Unveiling the impact of glutathione (GSH) and p53 gene deletion on tumor cell metabolism by amino acid and proteomics analysis
Highlight box
Key findings
• The glutathione (GSH) treatment and deletion of p53 gene simultaneously triggered the down-regulation of amino acids and protein metabolism in tumor cells.
What is known and what is new?
• GSH is an important antioxidant, which can affect the changes of amino acids and proteins.
• We added experiments on the response process of p53 gene to GSH, and found that the deletion of p53 can affect the function of GSH.
What is the implication, and what should change now?
• Increasing the use of GSH may increase the viability of tumor cells, but GSH will significantly reduce the viability of tumor cells in the absence of p53. Therefore, how to use GSH to regulate the activity of tumor cells needs to clarify the gene situation of p53, and then determine whether GSH is used to inhibit the growth of tumor cells.
Introduction
Glutathione (GSH) plays an important role in many biological processes (BP). GSH maintains cellular redox homeostasis (1), contributes to iron-sulfur cluster maturation (2), acts as a signaling molecule to directly activate gene expression (3,4), and regulates cell apoptosis (5). GSH is a double-edged sword that can enhance or hinder anticancer therapy. High GSH levels in tumor cells are associated with tumor progression, increased resistance to chemotherapeutic drugs (6), and modulation to enhance anti-neoplastic therapy (7). There is an inverse relationship between patient survival and tumor GSH (8). Excess GSH promotes tumor progression and metastasis, and imparts resistance to growing cancers (9). On the other hand, GSH can be exploited to trigger the delivery of anti-cancer drugs to cancer cells, which has shown promising results in ovarian cancer treatment. As GSH is an important cysteine storage, targeting this amino acid’s uptake by cancer cells could be another promising strategy to fight cancer (10).
p53 plays a key role in tumor suppression. The tumor suppressive function of p53 has long been attributed to its ability to induce apoptosis, cell cycle arrest, and senescence in cells. p53 and its metabolic pathways have important roles in tissue homeostasis, metabolic diseases and cancer (11). The tumor suppressive function of p53 has long been attributed to its ability to induce apoptosis, cell cycle arrest, and senescence in cells. p53 regulates various metabolic pathways to maintain the metabolic homeostasis of cells and their response to stress (12). An array of p53 mediated cellular outcomes have been characterized including cell cycle arrest, apoptosis, DNA repair, senescence, angiogenesis, cellular metabolism, reactive oxygen species (ROS), autophagy, cuproptosis (13) and ferroptosis (14,15). The mutant-p53 plays a role in promoting metastasis and is the principal cause of cancer-related death, which makes p53 an attractive target for possible therapeutic applications (16). p53 is closely related to ROS in tumorigenesis and suppression, but the relationship between p53 and antioxidants needs to be further studied. Expression of GSH peroxidase 3 (GPx3) is down-regulated in a variety of human malignancies. p53-induced gene 3 mediates cell death induced by GPx3 (17). Deletion of p53 leads to loss of the cellular antioxidant system, which result in elevated ROS levels and consequently tumorigenesis. p53 increases the expression of superoxide dismutase 2 (SOD2) and GSH peroxidase 1 (GPX1) and the production of NADPH, which further regulates glycolysis and apoptosis (6,18). Accumulated mutant-p53 protein inhibits the expression of solute carrier family 7 member 11 (SLC7A11) by binding to the master antioxidant transcription factor NRF2 and reduces GSH synthesis, which makes mutant-p53 tumors susceptible to oxidative damage leading to apoptosis in mutant-p53 tumor cells (19). p53 regulates ROS and metabolism, and the fact that metabolic disturbances and ROS accumulation are characteristics of carcinogenesis supports the idea that many of the tumor-suppressive effects of p53 can be mediated through regulation of metabolism and ROS (20). RASA2 negatively regulates p53 in cancer cells and therefore promotes radioresistance (21). Thus, more genes and proteins providing a new predictive biomarker and a potential therapeutic target for radioresistance.
Past studies have provided many results on the relationship between p53 and ROS (22-34), but studies of amino acid as well as protein metabolism have not been reported, with certain gaps in knowledge remain largely unexplored. How GSH regulates the growth and antioxidant process of tumor cells, and the changes of amino acids and proteins in them are still unclear. In this study, we knocked out the TP53 gene and experimented with cells before and after GSH treatment. We conducted cytological experiments, amino acid metabolic profiling and proteomic assays, and analyses showed that the overall level of amino acids decreased, with 41 proteins showing significant differences in all treatments, and a good correlation between amino acids and proteins was found. This study provides direct evidence for our understanding of the role of p53 in influencing ROS during tumor formation, demonstrating that GSH can increase the antioxidant capacity of cells by acting through p53, thereby altering tumorigenesis and reducing tumor cell viability. Therefore, GSH can be used as a tumor therapeutic drug acting through p53. We present this article in accordance with the MDAR reporting checklist (available at https://jgo.amegroups.com/article/view/10.21037/jgo-24-236/rc).
Methods
Cell lines and culture
SW480, a colorectal cancer (CRC) cell line was purchased from Suzhou Haixing Biosciences in Suzhou, China. These cellular entities flourished in a nutrient-rich DMEM medium, infused with 12.5 mM glucose and 4 mM glutamine, and fortified with 5% fetal bovine serum (Lot 10270, Gibco, Thermo Fisher Scientific, Waltham, MA, USA) and a safeguarding 1% streptomycin/penicillin blend. The environment was meticulously maintained at 37 ℃, with a gentle 5% CO2 atmosphere to their growth.
CRISPR/Cas9 knock-out (KO) TP53 gene
Harnessing the precision of CRISPR/Cas9 technology, we embarked on a mission to knockout the p53 gene (gene ID: 7157) within the SW480 cells (35). For this endeavor, we selected the pX330 p53 vector (Addgene, plasmid #42230), a tool renowned for its prowess in targeting the human mutant-p53 (35). The SW480 cells were then subjected to a transfection process, where they were introduced to the pX330 vector equipped with a sgRNA designed to specifically hone in on human p53, facilitated by the FugeneHD reagent (Promega, USA). The sgRNA sequence, a carefully crafted 5'-CTTCCCACAGGTCTCTGCTA-3', was the key to this genetic modification. Post-transfection, after a 48-hour incubation period, the cells were meticulously isolated and seeded onto 96 well plates. As these cells proliferated and formed colonies, we conducted a thorough examination of the p53 expression levels. Employing polymerase chain reaction (PCR), gel electrophoresis, and sequencing, we meticulously assessed the success of our gene knockout strategy.
3-(4,5-dimethyl-2-thiazolyl)-2,5-diphenyl-2-H-tetrazolium bromide (MTT) assay
In a meticulously orchestrated experiment, a cohort of 5×103 cells was sown into each well of a 96-well plate. These cells were nurtured in an incubator set to a steady 37 ℃, enveloped in a 5% CO2 atmosphere, for a period of 48 hours to allow them to settle and adhere. Upon reaching the halfway mark of the incubation, the medium was gently decanted, making way for a new solution. The cells were then bathed in 100 µL of a 3-(4,5-dimethylthiazol-2-yl)-2,5-diphenyl tetrazolium bromide (MTT, Sigma, USA) solution, with a concentration of 5 mg/mL, for a duration of 2 to 4 hours. This solution, a staple in the world of cell viability assays, was chosen for its ability to stain living cells. After the MTT solution was discarded, 100 µL of dimethyl sulfoxide (DMSO) (Invitrogen, Carlsbad, USA) was introduced to each well. This was followed by a gentle mixing process for 10 minutes, allowing the DMSO to dissolve the formazan crystals formed by the metabolically active cells. Three replicates per condition were detected for absorbance at 570 nm using a microplate reader (Synergy H1, BioTek, USA). This step was crucial in quantifying the amount of formazan product, which was directly proportional to the number of living cells. The data presented were an amalgamation of the average values derived from three to five distinct experiments.
Detection of amino acids by liquid chromatography-mass spectrometry/mass spectrometry (LC-MS/MS)
Amino acids were quantified by LC-MS/MS as described previously (36) with minor modifications to run on ultra performance liquid chromatography (UPLC) coupled to a mass spectrometer. Cell samples were washed twice with cold phosphate buffer saline (PBS), followed by bead-beating in 80% methanol: water (LC-MS grade methanol, Fisher Scientific, Carlsbad, USA) at −20 ℃; 100 µL of sulfosalicylic acid solution containing isotope internal standard was added into a centrifuge tube containing cells. Following which, vortex sample for 3 min, then centrifuge at 14,000 rpm for 10 min. Transfer 70 µL of boric acid buffer, 10 µL of supernatant and 20 µL of AccQ (Waters, USA) solution into a 96-well sample receiving plate and shake for 1 min. To analyze sample, 20 µL of the above mixed solution was isolated and mixed gently with 180 µL of water for 1 min. The mixed solution was transferred to an autosampler vial, and 10 µL of the mixture was then used for UPLC-MS/MS analysis (UPLC I Class, XEVO TQS, Waters, USA). The separation was performed on a BEH C18 column (130 Å, 1.7 µm, 1 mm × 100 mm, 1/pkg, Waters, USA). Mobile phases consisted of: (A) 100% water, containing 0.1% formic acid (FA) and (B) 100% acetonitrile. The following gradient was applied: 0–0.1 min, 1% B; 0.1–3.5 min, to 20% B; 3.5–4.0 min, 99% B; 4.0–4.5 min, 98% B; 4.5–4.6 min, 1% B; 4.6–5.5 min, 1% B. The flow rate was 0.45 mL/min. Mass spectrometer was operated in the positive-ion mode using the following settings: capillary voltage of 2.0 kV, source temperature of 150 ℃, desolvation temperature of 500 ℃, cone gas flow rate of 150 L/h and desolvation gas flow rate of 1,000 L/h. The scan dwell time was set at 0.2 s for both the analytes. The optimized multi-reaction monitoring (MRM) ion source parameters are shown in Table 1.
Table 1
Compound | Full name | MRM (m/z) | Cone voltage (V) | Collision energy (V) |
---|---|---|---|---|
Orn | Ornithine | 237.1→171.1 | 30 | 15 |
Lys | Lysine | 244.1→171.1 | 30 | 15 |
Gly | Glycine | 246.2→171.1 | 30 | 20 |
Ala | Alanine | 260.2→171.1 | 30 | 20 |
Ser | Serine | 276.2→171.1 | 30 | 20 |
Pro | Proline | 286.2→171.1 | 30 | 20 |
Val | Valine | 288.2→171.1 | 30 | 15 |
Thr | Threonine | 290.2→171.1 | 30 | 20 |
Leu | Leucine | 302.2→171.1 | 30 | 20 |
lle | Isoleucine | 302.2→171.1 | 30 | 20 |
Asn | Asparagine | 303.2→171.1 | 30 | 20 |
Asp | Aspartic acid | 304.2→171.1 | 30 | 20 |
Glu | Glutamine | 318.2→171.1 | 30 | 20 |
Met | Methionine | 320.2→171.1 | 30 | 20 |
His | Histidine | 326.2→171.1 | 30 | 20 |
Phe | Phenylalanine | 336.2→171.1 | 30 | 20 |
Arg | Arginine | 345.2→171.1 | 30 | 20 |
Cit | Citrulline | 346.2→171.1 | 30 | 20 |
Tyr | Tyrosine | 352.2→171.1 | 30 | 20 |
Trp | Tryptophane | 375.2→171.1 | 30 | 20 |
MRM, multi-reaction monitoring.
Proteomics experiment and analysis
In the wake of GSH treatment, the cells were meticulously gathered and preserved at a frigid −80 ℃, post homogenization and centrifugation processes. The Bradford assay kit was employed to detect the protein concentrations, a method as reliable as it is precise. A precise 20 µg of protein was then carefully deposited into a collection tube, accompanied by an addition of trypsin at a meticulously calculated ratio of 50:1 (protein:trypsin). This concoction was then left to incubate at a steady 37 ℃ for a full 16 hours, allowing the trypsin to meticulously digest the proteins. Post-digestion, the peptides were subjected to a vacuum drying process, followed by dissolution in 0.1% trifluoroacetic acid (TFA). They were then passed through C18 cartridges (Empore SPE Cartridges C18, standard density) to remove any unwanted salts, a purification ritual performed under the watchful eye of vacuum centrifugation. The purified peptides were then reconstituted in FA, ready for the next phase of analysis.
The reconstituted peptides were then ushered into the analytical chamber of a Q-Exactive mass spectrometer (Thermo Fisher Scientific, Waltham, MA, USA), which was coupled with a nano high-performance liquid chromatography (UltiMate 3000 LC Dionex; Thermo Fisher Scientific) system. A harmonious duo, they provided a detailed profile of the peptides (37,38). For protein identification, MaxQuant (1.6.17) was summoned, scouring the reviewed FASTA database in UniProt with Homo sapiens as the organism of interest (39,40). The search parameters were meticulously set: peptide mass tolerance = ±15 ppm, MS/MS tolerance =0.02 Da, enzyme = trypsin, missed cleavage =2, fixed modification: carbamidomethyl (C), variable modification: oxidation (M), database pattern = decoy. The false discovery rate (FDR) for peptides and proteins was stringently set to 0.01. The protein expression data were then artfully presented in a heatmap, a visual tapestry of expression levels. The differentially expressed proteins (DEPs) between groups were identified as significantly upregulated or downregulated, based on a fold change (FC) ≥1.5 and P value <0.05 for upregulation, or a FC ≤0.667 and P value <0.05 for downregulation (experimental group/control group). For a deeper dive into the biological significance of these proteins, Metascape, a web-based resource, was employed to conduct Gene Ontology (GO) analysis. The Kyoto Encyclopedia of Genes and Genomes (KEGG) Orthology-Based Annotation System (KOBAS, http://bioinfo.org/kobas) online analysis tool was also utilized to perform KEGG pathway analyses, a method as thorough as it is insightful. Database enrichment analysis was performed using the UniProtKB database (Release 2016 10), a treasure trove of protein information. GO enrichment included three ontologies [BP, molecular function (MF), and cellular component (CC)], providing a comprehensive view of the BP, functions, and locations of the proteins. To further unravel the intricate web of protein-protein interactions (PPIs), STRING software was employed, followed by an import into Cytoscape software. This allowed for a detailed analysis of functional PPI networks, a map of the complex interplay between proteins.
Data processing, statistical analysis and drawing
The two-tailed Student’s t-test was used for statistical analyses. All data were expressed as the mean ± standard error of mean (SEM), and a P value <0.05 was considered to indicate statistical significance. Histograms were plotted using Graphpad prism v8.8. Venn (http://jvenn.toulouse.inra.fr/app/example.html) was used to create Venn diagrams. The correlation analysis was performed using Pearson correlation via the R language.
Results
GSH treatment significantly reduced the cell viability of KO p53 gene
After GSH treatment, the cell viability of the control cells increased with increasing concentration, but a substantial decrease was observed when the concentration reached 20 mM. Cell imaging results revealed conspicuous variations in cell morphology, with an appreciable increase in viability for the control cells, but a noticeable decrease for the KO cells (Figure 1A-1D). In contrast, the cell viability of KO p53 cells significantly declined following GSH treatment, with stability observed in the range of 1–10 mM, yet a marked decrease was evident under 20 and 50 mM treatments. Notably, at a GSH concentration of 10 mM, the most pronounced disparity in viability was observed between the control and KO cells (Figure 1E). Consequently, both groups of cells were subjected to individual treatment with 10 mM GSH. These findings underscore how GSH’s impact on cell viability depends on p53 gene expression. In cases where the p53 gene is present, cell viability was better maintained under the treatment of 10 mM GSH, resulting in a significant improvement in cell viability. Conversely, in the absence of the p53 gene, cell viability experienced a clear reduction under the treatment of 10 mM GSH (Figure 1F), as seen by not only a reduction below that of untreated cells but also registering a substantial decrease compared to GSH-treated control cells.
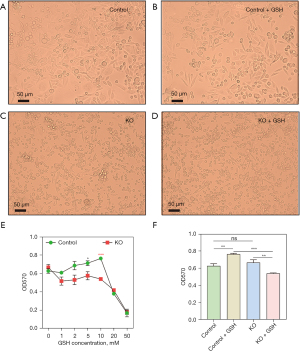
Principal component analysis (PCA) and orthogonal partial least squares discriminant analysis (OPLSDA) analysis of amino acid showed that there was a significant overall difference between control and KO cells
We employed LC-MS/MS to examine the profiles of twenty distinct amino acids found in cellular samples. Subsequently, PCA was conducted on these amino acid profiles (Figure 2A). Notably, a discernible separation emerged among the control cells following GSH treatment, indicating observable variations. In the case of the KO cells, GSH treatment also induced some separation, albeit not as distinct as that observed in the control group. A comparative evaluation of the PCA scores revealed a conspicuous divergence between the control and KO cells, suggesting the existence of significant overall differences between GSH-treated control and KO samples. To further scrutinize these distinctions, we employed an OPLSDA model to evaluate the amino acid profiles (Figure 2B). The analysis revealed a robust separation among all four groups, demonstrating the clear distinguish ability of these samples through supervised analysis. These results underscore the pronounced overall differences between GSH-treated control and KO samples.
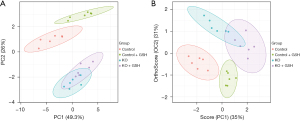
Volcanic diagram analysis of amino acids
Analysis of the volcano plot revealed distinct amino acid distribution patterns. Notably, regardless of the comparison made, there was a consistent reduction in amino acids. The results demonstrate a significant decrease in amino acids following treatment with GSH, as well as a comparable reduction after KO treatment. In a comparison with control cells, the KO cells exhibit alterations in six amino acids, specifically taurine (Tau), methionine (Met), aspartic acid (Asp), hydroproline (Hyp), citrulline (CIT) and Lysine (Lys) (Figure 3A). After GSH treatment, the volcano plot for control cells depicts differences in four amino acids: CIT, HYP, Valine (Val) and Lys (Figure 3B). Meanwhile, KO cells, post-GSH treatment, display an alteration in the amino acid Met (Figure 3C). Furthermore, when comparing KO cells after GSH treatment to control cells under the same conditions, we observed variations in three metabolites: Met, Asp and Tau (Figure 3D). An analysis of the amino acid disparities highlights that KO treatment exerts the most significant influence on cellular amino acids, resulting in alterations in six different amino acids. In contrast, KO cells exhibit the least variation following GSH treatment, with only alterations in a single amino acid. Notably, control cells, post-GSH treatment, display differences in four amino acids. Moreover, the distinctions in KO cells after GSH treatment are entirely unique, suggesting that KO cells exhibit reduced sensitivity to GSH, and their response to GSH differs markedly from that of control cells. This phenomenon may be attributed to the deletion of the p53 gene.
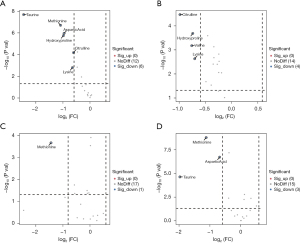
Amino acid analysis of common differences
Figure 4A presents the results of the Venn analysis, which revealed how two specific amino acids exhibited significant differences in all four comparisons. These amino acids were identified as Met, as shown in Figure 4B, and phenylalanine (Phe), depicted in Figure 4C. In Figure 4B, we observe a notable decrease in Met content within KO cells following GSH treatment. The Met content within KO cells post-GSH treatment significantly lags behind that of control cells. Notably, Figure 4C highlights a consistent trend in Phe levels, indicating a decrease in both KO cells and control cells after GSH treatment. However, the decline in Phe content is more pronounced in KO cells, with a reduction of 54% compared to 20% in control cells. This collective evidence suggests that the reductions in Met and Phe are significantly associated with the p53 gene deletion and are exacerbated following GSH treatment, potentially representing an effective response following p53 gene deletion.
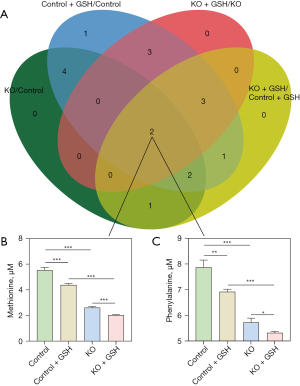
PCA and OPLSDA analysis of proteomics showed that there was a significant overall difference between control and KO cells
In our study, we employed the proteomics method to detect the proteins within cells, which ultimately identified and quantified a total of 1,089 proteins. Subsequently, we conducted PCA as depicted in Figure 5A, and OPLSDA as presented in Figure 5B to analyze this dataset. The results of these analyses were striking, as they demonstrated a clear demarcation among the four distinct groups. This clear separation underscores the substantial protein alterations occurring both before and after p53 gene KO and before and after treatment with GSH. These findings underscore the pervasive and significant changes in protein expression patterns associated with these critical experimental conditions.
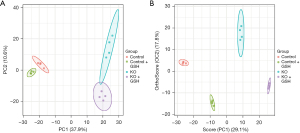
Volcanic diagram analysis of proteomics
Through our analysis of quantitative data on 1,089 proteins, the volcano plots reveal an insightful perspective on both the number and directional trends of distinct proteins. In comparison to control cells, the KO cells exhibit noteworthy differences. Specifically, 59 proteins displayed significant increases, while 48 proteins exhibited significant decreases (Figure 6A). Meanwhile, in control cells subjected to GSH treatment, we identified a substantial shift, with 168 proteins showing a significant increase and 82 proteins displaying significant decreases (Figure 6B). In GSH-treated KO cells, the protein dynamics exhibited an even more dramatic transformation, with 382 proteins showing significant increases and 332 proteins revealing significant decreases (Figure 6C). The extent of protein alterations in GSH-treated KO cells considerably surpassed that in GSH-treated control cells. This significant difference is exemplified by the count of proteins that increased, with GSH-treated KO cells showcasing 382 increases (Figure 6C), a stark contrast to GSH-treated control cells with 168 increases (Figure 6B). Moreover, the decreases are similarly pronounced, with GSH-treated KO cells revealing 332 decreased proteins (Figure 6C) compared to 82 in GSH-treated control cells (Figure 6B). Comparatively, the effect of GSH treatment on KO cells, in contrast to control cells, is more pronounced. This shift is especially evident when comparing the number of differential proteins, which rose from 59 (Figure 6A) to 426 (Figure 6D) in KO cells after GSH treatment. In the case of control cells after GSH treatment, the count increased from 48 (Figure 6A) to 312 (Figure 6D). These findings indicate that KO cells exhibit heightened sensitivity to GSH, which, in turn, significantly amplifies the influence of p53 gene deletion. This heightened sensitivity correlates with an increased capacity for cells to mitigate ROS levels in their environment, suggesting a direct consequence of p53 gene deletion.
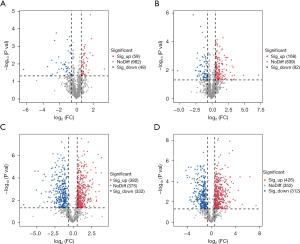
Protein analysis of common trend differences
The Venn analysis presented in Figure 7A, which compared four sets of differential proteins, revealed that a common set of 41 proteins is shared among them. These 41 proteins can be effectively classified and delineated based on their expression profiles through thermal cluster analysis, as demonstrated in Figure 7B. Furthermore, a trend analysis of these 41 common proteins indicated that eight of them exhibit similar directional changes in response to KO and GSH treatment. Specifically, these eight proteins either decreased after KO and GSH treatment or increased in expression following both interventions. These consistent trends are visually represented in Figure 7C-7J. Among these eight proteins, five demonstrated a substantial decrease in expression, including C1QBP (Figure 7C), CALCOCO2 (Figure 7D), KRT18 (Figure 7F), PDS5B (Figure 7H), and TOM1L1 (Figure 7J). Conversely, the remaining three proteins, KIF4A (Figure 7E), PABPC4 (Figure 7G), and PSMC2 (Figure 7I), exhibited a notable increase in expression levels following KO and GSH treatment. These findings offer valuable insights into the specific proteins that may play a significant role in the observed changes associated with KO and GSH treatment.
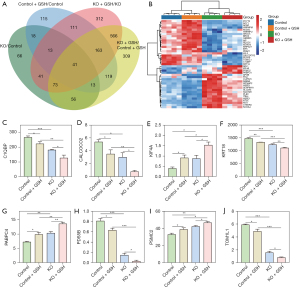
Functional analysis of proteomics
We conducted an in-depth analysis of the 41 proteins exhibiting consistent differences (identical proteins identified in the Venn analysis above). This comprehensive analysis encompassed GO analysis, KEGG analysis, Reactome analysis, and a protein interaction study. The GO analysis highlighted the primary functions of these differential proteins, which encompassed CC, cellular processes, cellular anatomical entities, and BP (Figure 8A). In the KEGG analysis, the primary functions of these proteins were associated with various pathways, such as metabolic pathways, spliceosome, spinocerebellar ataxia, shigellosis, and RNA degradation (Figure 8B). The Reactome analysis revealed the predominant roles of these differential proteins in the immune system, metabolism, and signal transduction processes (Figure 8C). Furthermore, the protein interaction analysis demonstrated strong interactions among these proteins, particularly among proteins such as HSPE1, HSPD1, C1QBP, SQSTM1, CALCOCO2, HNRNPU, XRCC5, HNRNPM, which were found to interact with three or more proteins (Figure 8D).
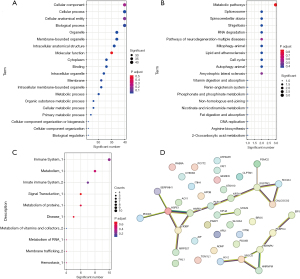
Correlation analysis of differential amino acids and differential proteins
We conducted a comprehensive analysis of the correlation between two distinct amino acids and eight proteins that exhibited a consistent trend (Figure 9A). Our investigation revealed a strong correlation between these amino acids and six proteins (Figure 9B). Importantly, all correlation coefficients exceeded 0.6. Specifically, Met and Phe exhibited robust positive correlations with the protein CALCOCO2, with correlation coefficients of 0.74 and 0.76, respectively. Similarly, Met and Phe displayed significant positive correlations with the protein KRT18, with correlation coefficients of 0.72 and 0.74, respectively. Conversely, Met and Phe demonstrated notable negative correlations with the proteins PABPC4, with correlation coefficients of −0.75 and −0.71, respectively, as well as KIF4A, with correlation coefficients of −0.74 and −0.76, respectively. This correlation analysis provides compelling evidence of both positive and negative correlations between amino acids and proteins, shedding new light on the functional implications of p53 under different GSH conditions. Moreover, it provides a deeper understanding of how amino acid metabolism influences protein synthesis and degradation, ultimately impacting protein functionality.
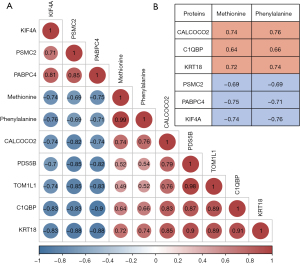
Discussion
GSH emerges as a central player in a myriad of cellular processes, ranging from cell differentiation to proliferation and apoptosis. The intricate equilibrium of GSH levels within the body significantly influences the onset and progression of various human diseases, most notably cancer. While a depletion of GSH or a drop in the GSH/GSH disulfide (GSSG) ratio amplifies susceptibility to oxidative stress, a phenomenon intricately linked to cancer development, increased GSH levels bolster a cell’s antioxidant capacity and enhance resistance to oxidative stress. This resistance is prominently observed across various cancer cell types (41). The GSH antioxidant pathway assumes pivotal importance in the context of cancer, with numerous tumors exhibiting heightened levels of the free radical scavenger, GSH (42). It is reasonable to posit that GSH plays a critical role in both cell proliferation and tumor resistance, exemplified by the elevation of GSH levels in pancreatic cancer, notably within the AsPC-1 cell line (43). GSH, functioning as an endogenously produced non-enzymatic antioxidant, plays a pivotal role in regulating intracellular redox-sensitive signal transduction (44), further establishing its significance as a marker for various human diseases (45).
p53 is a crucial tumor suppressor that regulates diverse cellular responses to protect against cancer development. p53 signaling through alterations in p53 regulators or mutations is observed in human colorectal carcinoma, with TP53 mutations occurring in 43% of tumors and compromised p53 functioning commonly found in the remaining tumors due to genetic modifications in proteins involved in p53 regulation (46). Mutations in the p53 gene significantly influence patient prognosis and can inform the application of targeted therapeutic strategies, including immunotherapy, and impact the intrinsic biology of the cancer cells and the surrounding tumor microenvironment (47). p53 serves to upregulate the expression of SLC1A3, an aspartate/glutamate transporter that facilitates the utilization of aspartate to sustain cellular functions when extracellular glutamine is limited. This was investigated in HCT116 isogenic cell lines, where the knockout of p53 (KO) resulted in a notable decline in cellular amino acid levels. Specifically, glutamate, glutamine, aspartate, citrate, α-KG, malate, and fumarate displayed reduced concentrations (48). These outcomes align with our research findings. Upon p53 knockout, a comprehensive reduction in amino acid levels was observed (Figure 3). Of note, both Met and Phe exhibited marked and consistent decreases across all treatment conditions (Figure 4). p53, functioning as a central hub in cellular redox regulation, emerges as a therapeutic target in the realm of cancer (49). p53 governs cellular energy metabolism and orchestrates antioxidant defense mechanisms. Notably, one of its key effectors, glutaminase 2 (GLS2), emerges as a significant player in cellular antioxidant defense. It elevates reduced GSH levels while simultaneously quelling ROS levels. This dual action serves as a bulwark against oxidative stress-induced apoptosis, such as that induced by H2O2 (50). In addition, the accumulated mutant-p53 protein exerts a repressive influence by dampening the expression of SLC7A11, a pivotal component of the cystine/glutamate antiporter, system xC. This occurs through direct binding to the master antioxidant transcription factor NRF2, culminating in reduced GSH synthesis. Consequently, tumor cells bearing mutant-p53 exhibit heightened vulnerability to oxidative damage, a vulnerability that can be exploited by inhibitors targeting system xC, selectively eliminating cancer cells harboring stabilized mutant-p53 proteins. This dual strategy depletes the GSH pool in mutant-p53 tumors, leading to the substantial accumulation of ROS and widespread cell death (19). Furthermore, p53 emerges as a mediator of its tumor suppressor function through ROS regulation. In cases of p53 loss-of-function mutation, a hallmark event in over 50% of human cancers, ROS accumulation ensues, facilitating pro-tumorigenic signaling and cellular transformation (51). Dietary supplementation of the antioxidant N-acetyl cysteine (NAC) has shown efficacy in reducing tumor growth within a p53-deficient mouse model (33).
In our final analysis, we have identified four proteins that exhibit a correlation with specific amino acids (correlation coefficient exceeding 0.7). These proteins are likely to exert a significant influence on p53’s response to GSH stimulation. The identified proteins include CALCOCO2, KRT18, PABPC4, and KIF4A, all of which are closely associated with the development of cancer. CALCOCO2 as an oncogene in prostate cancer (PCa), where its knockdown inhibits cell proliferation, enhances apoptosis, modulates cyclin-E1 and p53 expression, and is linked to autophagosome assembly and nucleic acid metabolism, suggesting its potential as a diagnostic and therapeutic target in PCa (52). Keratin 18 (KRT18) has been suggested to be overexpressed in most types of human tumor, KRT18 protein expression was markedly increased in CRC cancer tissues and cell lines, high KRT18 expression was associated with advanced clinical stage, deep tumor invasion, lymph node metastasis, distant metastasis, poor differentiation and unfavorable prognosis in CRC patients (53). PABPC4 (cytoplasmic poly(A) binding protein 4) is an RNA-processing protein that plays an important role in the regulation of gene expression, and highly expressed in human CRC and correlates with better prognosis (54). p53 signaling pathway related KIF4A could inhibit the progression of colon cancer through inhibiting proliferation as well as migration of the cancer cell and promoting apoptosis of cancer cell (55). KIF4A was found to be upregulated in patients with CRC and downregulation of KIF4A reduced cell proliferation in CRC cells. KIF4A may be a potential therapeutic target, which may improve the outcomes of patients with CRC (56).
In our proteomics study, a marked shift in protein profiles emerged following p53 knockout and subsequent GSH treatment. These alterations spanned a diverse spectrum of proteins, with a significant subset exhibiting both increased and decreased abundance. Notably, GSH application accentuated protein expression variations, underscoring its substantial influence. This heightened protein responsiveness to GSH implies its increased sensitivity to the intricate interplay between these molecules. Such heightened sensitivity may be ascribed to the reduction in oxidative stress levels, thereby provoking robust adaptive responses from proteins to sustain cellular equilibrium.
Conclusions
This is a key result in the influence of GSH on protein after p53 knockout, specifically SW480 cell lines. It was revealed that GSH treatment induces profound alterations in amino acid and protein profiles within the cellular genome. These intriguing observations have unveiled novel phenomena, shedding light on the intricate interplay between GSH and the cellular proteome. The deletion of the p53 gene exerts a profound influence on tumor cell proliferation. Moreover, tumor cell proliferation is substantially impacted by elevated GSH levels. The combined treatment of GSH and p53 gene deletion significantly accelerates tumor cell death. Notably, this treatment results in a substantial decline in amino acid metabolism, with a particular down-regulation of amino acids Met and Phe. Furthermore, GSH treatment in conjunction with p53 gene deletion induces substantial alterations in the protein profiles of tumor cells. Among the 41 significantly changed proteins, eight exhibit consistent changes, with five experiencing a reduction and three showing an increase. These proteins primarily participate in crucial cellular metabolic processes and immune functions. In summary, the concurrent application of GSH treatment and p53 gene deletion leads to remarkable modifications in the amino acid and protein metabolism of tumor cells, primarily involving metabolic down-regulation. This change leads to compromised cellular metabolic activities and immune functions, ultimately driving tumor cell death. These novel findings hold significant promise and may pave the way for the development of simple and effective approaches for anticancer treatments.
Acknowledgments
Funding: This study was supported by
Footnote
Reporting Checklist: The authors have completed the MDAR reporting checklist. Available at https://jgo.amegroups.com/article/view/10.21037/jgo-24-236/rc
Data Sharing Statement: Available at https://jgo.amegroups.com/article/view/10.21037/jgo-24-236/dss
Peer Review File: Available at https://jgo.amegroups.com/article/view/10.21037/jgo-24-236/prf
Conflicts of Interest: All authors have completed the ICMJE uniform disclosure form (available at https://jgo.amegroups.com/article/view/10.21037/jgo-24-236/coif). The authors have no conflicts of interest to declare.
Ethical Statement: The authors are accountable for all aspects of the work in ensuring that questions related to the accuracy or integrity of any part of the work are appropriately investigated and resolved.
Open Access Statement: This is an Open Access article distributed in accordance with the Creative Commons Attribution-NonCommercial-NoDerivs 4.0 International License (CC BY-NC-ND 4.0), which permits the non-commercial replication and distribution of the article with the strict proviso that no changes or edits are made and the original work is properly cited (including links to both the formal publication through the relevant DOI and the license). See: https://creativecommons.org/licenses/by-nc-nd/4.0/.
References
- Kannan N, Nguyen LV, Makarem M, et al. Glutathione-dependent and -independent oxidative stress-control mechanisms distinguish normal human mammary epithelial cell subsets. Proc Natl Acad Sci U S A 2014;111:7789-94. [Crossref] [PubMed]
- Kumar C, Igbaria A, D'Autreaux B, et al. Glutathione revisited: a vital function in iron metabolism and ancillary role in thiol-redox control. EMBO J 2011;30:2044-56. [Crossref] [PubMed]
- Fratelli M, Goodwin LO, Ørom UA, et al. Gene expression profiling reveals a signaling role of glutathione in redox regulation. Proc Natl Acad Sci U S A 2005;102:13998-4003. [Crossref] [PubMed]
- Reniere ML, Whiteley AT, Hamilton KL, et al. Glutathione activates virulence gene expression of an intracellular pathogen. Nature 2015;517:170-3. [Crossref] [PubMed]
- Franco R, Cidlowski JA. Apoptosis and glutathione: beyond an antioxidant. Cell Death Differ 2009;16:1303-14. [Crossref] [PubMed]
- Kennedy L, Sandhu JK, Harper ME, et al. Role of Glutathione in Cancer: From Mechanisms to Therapies. Biomolecules 2020;10:1429. [Crossref] [PubMed]
- Balendiran GK, Dabur R, Fraser D. The role of glutathione in cancer. Cell Biochem Funct 2004;22:343-52. [Crossref] [PubMed]
- Gamcsik MP, Kasibhatla MS, Teeter SD, et al. Glutathione levels in human tumors. Biomarkers 2012;17:671-91. [Crossref] [PubMed]
- Bansal A, Simon MC. Glutathione metabolism in cancer progression and treatment resistance. J Cell Biol 2018;217:2291-8. [Crossref] [PubMed]
- Nunes SC, Serpa J. Glutathione in Ovarian Cancer: A Double-Edged Sword. Int J Mol Sci 2018;19:1882. [Crossref] [PubMed]
- Lahalle A, Lacroix M, De Blasio C, et al. The p53 Pathway and Metabolism: The Tree That Hides the Forest. Cancers (Basel) 2021;13:133. [Crossref] [PubMed]
- Liu J, Zhang C, Hu W, et al. Tumor suppressor p53 and metabolism. J Mol Cell Biol 2019;11:284-92. [Crossref] [PubMed]
- Xiong C, Ling H, Hao Q, et al. Cuproptosis: p53-regulated metabolic cell death? Cell Death Differ 2023;30:876-84. [Crossref] [PubMed]
- Hernández Borrero LJ, El-Deiry WS. Tumor suppressor p53: Biology, signaling pathways, and therapeutic targeting. Biochim Biophys Acta Rev Cancer 2021;1876:188556. [Crossref] [PubMed]
- Xu R, Wang W, Zhang W. Ferroptosis and the bidirectional regulatory factor p53. Cell Death Discov 2023;9:197. [Crossref] [PubMed]
- Muller PA, Vousden KH. p53 mutations in cancer. Nat Cell Biol 2013;15:2-8. [Crossref] [PubMed]
- Wang H, Luo K, Tan LZ, et al. p53-induced gene 3 mediates cell death induced by glutathione peroxidase 3. J Biol Chem 2012;287:16890-902. [Crossref] [PubMed]
- Bensaad K, Tsuruta A, Selak MA, et al. TIGAR, a p53-inducible regulator of glycolysis and apoptosis. Cell 2006;126:107-20. [Crossref] [PubMed]
- Liu DS, Duong CP, Haupt S, et al. Inhibiting the system xC-/glutathione axis selectively targets cancers with mutant-p53 accumulation. Nat Commun 2017;8:14844. [Crossref] [PubMed]
- Budanov AV. The Role of Tumor Suppressor p53 in the Antioxidant Defense and Metabolism. In: Deb SP, Deb S (eds) Mutant p53 and MDM2 in Cancer. Dordrecht: Springer Netherlands, pp. 337-58.
- Li J, Zong Y, Tuo Z, et al. The role of RASA2 in predicting radioresistance in lung cancer through regulation of p53. Transl Lung Cancer Res 2024;13:587-602. [Crossref] [PubMed]
- He Z, Simon HU. A novel link between p53 and ROS. Cell Cycle 2013;12:201-2. [Crossref] [PubMed]
- Cao J, Liu X, Yang Y, et al. Decylubiquinone suppresses breast cancer growth and metastasis by inhibiting angiogenesis via the ROS/p53/ BAI1 signaling pathway. Angiogenesis 2020;23:325-38. [Crossref] [PubMed]
- Shi T, van Soest DMK, Polderman PE, et al. DNA damage and oxidant stress activate p53 through differential upstream signaling pathways. Free Radic Biol Med 2021;172:298-311. [Crossref] [PubMed]
- Jiang L, Hickman JH, Wang SJ, et al. Dynamic roles of p53-mediated metabolic activities in ROS-induced stress responses. Cell Cycle 2015;14:2881-5. [Crossref] [PubMed]
- Cordani M, Butera G, Pacchiana R, et al. Mutant p53-Associated Molecular Mechanisms of ROS Regulation in Cancer Cells. Biomolecules 2020;10:361. [Crossref] [PubMed]
- Zhang Y, Han CY, Duan FG, et al. p53 sensitizes chemoresistant non-small cell lung cancer via elevation of reactive oxygen species and suppression of EGFR/PI3K/AKT signaling. Cancer Cell Int 2019;19:188. [Crossref] [PubMed]
- Vigneron A, Vousden KH. p53, ROS and senescence in the control of aging. Aging (Albany NY) 2010;2:471-4. [Crossref] [PubMed]
- Johnson TM, Yu ZX, Ferrans VJ, et al. Reactive oxygen species are downstream mediators of p53-dependent apoptosis. Proc Natl Acad Sci U S A 1996;93:11848-52. [Crossref] [PubMed]
- Meek DW. Regulation of the p53 response and its relationship to cancer. Biochem J 2015;469:325-46. [Crossref] [PubMed]
- Ostrakhovitch EA, Cherian MG. Role of p53 and reactive oxygen species in apoptotic response to copper and zinc in epithelial breast cancer cells. Apoptosis 2005;10:111-21. [Crossref] [PubMed]
- Liu B, Chen Y, St Clair DK. ROS and p53: a versatile partnership. Free Radic Biol Med 2008;44:1529-35. [Crossref] [PubMed]
- Sablina AA, Budanov AV, Ilyinskaya GV, et al. The antioxidant function of the p53 tumor suppressor. Nat Med 2005;11:1306-13. [Crossref] [PubMed]
- Chen Y, Liu K, Shi Y, et al. The tango of ROS and p53 in tissue stem cells. Cell Death Differ 2018;25:639-41. [Crossref] [PubMed]
- Xue W, Chen S, Yin H, et al. CRISPR-mediated direct mutation of cancer genes in the mouse liver. Nature 2014;514:380-4. [Crossref] [PubMed]
- Sharma G, Attri SV, Behra B, et al. Analysis of 26 amino acids in human plasma by HPLC using AQC as derivatizing agent and its application in metabolic laboratory. Amino Acids 2014;46:1253-63. [Crossref] [PubMed]
- Li Q, Zhang L, Xu Y. Label-free LC-MS/MS proteomics analyses reveal proteomic changes in oxidative stress and the SOD antioxidant strategy in TM cells. Clin Proteomics 2022;19:12. [Crossref] [PubMed]
- Wang M, You J, Bemis KG, et al. Label-free mass spectrometry-based protein quantification technologies in proteomic analysis. Brief Funct Genomic Proteomic 2008;7:329-39. [Crossref] [PubMed]
- Cox J, Matic I, Hilger M, et al. A practical guide to the MaxQuant computational platform for SILAC-based quantitative proteomics. Nat Protoc 2009;4:698-705. [Crossref] [PubMed]
- Cox J, Hein MY, Luber CA, et al. Accurate proteome-wide label-free quantification by delayed normalization and maximal peptide ratio extraction, termed MaxLFQ. Mol Cell Proteomics 2014;13:2513-26. [Crossref] [PubMed]
- Traverso N, Ricciarelli R, Nitti M, et al. Role of glutathione in cancer progression and chemoresistance. Oxid Med Cell Longev 2013;2013:972913. [Crossref] [PubMed]
- Harris IS, Treloar AE, Inoue S, et al. Glutathione and thioredoxin antioxidant pathways synergize to drive cancer initiation and progression. Cancer Cell 2015;27:211-22. [Crossref] [PubMed]
- Schnelldorfer T, Gansauge S, Gansauge F, et al. Glutathione depletion causes cell growth inhibition and enhanced apoptosis in pancreatic cancer cells. Cancer 2000;89:1440-7. [Crossref] [PubMed]
- Kwon DH, Cha HJ, Lee H, et al. Protective Effect of Glutathione against Oxidative Stress-induced Cytotoxicity in RAW 264.7 Macrophages through Activating the Nuclear Factor Erythroid 2-Related Factor-2/Heme Oxygenase-1 Pathway. Antioxidants (Basel) 2019;8:82. [Crossref] [PubMed]
- Teskey G, Abrahem R, Cao R, et al. Glutathione as a Marker for Human Disease. Adv Clin Chem 2018;87:141-59. [Crossref] [PubMed]
- Liebl MC, Hofmann TG. The Role of p53 Signaling in Colorectal Cancer. Cancers (Basel) 2021;13:2125. [Crossref] [PubMed]
- Michel M, Kaps L, Maderer A, et al. The Role of p53 Dysfunction in Colorectal Cancer and Its Implication for Therapy. Cancers (Basel) 2021;13:2296. [Crossref] [PubMed]
- Tajan M, Hock AK, Blagih J, et al. A Role for p53 in the Adaptation to Glutamine Starvation through the Expression of SLC1A3. Cell Metab 2018;28:721-736.e6. [Crossref] [PubMed]
- Eriksson SE, Ceder S, Bykov VJN, et al. p53 as a hub in cellular redox regulation and therapeutic target in cancer. J Mol Cell Biol 2019;11:330-41. [Crossref] [PubMed]
- Hu W, Zhang C, Wu R, et al. Glutaminase 2, a novel p53 target gene regulating energy metabolism and antioxidant function. Proc Natl Acad Sci U S A 2010;107:7455-60. [Crossref] [PubMed]
- Levine AJ, Oren M. The first 30 years of p53: growing ever more complex. Nat Rev Cancer 2009;9:749-58. [Crossref] [PubMed]
- Cui F, Wang S, Tan J, et al. Calcium-binding and coiled-coil domain 2 promotes the proliferation and suppresses apoptosis of prostate cancer cells. Exp Ther Med 2021;21:405. [Crossref] [PubMed]
- Zhang J, Hu S, Li Y. KRT18 is correlated with the malignant status and acts as an oncogene in colorectal cancer. Biosci Rep 2019;39:BSR20190884. [Crossref] [PubMed]
- Liu D, Yin B, Wang Q, et al. Cytoplasmic poly(A) binding protein 4 is highly expressed in human colorectal cancer and correlates with better prognosis. J Genet Genomics 2012;39:369-74. [Crossref] [PubMed]
- Lan Y, Yang X, Wei Y, et al. Explore Key Genes and Mechanisms Involved in Colon Cancer Progression Based on Bioinformatics Analysis. Appl Biochem Biotechnol 2024; Epub ahead of print. [Crossref] [PubMed]
- Matsumoto Y, Saito M, Saito K, et al. Enhanced expression of KIF4A in colorectal cancer is associated with lymph node metastasis. Oncol Lett 2018;15:2188-94. [PubMed]