined the relationships between MMP11 expression and various immune signatures to broaden the recognition of the association between MMP11 and immune infiltration, which included chemokines, receptors, and three kinds of immunomodulators. Chemokines and chemokine receptors are required for immune cell infiltration into tumors. The heatmap results showed that some chemokines and chemokine receptors were closely associated with the expression of MMP11 in human tumors (Figure 10A,10B). An association was also found between MMP11 expression and chemokines in PAAD. Notably, MMP11 expression was significantly associated with CCL3, CCL7, CCL13, CCL14, CCL17, CCL18, CCL22, CX3CL1, CXCL5, CXCL8, CXCL10, and CXCL14 (Figure 10C). Additionally, we showed that MMP11 expression was also significantly associated with chemokine receptors, including CCR8, CCR10, CXCR3, and CX3CR1 (Figure 10D). Most of these chemokines and chemokine receptors were found to be upregulated as MMP11 expression levels increased, which suggests that high MMP11 expression might be involved in immune cell migration to the TME.
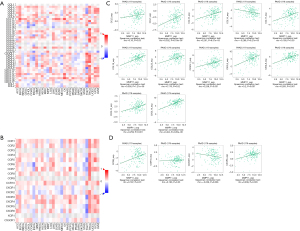
Immune checkpoint inhibitors (ICIs) are a novel tumor immunotherapy approach that have steadily improved the prognosis of patients with a variety of cancers. There are three categories of immunoinhibitors: immunostimulators, and MHC molecules. As Figures 11,12 show, several immunoinhibitors, immunostimulators, and MHC molecules were associated with the expression of MMP11 in tumors. A number of immune inhibitors, including ADORA2A, CD160, HAVCR2, KDR, LGALS9, PDCD1LG2, PVRL2, TGFB1, and TGFBR1, were strongly correlated with MMP11 expression in PAAD. In relation to the immunostimulators, MMP11 expression was significantly correlated with CD276, CD40, CD70, CD80, CD86, ENTPD1, HHLA2, IL2RA, IL6R, MICB, TMEM173, TNFRSF14, TNFRSF18, TNFRSF25, TNFRSF4, TNFRSF9, TNFSF4, TNFSF9, and ULBP1. Additionally, an intense association was found between MMP11 expression and MHC molecules, including B2M, HLA-A, HLA-B, HLA-C, HLA-DQA2, HLA-F, TAP1, TAP2, and TAPBP.
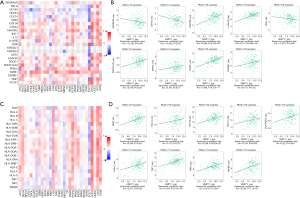
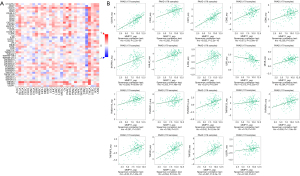
Programmed cell death protein 1 (PD-1) (PDCD-1), programmed cell death protein ligand 1 (PD-L1) (CD274), cytotoxic T lymphocyte antigen 4 (CTLA-4), and HAVCR2 are significant immune checkpoints responsible for tumor immune evasion. Thus, we further examined their relationship with MMP11 expression in the GEPIA database. An important positive correlation was found between MMP11 and PD-L1, CTLA-4, and HAVCR2 in PAAD; however, no relationship was found between PD-1 and MMP11 (Figure 13). This may explain why PD-1 ICIs are less effective in PAAD.
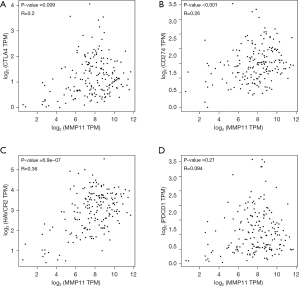
In general, we showed that MMP11 is broadly involved in regulating various immune molecules in PAAD to influence immune infiltration in the TME.
Discussion
This study showed that high MMP11 mRNA levels were associated with a worse prognosis in several cancers, including PAAD. Through a comprehensive bioinformatics investigation, we systematically analyzed the clinical significance and expression level of MMP11 in PAAD. Furthermore, our findings also revealed that MMP11 expression was strongly correlated with the numbers of tumor-infiltrating immune cells in PAAD, depending on the levels of markers for different immune cell types. As a result, our research provides new insights into the fatal functions of MMP11 and suggests that it could be a prognostic biomarker of immune infiltration in PAAD.
Our findings showed that MMP11 was overexpressed in many cancers and was associated with a poor prognosis. Previous research has shown that the downregulation of MMP11 expression reduced the growth ability and colony formation ability of cancer cells (17-19). Unlike other MMP members, MMP11 has unique functions and signaling pathways. One study showed that MMP11 enhanced endoplasmic reticulum stress, altered the mitochondrial unfolded protein response by metabolic reprogramming, and increased the IGF1/AKT/FoxO1 signaling pathway to promote the growth of early mouse mammary gland tumors (20). A study of breast cancer cell lines showed that MMP11 inhibited Smad2 degradation and activated the transforming growth factor-β (TGF-β) signaling pathway through the ubiquitin proteasome pathway, thereby significantly increasing cell proliferation and colony formation (8). Another study showed that MMP11-overexpressing macrophages secreted chemokine CCL2 and activated the MAPK pathway in breast cancer cells through its receptor CCR2, thereby promoting the migration of human epidermal growth factor receptor 2 (HER2) + breast cancer cells (21). However, little is known about the role of MMP11 in PAAD and the immune system.
Thus, we evaluated MMP11 expression in PAAD using TCGA, Kaplan-Meier plotter, and GEPIA databases and clinical samples. We discovered that MMP11 was overexpressed in PAAD tissues compared with normal pancreatic tissues. The expression of MMP11 was significantly correlated with tumor stage, histological grade, and pathological stage. The Kaplan-Meier survival analysis revealed that OS and DFS were worse in patients with elevated MMP11 expression. High MMP11 expression was also associated with a poor prognosis and advanced cancer stage. The ROC analysis also confirmed the diagnostic value of MMP11. Thus, MMP11 could be applied as a latent worthy diagnostic and prognostic biomarker for PAAD. We believe that in the future, serum MMP11 expression detection can become an important method for non-invasive diagnosis of PAAD. At the same time, detection of MMP11 expression in serum and pathology of PAAD can improve the prognosis evaluation and preoperative or postoperative decision-making.
Gene mutations are closely associated to tumors and are usually related to a poor prognosis; however, the somatic mutation frequency of MMP11 in PAAD was low, and mainly composed of missense mutations. We then examined the relationship between the DNA methylation levels of MMP11 and the prognosis of patients with PAAD. The hypermethylation of two CpG sites (i.e., cg27598806 and cg12395479) was associated with better OS.
To further clarify the potential biological functions of MMP11, we conducted GO and Reactome pathway enrichment analyses and found that MMP11 and its interacting genes were mainly involved in collagen degradation, ECM degradation, and IL-4 and IL-13 signaling. Numerous studies have shown that the tumor-related ECM is involved in stimulating tumor cell growth, invasion, metastasis, and angiogenesis, and counteracted cell death and drug diffusion (22,23). Stroma constitutes the bulk of tumors in pancreatic cancer, accounting for 90% of the total tumor mass, composed of many cellular and non-cellular components, including collagen I, collagen IV, laminin, and fibronectin (24). ECM is highly dysregulated in cancer, and its loss of homeostasis and integrity is considered one of the signs of cancer, which usually leads to cancer progression and metastasis (23).
The Th2 cytokines, IL-4, and IL-13 are related in structure and function. They regulate immune responses and the immune microenvironment, especially in cancer. The IL-4/-13 cytokine receptor system has a noticeable indigenous effect on the survival, progression, and metastasis of cancer cells (25-27). The increased activity of IL-4 and IL-13 in pancreatic cancer is closely related to tumor occurrence and metastasis (28). IL-4 and IL-13 have been proven to be fatal regulatory cytokines in the TME and to activate tumor-associated macrophages (TAMs) and MDSCs that mediate tumor-promoting activity (29,30). The IL-4 receptor is mediated through the phosphorylation activation pathway of the JAK protein, of which the main pathways are IRS/PI3K/AKT, JAK/STAT6, and mTOR. The IL-4 receptor is activated and expressed in pancreatic cancer to mediate tumor-promoting activity and enhance anti-tumor response (31). A study has shown that the loss of IL-13Rα1 induces the apoptosis of pancreatic cancer cells and is related to the transition from epithelium to mesenchyme. Additionally, the absence of IL-13Rα1 also eliminates the effects of exogenous IL-4 and IL-13 stimulation (32). These studies verified the function of MMP11 in PAAD occurrence and progression, as well as the relationship between MMP11 and immune regulation of PAAD.
Tumor immunotherapy has made significant advancements recently. Notably, ICIs represented by antibodies against PD-1, PD-L1, and CTLA-4 have been authorized for the treatment of various clinical tumors (33). Compared with chemotherapy, immunotherapy is more specific, targeted, and less toxic; however, in PAAD, the efficacy of immunotherapy alone is not ideal. PAAD is usually deemed a poorly immunogenic cancer type, and the analysis of large PAAD genomic data sets has shown that only a small fraction of PAAD are immunocompetent (34). This may be due to the low mutation burden of PAAD and the dense, inaccessible TME, which is characterized by fibrosis, hypoxia, and immunosuppression, which makes the tumor immune “cold”.
Our research proposes a correlation between MMP11 expression and the infiltration of immune cells in PAAD. MMP11 expression was positively associated with CD4+ T cells, macrophages, neutrophils, DCs, NK cells, and Tregs. Additionally, the increase of MMP11 expression was related to immunomodulators, chemokines, and chemokine receptors. In addition, MMP11 was positively associated with the biomarkers of these infiltrating immune cells.
DCs are a group of diverse specialized antigen-presenting cells that have an important function in the initiation and regulation of innate and adaptive immune response (35). Usually known as “nature’s adjuvants”, DCs are considered the most effective antigen-presenting cells, and can activate naive and memory immune responses (36). At present, researchers are very interested in regulating DC function to increase cancer immunotherapy. Many strategies have been developed for DCs in cancer, including using immunomodulators to mobilize and activate endogenous DCs, and producing DC-based vaccines. We discovered that MMP11 was positively correlated with DC markers, such as HLA-DRA and CD11c, which suggests that targeting MMP11 may affect the function of DCs in PAAD.
TAMs are a pivotal component of the cancer microenvironment, and promote the occurrence and malignant progression of cancer by stimulating angiogenesis, increasing tumor cell migration, invasion, and intravasation, and inhibiting anti-tumor immunity (37,38). The relationship between MMP11 expression and M1/M2 macrophage is positive correlation. This indicates that MMP11 has the potential function of regulating TAM polarization.
Tregs play a central role in self-tolerance and the inhibition of an effective anti-tumor immune response (39). Treg frequency has been shown to be positively correlated with tumor metastasis and a poor prognosis in PAAD (40). We founded that upregulated MMP11 expression was closely associated to Treg markers (FOXP3, CCR8, and TGF-β) and T cell exhausted markers (CTLA-4, TIM-3, and PD-L1).
Immune checkpoint blockade is the main immunotherapy strategy. MMP11 plays a vital role in the recruitment and regulation of TILs in PAAD, but further study is needed on the molecular mechanism and function of MMP11 in regulating TME. Targeting MMP11 may have value in enhancing the efficacy of immunotherapy and improving drug resistance in PAAD. Further research on MMP11 related signaling pathways and their impact on cancer biological behavior may lead to breakthroughs in PAAD treatment. Thus, further study on the molecular mechanism and function of MMP11 in regulating TME should be conducted.
Conclusions
In this study, we demonstrated the value of MMP11 in the diagnosis and prognosis of PAAD. MMP11 may not only be involved in the occurrence and progress of PAAD, but may also be involved in the immune regulation of PAAD. Thus, MMP11 may be a latent diagnosis and prognosis biomarker and a target for therapy in PAAD. These findings provide an opportunity to promote the development of new immunotherapy strategies.
Acknowledgments
Funding: This work was supported by
Footnote
Reporting Checklist: The authors have completed the REMARK reporting checklist. Available at https://jgo.amegroups.com/article/view/10.21037/jgo-24-425/rc
Peer Review File: Available at https://jgo.amegroups.com/article/view/10.21037/jgo-24-425/prf
Conflicts of Interest: All authors have completed the ICMJE uniform disclosure form (available at https://jgo.amegroups.com/article/view/10.21037/jgo-24-425/coif). The authors have no conflicts of interest to declare.
Ethical Statement: The authors are accountable for all aspects of the work in ensuring that questions related to the accuracy or integrity of any part of the work are appropriately investigated and resolved. The study was conducted in accordance with the Declaration of Helsinki (as revised in 2013).
Open Access Statement: This is an Open Access article distributed in accordance with the Creative Commons Attribution-NonCommercial-NoDerivs 4.0 International License (CC BY-NC-ND 4.0), which permits the non-commercial replication and distribution of the article with the strict proviso that no changes or edits are made and the original work is properly cited (including links to both the formal publication through the relevant DOI and the license). See: https://creativecommons.org/licenses/by-nc-nd/4.0/.
References
- Siegel RL, Miller KD, Wagle NS, et al. Cancer statistics, 2023. CA Cancer J Clin 2023;73:17-48. [Crossref] [PubMed]
- Gugenheim J, Crovetto A, Petrucciani N. Neoadjuvant therapy for pancreatic cancer. Updates Surg 2022;74:35-42. [Crossref] [PubMed]
- Maggino L, Malleo G, Crippa S, et al. CA19.9 Response and Tumor Size Predict Recurrence Following Post-neoadjuvant Pancreatectomy in Initially Resectable and Borderline Resectable Pancreatic Ductal Adenocarcinoma. Ann Surg Oncol 2023;30:207-19. [Crossref] [PubMed]
- Hu ZI, O'Reilly EM. Therapeutic developments in pancreatic cancer. Nat Rev Gastroenterol Hepatol 2024;21:7-24. [Crossref] [PubMed]
- Basset P, Bellocq JP, Wolf C, et al. A novel metalloproteinase gene specifically expressed in stromal cells of breast carcinomas. Nature 1990;348:699-704. [Crossref] [PubMed]
- Bai L, Huo R, Fang G, et al. MMP11 is associated with the immune response and immune microenvironment in EGFR-mutant lung adenocarcinoma. Front Oncol 2023;13:1055122. [Crossref] [PubMed]
- Gao C, Zhang Y, Sun H. Mechanism of miR-340-5p in laryngeal cancer cell proliferation and invasion through the lncRNA NEAT1/MMP11 axis. Pathol Res Pract 2022;236:153912. [Crossref] [PubMed]
- Zhuang Y, Li X, Zhan P, et al. MMP11 promotes the proliferation and progression of breast cancer through stabilizing Smad2 protein. Oncol Rep 2021;45:16. [Crossref] [PubMed]
- Huang HC, Shiu BH, Su SC, et al. The Impact of Matrix Metalloproteinase-11 Polymorphisms on Colorectal Cancer Progression and Clinicopathological Characteristics. Diagnostics (Basel) 2022;12:1685. [Crossref] [PubMed]
- Tang Z, Li C, Kang B, et al. GEPIA: a web server for cancer and normal gene expression profiling and interactive analyses. Nucleic Acids Res 2017;45:W98-W102. [Crossref] [PubMed]
- Vasaikar SV, Straub P, Wang J, et al. LinkedOmics: analyzing multi-omics data within and across 32 cancer types. Nucleic Acids Res 2018;46:D956-63. [Crossref] [PubMed]
- Szklarczyk D, Gable AL, Nastou KC, et al. The STRING database in 2021: customizable protein-protein networks, and functional characterization of user-uploaded gene/measurement sets. Nucleic Acids Res 2021;49:D605-12. [Crossref] [PubMed]
- Tate JG, Bamford S, Jubb HC, et al. COSMIC: the Catalogue Of Somatic Mutations In Cancer. Nucleic Acids Res 2019;47:D941-7. [Crossref] [PubMed]
- Mao X, Xu J, Wang W, et al. Crosstalk between cancer-associated fibroblasts and immune cells in the tumor microenvironment: new findings and future perspectives. Mol Cancer 2021;20:131. [Crossref] [PubMed]
- Cao LL, Kagan JC. Targeting innate immune pathways for cancer immunotherapy. Immunity 2023;56:2206-17. [Crossref] [PubMed]
- Bandi DSR, Sarvesh S, Farran B, et al. Targeting the metabolism and immune system in pancreatic ductal adenocarcinoma: Insights and future directions. Cytokine Growth Factor Rev 2023;71-72:26-39. [Crossref] [PubMed]
- Martin-Morales L, Manzano S, Rodrigo-Faus M, et al. Germline gain-of-function MMP11 variant results in an aggressive form of colorectal cancer. Int J Cancer 2023;152:283-97. [Crossref] [PubMed]
- Ge F, Zeng C, Wang J, et al. Cancer-associated fibroblasts drive early pancreatic cancer cell invasion via the SOX4/MMP11 signalling axis. Biochimica et Biophysica Acta (BBA) - Molecular Basis of Disease 2024;1870:166852. [Crossref] [PubMed]
- Song Z, Luo J, Wu M, et al. linc00511 Knockdown Inhibits Lung Cancer Progression by Regulating miR-16-5p/MMP11. Crit Rev Eukaryot Gene Expr 2023;33:17-30. [Crossref] [PubMed]
- Tan B, Jaulin A, Bund C, et al. Matrix Metalloproteinase-11 Promotes Early Mouse Mammary Gland Tumor Growth through Metabolic Reprogramming and Increased IGF1/AKT/FoxO1 Signaling Pathway, Enhanced ER Stress and Alteration in Mitochondrial UPR. Cancers (Basel) 2020;12:2357. [Crossref] [PubMed]
- Kang SU, Cho SY, Jeong H, et al. Matrix metalloproteinase 11 (MMP11) in macrophages promotes the migration of HER2-positive breast cancer cells and monocyte recruitment through CCL2-CCR2 signaling. Lab Invest 2022;102:376-90. [Crossref] [PubMed]
- Sleeboom JJF, van Tienderen GS, Schenke-Layland K, et al. The extracellular matrix as hallmark of cancer and metastasis: From biomechanics to therapeutic targets. Sci Transl Med 2024;16:eadg3840. [Crossref] [PubMed]
- Yuan Z, Li Y, Zhang S, et al. Extracellular matrix remodeling in tumor progression and immune escape: from mechanisms to treatments. Mol Cancer 2023;22:48. [Crossref] [PubMed]
- Slapak EJ, Duitman J, Tekin C, et al. Matrix Metalloproteases in Pancreatic Ductal Adenocarcinoma: Key Drivers of Disease Progression? Biology (Basel) 2020;9:80. [Crossref] [PubMed]
- Gunassekaran GR, Poongkavithai Vadevoo SM, Baek MC, et al. M1 macrophage exosomes engineered to foster M1 polarization and target the IL-4 receptor inhibit tumor growth by reprogramming tumor-associated macrophages into M1-like macrophages. Biomaterials 2021;278:121137. [Crossref] [PubMed]
- LaMarche NM, Hegde S, Park MD, et al. An IL-4 signalling axis in bone marrow drives pro-tumorigenic myelopoiesis. Nature 2024;625:166-74. [Crossref] [PubMed]
- Jaén M, Martín-Regalado Á, Bartolomé RA, et al. Interleukin 13 receptor alpha 2 (IL13Rα2): Expression, signaling pathways and therapeutic applications in cancer. Biochim Biophys Acta Rev Cancer 2022;1877:188802. [Crossref] [PubMed]
- Shi J, Song X, Traub B, et al. Involvement of IL-4, IL-13 and Their Receptors in Pancreatic Cancer. Int J Mol Sci 2021;22:2998. [Crossref] [PubMed]
- Little AC, Pathanjeli P, Wu Z, et al. IL-4/IL-13 Stimulated Macrophages Enhance Breast Cancer Invasion Via Rho-GTPase Regulation of Synergistic VEGF/CCL-18 Signaling. Front Oncol 2019;9:456. [Crossref] [PubMed]
- Zhang Y, Liu Y, Wang J, et al. Atractylenolide II inhibits tumor-associated macrophages (TAMs)-induced lung cancer cell metastasis. Immunopharmacol Immunotoxicol 2022;44:227-37. [Crossref] [PubMed]
- Kwaśniak K, Czarnik-Kwaśniak J, Maziarz A, et al. Scientific reports concerning the impact of interleukin 4, interleukin 10 and transforming growth factor β on cancer cells. Cent Eur J Immunol 2019;44:190-200. [Crossref] [PubMed]
- Shi J, Shen X, Kang Q, et al. Loss of Interleukin-13-Receptor-Alpha-1 Induces Apoptosis and Promotes EMT in Pancreatic Cancer. Int J Mol Sci 2022;23:3659. [Crossref] [PubMed]
- Galluzzi L, Humeau J, Buqué A, et al. Immunostimulation with chemotherapy in the era of immune checkpoint inhibitors. Nat Rev Clin Oncol 2020;17:725-41. [Crossref] [PubMed]
- Shi Y, Wang Y, Dong H, et al. Crosstalk of ferroptosis regulators and tumor immunity in pancreatic adenocarcinoma: novel perspective to mRNA vaccines and personalized immunotherapy. Apoptosis 2023;28:1423-35. [Crossref] [PubMed]
- Wculek SK, Cueto FJ, Mujal AM, et al. Dendritic cells in cancer immunology and immunotherapy. Nat Rev Immunol 2020;20:7-24. [Crossref] [PubMed]
- Kang BH, Lee HK. Dendritic Cell-Based Immunotherapy in Hot and Cold Tumors. Int J Mol Sci 2022;23:7325. [Crossref] [PubMed]
- Shu Y, Cheng P. Targeting tumor-associated macrophages for cancer immunotherapy. Biochim Biophys Acta Rev Cancer 2020;1874:188434. [Crossref] [PubMed]
- Storz P. Roles of differently polarized macrophages in the initiation and progressionof pancreatic cancer. Front Immunol 2023;14:1237711. [Crossref] [PubMed]
- Tay C, Tanaka A, Sakaguchi S. Tumor-infiltrating regulatory T cells as targets of cancer immunotherapy. Cancer Cell 2023;41:450-65. [Crossref] [PubMed]
- Zhang Y, Lazarus J, Steele NG, et al. Regulatory T-cell Depletion Alters the Tumor Microenvironment and Accelerates Pancreatic Carcinogenesis. Cancer Discov 2020;10:422-39. [Crossref] [PubMed]
(English Language Editor: L. Huleatt)