Senescence-related signatures predict prognosis and response to immunotherapy in colon cancer
Highlight box
Key findings
• Analysis of The Cancer Genome Atlas (TCGA) and Gene Expression Omnibus (GEO) databases led to the discovery of a new biomarker CDKN2A for prognostic evaluation in colon cancer patients, and the results were validated by immunohistochemistry using clinical patients’ pathology samples. A survival prediction model comprising five senescence genes for colon cancer patients was then established. Finally, a new potential predictor for colon cancer patients in immunotherapy responsiveness was discovered.
What is known, and what is new?
• CDKN2A is a highly expressed gene in intestinal cancer tumor tissues, and ETS2 is a lowly expressed gene in intestinal cancer tissues.
• Both CDKN2A and ETS2 can be used as survival prognostic markers in bowel cancer patients.
What is the implication, and what should change now?
• We discovered a new survival prediction model and new potential predictors for colon cancer immunotherapy.
Introduction
Colon adenocarcinoma (COAD) is the third most prevalent in males and the second most prevalent in females, and is the prevailing subtype in colorectal cancer (CRC), and it was estimated that there were approximately 1.9 million new cases of COAD and 0.9 million COAD-related deaths worldwide in 2020 (1-3). The treatment methods for CRC include traditional surgery, chemotherapy, radiation therapy, and immunotherapy; however, the five-year survival rate of advanced COAD remains low due to postoperative recurrence and metastasis (4). There is increasing evidence that aggressive COAD may benefit from immunotherapy and targeted therapy, but only 20% of patients benefit from immunotherapy (5-7). Thus, the exploration of potential cancer progression and prognostic biomarkers is of great importance for COAD prognosis predictions and immunotherapy evaluations.
Cellular senescence is a protective mechanism that maintains homeostasis and is used to eliminate diseased, dysfunctional, and unwanted cells (8). Cellular senescence has long been considered a protective mechanism against tumors. However, multiple experiments have shown that aging cancer cells have different proliferative signals and can avoid apoptosis, induce angiogenesis, stimulate invasion and metastasis, and inhibit tumor immunity (9-12). The accumulation of cellular damage leads to cellular senescence and cancer. Numerous studies have shown that senescent cells that are not cleared by the immune system promote the senescence-associated secretory phenotype and release cytokines, growth factors, and extracellular matrix components, leading to the aging process and tumor development (13-16). An increasing number of studies have shown a significant correlation between the senescence-related signature (SRS) and the prognosis of tumor patients (17,18). However, there is no report relating the SRS to CRC in terms of clinical relevance and prognosis.
CDKN2A serves as an important tumor suppressor gene in various human malignancies, including CRC, and its activation prevents carcinogenesis via the induction of cell growth arrest and senescence (17). Aging is related to replicative senescence, and CDKN2A expression levels increase with aging in most mammalian tissues (19-22). During the aging process, the regenerative ability of stem cells in different tissues also decreases (23). In vitro studies have found that increased CDKN2A expression leads to reduced stem cell proliferation, thereby confirming the relationship between CDKN2A expression and stem cell regeneration (24-26). Currently, little research has been conducted on ETS2 in tumors, and some studies have indicated that ETS2 is prone to accumulate DNA damage (27-29); however, in a study by Chen et al. ETS2 was found to be a pro-oncogene in CRC (30). Therefore, further research needs to be conducted to explore whether CDKN2A and ETS2 have potential value in predicting prognosis and immune therapy response.
In the present study, to systematically evaluate the correlation between cellular senescence and prognosis in CRC, we applied bioinformatics calculation methods to establish a risk model based on cellular senescence-related genes. We also explored this risk model as a potential biomarker for colon cancer patient’s prognosis and immunotherapy response and examined whether it could provide a reference for personalized therapy. To further validate our model, we also explored the clinical importance of CDKN2A and ETS2 in CRC. In summary, our findings pinpointed senescence-related hallmarks and showed their ability to reliably forecast the prognosis of CRC patients. Moreover, we found that CDKN2A and ETS2 have the potential to serve as biomarkers for CRC. We present this article in accordance with the TRIPOD reporting checklist (available at https://jgo.amegroups.com/article/view/10.21037/jgo-24-339/rc).
Methods
Data collection and processing
In the present study, RNA-sequencing (RNA-seq) data (n=437), masked mutation data (n=375), and clinical characteristics COAD data (n=385) from The Cancer Genome Atlas (TCGA) database were downloaded and analyzed on April 12, 2022 (https://portal.gdc.cancer.gov/analysis_). External cohort information validation was performed on the RNA-seq data and clinical COAD information (n=566) was extracted from the Gene Expression Omnibus (GEO) database (https://www.ncbi.nlm.nih.gov/gds). The data discussed in this publication have been deposited in NCBI’s GEO (31) and are accessible through GEO Series accession number GSE40967 (https://www.ncbi.nlm.nih.gov/geo/query/acc.cgi?acc=GSE40967) which has large sample size and comprehensive clinical information. Mvigor210 clinical data (n=348) were downloaded and used to verify whether the screened genes could distinguish between high- and low-risk groups in the immunotherapy clinical database.
Identification of cellular senescence-related differentially expressed genes (DEGs)
TCGA and GEO expression data were normalized to transcripts per million (TPM) values. A collection of genes related to cellular aging was downloaded from CellAge: The Database of Cell Senescence Genes (https://genomics.senescence.info/cells) (32). Tumor mutational burden (TMB) is the number of mutated bases per million bases in each sample. By investigating the TMB sample, specific details of the sample can be determined, such as gene name, mutation location, mutation classification, mutation type, and base change. All R packages [limma (33), glmnet (34), survival (35), caret (36), survminer (37)] are downloaded from https://www.r-project.org/ (38). TCGA and GEO data were analyzed using the R “limma” package and the expression levels of the overlapping genes were filtered and retained. After data correction, the expression levels of the senescence genes in these two databases were extracted. According to the difference in TCGA cellular senescence gene expression between the normal and tumor samples of COAD, we screened out 44 DEGs (with a fold-difference of 1 time the normal value; P<0.05).
Establishment and validation of a prognostic model of cellular senescence-related genes
The limma algorithm was used to identify the DEGs between the senescence clusters. Next, a univariate Cox regression analysis was used to calculate the prognostic DEGs and genes with a hazard ratio (HR) <1 or >1 were considered protective or risk genes, respectively (P<0.05). The risk score was calculated as follows: risk score = ∑i6Xi ×Yi (X: coefficients, Y: gene expression level). To avoid overmatching, based on the risk score, the “glmnet” and “survival” packages were used for the least absolute shrinkage and selection operator (LASSO) regression analysis to identify significant genes. TCGA and GEO data were used as the experimental group and the verification group, respectively. Next, patients with CRC were assigned to high- and low-risk subgroups based on their risk score relative to the median risk score. A Kaplan-Meier survival analysis was conducted to evaluate the prognosis of the low- and high-risk CRC patients. Receiver operating characteristic (ROC) curves were used to confirm the senescence-related prognostic model predictive stability. To explore the potential mechanisms of low- and high-risk CRC, Gene Ontology (GO) and Kyoto Encyclopedia of Genes and Genomes (KEGG) analyses of the DEGs in low- and high-risk CRC were performed using the limma algorithm (P<0.05). A gene set enrichment analysis (GSEA) was conducted to determine the pathway activity in the different risk groups (P<0.05).
Analysis of immune infiltration
Correlation data between the senescence-related gene expression and immune cell infiltration (e.g., B cells, cluster of differentiation CD4+ T cells, CD8+ T cells, neutrophils, macrophages, and dendritic cells) were obtained from the “GENE” module in the TIMER database (http://timer.cistrome.org).
Validation of the IMvigor210 data model
We verified the expression data and survival status of the five significant genes screened by TCGA using the IMvigor210 clinical data. Next, R language packages (“survival”, “caret”, “glmnet”, and “survminer”) were used to extract the expression data of the model genes. Each patient’s risk score was then calculated using the formula of the model. Subsequently, we compared the differences in survival between the high- and low-risk groups, obtained the expression data of the immune checkpoint-related genes, examined the survival differences between the groups, and created combined survival curves to verify the accuracy of the risk prognosis genes.
Patients and tissue specimens
All the clinical samples were sourced from the Affiliated Cancer Hospital and Institute of Guangzhou Medical University. Inclusion criteria: age >18 years; all received surgical treatment; all have postoperative pathology specimen results; complete clinical information. Exclusion criteria: lost patients; patients with multifocal tumors; non-surgical patients. Formalin-fixed and paraffin-embedded samples from 90 patients were collected from September 2014 to September 2021. All the patients who underwent surgery were included in the study, while all the patients who received chemotherapy, immunotherapy, and targeted therapy were excluded from the study. All the participants were informed of and agreed to participate in this research study, which was approved by Ethics Committee of Affiliated Cancer Hospital and Institute of Guangzhou Medical University [No. GYZL-ZN-2023(041)]. The study was conducted in accordance with the Declaration of Helsinki (as revised in 2013).
Immunohistochemical (IHC) analysis
Hematoxylin and eosin (H&E) staining or IHC tests were performed to analyze the target protein. Specifically, after dewaxing in xylene and hydration with alcohol gradients (100% for 5 minutes, 95% for 5 minutes, 85% for 5 minutes, and 75% for 5 minutes), endogenous peroxidase activity was blocked by incubation with 2% hydrogen peroxide. Next, antigen recovery was performed by microwave irradiation in 10 mM of citrate buffer at 95 ℃ for 15 minutes. The slices were then incubated overnight with primary antibodies (CDKN2A: 1:200, Abcam, ab108349, Cambridge, UK; ETS2: 1:20, Affinity DF7129, Ancaster, Canada) at 4 ℃. The secondary antibody (LSAB + Kit, Dako, Shanghai, China) linked to streptavidin was applied for 30 minutes, followed by streptavidin peroxidase for 15 minutes.
Statistical analysis
SPSS (25th edition, IBM, Armonk, New York, USA) was used for the statistical analysis. The Student’s t-test and the Chi-square test were used to estimate the differences between the groups. A P value <0.05 was considered statistically significant, and a P value <0.001 was considered highly statistically significant.
Results
Identification of DEGs in CRC
First, we screened 44 DEGs (of which 33 were highly expressed and 11 were lowly expressed) by analyzing the profiles from TCGA-COAD data set that comprised 398 COAD samples and 39 normal samples (Figure 1A). In the forest plot, the low-risk genes are represented by green columns, while the high-risk genes are represented by red columns represent (Figure 1B). To further narrow down the scope of the target genes, the LASSO regression algorithm was used to identify five senescence-related genes (i.e., CDKN2A, CXCL1, ETS2, SERPINE2, and SNAI1) (Figure 1C-1E). In addition, we performed a gene-to-gene correlation analysis of these five senescence-related genes (Figure S1). Positive correlations are shown in red, while negative correlations are shown in green (Figure 1F).
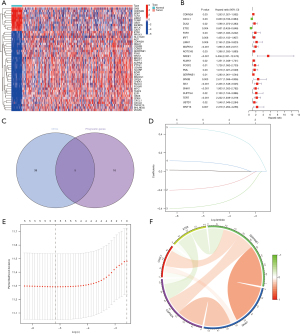
Development of a prognostic gene model in TCGA and GEO cohorts
To establish a predictive model, 379 TCGA samples and 562 GEO samples of COAD were obtained. We also processed the risk stratification of the five genes in TCGA and GEO data sets. CDKN2A, SERPINE2, and SNAI1 were classified as high-risk genes with increased expression levels, while CXCL1 and ETS2 were classified as low-risk genes with reduced expression levels (Figure 2A and Figure S2A). As shown in Figure 2B,2C and Figure S2B,S2C, the scoring heatmaps of the two databases were largely consistent, indicating that the survival-related prognostic gene model had been successfully established. Next, we analyzed the predictive value of the risk genes in patients with COAD obtained from TCGA and GEO data sets using Kaplan-Meier plotter. In relation to the risk classification of COAD, a strong association was found between the presence of high-risk senescence genes and lower overall survival rates, while the low-risk senescence genes were associated with better OS (Figure 2D and Figure S2D). To further validate the successful establishment of the prediction model, a time-dependent ROC analysis was performed. As shown in Figure 2E and Figure S2E, after processing the data from TCGA, we concluded that the predictions of the model for years 1, 3, and 5 were significant, while in relation to the GEO data, the predictions for years 1 and 3 were significant.
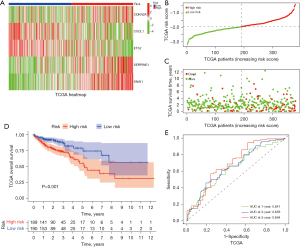
Diagnostic and prognostic value of senescence-related genes in COAD
Next, we examined the correlations between the senescence-related genes and the prognosis of patients with COAD and tested whether the risk assessment of the screening genes could serve as an independent prognostic factor. Thus, multivariate and univariate Cox analyses were performed to analyze the clinical information (age, sex, tumor stage, and clinical factors) of patients with COAD obtained from TCGA. As shown in (Figure 3A), the risk score can be used as an important independent factor for predicting survival in the univariate analysis [HR =3.455, 95% confidence interval (CI): 2.146–5.565]. Further, after adjusting for other factors, a comprehensive analysis showed that the risk score was an important independent factor in the multivariate analysis (HR =2.588, 95% CI: 1.524–4.396) (Figure 3B). Besides, age was also a negative prognostic factor in univariate analysis (HR =1.032, 95% CI: 1.009–1.055) and in the multivariate analysis (HR =1.040, 95% CI: 1.018–1.063) (Figure 3A,3B). Next, we analyzed high- and low-risk groups of 5 prognostic gene model patients’ survival probability in stage I–II, T stage 3–4 and M stage 0. The results showed that the high-risk group patients had a poorer survival compared to low-risk group (Figure 3C-3E).
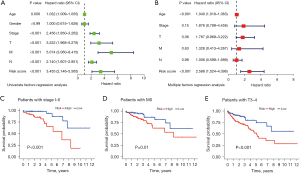
TMB and immune infiltration
To further investigate the relationship between the TMB and senescence-related genes in COAD, we divided the TCGA-TMB data set into high- and low-expression groups merged to high- and low-risk groups of prognostic gene model. Compared with the high TMB expression group, the low TMB expression group showed better survival (P=0.03) (Figure 4A). Moreover, the prognosis of patients was even worse when high TMB was accompanied by high-risk genes, while the prognosis of patients was better when low TMB is accompanied by low-risk genes (P=0.001) (Figure 4B).
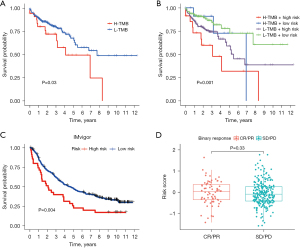
We then used IMvigor data survival of high- and low-risk patients. Patients classified as high-risk had a poorer prognosis compared to those in the low-risk group (P=0.004) (Figure 4C); however, there was no statistically significant difference in the treatment response [complete response (CR)/partial response (PR) vs. stable disease (SD)/progressive disease (PD)] between these groups (Figure 4D). To further investigate the impact of immune-related genes and senescence-related genes on patient prognosis, we categorized the patients into four groups (high gene expression + high risk, high gene expression + low risk, low gene expression + high risk, and low gene expression + low risk). The final results showed statistically significant differences in the comparisons between all four groups, which suggests that these two factors have a synergistic effect on patient prognosis (Figure S3).
A GSEA based on the senescence-related genes in CRC and KEGG pathway enrichment analysis revealed that the predominant pathways were melanogenesis, vascular smooth muscle contraction, leukocyte transendothelial migration, focal adhesion, and basal cell carcinoma (Figure 5A). Moreover, we also analyzed the correlation between immune checkpoint-related genes and risk genes using public databases. The immune checkpoint expression analysis identified the DEGs, including LAG3, PDCD1, and PDCD1LG2, in the high- and low-risk groups (Figure 5B). Moreover, to further explore the connection between immune cells and senescence-related genes in the tumor microenvironment (TME), we performed a detailed interpretation using timer immune infiltration databases including CIBERSORT, CIBERSORT-ABS, XCELL, EPIC, MCPCOUNTER, and QUANTISEQ. A statistically significant difference between the high- and low-risk groups was found for a variety of immune cells, including macrophages (Figure 5C and Figure S4). The KEGG biological process terms were mainly enriched in the cellular senescence, bladder cancer, cell cycle, human T-cell leukemia virus 1 infection, and the p53 signaling pathway, while the GO biological process terms were mainly enriched in the mitotic cell cycle phase transition, the regulation of the mitotic cell cycle phase transition, the regulation of the cell cycle phase transition, the regulation of the mitotic cell cycle, and the negative regulation of cell cycle (Figure 5D).
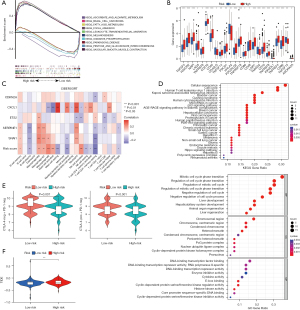
The immunotherapy correlation analysis suggested that patients in the high-risk group had worse immunotherapy outcomes than patients in the low-risk group when they were CTLA-4-positive PD-1-negative and CTLA-4-negative PD-1-negative (Figure 5E). An immunization escape correlation analysis was performed in the high- and low-risk groups, and the outcomes revealed that the likelihood of immunization escape was higher in the high-risk group than in the low-risk group, which suggests that the outcome of immunotherapy may be less successful for patients in the high-risk group than in the low-risk group (Figure 5F).
Clinical sample results
To further validate the above results in the clinical samples, we obtained clinical information, including sex, age, stage, time, treatment method, time of death, height, weight, pathological results, biochemical indicators, and tumor markers, from a total of 90 patients (Table S1). Consistent with the results from public databases (Figure 6A), we examined the expression of ETS2 and CDKN2A in cancer and paracancerous tissues by IHC and found that CDKN2A was significantly more highly expressed in tumor tissues than paracancerous tissues, while ETS2 was more lowly expressed in tumor tissues (P valve <0.001) (Figure 6B,6C). Further, we analyzed the correlation between the clinical parameters [stage, age, lesion site, CA19-9, platelet, CA72-4, ETS2, and CDKN2A] of the clinical samples we collected and the prognosis of patients through Kaplan-Meier survival estimates (Table S1). All the parameters mentioned above were strongly associated with patient prognosis (Figure S5). ETS2 was associated with a good prognosis for patients, while CDKN2A was associated with a poor prognosis (Figure 6D). Next, we conducted a survival analysis for all patients, and the 5-year OS rate was 60.8%±5.4%. The 5-year survival rates of high and low CDKN2A expression in cancer tissue were 45.4%±9.6% and 66.4%±6.4%, respectively (P=0.03), while the 5-year survival rates of high and low ETS2 expression in the adjacent cancer tissues were 72.0%±8.5% and 55.2%±6.8%, respectively (P=0.03) (Figure 6D). Moreover, stage, age, location, and biomarkers were closely related to the prognosis of patients (Table S2). Variables with a significance level of P≤0.1 in univariate survival analysis were included in the multivariate analysis. The multivariate analysis showed that stage (HR =3.183, P=0.003), age (HR =3.636, P<0.001), CDKN2A (HR =2.070, P=0.02), CA19-9 (HR =3.237, P=0.002), and lactate dehydrogenase (LDH) (HR =2.556, P=0.06) were all independent adverse prognostic factors for OS (Table S2).
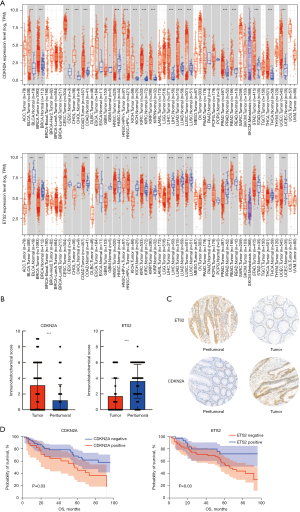
Discussion
A thorough bioinformatics analysis of senescence-related genes was conducted in this study, utilizing multiple public databases. Our results demonstrated that CRC-screened senescence-related genes were closely related to the prognosis and immunotherapy efficacy of COAD. Our key finding was that CDKN2A and ETS2 could serve as high- and low-risk senescence-related genes, respectively, to precisely predict the prognosis of COAD patients. Based on the abnormal expression levels of CDKN2A and ETS2 in COAD, Kaplan-Meier and IHC analyses were conducted, and the results showed that CDKN2A and ETS2 exhibited strong associations to various immune checkpoint genes, and their expression levels may indirectly signify the abundance of these two types of immune infiltration in the TME. Thus, CDKN2A and ETS2 appear to play a subtle role in tumor initiation or development based on differential expression profiles and, to some extent, may affect the effectiveness of immunotherapy.
With the widespread use of genetic testing in clinical work, an increasing number of biomarkers capable of predicting patient prognosis are being identified. However, there is still a lack of representative molecular markers that can be used to predict the prognosis of CRC patients and whether they can benefit from immunotherapy. Our results showed that five senescence-related genes’ signatures (i.e., CDKN2A, CXCL1, ETS2, SERPINE2, and SNAI1) were strongly associated with the prognosis of CRC patients. Of these, ETS2 and CXCL1 were considered protective genes, while CDKN2A, SERPINE1 and SNAI1 predicted a poor prognosis for CRC patients.
ETS2 plays an important role in tumors and aging and can lead to telomere lengthening and lifespan extension through the reconstitution of telomerase activity (39). Several studies indicate that ETS2 is a highly expressed oncogene and associated with the development of CRC and other cancers (30,40), however our study revealed that the high level of ETS2 in IHC was linked to a more favorable clinical outcome in CRC patients, this might be connected to differences patient treatment, therefore more larger sample sizes data or multi-center clinical data validations might be needed. We analyzed TCGA and GEO data, and our findings suggested that CXCL1 is a low-risk gene that protects against colon cancer. Conversely, Liu et al. concluded that CXCL1 promotes the development of ulcerative colitis-associated cancers (41). CDKN2A has been recognized to be a very important oncogene in tumors. Research has shown that it is involved in the process of cell senescence; however, the physiologic role of CDKN2A remains unclear (42). Dong et al. found that high level of CDKN2A is associated with poor prognosis in CRC and may guide PD-1-mediated immunotherapy (43) which is in line with our results of CDKN2A. We also found CDKN2A involved senescence-related model have a good prediction in survival prognosis and immunotherapy efficacy for CRC patients and we further validated the above findings with the clinical information from Guangzhou Medical University Affiliated Cancer Hospital. SERPINE2 plays a role in regulating radiosensitivity and the DNA damage response (DDR) in lung cancer. It directly interacts with MRE11 homolog and ATM to facilitate their phosphorylation in homologous recombination-mediated DNA double-strand break (DSB) repair and predicts a limited response to radiotherapy in non-small cell lung cancer patients. This is consistent with our results that showed that the high expression of SERPINE2 indicates a worse prognosis in colon cancer patients (44). SNAI1, like the two genes mentioned above, is classified as a high-risk gene and is negatively correlated with the prognosis of colon cancer. Like previous reports on the role of SNAI1 in tumors (45,46), we found that SNAI1 plays a cancer-promoting role in breast cancer.
Overall, the comparison of our findings with previous studies provides further evidence that high- and low-risk signatures may be used to predict the prognosis of patients with colon cancer. However, many clinical samples are still needed to verify the feasibility of this idea, as well as many in vitro and in vivo experiments to confirm the specific molecular mechanisms of the aforementioned genes.
The field of cancer treatment has been transformed by the effective use of cellular immunotherapy and vaccines (47). Among these approaches, the application of immunotherapy in colon cancer has benefited an increasing number of colon cancer patients, but this benefit is limited to less than 20% of patients with microsatellite instability-high (MSI-H) status (48). Therefore, it is particularly important to find a biomarker that can more accurately guide the application of immunotherapy for CRC. Up to now, immune-related gene expression has been identified as a prognostic indicator for immunotherapy effectiveness across different cancer types (49). Herein, we verified the association between senescence-related genes (CDKN2A, CXCL1, ETS2, SERPINE2 and SNAI1) and immune checkpoint genes, including PD-1, PD-L1 and LAG-3, in the TIMER database. According to our data, the high-risk group exhibited the most significant positive association with these immune checkpoint genes in colon cancer; moreover, we also analyzed the correlations between the five SRSs and multiple immune cells in colon cancer based on TCGA and TIMER databases. Our results showed that the above-mentioned five genes are mainly associated with macrophages, myeloid dendritic cells, natural killer cells, and neutrophils. The high-risk group showed a positive correlation with macrophages, while the low-risk group showed a negative correlation with macrophages. These findings may explain the difference in prognosis between these patients. Patients with MSI-H are more likely to benefit from immunotherapy, but the number of such cases in this study was low and therefore could not perform a prognostic analysis. Taken together, our findings suggest that the five screened senescence-related genes show a strong connection to immune-related functions and pathways in CRC.
Notably, we further investigated the expression of CDKN2A and ETS2 in CRC and their correlation with DNA mismatch repair (MMR) by IHC experiments. The Kaplan-Meier plot and logarithmic rank test results indicated that high CDKN2A expression was associated with a worse prognosis of CRC patients, while high ETS2 expression was positively associated with the prognosis of patients with CRC. To date, few studies have examined ETS2 in tumors, and only a few studies have shown that ETS2 is associated with DNA damage (29,50,51). This may be why ETS2 improves the prognosis of tumor patients (27). CDKN2A, also known as P16, is currently a very common factor in tumor research, and consistent with our findings, it has been confirmed to be a poor prognostic factor in patients with CRC due to its hypermethylation (52).
Overall, we performed a comprehensive analysis of cellular senescence-associated genes using a bioinformatics approach, revealing their important role in colon cancer prognosis and immune infiltration. However, the present study still had some limitations. First, some of our results were limited to single methods or databases, and a cross-validation of the data from multiple sources is lacking. Further, based on our analysis of data from public databases, we concluded CDKN2A and ETS2 are associated with prognosis and immune response in CRC; however, it is not yet known whether they influence prognosis by modulating immune processes. In the upcoming times, we might enhance the clinical case compilation of CDKN2A or ETS2 in various clinical facilities or larger-scale clinical data to substantiate its prognostic value in CRC patients or its significance in immunotherapy, and delve into the mechanism through cellular or animal trials.
Conclusions
Senescence-associated gene signatures, specifically CDKN2A and ETS2, could serve as biomarkers for predicting CRC prognosis and could be useful tools for guiding immunotherapy.
Acknowledgments
Funding: This study received funding from
Footnote
Reporting Checklist: The authors have completed the TRIPOD reporting checklist. Available at https://jgo.amegroups.com/article/view/10.21037/jgo-24-339/rc
Data Sharing Statement: Available at https://jgo.amegroups.com/article/view/10.21037/jgo-24-339/dss
Peer Review File: Available at https://jgo.amegroups.com/article/view/10.21037/jgo-24-339/prf
Conflicts of Interest: All authors have completed the ICMJE uniform disclosure form (available at https://jgo.amegroups.com/article/view/10.21037/jgo-24-339/coif). L.L. and H.L. are from Guangzhou Youdi Bio-technology Co., Ltd., Guangzhou, China. The other authors have no conflicts of interest to declare.
Ethical Statement: The authors are accountable for all aspects of the work in ensuring that questions related to the accuracy or integrity of any part of the work are appropriately investigated and resolved. All the participants were informed of and agreed to participate in this research study, and the study was approved by Ethics Committee of Affiliated Cancer Hospital and Institute of Guangzhou Medical University [No. GYZL-ZN-2023(041)]. The study was conducted in accordance with the Declaration of Helsinki (as revised in 2013).
Open Access Statement: This is an Open Access article distributed in accordance with the Creative Commons Attribution-NonCommercial-NoDerivs 4.0 International License (CC BY-NC-ND 4.0), which permits the non-commercial replication and distribution of the article with the strict proviso that no changes or edits are made and the original work is properly cited (including links to both the formal publication through the relevant DOI and the license). See: https://creativecommons.org/licenses/by-nc-nd/4.0/.
References
- Ciardiello F, Ciardiello D, Martini G, et al. Clinical management of metastatic colorectal cancer in the era of precision medicine. CA Cancer J Clin 2022;72:372-401. [Crossref] [PubMed]
- Biller LH, Schrag D. Diagnosis and Treatment of Metastatic Colorectal Cancer: A Review. JAMA 2021;325:669-85. [Crossref] [PubMed]
- Cui Z, Amevor FK, Zhao X, et al. Potential therapeutic effects of milk-derived exosomes on intestinal diseases. J Nanobiotechnology 2023;21:496. [Crossref] [PubMed]
- Kanani A, Veen T, Søreide K. Neoadjuvant immunotherapy in primary and metastatic colorectal cancer. Br J Surg 2021;108:1417-25. [Crossref] [PubMed]
- Overman MJ, Lonardi S, Wong KYM, et al. Durable Clinical Benefit With Nivolumab Plus Ipilimumab in DNA Mismatch Repair-Deficient/Microsatellite Instability-High Metastatic Colorectal Cancer. J Clin Oncol 2018;36:773-9. [Crossref] [PubMed]
- Overman MJ, McDermott R, Leach JL, et al. Nivolumab in patients with metastatic DNA mismatch repair-deficient or microsatellite instability-high colorectal cancer (CheckMate 142): an open-label, multicentre, phase 2 study. Lancet Oncol 2017;18:1182-91. [Crossref] [PubMed]
- André T, Shiu KK, Kim TW, et al. Pembrolizumab in Microsatellite-Instability-High Advanced Colorectal Cancer. N Engl J Med 2020;383:2207-18. [Crossref] [PubMed]
- van Deursen JM. The role of senescent cells in ageing. Nature 2014;509:439-46. [Crossref] [PubMed]
- Baker DJ, Childs BG, Durik M, et al. Naturally occurring p16(Ink4a)-positive cells shorten healthy lifespan. Nature 2016;530:184-9. [Crossref] [PubMed]
- Liu X, Hartman CL, Li L, et al. Reprogramming lipid metabolism prevents effector T cell senescence and enhances tumor immunotherapy. Sci Transl Med 2021;13:eaaz6314. [Crossref] [PubMed]
- Fassl A, Geng Y, Sicinski P. CDK4 and CDK6 kinases: From basic science to cancer therapy. Science 2022;375:eabc1495. [Crossref] [PubMed]
- Eggert T, Wolter K, Ji J, et al. Distinct Functions of Senescence-Associated Immune Responses in Liver Tumor Surveillance and Tumor Progression. Cancer Cell 2016;30:533-47. [Crossref] [PubMed]
- Cuollo L, Antonangeli F, Santoni A, et al. The Senescence-Associated Secretory Phenotype (SASP) in the Challenging Future of Cancer Therapy and Age-Related Diseases. Biology (Basel) 2020;9:485. [Crossref] [PubMed]
- Mavrogonatou E, Pratsinis H, Kletsas D. The role of senescence in cancer development. Semin Cancer Biol 2020;62:182-91. [Crossref] [PubMed]
- Liu H, Zhao H, Sun Y. Tumor microenvironment and cellular senescence: Understanding therapeutic resistance and harnessing strategies. Semin Cancer Biol 2022;86:769-81. [Crossref] [PubMed]
- Alessio N, Aprile D, Peluso G, et al. IGFBP5 is released by senescent cells and is internalized by healthy cells, promoting their senescence through interaction with retinoic receptors. Cell Commun Signal 2024;22:122. [Crossref] [PubMed]
- Lin W, Wang X, Wang Z, et al. Comprehensive Analysis Uncovers Prognostic and Immunogenic Characteristics of Cellular Senescence for Lung Adenocarcinoma. Front Cell Dev Biol 2021;9:780461. [Crossref] [PubMed]
- Garbarino O, Lambroia L, Basso G, et al. Spatial resolution of cellular senescence dynamics in human colorectal liver metastasis. Aging Cell 2023;22:e13853. [Crossref] [PubMed]
- Sørensen CE, Tritsaris K, Reibel J, et al. Elevated p16ink4a Expression in Human Labial Salivary Glands as a Potential Correlate of Cognitive Aging in Late Midlife. PLoS One 2016;11:e0152612. [Crossref] [PubMed]
- Le O, Palacio L, Bernier G, et al. INK4a/ARF Expression Impairs Neurogenesis in the Brain of Irradiated Mice. Stem Cell Reports 2018;10:1721-33. [Crossref] [PubMed]
- Bitencourt TC, Vargas JE, Silva AO, et al. Subcellular structure, heterogeneity, and plasticity of senescent cells. Aging Cell 2024;23:e14154. [Crossref] [PubMed]
- Uyar B, Palmer D, Kowald A, et al. Single-cell analyses of aging, inflammation and senescence. Ageing Res Rev 2020;64:101156. [Crossref] [PubMed]
- Conboy IM, Conboy MJ, Smythe GM, et al. Notch-mediated restoration of regenerative potential to aged muscle. Science 2003;302:1575-7. [Crossref] [PubMed]
- Zhu P, Zhang C, Gao Y, et al. The transcription factor Slug represses p16(Ink4a) and regulates murine muscle stem cell aging. Nat Commun 2019;10:2568. [Crossref] [PubMed]
- Zhu J, Yang S, Qi Y, et al. Stem cell-homing hydrogel-based miR-29b-5p delivery promotes cartilage regeneration by suppressing senescence in an osteoarthritis rat model. Sci Adv 2022;8:eabk0011. [Crossref] [PubMed]
- Varn FS, Johnson KC, Martinek J, et al. Glioma progression is shaped by genetic evolution and microenvironment interactions. Cell 2022;185:2184-2199.e16. [Crossref] [PubMed]
- Pandey S, Hajikazemi M, Zacheja T, et al. Telomerase subunit Est2 marks internal sites that are prone to accumulate DNA damage. BMC Biol 2021;19:247. [Crossref] [PubMed]
- Majercikova Z, Dibdiakova K, Gala M, et al. Different Approaches for the Profiling of Cancer Pathway-Related Genes in Glioblastoma Cells. Int J Mol Sci 2022;23:10883. [Crossref] [PubMed]
- Do PM, Varanasi L, Fan S, et al. Mutant p53 cooperates with ETS2 to promote etoposide resistance. Genes Dev 2012;26:830-45. [Crossref] [PubMed]
- Chen Y, Ying Y, Wang M, et al. A distal super-enhancer activates oncogenic ETS2 via recruiting MECOM in inflammatory bowel disease and colorectal cancer. Cell Death Dis 2023;14:8. [Crossref] [PubMed]
- Edgar R, Domrachev M, Lash AE. Gene Expression Omnibus: NCBI gene expression and hybridization array data repository. Nucleic Acids Res 2002;30:207-10. [Crossref] [PubMed]
- Tacutu R, Thornton D, Johnson E, et al. Human Ageing Genomic Resources: new and updated databases. Nucleic Acids Res 2018;46:D1083-90. [Crossref] [PubMed]
- Ritchie ME, Phipson B, Wu D, et al. limma powers differential expression analyses for RNA-sequencing and microarray studies. Nucleic Acids Res 2015;43:e47. [Crossref] [PubMed]
- Friedman J, Hastie T, Tibshirani R. Regularization Paths for Generalized Linear Models via Coordinate Descent. J Stat Softw 2010;33:1-22. [Crossref] [PubMed]
- survival. A Package for Survival Analysis in R. R package version 3.6-4 2024. Available online: https://CRAN.R-project.org/package=survival
- Kuhn M. Building Predictive Models in R Using the caret Package. Journal of Statistical Software 2008;28:1-26. [Crossref]
- Kassambara A, Kosinski M, Biecek P. survminer: Drawing Survival Curves using 'ggplot2'. Version 0.4.9, 2021. Available online: https://CRAN.R-project.org/package=survminer
- Mair P, Hofmann E, Gruber K, et al. Motivation, values, and work design as drivers of participation in the R open source project for statistical computing. Proc Natl Acad Sci U S A 2015;112:14788-92. [Crossref] [PubMed]
- Yu P, Qu N, Zhu R, et al. TERT accelerates BRAF mutant-induced thyroid cancer dedifferentiation and progression by regulating ribosome biogenesis. Sci Adv 2023;9:eadg7125. [Crossref] [PubMed]
- Ren Y, Chen B, Zhang M. Distinct Prognostic and Immunological Roles of ETS1 and ETS2: A Pan-Cancer Analysis. Biomed Res Int 2023;2023:4343350. [Crossref] [PubMed]
- Liu ZY, Zheng M, Li YM, et al. RIP3 promotes colitis-associated colorectal cancer by controlling tumor cell proliferation and CXCL1-induced immune suppression. Theranostics 2019;9:3659-73. [Crossref] [PubMed]
- Huang W, Hickson LJ, Eirin A, et al. Cellular senescence: the good, the bad and the unknown. Nat Rev Nephrol 2022;18:611-27. [Crossref] [PubMed]
- Dong Y, Zheng M, Wang X, et al. High expression of CDKN2A is associated with poor prognosis in colorectal cancer and may guide PD-1-mediated immunotherapy. BMC Cancer 2023;23:1097. [Crossref] [PubMed]
- Zhang J, Wu Q, Zhu L, et al. SERPINE2/PN-1 regulates the DNA damage response and radioresistance by activating ATM in lung cancer. Cancer Lett 2022;524:268-83. [Crossref] [PubMed]
- Xie W, Jiang Q, Wu X, et al. IKBKE phosphorylates and stabilizes Snail to promote breast cancer invasion and metastasis. Cell Death Differ 2022;29:1528-40. [Crossref] [PubMed]
- López-Menéndez C, Vázquez-Naharro A, Santos V, et al. E2A Modulates Stemness, Metastasis, and Therapeutic Resistance of Breast Cancer. Cancer Res 2021;81:4529-44. [Crossref] [PubMed]
- Ganesh K. Optimizing immunotherapy for colorectal cancer. Nat Rev Gastroenterol Hepatol 2022;19:93-4. [Crossref] [PubMed]
- Hou W, Yi C, Zhu H. Predictive biomarkers of colon cancer immunotherapy: Present and future. Front Immunol 2022;13:1032314. [Crossref] [PubMed]
- He X, Xu C. Immune checkpoint signaling and cancer immunotherapy. Cell Res 2020;30:660-9. [Crossref] [PubMed]
- Patton SE, Martin ML, Nelsen LL, et al. Activation of the ras-mitogen-activated protein kinase pathway and phosphorylation of ets-2 at position threonine 72 in human ovarian cancer cell lines. Cancer Res 1998;58:2253-9. [PubMed]
- Vainshelbaum NM, Salmina K, Gerashchenko BI, et al. Role of the Circadian Clock "Death-Loop" in the DNA Damage Response Underpinning Cancer Treatment Resistance. Cells 2022;11:880. [Crossref] [PubMed]
- Ajithkumar P, Vasantharajan SS, Pattison S, et al. Exploring Potential Epigenetic Biomarkers for Colorectal Cancer Metastasis. Int J Mol Sci 2024;25:874. [Crossref] [PubMed]